ScholarWorks at University of Montana
Home > The Mathematics Enthusiast > Vol. 2 > No. 2 (2005)

Problem Solving and Problem Posing in a Dynamic Geometry Environment
Constantinos Christou Nicholas Mousoulides Marios Pittalis Demetra Pitta-Pantazi
In this study, we considered dynamic geometry software (DGS) as the tool that mediates students’ strategies in solving and posing problems. The purpose of the present study was twofold. First, to understand the way in which students can solve problems in the setting of a dynamic geometry environment, and second, to investigate how DGS provides opportunities for posing new problems. Two mathematical problems were presented to six pre-service teachers with prior experience in dynamic geometry. Each student participated in two interview sessions which were video recorded. The results of the study showed that DGS, as a mediation tool, encouraged students to use in problem solving and posing the processes of modeling, conjecturing, experimenting and generalizing. Furthermore, we found that DGS can play a significant role in engendering problem solving and posing by bringing about surprise and cognitive conflict as students use the dragging and measuring facilities of the software.
Recommended Citation
Christou, Constantinos; Mousoulides, Nicholas; Pittalis, Marios; and Pitta-Pantazi, Demetra (2005) "Problem Solving and Problem Posing in a Dynamic Geometry Environment," The Mathematics Enthusiast : Vol. 2 : No. 2 , Article 6. DOI: https://doi.org/10.54870/1551-3440.1029 Available at: https://scholarworks.umt.edu/tme/vol2/iss2/6
Digital Object Identifier (DOI)
10.54870/1551-3440.1029
Since April 21, 2015
Included in
Mathematics Commons
- Journal Home
- Aims & Scope
- Editorial Board
- TME - 2023 Circulation Statistics
- TME - 2022 Circulation Statistics
- TME - 2021 Circulation Statistics
- Most Popular Papers
- Receive Email Notices or RSS
Advanced Search
ISSN: 1551-3440
Home | About | FAQ | My Account | Accessibility Statement
Privacy Copyright
About UM | Accessibility | Administration | Contact UM | Directory | Employment | Safety
- DOI: 10.1007/978-1-4614-6258-3_19
- Corpus ID: 57404205
Problem-Posing Activities in a Dynamic Geometry Environment: When and How
- Published 2015
- Education, Computer Science, Mathematics
8 Citations
Opportunities to pose problems using digital technology in problem solving environments..
- Highly Influenced
- 12 Excerpts
A Model Design To Be Used In Teaching Problem Posing To Develop Problem-Posing Skills
Comparison of prospective mathematics teachers’ problem posing abilities in paper-pencil test and on dynamic geometry environment in terms of creativity, the effectiveness of ‘what if not’ strategy coupled with dynamic geometry software in an inquiry-based geometry classroom, analysis of the problems posed by pre-service primary school teachers in terms of type, cognitive structure and content knowledge, exploring engineering undergraduate students’ attitudes toward mathematical problem posing, tecnología digital y formulación de problemas durante el proceso de resolución de problemas = digital technology and formulation of problems during the process of solving problems., learning to teach through problem posing: a teacher’s journey in a networked teacher−researcher partnership, 40 references, engaging in problem posing activities in a dynamic geometry setting and the development of prospective teachers’ mathematical knowledge, problem posing via “what if not” strategy in solid geometry — a case study.
- Highly Influential
The Mathematics Enthusiast The Mathematics Enthusiast
Posing mathematical problems: an exploratory study., on development of students' abilities in problem posing: a case of plan geometry, probing adults' conceptual understanding and transfer of learning via problem posing, problem posing: an opportunity for increasing student responsibility., prospective teachers’ skills in problem posing with regard to different problem posing models, what makes a problem mathematically interesting inviting prospective teachers to pose better problems, to see or not to see: analyzing difficulties in geometry from the perspective of visual perception, related papers.
Showing 1 through 3 of 0 Related Papers
Advertisement
Delving into the Nature of Problem Solving Processes in a Dynamic Geometry Environment: Different Technological Effects on Cognitive Processing
- Original Research
- Published: 02 June 2016
- Volume 22 , pages 37–64, ( 2017 )
Cite this article
- Ana Kuzle 1
960 Accesses
10 Citations
2 Altmetric
Explore all metrics
Students regularly struggle with mathematical tasks, particularly those concerning non-routine problems in geometry. Although educators would like for their learners to transfer their knowledge to non-routine and real-life situations, students run into a number of difficulties. The goal of this exploratory study was to analyze three participants’ problem solving processes in a dynamic geometry software (DGS), and therefore, gain insights about how DGS was used to support solving non-routine geometry problems. Here I viewed the DGS as a cognitive tool that can enhance and reorganize the problem solving process. The three participants were in different phases of their educational career in mathematics and/or mathematics education (bachelor, master, and doctoral student). Only one problem—the Land Boundary Problem—from the TIMSS video study will be discussed here. In this problem, the participants had to straighten a bent fence between two farmers’ land so that each farmer would keep the same amount of land. All three participants solved the problem, but used the same computer-based problem-solving tool differently. While a DGS allowed and supported some participants to discover new methods of thinking, and unanticipated ways of using it, it also inhibited the problem solving processes through development of tool-dependency by some. Its different use was dependent on the presence of managerial decisions, ability to manage different resources, and problem solving experience. Based on these findings, I make recommendations for technology-embedded problem solving with an emphasis on the importance of appropriate tool use in educational settings and offer some teaching methods that may be worthwhile for research.
This is a preview of subscription content, log in via an institution to check access.
Access this article
Subscribe and save.
- Get 10 units per month
- Download Article/Chapter or eBook
- 1 Unit = 1 Article or 1 Chapter
- Cancel anytime
Price includes VAT (Russian Federation)
Instant access to the full article PDF.
Rent this article via DeepDyve
Institutional subscriptions
Similar content being viewed by others
Problem-Posing Activities in a Dynamic Geometry Environment: When and How
High School Teachers’ Use of a Dynamic Geometry System to Formulate Conjectures and to Transit from Empirical to Geometric and Algebraic Arguments in Problem-Solving Approaches
Mathematical problem solving with technology: the techno-mathematical fluency of a student-with-geogebra.
On the market many different DGS are available, such as Geometer’s Sketchpad (GSP), GeoGebra, Cinderella and Cabri.
In this study, I used the well-received interactive geometry software, the Geometer’s Sketchpad (GSP). I use the term DGS throughout the paper, because the GSP possesses typical features of a DGS. For that reason, the results are transferable to any DGS.
When the problem did not get solved within 90 min, I stopped the session and went onto the second part of the session. The participants solved the problem in the next session, which was then again followed by a semi-structured interview. This occurred only once.
By applying the working backwards strategy, students find the solution to a problem by starting with the answer and using inverse operations to undo the steps stated in the problem (Pólya 1945/ 1973 ).
Wandering dragging, that is moving the basic points on the screen more or less randomly, without a plan, in order to discover interesting configurations or regularities in the figures.
Arzarello, F., Micheletti, C., Olivero, F., Robutti, O., Paola, D., & Gallino, G. (1998). Dragging in Cabri and modalities of transition from conjectures to proofs in geometry. In A. Olivier & K. Newstead (Eds.), Proceedings of the 22nd annual conference of the international group for the psychology of mathematics education (Vol. 2, pp. 32–39). Stellenbosch.
Bereiter, C., & Scardamalia, M. (1987). The psychology of written composition . Hillsdale, NJ: Erlbaum.
Google Scholar
Berg, B. L. (2007). Qualitative research methods for the social sciences (6th ed.). Boston, MA: Pearson.
Bransford, J. D., Brown, A. L., & Cocking, R. R. (2004). How people learn. Brain, mind, experience, and school . Washington, DC: National Academy Press.
Brown, A. L. (1987). Metacognition, executive control, self regulation and other more mysterious mechanisms. In F. E. Weinert & R. H. Kluwe (Eds.), Metacognition, motivation and understanding (pp. 65–116). Hillsdale, NJ: Erlbaum.
Carlson, M. P. (1999). The mathematical behavior of six successful mathematics graduate students: Influences leading to mathematical success. Educational Studies in Mathematics, 40 (3), 237–258.
Article Google Scholar
Carlson, M. P., & Bloom, I. (2005). The cycle nature of problem solving: An emergent multidimensional problem-solving framework. Educational Studies in Mathematics, 58 , 45–75.
Dörfler, W. (1991). Der Computer als kognitives Werkzeug und kognitives Medium [Computer as a cognitive tool and a cognitive medium]. In W. Dörfler, W. Peschek, E. Schneider, & K. Wegenkittl (Eds.), Computer - Mensch - Mathematik (pp. 51–76). Wien, Stuttgart: Hölder-Pichler-Tempsky; B.G.Teubner.
Ericsson, K. A., & Simon, H. A. (1993). Protocol analysis: Verbal reports as data (Rev. ed.) . Cambridge, MA: MIT Press.
Fey, J. T., Hollenbeck, R. M., & Wray, J. A. (2010). Technology and the mathematics curriculum. In B. J. Reys, R. E. Reys, & R. Rubenstein (Eds.), Mathematics curriculum: Issues, trends, and future directions (pp. 41–49). Reston, VA: National Council of Teachers of Mathematics.
Flavell, J. H. (1976). Metacognition aspects of problem solving. In L. B. Resnick (Ed.), The nature of intelligence (pp. 231–236). Hillsdale, NJ: Erlbaum.
Flavell, J. H. (1981). Cognitive monitoring. In W. P. Dickson (Ed.), Children’s oral communication skills (pp. 35–60). New York, NY: Academic Press.
Garofalo, J., & Lester, F. K. (1985). Metacognition, cognitive monitoring, and mathematical performance. Journal for Research in Mathematics Education, 16 , 163–176.
Goldhammer, F., Naumann, J., Stelter, A., Toth, K., Rölke, H., & Kleme, E. (2014). The time on task effect in reading and problem solving is moderated by task difficulty and skill: Insights from a computer-based large-scale assessment. Journal of Educational Psychology, 106 (3), 608–626.
Haug, R. (2012). Problemlösen lernen mit digitalen Medien. Förderung grundlegender Problemlösetechniken durch den Einsatz dynamischer Werkzeuge [Problem solving learning with digital media. Promoting the fundamental problem-solving techniques through the use of dynamic tools]. Wiesbaden: Vieweg + Teubner Verlag.
Herrera, M., Preiss, R., & Riera, G. (2008, July). Intellectual amplification and other effects “with,” “of” and “through” technology in teaching and learning mathematics . Paper presented at the Meeting of the International Congress of Mathematics Instruction. Monterrey, Mexico. Retrieved February 13, 2014, from dg.icme11.org/document/get/76 .
Hollebrands, K. F. (2007). The role of a dynamic software program for geometry in the strategies high school mathematics students employ. Journal for Research in Mathematics Education, 38 (2), 164–192.
Hölzl, R. (2001). Using dynamic geometry software to add contrast to geometric situations—A case study. International Journal of Computers for Mathematical Learning, 6 , 63–86.
Hoyles, C., & Noss, R. (2003). What can digital technologies take from and bring to research in mathematics education? In A. J. Bishop, M. A. Clemens, C. Keitel, J. Kilpatrick, & F. K. S. Leung (Eds.), Second international handbook of mathematics education (pp. 323–349). Dordrecht: Kluwer Academic.
Chapter Google Scholar
Kantowski, M. G. (1977). Processes involved in mathematical problem solving. Journal for Research in Mathematics Education, 8 (3), 163–180.
Kim, B., & Reeves, T. C. (2007). Reframing research on learning with technology: In search of the meaning of cognitive tools. Instructional Science, 35 (3), 207–256.
Kultusministerkonferenz. (2003). Bildungsstandards im Fach Mathematik für den mittleren Schulabschluss [Educational standards for the middle school] . Bonn: KMK.
Kuzle, A. (2011). Preservice teachers’ patterns of metacognitive behavior during mathematics problem solving in a dynamic geometry environment . Doctoral dissertation. University of Georgia–Athens, GA.
Kuzle, A. (2013). Patterns of metacognitive behavior during mathematics problem-solving in a dynamic geometry environment. International Electronic Journal of Mathematics Education , 8 (1), 20–40.
Kuzle, A. (2015). Nature of metacognition in a dynamic geometry environment. LUMAT – Research and Practice in Math, Science and Technology Education , 3 (5), 627–646.
Laborde, C. (2000). Dynamic geometry environments as a source of rich learning contexts for the complex activity of proving. Educational Studies in Mathematics, 44 (1), 151–161.
Laborde, C., Kynigos, C., Hollebrands, K., & Sträßer, R. (2006). Teaching and learning geometry with technology. In A. Gutierrez & P. Boero (Eds.), Handbook of research on the psychology of mathematics education: Past, present and future (pp. 275–304). Rotterdam: Sense.
Lajoie, S. P. (Ed.). (2000). Computers as cognitive tools: No more walls (Vol. 2). Mahwah, NJ: Erlbaum.
Lajoie, S. P. (2005). Cognitive tools for the mind: The promises of technology: Cognitive amplifiers or bionic prosthetics? In R. J. Sternberg & D. Preiss (Eds.), Intelligence and technology: Impact of tools on the nature and development of human skills (pp. 87–102). Mahwah, NJ: Erlbaum.
National Council of Teachers of Mathematics. (2000). Principles and standards for school mathematics . Reston, VA: National Council of Teachers of Mathematics.
National Council of Teachers of Mathematics. (2005). Technology-supported mathematics learning environments . Reston, VA: National Council of Teachers of Mathematics.
Olive, J., & Makar, K. (2010). Mathematical knowledge and practices resulting from access to digital technologies. In C. Hoyles & J.-B. Lagrange (Eds.), Mathematics education and technology—Rethinking the terrain. The 17th ICMI Study (pp. 133–177). New York, NY: Springer.
Patton, M. Q. (2002). Qualitative research and evaluation methods . Thousand Oaks, CA: Sage.
Pea, R. D. (1985). Beyond amplification: Using the computer to recognize mental functioning. Educational Psychologist, 20 (4), 167–182.
Pólya, G. (1973). How to solve it: A new aspect of mathematical method . Princeton, NJ: Princeton University Press. (Original work published 1945).
Proske, A., Damnik, G., & Körndle, H. (2011). Learners-as-Designers: Wissensräume mit kognitiven Werkzeugen aktiv nutzen und konstruieren [Learners-as-designers: Active use and construction of knowledge spaces with cognitive tools]. In T. Köhler & J. Neumann (Eds.), Wissensgemeinschaften. Digitale Medien - Öffnung und Offenheit in Forschung und Lehre (pp. 198–208). Münster: Waxmann.
Salomon, G., & Perkins, D. (2005). Do technologies make us smarter? Intellectual amplification with, of, and through technology. In R. J. Sternberg & D. D. Preiss (Eds.), Intelligence and technology: The impact of tools on the nature and development of human abilities (pp. 71–86). Mahwah, NJ: Erlbaum.
Salomon, G., Perkins, D. N., & Globerson, T. (1991). Partners in cognition: Extending human intelligence with intelligent technologies. Educational Researcher, 20 (3), 2–9.
