Information
- Author Services

Initiatives
You are accessing a machine-readable page. In order to be human-readable, please install an RSS reader.
All articles published by MDPI are made immediately available worldwide under an open access license. No special permission is required to reuse all or part of the article published by MDPI, including figures and tables. For articles published under an open access Creative Common CC BY license, any part of the article may be reused without permission provided that the original article is clearly cited. For more information, please refer to https://www.mdpi.com/openaccess .
Feature papers represent the most advanced research with significant potential for high impact in the field. A Feature Paper should be a substantial original Article that involves several techniques or approaches, provides an outlook for future research directions and describes possible research applications.
Feature papers are submitted upon individual invitation or recommendation by the scientific editors and must receive positive feedback from the reviewers.
Editor’s Choice articles are based on recommendations by the scientific editors of MDPI journals from around the world. Editors select a small number of articles recently published in the journal that they believe will be particularly interesting to readers, or important in the respective research area. The aim is to provide a snapshot of some of the most exciting work published in the various research areas of the journal.
Original Submission Date Received: .
- Active Journals
- Find a Journal
- Journal Proposal
- Proceedings Series
- For Authors
- For Reviewers
- For Editors
- For Librarians
- For Publishers
- For Societies
- For Conference Organizers
- Open Access Policy
- Institutional Open Access Program
- Special Issues Guidelines
- Editorial Process
- Research and Publication Ethics
- Article Processing Charges
- Testimonials
- Preprints.org
- SciProfiles
- Encyclopedia
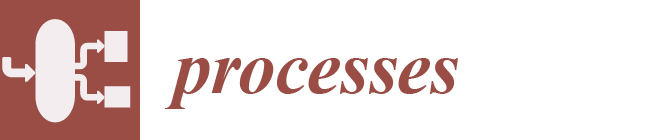
Article Menu
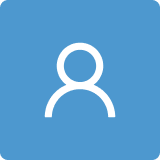
- Subscribe SciFeed
- Recommended Articles
- Google Scholar
- on Google Scholar
- Table of Contents
Find support for a specific problem in the support section of our website.
Please let us know what you think of our products and services.
Visit our dedicated information section to learn more about MDPI.
JSmol Viewer
A basic study on establishing the automatic sewing process according to textile properties.
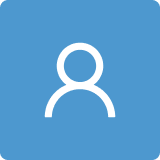
1. Introduction
2. materials and methods, 2.1. development of automatic feeding system for sewing, 2.2. preparation and measurement of the mechanical properties of the fabric samples, 2.3. sewing pattern and condition, 2.4. evaluation of sewability at the sewn seam, 3. results and discussion, 3.1. comparison of the sewing appearance by templates, 3.2. sewability according to presser height, 3.3. sewability according to sewing speed, 3.4. analysis of the correlation between the textile properties and sewing conditions, 4. conclusions, author contributions, institutional review board statement, informed consent statement, data availability statement, conflicts of interest.
- Sima, V.; Gheorghe, I.G.; Subic, J.; Nancu, D. Influences of the Industry 4.0 Revolution on the human capital development and consumer behavior: A systematic review. Sustainability 2020 , 12 , 4035. [ Google Scholar ] [ CrossRef ]
- Arakelyan, E.K.; Kosoy, A.A.; Mezin, S.V.; Pashchenko, F.F. Application of the basic principles of “Industry 4.0” in the intellectualization of automated control systems of modern thermal power plants. Procedia Comput. Sci. 2021 , 184 , 865–870. [ Google Scholar ]
- Ashima, R.; Haleem, A.; Bahl, S.; Javaid, M.; Mahla, S.K.; Singh, S. Automation and manufacturing of smart materials in additive manufacturing technologies using Internet of Things towards the adoption of industry 4.0. Mater. Today 2021 , 45 , 5081–5088. [ Google Scholar ]
- Ribeiro, J.; Lima, R.; Eckhardt, T.; Paiva, S. Robotic process automation and artificial intelligence in industry 4.0: A literature review. Procedia Comput. Sci. 2021 , 181 , 51–58. [ Google Scholar ] [ CrossRef ]
- Nayak, R.; Padhye, R. Automation in Garment Manufacturing ; Woodhead Publishing: Duxford, UK, 2018; p. 290. [ Google Scholar ]
- An, I.Y. The fourth industrial revolution: The potential impacts of artificial intelligence and automation technologies on gender equality. Pitt Policy J. 2020 , 11 , 108–123. [ Google Scholar ]
- Kondratas, A. Robotic gripping device for garment handling operations and its adaptive control. Fibers Text. East. Eur. 2005 , 13 , 84–89. [ Google Scholar ]
- Le, T.H.L.; Jilich, M.; Landini, A.; Zoppi, M.; Zlatanov, D.; Molfino, R. On the development of a specialized flexible gripper for garment handling. J. Autom. Control Eng. 2013 , 1 , 255–259. [ Google Scholar ] [ CrossRef ] [ Green Version ]
- Suh, M. Automated cutting and sewing for industry 4.0 at ITMA 2019. J. Text. App. Technol. Manag. 2019 , 1–13. Available online: https://ojs.cnr.ncsu.edu/index.php/JTATM/article/view/16453 (accessed on 10 July 2021).