Schoenfeld, A. H. (1981). Episodes and executive decisions in mathematical problem solving (ERIC Documentation Reproduction Service No. ED201505). Paper presented at the Annual meeting of the American educational research association, Los Angeles, CA.
Schoenfeld, A. H. (1985). Mathematical problem solving . Orlando, FL: Academic Press.
Schoenfeld, A. H. (1987). What’s all the fuss about metacognition? In A. H. Schoenfeld (Ed.), Cognitive science and mathematics education (pp. 189–215). Hillsdale, NJ: Erlbaum.
Schoenfeld, A. H. (1992). Learning to think mathematically: Problem solving, metacognition, and sense-making in mathematics. In D. Grouws (Ed.), Handbook of research on mathematics teaching and learning (pp. 334–370). New York, NY: Macmillan.
Tall, D. (1989). Concepts images, generic organizers, computers and curriculum change. For the Learning of Mathematics, 9 (3), 37–42.
Wilson, J., & Clarke, D. (2004). Towards the modelling of mathematical metacognition. Mathematics Education Research Journal, 16 (2), 25–48.
Wilson, J. W., Fernandez, M. L., & Hadaway, N. (1993). Mathematical problem solving. In P. S. Wilson (Ed.), Research ideas for the classroom: High school mathematics (pp. 57–78). New York, NY: Macmillan.
Zbiek, R. M., Heid, M. K., Blume, G. W., & Dick, T. (2007). Research on technology in mathematics education: A perspective of constructs. In F. Lester (Ed.), Second handbook of research on mathematics teaching and learning (pp. 1169–1207). Charlotte, NC: Information Age.
Zimmerman, B. J. (2002). Becoming a self-regulated learner: An overview. Theory Into Practice, 41 (2), 64–70.
Download references
Author information
Authors and affiliations.
University of Potsdam, Karl-Liebknecht-Str. 24-25, 14476, Potsdam, Germany
You can also search for this author in PubMed Google Scholar
Corresponding author
Correspondence to Ana Kuzle .
Appendix 1: Macroscopic Episodes of the Framework for Analyzing Students’ Problem Solving Processes in DGS with Descriptors
Episode | Description of episodes with possible problem solving processes |
---|---|
Reading episode | Student reads the problem statement silently or aloud (instance of doing). That person can, in addition, engage in monitoring and control strategies to avoid missteps in this early stage of the problem solving process (metacognitive). |
Understanding episode | The problem solver engages in behaviors attending to clarifying the meaning of the problem or making comments about it. For instance, that person may note conditions of the problem, state the goals of the problem, represent the problem (draw a diagram), and assess the current knowledge relative to the task. In other words, the problem solver engages in various both cognitive and metacognitive processes that reflect attempts to make sense of the problem. |
Analysis episode | If there is no apparent way to proceed, the problem solver may engage in coherent, well-structured and reasonable actions that are closely related to the conditions or goals of the problem. In it, that person attempts to fully understand the problem, decomposes the problem in its basic elements, or simplifies or reformulates it (cognitive), examines the relationships between the given information, conditions and the goals of the problem, and chooses and evaluates appropriate perspectives to solve the problem using different appropriate strategies to assist that person (metacognitive) (e.g., examine special cases, reduces the number of constraints). |
Exploration episode | The exploration episode is less well structured and further removed from the given problem (broad tour through the problem space). The problem solver searches for relevant information that can be incorporated into the analysis, planning, and implementation sequence relying on the previous knowledge and experience. In it a problem solver may find, for instance a variety of heuristics, the examination of related problems, analogies. Since exploration is weakly structured, monitoring and regulatory activities are important for keeping the exploration focused and controlled (metacognitive). Otherwise, lengthy unproductive problem solving (so called “wild goose chase”) will occur or even promising alternative(s) can get dismissed (cognitive). |
Planning episode | During the planning episode, the behaviors pertaining to concretely solving the problem are made. In other words, the student creates a plan, i.e. selects steps and strategies that may lead to problem solution, which then gets implemented. However, such behaviors can be well thought out, evaluated, and therefore focused (metacognitive). But also the steps and strategies can be made without careful attention to their productivity or appropriateness (cognitive). |
Implementation episode | The plan gets implemented on the basis of the previous episodes. Hence, here the givens get transformed into the goals of the problems (cognitive). However, metacognitive decisions (monitoring the process, evaluating considered decisions, regulating the steps to be implemented) are crucial to avoid faulty steps. |
Verification episode | The student reviews and tests whether the solution passes specific or general tests in relation to problem requirements (e.g., redoing the steps (cognitive); evaluating the reasonableness of the solution (metacognitive)). |
Observation-interaction sub-episode | This sub-episode can be embedded within any episode and denotes non-verbal interaction between the user and the technology (here DGS). Here a distinction between static and dynamic behaviors was made. Under static behavior I understand such behaviors that do not involve any tacit use of technology, but those such as observing the screen. On the other hand, dynamic behaviors denote such behaviors in which activities are tacit. Such behaviors include but are not limited to, dragging, using measuring and construction tools, using locus and trace function. Observation-interaction behaviors can subsequently prompt different cognitive and metacognitive behaviors within the episode or a new episode. |
Transition | Transition is a junction between the other episodes and occurs only when a student assesses the current solution state and makes decisions about pursuing a new direction to solve the problem. According to Schoenfeld ( ), this is the episode where managerial decisions or their absence “will make or break a solution” (p. 26). |
Appendix 2: Non-routine Geometry Problems
2.1 problem 1: the longest segment problem.
Given two intersecting circles. Draw a line through one of the intersection points, say, A. That line also intersects circles in exactly two points, say, B and C. What choice of the point B results in the segment BC such that the segment BC is the longest?
Formulate and prove your conjecture.
Find the construction for a point B such that the length of BC in the longest.
Justify your answers as best as you can.
2.2 Problem 2: Inscribed Square
Given an acute triangle. Develop a Euclidean construction for inscribing a square in the triangle such that two of its vertices are on the base of the triangle and the other two vertices are on the other two sides of the triangle.
2.3 Problem 3: The Airport Problem
Three towns, Athens, Bogart and Columbus, are equally distant from each other and connected by straight roads. An airport will be constructed such that the sum of its distances to the roads is as small as possible.
What are possible locations for the airport?
What is the best location for the airport?
Give a geometric interpretation for the sum of the distances of the optimal point to the sides of the triangle.
2.4 Problem 4: The Treasure Island Problem
Among his great-grandfather’s papers, Marco found a parchment describing the location of a pirate treasure buried on a deserted island. The island contained a pine three, an oak tree, and a gallows where traitors were hung. The parchment was accompanied by the following directions:
Walk from the gallows to the pine tree, counting the number of steps. At the pine tree, turn 90° to the right. Walk the same distance and put a spike in the ground. Return to the gallows ad walk to the oak tree, counting your steps. At the oak tree, turn 90° to the left, walk the same number of steps, and put another spike in the ground. The treasure is halfway between the spikes.
Marco found the island and the two trees but could not find no trace of the gallows or the spikes, as both had probably rotted. In desperation, he began to dig at random but soon gave up because the island was too large. Your quest is to devise a plan to find the exact location of the treasure.
2.5 Problem 5: The Land Boundary Problem
The boundary between two farmers’ land is bent, and they would both like to straighten it out, but each wants to keep the same amount of land. Solve the problem for them.
What if the common border has three segments? Justify your answers as best as you can.
Appendix 3: A Priori Analysis of Possible Problem Solving Processes and Technology Use
Here I outline several approaches that could be used to solve the Land Boundary Problem in the light of the outlined framework.
3.1 Episode 1: Reading the Problem (Cognitive and Metacognitive)
The participants read the problem (cognitive). However, if one reads the problem too fast or fails to note relevant information, it is unlikely to result in a solution. On the other hand, one may read statements slowly, marking relevant words, such as “keep the same amount” or “straighten out”, and/or making the connections between the problem representation and the text. Thus, different controlling strategies (metacognitive) may be employed, which can be executed also with editing tools of DGS.
3.2 Episode 2: Understanding the Problem (Metacognitive)
The participants need to understand the given, the goals, and the conditions that must be met when solving the problem (sense making).
The bended boundary must be straightened.
The areas of the two lands must not change.
Also they may use construction tools of DGS to add different auxiliary lines to better understand the problem.
3.3 Episode 3: Analyzing the Problem (Cognitive and Metacognitive)
In this episode the participants focus on establishing connections within the given problem (givens, conditions, goals). In order to do so, the participants need to decompose the problem into its basic structure (cognitive), find relationships between the givens and the goals on the basis of previous knowledge relevant to the problem and consider approaches that might be appropriate for solving the problem (metacognitive). Thus, they evaluate the relevancy of chosen approaches and consequences for the following steps.
The problem can be reformulated that the bounded fence has to be straightened with areas of the two lands staying the same.
The bended fence edges could be connected and with it a triangle is built.
Because area is a function of one side and an altitude on that side, the participants need to think about how construct a triangle with the same area but with one side lying on the land boundary.
3.4 Episode 4: Exploring the Problem (Cognitive and Metacognitive)
The participants engage in a search for information relevant to the problem that can be later on used. For instance, the participant, by using DGS, can make a series of random guesses as to where the border can be constructed and then with repeated editing try to find an approach to reconstruct it. Here however, the guess is not based on a thoughtful hypothesis, but on random guesses. Such actions are unmonitored and not evaluated, and will likely lead to unproductive actions and will not result in a solution (cognitive). On the other hand, if the participant is altering the givens of the problem (e.g., moving the borders, or the fence point) to gain more information and making well thought guesses, testing them, and refining them to meet the goals, while also monitoring and regulating thoughts, then the exploration will lead to a productive effort (metacognitive). Thus, through dragging modalities of DGS, the participant can engage in a systematic trial-and-error-analyze strategy. Based on the feedback through visualization of undertaken activities, the student can engage in other behaviors, such as to better understand the problem or to gain more information, or proceed to the planning episode.
3.5 Episode 5: Planning the Problem (Cognitive and Metacognitive)
There are many ways that a participant may attempt to solve the problem.
One can decide to work backwards—draw border using technological affordances of the tool such that the goals of the problem are met.
One can decide to work forwards and try to construct the new border on the basis of mathematical knowledge. Here, drawing a parallel to the base side of a triangle will lead to a solution of the problem—any of the two intersections of this parallel line with the land boundaries allows constructing a new straight fence.
Depending on the quality of the thought processes, the behaviors can be either of cognitive or metacognitive nature.
3.6 Episode 6: Implementing the problem (Cognitive and Metacognitive)
Once a plan is devised, the participants will try to implement it. When the execution of steps is sequential and monitored, this might lead to a solution or revelation that the plan was not good and because of it, they may devise a new plan (metacognitive). When implementation is not monitored, the participant may get buried in the implementation of a not well-thought out plan that is unlikely to result in a solution (cognitive). These behaviors can be easily executed using construction tools of DGS.
3.7 Episode 7: Verifying the Solution (Cognitive and Metacognitive)
The participants may verify the plan by using the measure area function of the software (cognitive) or use mathematical reasoning and ability to monitor the results to check whether the conditions of the problem were met (metacognitive).
Rights and permissions
Reprints and permissions
About this article
Kuzle, A. Delving into the Nature of Problem Solving Processes in a Dynamic Geometry Environment: Different Technological Effects on Cognitive Processing. Tech Know Learn 22 , 37–64 (2017). https://doi.org/10.1007/s10758-016-9284-x
Download citation
Published : 02 June 2016
Issue Date : April 2017
DOI : https://doi.org/10.1007/s10758-016-9284-x
Share this article
Anyone you share the following link with will be able to read this content:
Sorry, a shareable link is not currently available for this article.
Provided by the Springer Nature SharedIt content-sharing initiative
- Cognitive tool
- Dynamic geometry software
- Mathematical problem solving
- Metacognition
- Non-routine geometry problems
- Teacher education
- Find a journal
- Publish with us
- Track your research
Construct Dynamic Geometry Together
Table of contents, before you construct together.
- Stick Figures
- Dynamic Points, Lines and Circles
Construct Dynamic-Geometry Objects
- The Drag Test
- Dependent Geometric Figures
- Constructing Polygons
- Construct an Equilateral Triangle
- Find Dynamic Triangles
Construct Using Circles
- Construct the Midpoint
- Construct a Perpendicular Line
- Construct a Parallel Line
Construct Other Triangles
- Triangles with Dependencies
- An Isosceles Triangle
- A Right Triangle
- An Isosceles-Right Triangle
Construct Using the Compass
- Copy a Length
- Use the Compass Tool
- Make Dependent Segments
- Add Segment Lengths
- Copy vs. Construct a Congruent Triangle
- Construct a Congruent Angle
Construct Inscribed Polygons
- The Inscribed Triangles Challenge Problem
- The Inscribed Quarilaterals Challenge Problem
- Prove Inscribed Triangles
Construct Congruent Triangles
- Combinations of Sides and Angles of Congruent Triangles
- Side-Side-Side (SSS)
- Side-Angle-Side (SAS)
- Angle-Side-Angle (ASA)
- Side-Side-Angle (SSA)
Construct using Transformations
- Translate by a Vector
- Reflect about a Line
- Rotate Around a Point
- Combine Transformations
- Create Dynamic Patterns