- Lee, S.; Rho, S.H.; Lee, S.; Lee, J.; Lee, S.W.; Lim, D.; Jeong, W. Implementation of an automated manufacturing process for smart clothing: The case study of a smart sports bra. Processes 2021 , 9 , 289. [ Google Scholar ] [ CrossRef ]
- Yoshimi, T.; Takezawa, K.; Hirayama, M. An improvement of trajectory tracking accuracy of automatic sewing robot system by variable gain learning control. IFAC Paper OnLine 2018 , 51 , 1–6. [ Google Scholar ] [ CrossRef ]
- Thilagavathi, G.; Viju, S. Process Control in Textile Manufacturing ; Woodhead Publishing: Duxford, UK, 2013; pp. 428–473. [ Google Scholar ]
- Rajput, B.; Kakde, M.; Gulhane, S.; Raichurkar, P.P. Effect of sewing parameters on seam strength and seam efficiency. Trends Text. Eng. Fash. Technol. 2018 , 4 , 4–5. [ Google Scholar ]
- Gurarda, A. Seam performance of garments. In Textile Manufacturing Processes ; Intech: London, UK, 2019. [ Google Scholar ]
- Haghighat, E.; Etrati, S.M.; Najar, S.S. Evaluation of woven denim fabric sewability based on needle penetration force. J. Eng. Fiber Fabr. 2014 , 9 , 47–60. [ Google Scholar ] [ CrossRef ]
- Nayak, R. Sewing performance of stretch denim. J. Text. Appar. Technol. Manag. 2010 , 6 , 1–9. [ Google Scholar ]
- Juciene, M.; Vobolis, J. Correlation between the seam stitch length of the sewing garment and friction forces. Mater. Sci. 2007 , 132 , 74–78. [ Google Scholar ]
- Juciene, M.; Vobolis, J. Influence of fabric external friction force and certain parameters of a sewing machine upon stitch length. Mater. Sci. 2004 , 10 , 101–104. [ Google Scholar ]
- Gribaa, S.; Amar, S.B.; Dogui, A. Influence of sewing parameters upon the tensile behavior of textile assembly. Int. J. Cloth. Sci. Technol. 2006 , 18 , 235–346. [ Google Scholar ] [ CrossRef ] [ Green Version ]
- Dobilaite, V.; Juciene, M. Influence of sewing machine parameters on seam pucker. Strain 2007 , 56 , 286–292. [ Google Scholar ]
- Suh, J.K. Analysis of the structure of lockstitched seam according to sewing thread tension. Fash. Text. Res. J. 2001 , 3 , 25–30. [ Google Scholar ]
- Midha, V.K.; Kothari, V.K.; Chatopadhyay, R.; Mukhopadhyay, A. Effect of high-speed sewing on the tensile properties of sewing threads at different stages of sewing. Int. J. Cloth. Sci. Technol. 2009 , 21 , 217–238. [ Google Scholar ] [ CrossRef ]
- Naeem, M.S.; Mazari, A.; Khan, I.A.; Iftikhar, F. Effect of sewing speed on seam strength. Fibres Text. 2014 , 2 , 24–29. [ Google Scholar ]
- Vobolis, J.; Jucience, M.; Punys, J.; Vaitkevicius, V. Influence of selected machine and material parameters on the stitch length and its irregularity. Fibers Text. East. Eur. 2003 , 11 , 50–55. [ Google Scholar ]
- Ebrahim, F.F.S. Influence of mechanical properties of cotton fabrics on seam quality. Life Sci. J. 2012 , 9 , 831–836. [ Google Scholar ]
- Oh, H.; Oh, K.W.; Park, S. A study of the improvement of foam material sealing technology for wetsuits. Fash. Text. 2019 , 6 , 25. [ Google Scholar ] [ CrossRef ]
Click here to enlarge figure
Fabric Number | Structure | Thickness (mm) | Weight (g/m ) | Composition | Description |
---|---|---|---|---|---|
1 | Plain woven | 0.08 | 39 | Nylon 100% | Rip woven |
2 | Plain woven | 0.10 | 68 | Polyester 100% | Light weight woven |
3 | Plain woven | 0.30 | 115 | Nylon 70%, polyester 24%, polyurethane 6% | Span woven |
4 | Plain woven | 0.38 | 170 | Wool 40%, polyester 60% | Summer wool |
5 | Tricot knit | 0.56 | 225 | Polyester 79%, polyurethane 21% | Compression knit |
6 | Plain woven | 0.76 | 271 | Cotton 100% | Oxford |
7 | 2-layer tricot | 0.90 | 417 | Polyester 80%, polyurethane 20% | Neoprene |
8 | Pile knit | 0.94 | 316 | Cotton 100% | Corduroy |
9 | Twill woven | 0.94 | 411 | Cotton 100% | Denim |
10 | Twill woven | 0.95 | 362 | Cotton 100% | Chino |
11 | 3-layer tricot | 1.06 | 342 | Nylon 78%, polyurethane 22% (face), polyester 96%, polyurethane 4% (back) | Fleece knit |
12 | Double cloth woven | 1.51 | 517 | Wool 100% | Wool felt |
Sewability | Without Template | Flat Surface Template | Rough Surface Template |
---|---|---|---|
Appearance | |||
Stitch width (mm) | 1.0 ± 1.4 | 4.0 ± 0.7 | 4.3 ± 0.4 |
Seam strength (kgf/cm ) | 0.0 | 51.9 | 118.9 |
Seam efficiency (%) | 0.0 | 41.3 | 94.7 |
Sewability Factor | Fabric Number | Presser Height (mm) | |||||||
---|---|---|---|---|---|---|---|---|---|
0.0 | 0.5 | 1.0 | 1.5 | 2.0 | 2.5 | 3.0 | 3.5 | ||
SPI | 1 | 13.0 | 13.0 | 13.0 | 13.0 | 13.0 | 13.0 | 13.0 | 13.0 |