Construct Quadrilaterals
- Construct Quadrilaterals with Special Constraints
- Construct a Rhombus
- Quadrilateral Areas
- Build a Hierarchy of Quadrilaterals
Advanced Constructions
- The Centroid of a Triangle
- The Circumcenter of a Triangle
- The Orthocenter of a Triangle
- The Incenter of a Triangle
- The Euler Segment of a Triangle
- The Nine-Point Circle of a Triangle
Solve Construction Problems
- Treasure Hunt
- Square and Circle
- Cross an Angle
- How Many Ways Can You Invent?
- Dependencies in the World
- Into the Future
New Resources
- A retro cityscape with mathematics
- Ball Structure 2
- Parallel or Not? (V2)
- aMazing Patterns
- Odd or Even
Discover Resources
- bleistift-komplettsimulation
- Incenter Christian Jax Murray
- Circle Theorems: Angles of Cyclic Quadrilateral
- p1a2arancha
Discover Topics
- Equilateral Triangles
- Quadratic Functions
- Exponential Functions
- Translation
- Incircle or Inscribed Circle
Reset password New user? Sign up
Existing user? Log in
Dynamic Programming
Already have an account? Log in here.
- Karleigh Moore
- Norbert Madarász
Dynamic programming refers to a problem-solving approach, in which we precompute and store simpler, similar subproblems, in order to build up the solution to a complex problem. It is similar to recursion , in which calculating the base cases allows us to inductively determine the final value. This bottom-up approach works well when the new value depends only on previously calculated values.
An important property of a problem that is being solved through dynamic programming is that it should have overlapping subproblems. This is what distinguishes DP from divide and conquer in which storing the simpler values isn't necessary.
To show how powerful the technique can be, here are some of the most famous problems commonly approached through dynamic programming:
- Backpack Problem : Given a set of treasures with known values and weights, which of them should you pick to maximize your profit whilst not damaging your backpack which has a fixed capacity?
- Egg Dropping : What is the best way to drop \(n\) eggs from an \(m\)-floored building to figure out the lowest height from which the eggs when dropped crack?
- Longest Common Subsequence : Given two sequences, which is the longest subsequence common to both of them?
- Subset Sum Problem : Given a set and a value \(n,\) is there a subset the sum of whose elements is \(n?\)
- Fibonacci Numbers : Is there a better way to compute Fibonacci numbers than plain recursion?
In a contest environment, dynamic programming almost always comes up (and often in a surprising way, no matter how familiar the contestant is with it).
Motivational Example: Change of Coins
Recursion with memoization, bidimensional dynamic programming: example, example: maximum paths.
What is the minimum number of coins of values \(v_1,v_2, v_3, \ldots, v_n\) required to amount a total of \(V?\) You may use a denomination more than once.
Optimal Substructure
The most important aspect of this problem that encourages us to solve this through dynamic programming is that it can be simplified to smaller subproblems.
Let \(f(N)\) represent the minimum number of coins required for a value of \(N\).
Visualize \(f(N)\) as a stack of coins. What is the coin at the top of the stack? It could be any of \(v_1,v_2, v_3, \ldots, v_n\). In case it were \(v_1\), the rest of the stack would amount to \(N-v_1;\) or if it were \(v_2\), the rest of the stack would amount to \(N-v_2\), and so on.
How do we decide which is it? Sure enough, we do not know yet. We need to see which of them minimizes the number of coins required.
Going by the above argument, we could state the problem as follows:
\[f(V) = \min \Big( \big\{ 1 + f(V - v_1), 1 + f(V-v_2), \ldots, 1 + f(V-v_n) \big \} \Big). \]
Because the coin at the top of the stack also counts as one coin, and then we can look at the rest.
Overlapping Subproblems
It is easy to see that the subproblems could be overlapping.
For example, if we are trying to make a stack of $11 using $1, $2, and $5, our look-up pattern would be like this: \[\begin{align} f(11) &= \min \Big( \big\{ 1+f(10),\ 1+ f(9),\ 1 + f(6) \big\} \Big) \\ &= \min \Big ( \big \{ 1+ \min {\small \left ( \{ 1 + f(9), 1+ f(8), 1+ f(5) \} \right )},\ 1+ f(9),\ 1 + f(6) \big \} \Big ). \end{align} \] Clearly enough, we'll need to use the value of \(f(9)\) several times.
One of the most important aspects of optimizing our algorithms is that we do not recompute these values. To do this, we compute and store all the values of \(f\) from 1 onwards for potential future use.
The recursion has to bottom out somewhere, in other words, at a known value from which it can start.
For this problem, we need to take care of two things:
Zero : It is clear enough that \(f(0) = 0\) since we do not require any coins at all to make a stack amounting to 0.
Negative and Unreachable Values : One way of dealing with such values is to mark them with a sentinel value so that our code deals with them in a special way. A good choice of a sentinel is \(\infty\), since the minimum value between a reachable value and \(\infty\) could never be infinity.
The Algorithm
Let's sum up the ideas and see how we could implement this as an actual algorithm:
def coinsChange(V,v): dpTable = [float("inf")]*(V+1) dpTable[0] = 0 for i in xrange(1,V+1): for vi in v: if (i - vi) >= 0: dpTable[i] = min(dpTable[i],1+dpTable[i-vi]) return dpTable[V] |
We have claimed that naive recursion is a bad way to solve problems with overlapping subproblems. Why is that? Mainly because of all the recomputations involved.
Another way to avoid this problem is to compute the data first time and store it as we go, in a top-down fashion.
Let's look at how one could potentially solve the previous coin change problem in the memoization way. 1 2 3 4 5 6 7 8 9 10 11 12 def coinsChange ( V , v ): memo = {} def Change ( V ): if V in memo : return memo [ V ] if V == 0 : return 0 if V < 0 : return float ( "inf" ) memo [ V ] = min ([ 1 + Change ( V - vi ) for vi in v ]) return memo [ V ] return Change ( V )
Dynamic Programming vs Recursion with Caching
\(\hspace{20mm}\) | |
Faster if many sub-problems are visited as there is no overhead from recursive calls | \(\hspace{20mm}\) Intuitive approach |
The complexity of the program is easier to see | \(\hspace{20mm}\) Computes only those subproblems which are necessary |
There are \(k\) types of brackets each with its own opening bracket and closing bracket. We assume that the first pair is denoted by the numbers 1 and \(k+1,\) the second by 2 and \(k+2,\) and so on. Thus the opening brackets are denoted by \(1, 2, \ldots, k,\) and the corresponding closing brackets are denoted by \(k+1, k+2, \ldots, 2k,\) respectively.
Some sequences with elements from \(1, 2, \ldots, 2k\) form well-bracketed sequences while others don't. A sequence is well-bracketed if we can match or pair up opening brackets of the same type in such a way that the following holds:
- Every bracket is paired up.
- In each matched pair, the opening bracket occurs before the closing bracket.
- For a matched pair, any other matched pair lies either completely between them or outside them.
In this problem, you are given a sequence of brackets of length \(N\): \(B[1], \ldots, B[N]\), where each \(B[i]\) is one of the brackets. You are also given an array of Values: \(V[1],\ldots, V[N] \).
Among all the subsequences in the Values array, such that the corresponding bracket subsequence in the B Array is a well-bracketed sequence, you need to find the maximum sum.
Task: Solve the above problem for this input.
Input Format
One line, which contains \((2\times N + 2)\) space separate integers. The first integer denotes \(N.\) The next integer is \(k.\) The next \(N\) integers are \(V[1],..., V[N].\) The last \(N\) integers are \(B[1],..., B[N].\)
Constraints
- \(1 \leq k \leq 7\)
- \(-10^6 \leq V[i] \leq 10^6\), for all \(i\)
- \(1 \leq B[i] \leq 2k\), for all \(i\)
Illustrated Examples
For the examples discussed here, let us assume that \(k = 2\). The sequence 1, 1, 3 is not well-bracketed as one of the two 1's cannot be paired. The sequence 3, 1, 3, 1 is not well-bracketed as there is no way to match the second 1 to a closing bracket occurring after it. The sequence 1, 2, 3, 4 is not well-bracketed as the matched pair 2, 4 is neither completely between the matched pair 1, 3 nor completely outside of it. That is, the matched pairs cannot overlap. The sequence 1, 2, 4, 3, 1, 3 is well-bracketed. We match the first 1 with the first 3, the 2 with the 4, and the second 1 with the second 3, satisfying all the 3 conditions. If you rewrite these sequences using [, {, ], } instead of 1, 2, 3, 4 respectively, this will be quite clear.
Suppose \(N = 6, k = 3,\) and the values of \(V\) and \(B\) are as follows: Then, the brackets in positions 1, 3 form a well-bracketed sequence (1, 4) and the sum of the values in these positions is 2 (4 + (-2) =2). The brackets in positions 1, 3, 4, 5 form a well-bracketed sequence (1, 4, 2, 5) and the sum of the values in these positions is 4. Finally, the brackets in positions 2, 4, 5, 6 form a well-bracketed sequence (3, 2, 5, 6) and the sum of the values in these positions is 13. The sum of the values in positions 1, 2, 5, 6 is 16 but the brackets in these positions (1, 3, 5, 6) do not form a well-bracketed sequence. You can check the best sum from positions whose brackets form a well-bracketed sequence is 13.
We'll try to solve this problem with the help of a dynamic program, in which the state , or the parameters that describe the problem, consist of two variables.
First, we set up a two-dimensional array dp[start][end] where each entry solves the indicated problem for the part of the sequence between start and end inclusive.
We'll try to think what happens when we run across a new end value, and need to solve the new problem in terms of the previously solved subproblems. Here are all the possibilities:
- When end <= start , there are no valid subsequences.
- When b[end] <= k , i.e, the last entry is an open bracket, no valid subsequence can end with it. Effectively, the result is the same if we hadn't included the last entry at all.
- When b[end] > k , i.e, the last entry is a closing bracket, one has to find the best match for it, or simply ignore it, whichever maximizes the sum.
Can you use these ideas to solve the problem?
Very often, dynamic programming helps solve problems that ask us to find the most profitable (or least costly) path in an implicit graph setting. Let us try to illustrate this with an example.
You are supposed to start at the top of a number triangle and chose your passage all the way down by selecting between the numbers below you to the immediate left or right. Your goal is to maximize the sum of the elements lying in your path. For example, in the triangle below, the red path maximizes the sum.
To see the optimal substructures and the overlapping subproblems , notice that everytime we make a move from the top to the bottom right or the bottom left, we are still left with smaller number triangle, much like this:
We could break each of the sub-problems in a similar way until we reach an edge-case at the bottom:
In this case, the solution is a + max(b,c) .
A bottom-up dynamic programming solution is to allocate a number triangle that stores the maximum reachable sum if we were to start from that position . It is easy to compute the number triangles from the bottom row onward using the fact that the
\[\text{best from this point} = \text{this point} + \max(\text{best from the left, best from the right}).\]
Let me demonstrate this principle through the iterations. Iteration 1: 1 8 5 9 3 Iteration 2: 1 2 10 13 15 8 5 9 3 Iteration 3: 1 2 3 20 19 10 13 15 8 5 9 3 Iteration 4: 1 2 3 4 23 20 19 10 13 15 8 5 9 3 So, the effective best we could do from the top is 23, which is our answer.
Problem Loading...
Note Loading...
Set Loading...
- The Big Think Interview
- Your Brain on Money
- Explore the Library
- The Universe. A History.
- The Progress Issue
- A Brief History Of Quantum Mechanics
- 6 Flaws In Our Understanding Of The Universe
- Michio Kaku
- Neil deGrasse Tyson
- Michelle Thaller
- Steven Pinker
- Ray Kurzweil
- Cornel West
- Helen Fisher
- Smart Skills
- High Culture
- The Present
- Hard Science
- Special Issues
- Starts With A Bang
- Everyday Philosophy
- The Learning Curve
- The Long Game
- Perception Box
- Strange Maps
- Free Newsletters
- Memberships
The big myth that keeps people from loving math