2 | 13.0 | 13.0 | 13.0 | 13.0 | 13.0 | 13.0 | 13.0 | 13.0 | |
3 | 13.0 | 13.0 | 13.0 | 13.0 | 13.0 | 13.0 | 13.0 | 13.0 | |
4 | 13.0 | 12.5 | 13.0 | 13.0 | 12.5 | 12.5 | 13.0 | 13.0 | |
5 | 13.5 | 13.0 | 14.0 | 14.0 | 14.0 | 16.0 | 15.0 | 16.0 | |
6 | 13.0 | 13.0 | 13.0 | 13.0 | 13.0 | 13.0 | 13.0 | 13.0 | |
7 | 13.0 | 13.0 | 13.0 | 13.0 | 13.0 | 13.0 | 13.0 | 13.0 | |
8 | 13.0 | 13.0 | 13.0 | 13.0 | 13.0 | 13.0 | 13.0 | 13.0 | |
9 | 13.0 | 12.5 | 12.5 | 13.0 | 13.0 | 12.5 | 13.0 | 13.0 | |
10 | 13.0 | 13.0 | 13.0 | 13.0 | 13.0 | 12.5 | 12.5 | 12.5 | |
11 | 13.0 | 13.0 | 13.0 | 13.0 | 13.0 | 13.0 | 13.0 | 13.0 | |
12 | 13.0 | 13.0 | 13.0 | 13.0 | 13.0 | 13.0 | 13.0 | 13.0 | |
Stitch length (mm) | 1 | 1.98 | 1.97 | 2.00 | 1.94 | 1.93 | 1.97 | 1.77 | 2.02 |
2 | 2.15 | 1.95 | 2.05 | 2.10 | 2.07 | 2.06 | 1.95 | 2.12 | |
3 | 2.16 | 2.05 | 2.01 | 2.10 | 2.14 | 2.15 | 2.18 | 2.18 | |
4 | 2.05 | 2.11 | 2.08 | 2.07 | 2.06 | 1.97 | 2.06 | 2.04 | |
5 | 1.90 | 1.83 | 1.79 | 1.94 | 1.83 | 2.08 | 1.81 | 1.78 | |
6 | 1.84 | 1.86 | 1.88 | 1.91 | 1.91 | 1.86 | 1.86 | 1.92 | |
7 | 1.78 | 1.77 | 1.76 | 1.83 | 1.81 | 1.78 | 1.82 | 1.80 | |
8 | 1.86 | 1.87 | 1.90 | 1.84 | 1.88 | 1.88 | 1.87 | 1.80 | |
9 | 1.80 | 1.86 | 1.87 | 1.87 | 1.87 | 1.76 | 1.85 | 1.88 | |
10 | 1.76 | 1.85 | 1.84 | 1.83 | 1.82 | 1.90 | 1.88 | 1.86 | |
11 | 1.50 | 1.57 | 1.66 | 1.68 | 1.67 | 1.71 | 1.73 | 1.61 | |
12 | 1.62 | 1.63 | 1.56 | 1.62 | 1.62 | 1.61 | 1.59 | 1.57 | |
Width of seam allowance (mm) | 1 | 14.4 | 14.0 | 8.0 | 11.8 | 15.4 | 9.0 | 7.2 | 8.2 |
2 | 13.8 | 11.6 | 13.4 | 12.4 | 12.4 | 11.8 | 10.5 | 11.4 | |
3 | 15.2 | 14.6 | 14.6 | 13.6 | 15.0 | 13.6 | 15.2 | 12.6 | |
4 | 16.2 | 15.0 | 13.4 | 14.4 | 13.8 | 14.6 | 13.6 | 17.0 | |
5 | 13.0 | 15.0 | 12.4 | 14.4 | 14.6 | 13.2 | 11.4 | 7.4 | |
6 | 14.8 | 15.4 | 15.8 | 15.0 | 15.0 | 15.6 | 15.2 | 15.0 | |
7 | 15.2 | 15.2 | 13.6 | 14.8 | 14.2 | 13.4 | 14.8 | 14.4 | |
8 | 15.0 | 16.0 | 14.0 | 15.0 | 14.0 | 15.0 | 15.2 | 16.2 | |
9 | 14.2 | 15.0 | 13.8 | 14.6 | 14.8 | 14.8 | 14.0 | 15.2 | |
10 | 15.0 | 16.2 | 15.0 | 15.6 | 15.0 | 15.8 | 14.2 | 14.0 | |
11 | 16.4 | 15.0 | 14.8 | 15.4 | 16.8 | 15.4 | 14.2 | 13.4 | |
12 | 15.2 | 15.6 | 14.6 | 15.6 | 14.2 | 15.6 | 15.8 | 14.8 |
Sewability Factor | Fabric Number | Sewing Speed (RPM) | ||
---|---|---|---|---|
200 | 400 | 800 | ||
SPI | 1 | 13.0 | 13.0 | 13.0 |
2 | 13.0 | 13.0 | 13.0 | |
3 | 13.0 | 13.0 | 13.0 | |
4 | 13.0 | 13.0 | 12.5 | |
5 | 13.0 | 13.0 | 13.5 | |
6 | 13.0 | 12.5 | 12.5 | |
7 | 13.0 | 12.5 | 8.5 | |
8 | 13.0 | 13.0 | 11.5 | |
9 | 12.5 | 13.0 | 13.5 | |
10 | 12.5 | 13.0 | 13.0 | |
11 | 13.0 | 12.5 | 7.0 | |
12 | 13.0 | 13.0 | 13.0 | |
Stitch length (mm) | 1 | 2.0 | 2.0 | 2.0 |
2 | 2.1 | 1.8 | 1.7 | |
3 | 2.2 | 2.2 | 2.1 | |
4 | 2.1 | 2.0 | 1.9 | |
5 | 1.8 | 1.7 | 1.6 | |
6 | 1.9 | 2.2 | 2.2 | |
7 | 1.8 | 2.1 | 3.2 | |
8 | 1.9 | 2.1 | 2.6 | |
9 | 1.8 | 2.0 | 2.0 | |
10 | 1.9 | 2.1 | 2.0 | |
11 | 1.7 | 2.0 | 2.7 | |
12 | 1.6 | 1.9 | 1.7 | |
Width of seam allowance (mm) | 1 | 14.4 | 10.0 | 10.8 |
2 | 13.8 | 9.0 | 7.6 | |
3 | 15.2 | 14.4 | 14.0 | |
4 | 13.4 | 12.4 | 13.2 | |
5 | 15.0 | 12.2 | 12.4 | |
6 | 15.0 | 14.8 | 14.6 | |
7 | 14.8 | 14.4 | 14.4 | |
8 | 15.0 | 13.6 | 15.0 | |
9 | 14.8 | 14.0 | 15.0 | |
10 | 15.8 | 13.2 | 14.6 | |
11 | 14.2 | 14.2 | 15.6 | |
12 | 14.8 | 14.4 | 14.6 |
Sewability Factor | Fabric Number | Sewing Speed (RPM) | ||
---|---|---|---|---|
200 | 400 | 800 | ||
SPI | 6 | 12.5 | 13.5 | 13.0 |
7 | 12.5 | 12.5 | 12.5 | |
8 | 13.0 | 13.5 | 13.0 | |
9 | 13.0 | 12.5 | 12.5 | |
10 | 13.0 | 13.0 | 12.5 | |
11 | 11.5 | 12.5 | 8.0 | |
12 | 11.5 | 12.5 | 9.5 | |
Stitch width (mm) | 6 | 3.9 | 4.6 | 3.4 |
7 | 3.4 | 3.1 | 3.0 | |
8 | 4.2 | 4.9 | 4.9 | |
9 | 4.1 | 5.1 | 5.1 | |
10 | 5.2 | 5.7 | 5.9 | |
11 | 4.1 | 5.5 | 4.1 | |
12 | 3.2 | 4.8 | 3.3 |
Fabric Number | Thickness (mm) | Weight (g/m ) | Density | Tensile Strength (N/cm) | Elongation (%) | Flex Stiffness (mN·m) | Surface Roughness (mm) |
---|---|---|---|---|---|---|---|
1 | 0.08 | 39 | 361 | 71.2 | 42.5 | 0.04 | 1.25 |
2 | 0.10 | 68 | 270 | 124.0 | 35.9 | 0.10 | 1.75 |
3 | 0.30 | 115 | 204 | 113.3 | 51.3 | 0.04 | 3.52 |
4 | 0.38 | 170 | 137 | 57.7 | 34.4 | 0.06 | 7.02 |
5 | 0.56 | 225 | 186 | 59.7 | 285.7 | 0.04 | 0.95 |
6 | 0.76 | 271 | 116 | 145.7 | 17.4 | 0.30 | 12.87 |
7 | 0.90 | 417 | 194 | 302.5 | 257.5 | 0.61 | 1.31 |
8 | 0.94 | 316 | 84 | 100.8 | 14.8 | 0.48 | 10.08 |
9 | 0.94 | 411 | 120 | 244.2 | 26.3 | 3.18 | 5.75 |
10 | 0.95 | 362 | 108 | 211.2 | 20.9 | 0.80 | 6.82 |