- There is no secret code or single method to solve a mathematical problem.
- This “answer-getting” myth leads to disempowerment when learning math.
- To counteract the myth, don’t focus on the answer but the process.
Teaching math as if there’s only one correct way to solve a problem makes us think that we’re problem-solving, but instead, we’re “answer-getting.” I’ve seen it so many times, but none bothers me more than watching elementary and middle school students solve word problems in this way.
Consider this typical middle school prompt: A store is selling 6 bags of marbles for $18. What is the unit price for a bag of marbles? When I read this problem, I picture a child looking up at me and asking, “Does ‘of’ mean multiplication?” It has happened to me so many times when I visit math classrooms.
There’s no secret code. Of could mean “multiply,” but it might not. These are the highly counterproductive questions that children ask when they have been presented with a “single way” to solve word problems, such as looking for keywords.
In this example, the students may immediately multiply 6 × 18. If you then ask them why the unit price of a single bag of marbles would cost $108 — and be so much more than the price of 6 bags of marbles — they will look at you with uncertainty. This is the end result of answer-getting.
Problem-solving is a distinct cognitive experience. Instead, we ask, what is happening in the problem? It is not mindlessly following a single prescriptive set of steps. The way to solve this problem, and every problem, is to understand what is happening. But that means there will be many paths to the answer. How I understand the problem might be quite unlike how you understand it.
The right way is the wrong way
When we are taught to rely on a singular, step-by-step process as the true way to solve a math problem, we turn off our problem-solving brain. These skills require continuous work to keep them sharp, and the constant reliance on someone else’s “exact right” method dulls them. Over the years, we may even lose at least some of our problem-solving acumen by not using it.
This reliance also discourages courage — we need to take chances to solve problems, and insistence on following a singular method prevents us from risking wrong answers via experimentation.
We can solve problems in many distinct ways. In fact, trying different approaches is fun as well as instructive, and it is necessary when problem-solving gets hard — which is often when the problems are most worth solving. Engineers who write software code or build bridges make a conscious attempt to solve problems in more than one way, even when a solution is readily available to them.
Why not solve it and move on?
First of all, if you dig deeper to find more than one solution, you can decide which one among them is less expensive, more durable, or more elegant — whichever outcome matters most to you. Second, and perhaps more significant, when problem-solving gets really hard and the way ahead isn’t clear, you need to be ready to try anything. And the first step of the “try anything” approach is to back up and examine a problem from every angle, or at least from more angles than you initially see.
In the real world, of course, we often resort to looking at problems from fresh angles out of desperation. “Try anything” is the motto. As one member of a two-working-parents household with elementary school twins during the COVID-19 pandemic, we often were compelled to try anything to solve problems regarding work, social distancing protocols, on-and-off remote school, and limited childcare.
To expose the myth of a single correct method for the sham that it is, we need to understand the consequences of answer-getting versus problem-solving. Because we’ve been brainwashed into believing that answer-getting is good and because most of us spent years in answer-getting math curricula, we don’t realize the negative effects it has on us.
Here are typical ways we respond in an answer-getting environment:
- The mind goes blank. For a moment, nothing occurs to us because we’re not allowed to use our minds creatively.
- Racing heart. We react anxiously as we try to remember how the teacher did the math on the board. What was her first step again?
- Negative self-talk. For a moment, we have the germ of an idea, an instinct about how to start solving a challenging math problem, but because we’ve been conditioned to seek the answer only one way, we chastise ourselves for thinking we know better than what we’ve been taught, and we revert to standard operating procedure.
- Reluctance to talk through questions and concerns. We’re embarrassed to bring up these issues with others, assuming they are “right way” adherents. This reluctance to involve others is an obstacle to a creative, collaborative process.
The overarching effect of an answer-getting system is disempowerment. We feel defeated before even attempting to work on a problem.
Here are recent conversations I have had with children and adults on what this sort of math feels like:
“I want to use decimals. The teacher wants me to use fractions for no reason. I just have to do what he says. There is no freedom to do the math the way you want to do it, even if my way is easier for me. No one listens to me.”
“I actually remember getting dinged on a high school math test even when I had the right answer, but I had solved it my own way. As a teenager, that made me furious. Now looking back as an adult, I think about it like tennis. If you are drilling me so I learn or improve a new skill like backhand volleys, then I can understand the reasoning for forcing a specific approach. But if you have no reason whatsoever for forcing your way on me, it still steams me to think about it.”
A problem-solving approach conjures significantly contrasting responses — responses that reflect a sense of empowerment and courage. Ideally, schools would teach math with problem-solving as its driving principle rather than the myth of a single right way. To approach this ideal, however, we need to understand what problem-solving is all about.
Math should be taught as a collaborative process, much as other subjects are taught.
How to counteract the myth
We make math a performative rather than a learning experience. When the teacher asks the class, “What is the answer to 63 plus 37?” he turns math into an individual sport.
Add the myth of speed, and each student is scrambling to come up with the answer first and win the game. The answer becomes the only thing that matters, and both understanding and collaboration fall by the wayside.
No doubt, some of you might wonder if I’ve lost my math mind. After all, we need to get the answers right so that we can purchase the right amount of carpeting to cover a room’s floor or make sure that our rocket makes it to the moon.
Again, this is an issue of integrative complexity. Of course, we need to know what 63 + 37 equals. But if that’s all we know, then we’re missing out on a lot of what math offers.
Fortunately, we can learn in a way that we obtain precision as well as other benefits. Consider again 63 + 37. What if the teacher framed the question this way: “Don’t tell me the answer. It’s 100. How would you start calculating 63 plus 37 in your head? What is your first step?” Now math is a process.
I have gotten the chance to hear second graders’ brains working at this moment many times. Each time is a joy. One might say, “I broke this up as 60 plus 30 plus 3 plus 7. And the next thing in my head I saw was that it was 93 plus 7. And then I knew that was 100.” Another second grader might offer another option: “I looked at this for a moment, and I saw that 3 and 7 make 10. So I knew I had a 60 plus 30 plus 10. And I know that’s 100.”
This is the math people need in their lives. This is what is needed to build bridges. It’s also how you build a deep math sense.
Math should be taught as a collaborative process, much as other subjects are taught. We often view math as distinct from other subjects in K–8, as something that must be taught as an individual sport where everyone is on his or her own to come up with the right answer first. Other subjects are taught as team sports, ones where process matters, where students don’t rely on tricks, where students are encouraged to work together, and where a variety of ways to answer may be acceptable. But when it comes to math, collaboration and process work are subordinated or eliminated.
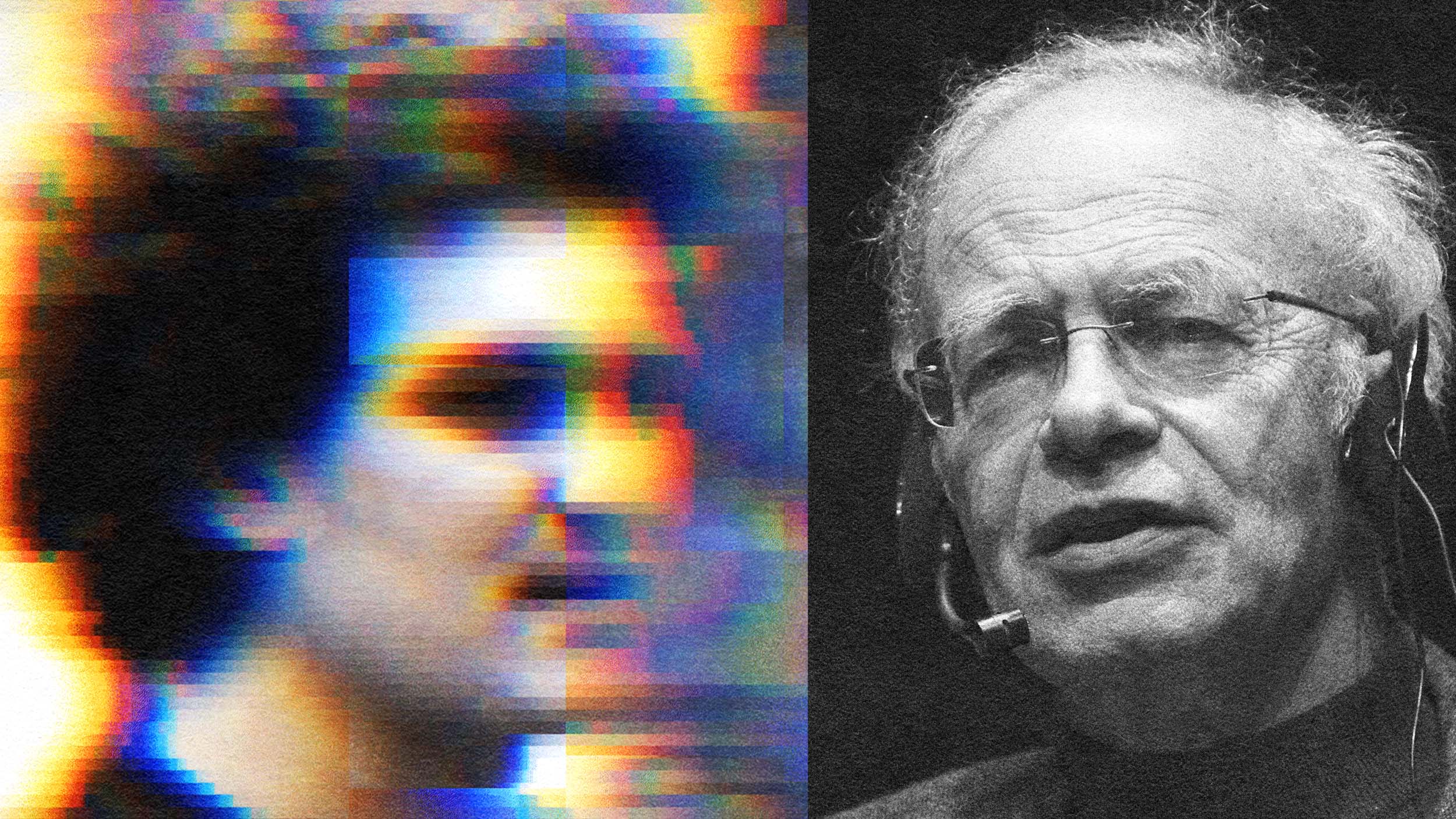
Sphero makes remarkably cool, programmable robots and STEAM-based educational tools that transform the way kids learn, create and invent through coding, science, music, and the arts.
- Lessons & Resources
- Sphero Global Challenge
- Schedule a Virtual Demo
- Professional Development
- Standards Alignment
- Funding & Grants
- Case Studies & Whitepapers
- App Downloads
- Sphero BOLT+
- Sphero BOLT
- Sphero indi
- Sphero RVR+
- Sphero littleBits
- Sphero Blueprint
- Sphero Mini
- Computer Science Foundations (Sphero BOLT)
- Age Brackets
- Early Elementary
- Upper Elementary
- Middle School
- Manage Your Team
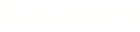
Enter your e-mail and password:
New customer? Create your account
Lost password? Recover password
Recover password
Enter your email:
Remembered your password? Back to login
Create my account
Please fill in the information below:
Already have an account? Login here
Innovative Math Teaching Strategies for the Digital Age

Let's face it, math has always had a bit of an image problem. For many students, math is the subject they love to hate . But here's the thing: strong math skills are becoming increasingly important in our increasingly data-driven world. That's why educators are turning to digital tools to make math more engaging, relevant, and fun.
From apps that turn algebra into an adventure game to websites that offer instant feedback on calculus problems, these digital tools are doing more than just jazzing up a math class. They're transforming how students engage with mathematical concepts, pushing them to think critically, and equipping them with problem-solving skills that will serve them well beyond the classroom.
And it seems to be working. According to a survey, 68% of US educators believe digital tools lead to higher student engagement.
Understanding The Digital Revolution in Math Education
Adopting digital tools in math classrooms isn’t about replacing textbooks with tablets. We're looking at tools that can help visualize complex equations, simulate real-world math problems, and even use AI to tailor lessons to each student's needs.
Beyond changing how we teach math, the digital revolution also changes how students experience, engage with, and ultimately learn math. Some of the benefits of incorporating digital tools in math classrooms include:
- Increased engagement: When math feels more like playing a video game than solving a worksheet, even the most math-phobic students suddenly raise their hands to participate.
- Personalized learning: These digital tools can adapt on the fly, serving up easier problems when a student is struggling or ramping up the difficulty when they're cruising. This differentiated instruction approach helps to address gaps in understanding and reinforces strengths, ensuring a more effective learning experience.
- Real-time feedback: Digital platforms provide immediate feedback on exercises and assessments, allowing students to instantly understand and correct their mistakes. This timely feedback is crucial for reinforcing learning.
The benefits of digital tools in math education extend beyond mathematical concepts . Very often, digital tools can be a road to other helpful knowledge. For example, when students store their calculations in the form of a Sharepoint backup , they learn about software and data management without even knowing it. The same goes for students using graphing calculators or statistical software; they're simultaneously developing data visualization and digital literacy skills that will serve them well in many future careers.