11 | 1.06 | 342 | 207 | 91.0 | 219.2 | 0.25 | 2.35 |
12 | 1.51 | 517 | 84 | 111.0 | 49.4 | 1.19 | 2.29 |
Textile Property | Sewability Factor | |||
---|---|---|---|---|
SPI | Stitch Length | Seam Allowance | Seam Strength | |
Stiffness | −0.046 | −0.233 * | 0.254 ** | −0.337 ** |
Thickness | −0.125 | −0.414 ** | 0.566 ** | −0.779 ** |
Weight | −0.160 | −0.376 ** | 0.546 ** | −0.781 ** |
Density | 0.005 | 0.178 | −617 ** | 0.746 ** |
Roughness | −0.019 | 0.111 | 0.392 ** | −0.284 ** |
Tensile strength | −0.053 | −0.068 | 0.204 * | −0.098 |
Elongation | −0.024 | −0.137 | −0.036 | −0.322 ** |
Effect Factor | Sewing Condition | ||
---|---|---|---|
Presser Height | Sewing Speed | ||
Textile properties | Stiffness | 0.116 | 0.000 |
Thickness | 0.200 * | 0.000 | |
Weight | 0.193 * | 0.000 | |
Density | −0.149 | 0.000 | |
Roughness | 0.064 | 0.000 | |
Tensile strength | 0.069 | 0.000 | |
Elongation | 0.000 | 0.000 | |
Sewability factor | SPI | −0.063 | −0.264 ** |
Stitch length | 0.085 | 0.376 ** | |
Seam allowance | −0.027 | −0.132 | |
Seam strength | −0.187 * | −0.026 |
Model | Unstandardized Coefficients | Standardized Coefficients | R | ΔR | ΔF | |||
---|---|---|---|---|---|---|---|---|
B | Std. Error | β | ||||||
Thickness | 1 | (Constant) | 61.145 | 3.383 | 0.608 | 0.001 | 0.291 | |
Thickness | −47.575 | 3.641 | −0.772 ** | |||||
Presser Height | −0.697 | 1.292 | −0.032 | |||||
2 | (Constant) | 71.110 | 4.458 | 0.641 | 0.033 | 10.674 * | ||
Thickness | −63.339 | 5.975 | −1.029 ** | |||||
Presser Height | −6.953 | 2.282 | −0.318 * | |||||
T-×-P | 9.135 | 2.796 | 0.459 * | |||||
Weight | 1 | (Constant) | 64.683 | 3.539 | 0.611 | 0.001 | 0.409 | |
Weight | −0.136 | 0.010 | −0.774 ** | |||||
Presser Height | −0.821 | 1.284 | −0.038 | |||||
2 | (Constant) | 75.074 | 4.779 | 0.641 | 0.030 | 9.657 * | ||
Weight | −0.179 | 0.017 | −1.017 ** | |||||
Presser Height | −7.411 | 2.456 | −0.339 * | |||||
W-×-P | 0.025 | 0.008 | 0.456 * |
Testing Sample | Seam Strength | |||||||
---|---|---|---|---|---|---|---|---|
By Equation (2) | By Equation (3) | |||||||
Thickness (mm) | Weight (g/m ) | Experimental value | Predicted value | Error | Experimental value | Predicted value | Error | |
1 | 0.29 | 70 | 56.4 | 50.6 | 5.8 | 56.4 | 59.7 | 3.3 |
2 | 0.76 | 271 | 20.0 | 23.0 | 3.0 | 20.0 | 25.9 | 5.9 |
3 | 0.94 | 316 | 14.8 | 14.0 | 0.8 | 14.8 | 19.2 | 4.4 |
Textile Properties | Sewability Factor | ||
---|---|---|---|
SPI | Stitch Width | Seam Strength | |
Stiffness | 0.086 | 0.019 | −0.506 ** |
Thickness | −0.424 * | −0.168 | −0.301 |
Weight | −0.354 * | −0.276 | −0.369 * |
Density | −0.348 * | −0.198 | 0.142 |
Roughness | 0.503 ** | 0.256 | 0.207 |
Tensile strength | 0.272 | 0.367 * | 0.053 |
Elongation | −0.442 ** | −0.354 * | 0.051 |
Effect Factor | Sewing Condition | ||
---|---|---|---|
Presser Height | Sewing Speed | ||
Textile properties | Stiffness | 0.100 | 0.000 |
Thickness | 0.698 ** | 0.000 | |
Weight | 0.470 ** | 0.000 | |
Density | −0.100 | 0.000 | |
Roughness | −0.376 * | 0.000 | |
Tensile strength | −0.185 | 0.000 | |
Elongation | 0.023 | 0.000 | |
Sewability factor | SPI | −0.416 * | −0.155 |
Stitch width | 0.221 | 0.297 | |
Seam strength | −0.211 | −0.235 |
MDPI stays neutral with regard to jurisdictional claims in published maps and institutional affiliations. |
Share and Cite
Lee, S.; Rho, S.; Lim, D.; Jeong, W. A Basic Study on Establishing the Automatic Sewing Process According to Textile Properties. Processes 2021 , 9 , 1206. https://doi.org/10.3390/pr9071206
Lee S, Rho S, Lim D, Jeong W. A Basic Study on Establishing the Automatic Sewing Process According to Textile Properties. Processes . 2021; 9(7):1206. https://doi.org/10.3390/pr9071206
Lee, Suhyun, Soohyeon Rho, Daeyoung Lim, and Wonyoung Jeong. 2021. "A Basic Study on Establishing the Automatic Sewing Process According to Textile Properties" Processes 9, no. 7: 1206. https://doi.org/10.3390/pr9071206
Article Metrics
Article access statistics, further information, mdpi initiatives, follow mdpi.
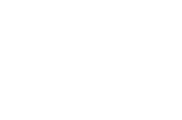
Subscribe to receive issue release notifications and newsletters from MDPI journals
Academia.edu no longer supports Internet Explorer.
To browse Academia.edu and the wider internet faster and more securely, please take a few seconds to upgrade your browser .
- We're Hiring!
- Help Center
Sewing Machine
- Most Cited Papers
- Most Downloaded Papers
- Newest Papers
- Elias Howe Follow Following
- Fashion History Follow Following