Types of Digital Learning Tools for Math Class
Educators have various digital tools at their fingertips to make math more engaging and accessible, each serving a unique purpose in the math education ecosystem. Some popular types include:
Interactive Apps for Math Learning
Interactive apps are digital tools designed to engage students in active learning through interactive elements such as animations, quizzes, and games.
These apps transform traditional learning methods into dynamic and engaging experiences in math education. They help students grasp complex concepts through interactive and visual means, making learning more enjoyable and effective.
These apps come in all shapes and sizes. There are practice and drill apps that reinforce math skills through repetitive exercises and quizzes. Some apps turn math problems into gamified learning , keeping students more engaged and motivated. Concept explanation apps provide in-depth explanations and visualizations of math concepts.
Some popular interactive apps for math learning include Photomath (which solves equations from photos), Prodigy (a math role-playing game), and GeoGebra (an interactive app that combines geometry, algebra, and calculus).
The magic of these apps lies in their ability to make math feel less like a chore and more like an adventure. They offer immediate feedback, celebrate small victories, and allow students to learn at their own pace. To succeed, you should:
- Start small: Start with one or two apps to see how they enhance learning and engagement.
- Align with curriculum: Choose apps that align with your curriculum goals and complement your teaching methods.
- Encourage exploration: Allow students to explore apps independently and find the best tools for them.
- Monitor progress: Use the data and feedback provided by these apps to monitor student progress and identify areas needing additional support.

Dynamic Websites for Math Education
These interactive math platforms provide a wealth of educational resources and activities, adapting to users' needs and inputs in real-time. They offer an immersive learning experience beyond static textbook content, making math education more accessible and interactive.
Most dynamic math websites offer interactive exercises, adaptive learning paths , visual aids that bring abstract concepts to life, progress tracking, and community features where students can collaborate and seek help.
Some popular examples of dynamic math websites include IXL Math, Brilliant, Mathigon, Desmos, and Wolfram Alpha.
Comprehensive Digital Platforms
Comprehensive digital platforms are integrated systems combining various tools and resources for teaching and learning math in a cohesive environment. They provide a mix of lessons, practice exercises, assessments, progress tracking, and often teacher support tools, all bundled into one package.
Khan Academy is an excellent example of a comprehensive digital platform for learning math. Other examples include DreamBox Learning, which uses adaptive technology to personalize math instruction, and Mathletics, which combines targeted curriculum content with engaging games and challenges.
Here's some practical advice on how to make the most of these platforms as an educator:
- Start with the basics: Integrate basic functionalities such as assignment distribution and grading, then gradually incorporate more advanced tools as you and your students become comfortable.
- Personalize learning: Use the platform's adaptive learning features to create personalized learning paths for your students, addressing their strengths and weaknesses.
- Monitor progress: Use the analytics and reporting features to regularly track student progress and adjust your instruction based on their performance and needs.
- Encourage student ownership: Teach students how to use the platform independently for self-study.
Promoting Critical Thinking Through Digital Learning Tools
Critical thinking is an essential skill in math education that enables students to analyze, evaluate, and solve complex problems. It goes beyond rote memorization of formulas and procedures; instead, it encourages students to understand underlying concepts, connect ideas, and apply their knowledge to real-world situations.
Digital learning tools play a significant role in developing critical thinking by providing interactive and engaging platforms for students to explore mathematical concepts.
For instance, virtual manipulatives and simulations allow students to experiment with variables and observe outcomes, promoting a deeper understanding of mathematical principles. Similarly, game-based apps challenge students to solve math puzzles and problems, encouraging them to think critically and develop strategic approaches.
Additionally, immediate feedback from digital tools helps students reflect on their answers, understand their mistakes, and refine their problem-solving strategies. Most importantly, don’t forget to:
- Incorporate open-ended questions: Use digital tools to present students with open-ended questions that require them to explain their reasoning and approach to solving problems.
- Use real-world scenarios: Design digital lessons that apply math concepts to real-world situations, encouraging students to think critically about how math is used in everyday life.
- Implement adaptive learning: Integrate adaptive learning technologies that adjust the difficulty of problems based on student performance, providing tailored challenges that promote analytical thinking.
- Blend gamification: Incorporate gamified learning tools that require strategic thinking and problem-solving to make the learning process engaging and thought-provoking.
Remember, the goal isn't to let the digital tools think for your students. Instead, students can use these tools to create an environment that encourages, supports, and challenges students to think critically.
Wrapping Up
Digital learning tools aren't just making math more fun (though they're definitely doing that). They're fundamentally changing how students interact with mathematical concepts and cultivating critical thinking and digital literacy skills that will serve them well beyond the classroom.
That said, keep in mind that these digital platforms aren't meant to replace traditional teaching—they're tools to enhance it. Used wisely, they can turn math from a dreaded subject into an engaging, interactive experience that students look forward to.
Opinions expressed by Sphero.com contributors are their own.
Written by:
Sphero Team
Keep your inbox inspired. Sign up for product updates, exclusive offers, and teacher resources.
Popular posts
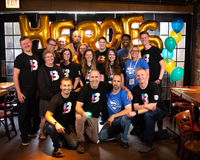
Featured products

Academia.edu no longer supports Internet Explorer.
To browse Academia.edu and the wider internet faster and more securely, please take a few seconds to upgrade your browser .
Enter the email address you signed up with and we'll email you a reset link.
- We're Hiring!
- Help Center
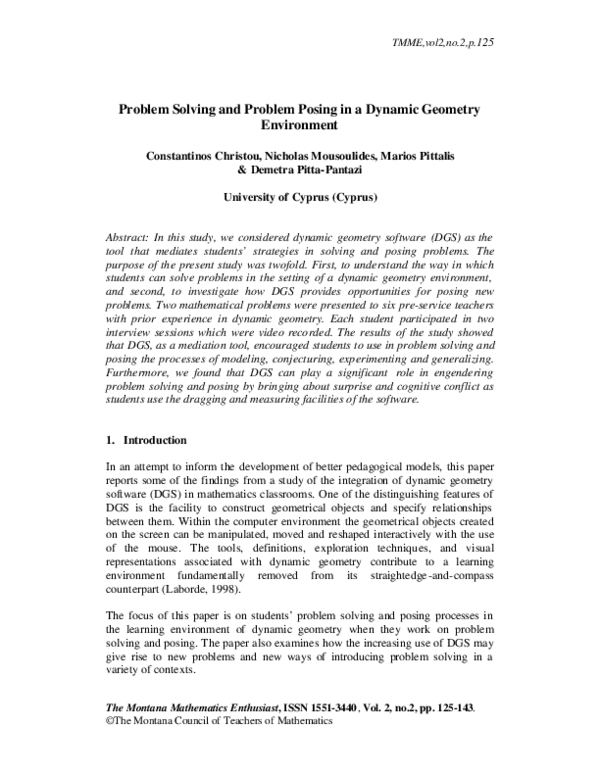
Problem Solving and Problem Posing in a Dynamic Geometry Environment