- The Industrial Revolution Follow Following
- History of Technology Follow Following
- Women's History Follow Following
- Military History Follow Following
- Cultural History Follow Following
Enter the email address you signed up with and we'll email you a reset link.
- Academia.edu Journals
- We're Hiring!
- Help Center
- Find new research papers in:
- Health Sciences
- Earth Sciences
- Cognitive Science
- Mathematics
- Computer Science
- Academia ©2024
To read this content please select one of the options below:
Please note you do not have access to teaching notes, the study of sewing damage and defects in garments.
Research Journal of Textile and Apparel
ISSN : 1560-6074
Article publication date: 8 May 2018
Issue publication date: 24 May 2018
The purpose of this review paper is to define the dominating factors (such as fiber, yarn, fabric structure, sewing thread, sewing needle and machine parameters) that affect the seam damages and causing defects. It also describes the various explanations of sewing defects in garment production and critically analyzes them for optimum selection of parameters and speeds for minimizing such faults. Hence, the knowledge of various factors which affect the sewing damages/defects will be helpful for garment manufacturers/researchers to know influence of the parameters and control the quality of producing seam.
Design/methodology/approach
This section is not applicable for a review paper.
Sewing damages such as needle cut and other sewing damages/defects are studied mostly in woven fabric. There are very few studies conducted on knitted fabric sewing damages/defects. The sewing damage problems do not have single solution that is capable of removing these damages in fabric. All the determined and affecting parameters related to fiber, yarn, fabric construction, sewing thread and sewing machine must be examined to design appropriate remedial measurement related to machine design, fabric parameters and sewing thread. This could help in minimizing or eliminating the needle cut and other sewing damage problems.
Originality/value
It is an original review work and is helpful for garment manufacturers/researchers to reduce the defects and be able to produce good quality seam.
- Fabric sewability
- Needle cut index
- Sewing damages
- Sewing defects
- Sewing needle
- Sewing thread
Choudhary, A.K. , Sikka, M.P. and Bansal, P. (2018), "The study of sewing damage and defects in garments", Research Journal of Textile and Apparel , Vol. 22 No. 2, pp. 109-125. https://doi.org/10.1108/RJTA-08-2017-0041
Emerald Publishing Limited
Copyright © 2018, Emerald Publishing Limited
Related articles
All feedback is valuable.
Please share your general feedback
Report an issue or find answers to frequently asked questions
Contact Customer Support
The Rise and Fall of the First American Patent Thicket: The Sewing Machine War of the 1850s
Arizona Law Review, Vol. 53, pp. 165-211, 2011
George Mason Law & Economics Research Paper No. 09-19
47 Pages Posted: 6 Mar 2009 Last revised: 10 Jun 2012
Adam Mossoff
George Mason University - Antonin Scalia Law School
Date Written: March 6, 2009
When Michael Heller proposed that excessively fragmented property rights in land can frustrate its commercial development, patent scholars began debating whether Heller's anticommons theory applies to property rights in inventions. Do "patent thickets" exist? The rise and fall of the first American patent thicket -- the Sewing Machine War of the 1850s -- confirms that patent thickets do exist and that they can frustrate commercial development of new products. But this historical patent thicket also challenges the widely held assumption that this is a modern problem arising from allegedly new issues in the patent system, such as incremental high-tech innovation and the impact of "patent trolls." The Sewing Machine War exhibited all of these phenomena, proving that these are hoary issues in patent law. The denouement of this patent thicket in the Sewing Machine Combination of 1856, the first privately formed patent pool, further challenges the conventional wisdom that patent thickets are best solved through public-ordering regimes that limit property rights in patents. The invention and incredible commercial success of the sewing machine is a striking account of early American technological, commercial, and legal ingenuity, which heralds important empirical lessons for how patent thicket theory is understood and applied today.