Related Papers
Miguel Labella , Antonio Codina Sánchez , Isabel M Romero
This paper reports part of a design research experiment aimed at studying the influence of Dynamic Geometry Systems (in particular, GeoGebra) in students' mathematical competencies development. It was conducted in two cycles with four classes at a secondary school. The results show evidences that the software helped students to develop mathematical competencies at different degrees. Geogebra provided students enough support for reaching a good level of performance in competencies related with using tools, managing representations, and problem solving. The software supported students in achieving basic to medium levels in competencies related to reasoning, argumentation and communication. In these last competencies, social interaction proved to be a key factor for students' progress.
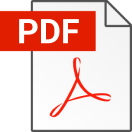
Mohamed El-Demerdash
Arzu Aydoğan Yenmez , Ayhan K Erbas
This article appeared in a journal published by Elsevier. The attached copy is furnished to the author for internal non-commercial research and education use, including for instruction at the authors institution and sharing with colleagues. Other uses, including reproduction and distribution, or selling or licensing copies, or posting to personal, institutional or third party websites are prohibited. In most cases authors are permitted to post their version of the article (e.g. in Word or Tex form) to their personal website or institutional repository. Authors requiring further information regarding Elsevier's archiving and manuscript policies are encouraged to visit: http://www.elsevier.com/copyright a b s t r a c t The purpose of this study was to investigate the effects of using a dynamic geometry environment (DGE) together with inquiry-based explorations on the sixth grade students' achievements in polygons and congruency and similarity of polygons. Two groups of sixth grade students were selected for this study: an experimental group composed of 66 students (34 boys and 32 girls); and a control group composed of 68 students (35 boys and 33 girls). The students in the experimental group taught with a DGE, while the students in the control group received textbook-based direct instruction. An achievement test was administered as pre-test, post-test, and delayed post-test in both groups. Qualitative data were collected through videotaped classroom observations. The results showed that the DGE together with open-ended explorations significantly improved students' performances in polygons and congruency and similarity of polygons. Furthermore, students in the experimental group showed greater interest and motivation toward learning geometry compared to those in the control group whom often showed lack of interest and curiosity. Also, students' comments and interpretations during lessons and tests were more accurate and advanced in the experimental group as they engage more in the DGE. Moreover, qualitative data suggested that boys showed greater interest in the computer-based learning environment than girls in the experimental groups although no significant gender effect on achievement was found.
Boris Koichu
International Journal of Science and Mathematics Education
Demetra Pitta-Pantazi
Anna Athanasopoulou
STAVROULA PATSIOMITOU (Δρ. Σταυρούλα Πατσιομίτου)
https://globaljournals.org/eBooks/A_Trajectory_for_the_Teaching_and_Learning_of_the_Didactics_of_Mathematics_using_ICT.pdf
In the current study, I shall describe characteristic elements of a ‘dynamic’ trajectory, a synthesis of experts of mybprevious research regarding an approximation process for the construction of number pi, enriched, analyzed and reviewed in the light of my recent theoretical considerations (not published as yet). Number pi is a mathematical abstract object but it can also be perceived as a result of a process. Specific examples from my experimental research using dynamic active representations will be analyzed in the methodology section. Moreover, a brief report of students (small groups or individuals) which participated in the process. My aim was the students to conceive the meaning of number pi as a limit using the iteration process of the Geometer’s Sketchpad dynamic geometry software. Finally, the role the active representations play in the learning trajectory made me think of a way to define what a ‘dynamic active learning trajectory’ is.
— A basic goal of the current study, which is an excerpt from a larger study, is to analyse students' interactions in the context of their working on transformations of tools, and specifically of custom tools in a microworld, the Geometer's Sketchpad. Custom tools are encapsulated objects created in a DGS environment. The construction of a custom tool and its subsequent implementation in a pair of students are the focus of this study. Custom tools can serve as structural units of knowledge, as conceptual objects and hence as 'schemes'. Moreover, they can become an 'alive' active tool for students' cognitive development. The paper will include the following parts: (a) how students learn in a constructivist framework; (b) a description of the van Hiele model, and especially the meanings of 'symbol and signal character'; (c) how a DGS environment functions as an 'alive' microworld; (d) the role of artifacts-[custom] tools as instruments-[custom] tools; (e) the research methodology of the current study (f) a detailed description of the experimental process (g) discussion and conclusion.
Loading Preview
Sorry, preview is currently unavailable. You can download the paper by clicking the button above.
RELATED PAPERS
Mahmoud Mohamed
International Group for the …
Marios Pittalis
efdergi.hacettepe.edu.tr
nazan sezen
The Journal of Mathematical Behavior
Roza Leikin
H.U. Journal of Education
Sema Selman
International Journal of Computers for Mathematical Learning
Gloriana Gonzalez
Miguel Cruz Ramírez , Mauro Misael Garcia Pupo
The International Review of Research in Open and Distributed Learning
Wing-Kwong Wong
Igor' Kontorovich
IJAR Indexing
Ercan Atasoy
Igor' Kontorovich , Roza Leikin
Jarmila Novotna
Caroline "Caro" Williams , Mitchell Nathan , Candace Walkington
Thomas Hillman
Journal of Mathematics and Statistics
Abdul Hafiz Abdullah
Maria Mariotti
Educational Studies in Mathematics
Angel Gutierrez
Michael de Villiers
Candace Walkington , Elizabeth L Pier
Florence Mihaela Singer
ana maria Correal
FULVIA FURINGHETTI
WORKING GROUP 9 Tools and technology in …
Mette Andresen
Global Journal of Computer Science and …
Foteini Moustaki
International Education Studies
Roslinda Rosli
… Journal for Technology …
maria alessandra Mariotti
British Journal of Educational Technology
Anna Sierpinska
International Journal of Engineering and Technology
Naufal Ishartono
RELATED TOPICS
- We're Hiring!
- Help Center
- Find new research papers in:
- Health Sciences
- Earth Sciences
- Cognitive Science
- Mathematics
- Computer Science
- Academia ©2024
Grab your spot at the free arXiv Accessibility Forum
Help | Advanced Search
Mathematics > Optimization and Control
Title: positive dynamic programming: a critique.
Abstract: In the article Positive Dynamic Programming, David Blackwell tries to answer the question concerning the existence of optimal stationary strategies for a positive dynamic programming problem. The principal results obtained in the paper are indicative of the existence of weakly optimal stationary plans where the optimal return need not be Borel measurable. More specifically, the main theorem establishes the condition for the weakly optimal stationary plan when the optimal return is bounded. Blackwell also gives an example that demonstrates that no optimal plan exists showing that the results obtained in his earlier work on discounted dynamic programming, cannot be generally extended to the positive case.
Subjects: | Optimization and Control (math.OC) |
Cite as: | [math.OC] |
(or [math.OC] for this version) |
Submission history
Access paper:.
- Other Formats

References & Citations
- Google Scholar
- Semantic Scholar
BibTeX formatted citation

Bibliographic and Citation Tools
Code, data and media associated with this article, recommenders and search tools.
- Institution
arXivLabs: experimental projects with community collaborators
arXivLabs is a framework that allows collaborators to develop and share new arXiv features directly on our website.
Both individuals and organizations that work with arXivLabs have embraced and accepted our values of openness, community, excellence, and user data privacy. arXiv is committed to these values and only works with partners that adhere to them.
Have an idea for a project that will add value for arXiv's community? Learn more about arXivLabs .
MIT Technology Review
- Newsletters
Google DeepMind’s new AI systems can now solve complex math problems
AlphaProof and AlphaGeometry 2 are steps toward building systems that can reason, which could unlock exciting new capabilities.
- Rhiannon Williams archive page

AI models can easily generate essays and other types of text. However, they’re nowhere near as good at solving math problems, which tend to involve logical reasoning—something that’s beyond the capabilities of most current AI systems.
But that may finally be changing. Google DeepMind says it has trained two specialized AI systems to solve complex math problems involving advanced reasoning. The systems—called AlphaProof and AlphaGeometry 2—worked together to successfully solve four out of six problems from this year’s International Mathematical Olympiad (IMO), a prestigious competition for high school students. They won the equivalent of a silver medal.
It’s the first time any AI system has ever achieved such a high success rate on these kinds of problems. “This is great progress in the field of machine learning and AI,” says Pushmeet Kohli, vice president of research at Google DeepMind, who worked on the project. “No such system has been developed until now which could solve problems at this success rate with this level of generality.”
There are a few reasons math problems that involve advanced reasoning are difficult for AI systems to solve. These types of problems often require forming and drawing on abstractions. They also involve complex hierarchical planning, as well as setting subgoals, backtracking, and trying new paths. All these are challenging for AI.
“It is often easier to train a model for mathematics if you have a way to check its answers (e.g., in a formal language), but there is comparatively less formal mathematics data online compared to free-form natural language (informal language),” says Katie Collins, an researcher at the University of Cambridge who specializes in math and AI but was not involved in the project.
Bridging this gap was Google DeepMind’s goal in creating AlphaProof, a reinforcement-learning-based system that trains itself to prove mathematical statements in the formal programming language Lean. The key is a version of DeepMind’s Gemini AI that’s fine-tuned to automatically translate math problems phrased in natural, informal language into formal statements, which are easier for the AI to process. This created a large library of formal math problems with varying degrees of difficulty.
Automating the process of translating data into formal language is a big step forward for the math community, says Wenda Li, a lecturer in hybrid AI at the University of Edinburgh, who peer-reviewed the research but was not involved in the project.
“We can have much greater confidence in the correctness of published results if they are able to formulate this proving system, and it can also become more collaborative,” he adds.
The Gemini model works alongside AlphaZero —the reinforcement-learning model that Google DeepMind trained to master games such as Go and chess—to prove or disprove millions of mathematical problems. The more problems it has successfully solved, the better AlphaProof has become at tackling problems of increasing complexity.
Although AlphaProof was trained to tackle problems across a wide range of mathematical topics, AlphaGeometry 2—an improved version of a system that Google DeepMind announced in January—was optimized to tackle problems relating to movements of objects and equations involving angles, ratios, and distances. Because it was trained on significantly more synthetic data than its predecessor, it was able to take on much more challenging geometry questions.
To test the systems’ capabilities, Google DeepMind researchers tasked them with solving the six problems given to humans competing in this year’s IMO and proving that the answers were correct. AlphaProof solved two algebra problems and one number theory problem, one of which was the competition’s hardest. AlphaGeometry 2 successfully solved a geometry question, but two questions on combinatorics (an area of math focused on counting and arranging objects) were left unsolved.
“Generally, AlphaProof performs much better on algebra and number theory than combinatorics,” says Alex Davies, a research engineer on the AlphaProof team. “We are still working to understand why this is, which will hopefully lead us to improve the system.”
Two renowned mathematicians, Tim Gowers and Joseph Myers, checked the systems’ submissions. They awarded each of their four correct answers full marks (seven out of seven), giving the systems a total of 28 points out of a maximum of 42. A human participant earning this score would be awarded a silver medal and just miss out on gold, the threshold for which starts at 29 points.
This is the first time any AI system has been able to achieve a medal-level performance on IMO questions. “As a mathematician, I find it very impressive, and a significant jump from what was previously possible,” Gowers said during a press conference.
Myers agreed that the systems’ math answers represent a substantial advance over what AI could previously achieve. “It will be interesting to see how things scale and whether they can be made faster, and whether it can extend to other sorts of mathematics,” he said.
Creating AI systems that can solve more challenging mathematics problems could pave the way for exciting human-AI collaborations, helping mathematicians to both solve and invent new kinds of problems, says Collins. This in turn could help us learn more about how we humans tackle math.
Artificial intelligence
How to opt out of meta’s ai training.
Your posts are a gold mine, especially as companies start to run out of AI training data.
- Melissa Heikkilä archive page
Why does AI hallucinate?
The tendency to make things up is holding chatbots back. But that’s just what they do.
- Will Douglas Heaven archive page
How generative AI could reinvent what it means to play
AI-powered NPCs that don’t need a script could make games—and other worlds—deeply immersive.
- Niall Firth archive page
Synthesia’s hyperrealistic deepfakes will soon have full bodies
With bodies that move and hands that wave, deepfakes just got a whole lot more realistic.
Stay connected
Get the latest updates from mit technology review.
Discover special offers, top stories, upcoming events, and more.
Thank you for submitting your email!
It looks like something went wrong.
We’re having trouble saving your preferences. Try refreshing this page and updating them one more time. If you continue to get this message, reach out to us at [email protected] with a list of newsletters you’d like to receive.