Keywords: A Stitch in Time: The Rise and Fall of the Sewing Machine Patent Thicket, commercial trust, e-Bay v. MercExchange, follow-on inventions, Industrial Revolution, Howe, Krems, Madersperger, monopoly, Newton, Singer, Song of the Shirt, Thimonnier, Useful Arts, Weisenthal
JEL Classification: D23, K11, O34
Suggested Citation: Suggested Citation
Adam Mossoff (Contact Author)
George mason university - antonin scalia law school ( email ).
3301 Fairfax Drive Arlington, VA 22201 United States 703-993-9577 (Phone)
Do you have a job opening that you would like to promote on SSRN?
Paper statistics, related ejournals, antonin scalia law school, george mason university, law & economics research paper series.
Subscribe to this free journal for more curated articles on this topic
Legal History eJournal
Cyberspace law ejournal.
Subscribe to this fee journal for more curated articles on this topic
Entrepreneurship & Law eJournal
Io: productivity, innovation & technology ejournal, intellectual property: patent law ejournal, law & society: international & comparative law ejournal, law & society: private law - intellectual property ejournal, economic history ejournal, young scholars law ejournal, institutions & transition economics: microeconomic issues ejournal, legal information, technology & law librarianship ejournal, innovation law & policy ejournal, recommended papers.
Efficient Patent Pools
By Jean Tirole and Josh Lerner
Regulating Network Industries: A Look at Intel
By Randal C. Picker
Cooperative Marketing Agreements between Competitors: Evidence from Patent Pools
By Marcin Strojwas , Jean Tirole , ...
By Jean Tirole , Marcin Strojwas , ...
Patent Pools and Cross-Licensing in the Shadow of Patent Litigation
By Jay Pil Choi
Do Patent Pools Encourage Innovation? Evidence from the 19th-Century Sewing Machine Industry
By Ryan Lampe and Petra Moser
IEEE Account
- Change Username/Password
- Update Address
Purchase Details
- Payment Options
- Order History
- View Purchased Documents
Profile Information
- Communications Preferences
- Profession and Education
- Technical Interests
- US & Canada: +1 800 678 4333
- Worldwide: +1 732 981 0060
- Contact & Support
- About IEEE Xplore
- Accessibility
- Terms of Use
- Nondiscrimination Policy
- Privacy & Opting Out of Cookies
A not-for-profit organization, IEEE is the world's largest technical professional organization dedicated to advancing technology for the benefit of humanity. © Copyright 2024 IEEE - All rights reserved. Use of this web site signifies your agreement to the terms and conditions.
- DOI: 10.1109/ICE.2017.8279995
- Corpus ID: 3432191
Monitoring and control of industrial sewing machines research on thread tension behavior in lockstitch machines
- Patricia Mellero , Sandra Biegas , +1 author F. Ferreira
- Published in International Conference on… 1 June 2017
- Engineering, Materials Science
Figures and Tables from this paper
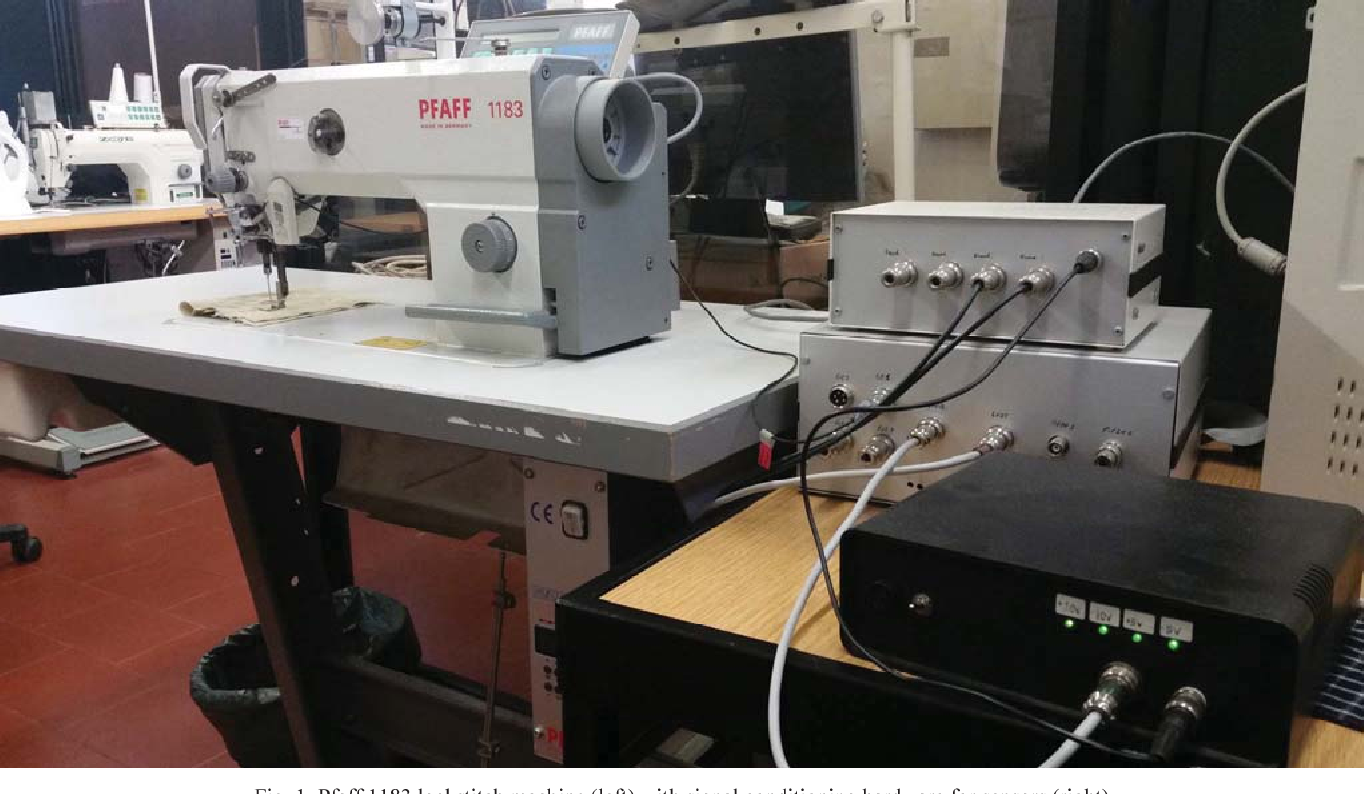
2 Citations
Fabric properties measurements using real-time sewing machine motor current signature, design and fabrication of an automatic tension monitoring and regulation system for needle thread, 16 references, parameter monitoring and control in industrial sewing machines - an integrated approach.
- Highly Influential
Measurement and analysis of needle penetration forces in industrial high-speed sewing machine
Automatic presser‐foot force control for industrial sewing machines, a study of thread tensions on a lockstitch sewing machine (part ii), sewing dynamics: objective measurement of fabric/machine interaction, impact of sewing needle and thread on the technological process of sewing knitwear, study on dynamic needle thread tensions in a single needle lock stitch (snls) sewing machine. ii. effect of sewing speed, thickness of fabric plies, thread linear density and pre-tensions of threads, thread tension control of permanent magnet synchronous motor based on load torque observer, the principles of intelligent textile and garment manufacturing systems, a study of thread tensions on a lockstitch sewing machine (part iii): further stitch formation analysis, related papers.
Showing 1 through 3 of 0 Related Papers
Start your sewing adventure with us.
Join Seamwork Now
Buttonhole sewing secrets every beginner should learn, a step-by-step guide to understanding, sewing, cutting, and troubleshooting buttonholes for perfect results every time., posted in: tutorials & techniques • september 18, 2024.
DEK: A step-by-step guide to understanding, sewing, cutting, and troubleshooting buttonholes for perfect results every time.
When I ask people what they find trickiest in sewing, buttonholes are always at the top of the list. They can feel intimidating, but with a few key techniques, buttonholes will become just another simple step in your sewing process.
If you’ve struggled with buttonholes in the past, have no fear. I’m going to share all the secrets you need to know, from start to finish. This is your complete guide to buttonholes. Watch the video above to see these tips in action, and keep reading for detailed notes.
In this guide, you’ll learn about:
- The tools and notions you’ll need
- How to select the right type of buttonhole for your fabric
- How to mark buttonhole placement
- Why you should stabilize your fabric
- How to create, cut, and reinforce your buttonholes
- Plus, some tips for troubleshooting common buttonhole issues
The Tools and Notions You’ll Need
Before you start, make sure you have the right tools. A good setup makes all the difference when it comes to smooth buttonhole sewing.
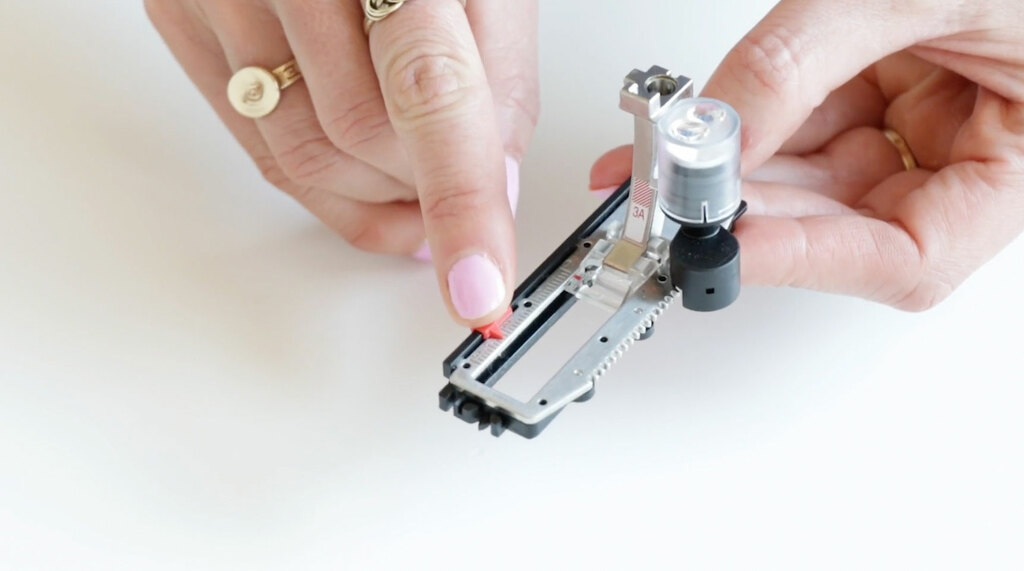
You’ll need:
- A sewing machine with a buttonhole foot (some machines have automatic buttonholes, while others require manual stitching)
- A water-soluble fabric marker
- Interfacing or stabilizer
- Sharp scissors or a buttonhole cutter (this specialized tool makes clean cuts and precise finishes)
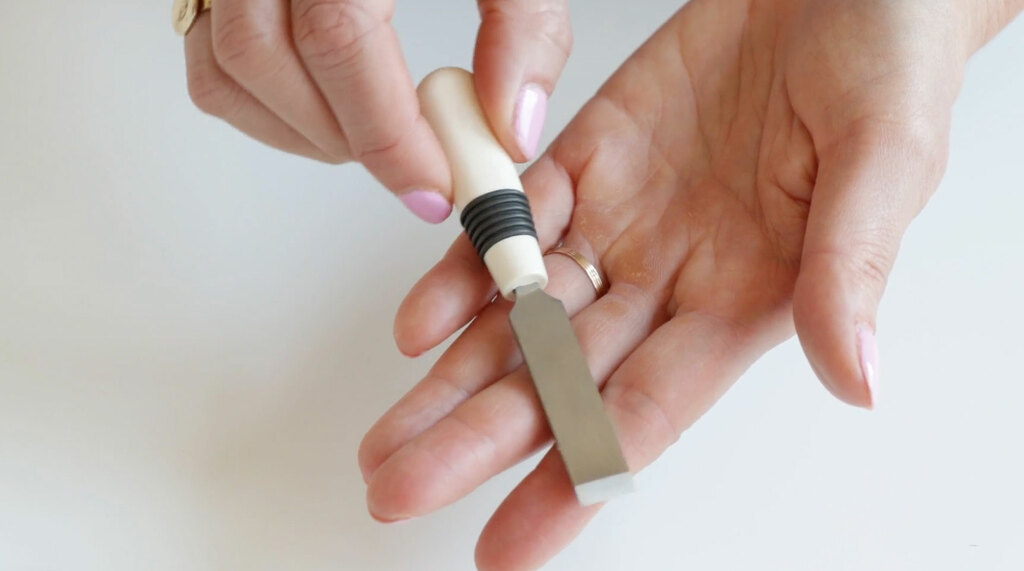
What’s a buttonhole cutter? It’s a sharp tool designed to cut your buttonholes with ease, helping you avoid fraying or damaging your fabric.