- Mideast News
- Art&Culture
- ynetespanol
- Privacy Policy
- Terms of Use
Google DeepMind's AlphaProof wins silver in Math Olympics
Alphaproof leverages formal mathematical language, pre-trained language models, reinforcement learning techniques, and synthetic data generation for training, enabling it to generate and verify solution candidates for increasingly difficult problems.
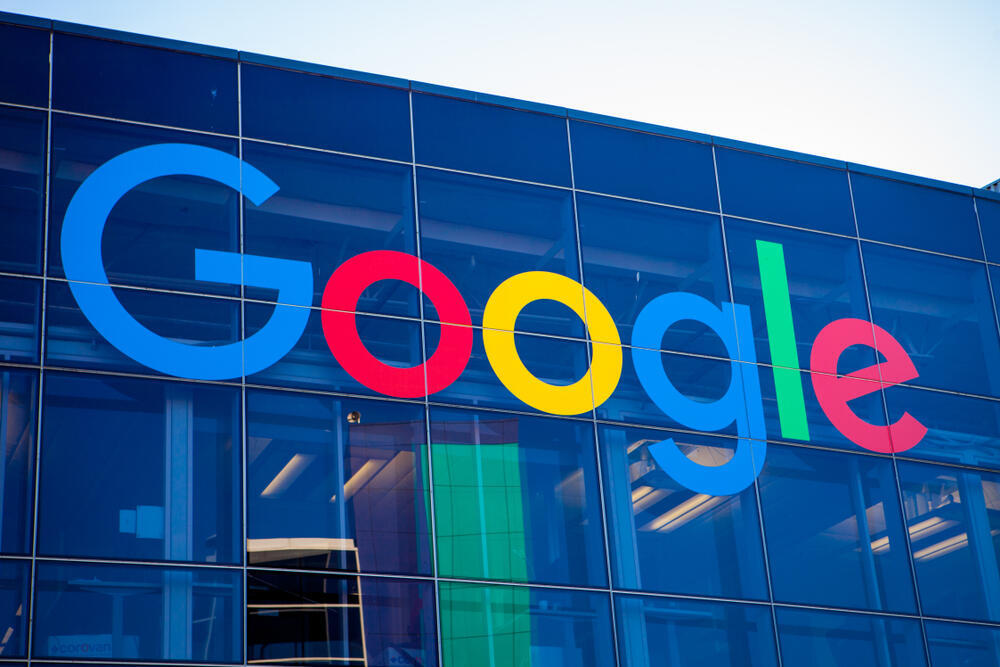
- Mathematical Sciences
- Dynamic Geometry Software
The Effectiveness of Dynamic Geometry Software Applications in Learning Mathematics: A Meta- Analysis Study
- January 2021
- International Journal of Interactive Mobile Technologies (iJIM) 15(2):18-37
- 15(2):18-37

- Universitas Pendidikan Indonesia

- Universitas Katolik Indonesia Santu Paulus

- Universitas Muhammadiyah Prof. Dr. Hamka
Abstract and Figures

Discover the world's research
- 25+ million members
- 160+ million publication pages
- 2.3+ billion citations
- Yetti Ariani

- Pengjin Wang

- Mustafa Demir
- Ahmet Çelik
- Tunahan Filiz
- Int J Sci Math Educ
- Sheng-Kuei Hsu
- Matthew F. Vazzana
- Winda Lestari
- Muliawan Firdaus
- Abdul Manaf
- PFLUG ARCH EUR J PHY

- Josefa León

- Surya Narayan Panda

- Khor Mui Kim

- Poopy Yaniawati
- Rully Indrawan

- Nur Hamidah
- Iis Nur Afidah
- Lutfi Wahyu Setyowati
- Junaedi Junaedi
- Dedek Kustiawati
- Tatang Herman
- Recruit researchers
- Join for free
- Login Email Tip: Most researchers use their institutional email address as their ResearchGate login Password Forgot password? Keep me logged in Log in or Continue with Google Welcome back! Please log in. Email · Hint Tip: Most researchers use their institutional email address as their ResearchGate login Password Forgot password? Keep me logged in Log in or Continue with Google No account? Sign up

IMAGES
VIDEO
COMMENTS
representations associated with dynamic geometry contribute to a learning environment fundamentally removed from its straightedge-and-compass counterpart (Laborde, 1998). The focus of this paper is on students' problem solving and posing processes in the learning environment of dynamic geometry when they work on problem solving and posing.
Problem-posing activities become richer and more profound when technology is involved, since the technical work involving computing and graphing is executed by the software more rapidly and efficiently (Ranasinghe & Leisher, 2009).One of the distinctive features of dynamic geometry software (DGS) is the facility to construct geometrical objects and specify relationships between them.
In this study, we considered dynamic geometry software (DGS) as the tool that mediates students' strategies in solving and posing problems. The purpose of the present study was twofold. First, to understand the way in which students can solve problems in the setting of a dynamic geometry environment, and second, to investigate how DGS provides opportunities for posing new problems. Two ...
Now switch to the Move tool and select the polygon. Copy (Command-C on a Mac; Control-C in Windows) and paste (Command-V on a Mac; Control-V in Windows) a new copy of the triangle. Drag the whole triangle (be careful not to drag any of the vertices; that would change the shape of the triangle) on top of A'B'C'.
This study attempted to take on this challenge. It was guided by one research question: how can we capture learner's growth in geometric understanding during problem solving in a dynamic geometry environment? 2. Conceptual framework: networking the Pirie-Kieren theory and instrumental genesis
Dynamic geometry tools are engaging students in active learning in geometry. Solving problems, posing questions, creating conjectures, searching for connections, considering counter-examples or formal deductive proofs are enriched with our ability to visualize and reason using diagrams. In each of these formats, dynamic geometry is
Abstract and Figures. In this study, we considered dynamic geometry software (DGS) as the tool that mediates students' strategies in solving and posing problems. The purpose of the present study ...
Problem-Posing Activities in a Dynamic Geometry Environment: When and How. January 2015. DOI: 10.1007/978-1-4614-6258-3_19. Authors: Ilana Lavy. Max Stern Yezreel Valley College. To read the full ...
mathematics problem solving was used to identify patterns of metacognitive processes in a dynamic geometry environment. During the reading, understanding, and analysis episodes, the participants
The emergence of dynamic geometry environments challenges researchers in mathematics education to develop theories that capture learner's growth in geometric understanding in this particular environment. This study coordinated the Pirie-Kieren theory and instrumental genesis to examine learner's growth in geometric understanding when solving problems in a dynamic geometry environment.
Dynamic geometry software (DGS) aims to enhance mathematics education. ... Software tools for geometrical problem solving: Potentials and pitfalls. International Journal of Computers for Mathematical Learning, 6(3), 235-256. Crossref. Google Scholar. ... Dynamic Software, Task Solving With or Without Guidelines, and Learnin...
Problem-posing activities should follow activities of problem solving through which the content knowledge of the learnt topic is built. Students should experience problem-posing activities starting at elementary school. ... they should be provided with the option to make sense of the objects via dynamic geometry software. Skip to search form ...
Students regularly struggle with mathematical tasks, particularly those concerning non-routine problems in geometry. Although educators would like for their learners to transfer their knowledge to non-routine and real-life situations, students run into a number of difficulties. The goal of this exploratory study was to analyze three participants' problem solving processes in a dynamic ...
the metacognition that students exhibit when solving nonroutine geometry problems in a dynamic geometry environment. In this study, dynamic tool software—namely, the Geometer's Sketchpad—was used by the participants. My intention was to focus on participants' decision making, reflection, reasoning, and problem solving as well as to ...
In particular, how students use dynamic geometry software to explore mathematical relationships, how they engage with the Standards for Mathematical Practice, and the important role of the teacher in this process are prominent themes in the lesson. ... Problem Solving and Problem Posing in Geometry with Dynamic Software Armando M. Martínez ...
Chatting about the activities in a small group will help you to think about geometry and problem solving on your own in the future. This GeoGebraBook includes 50 GeoGebra activities, grouped in 13 chapters. Chapters 1 and 13 are for you to work on by yourself. The other chapters are for you to work on with a team of two or three friends.
middle school mathematics teachers' geometric thinking and problem-solving performance on dynamic geometry environment. Mixed-method research design was used in the study. The study was conducted with 46 sampling strategy. The data were collected through the tests including open-ended and multiple-choice questions. In the quantitative part of the
Gebra's effectiveness as a tool for helping students solve linear optimization word problems (Molnar,´ 2016), in understanding plane geometry (Pereira et al., 2017), and visualizing concepts of eigenvalues and eigenvectors in linear algebra (Jose et al., 2017).´ Figure 1. GeoGebra to justify angle sum theorem.
Dynamic programming refers to a problem-solving approach, in which we precompute and store simpler, similar subproblems, in order to build up the solution to a complex problem. It is similar to recursion, in which calculating the base cases allows us to inductively determine the final value. This bottom-up approach works well when the new value depends only on previously calculated values.
Last Thursday, Google DeepMind announced it had built AI systems that can solve complex math problems. The systems—called AlphaProof and AlphaGeometry 2—worked together to successfully solve ...
This study aims to review the literature on applying dynamic geometry software to improve mathematical problem-solving skills. The research method used is Systematic Literature Review (SLR), which ...
The right way is the wrong way. When we are taught to rely on a singular, step-by-step process as the true way to solve a math problem, we turn off our problem-solving brain.
Increased engagement: When math feels more like playing a video game than solving a worksheet, even the most math-phobic students suddenly raise their hands to participate. Personalized learning: These digital tools can adapt on the fly, serving up easier problems when a student is struggling or ramping up the difficulty when they're cruising.
TMME,vol2,no.2,p.125 Problem Solving and Problem Posing in a Dynamic Geometry Environment Constantinos Christou, Nicholas Mousoulides, Marios Pittalis & Demetra Pitta-Pantazi University of Cyprus (Cyprus) Abstract: In this study, we considered dynamic geometry software (DGS) as the tool that mediates students' strategies in solving and posing problems.
In the article Positive Dynamic Programming, David Blackwell tries to answer the question concerning the existence of optimal stationary strategies for a positive dynamic programming problem. The principal results obtained in the paper are indicative of the existence of weakly optimal stationary plans where the optimal return need not be Borel measurable. More specifically, the main theorem ...
But that may finally be changing. Google DeepMind says it has trained two specialized AI systems to solve complex math problems involving advanced reasoning. The systems—called AlphaProof and ...
This paper describes the problem solving behavior of two preservice teachers as they worked individually on three nonroutine geometry problems. A dynamic tool software, namely the Geometer's ...
The result is unlikely to win the cash prize, but it represents the first progress in decades on a major knot in math's biggest unsolved problem, and it promises to spark new advances throughout number theory. "It's a sensational breakthrough," says Alex Kontorovich, a mathematician at Rutgers University.
While some problems were solved in minutes, others took up to three days, even though IMO participants are given 4.5 hours per problem. The computational power invested by Google DeepMind remains ...
The Effectiveness of Dynamic Geometry Software Applications in Learning Mathematics: A Meta- Analysis Study January 2021 International Journal of Interactive Mobile Technologies (iJIM) 15(2):18-37