If you don’t have one, sharp scissors work too!

Choosing the Right Type of Buttonhole
Not all buttonholes are created equal. Depending on your fabric and the type of garment you're sewing, you’ll want to select the right buttonhole style for the job.
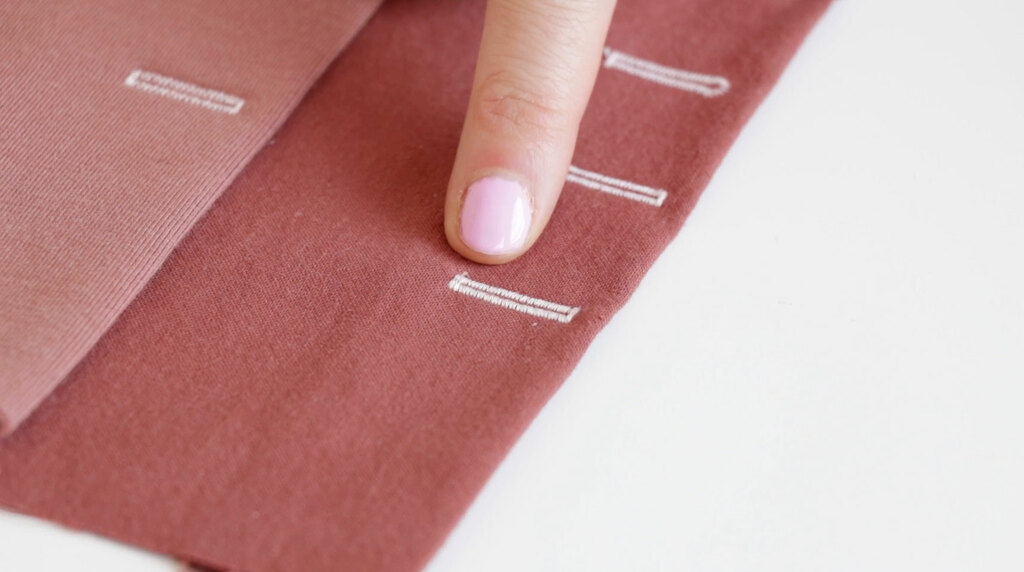
Classic rectangular buttonhole : A regular buttonhole is ideal for medium-weight woven fabrics, and it will likely be the buttonhole you use most frequently.
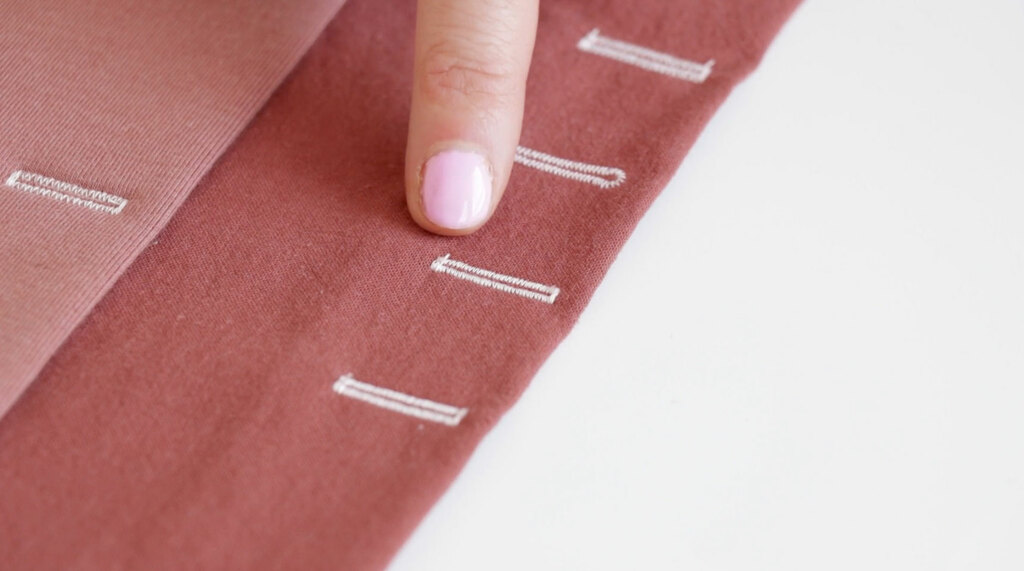
Narrow buttonhole : Perfect for lightweight fabrics, like silk or voile. If you don’t have this function on your machine, you can reduce the width on your buttonhole stitch to make it more delicate.
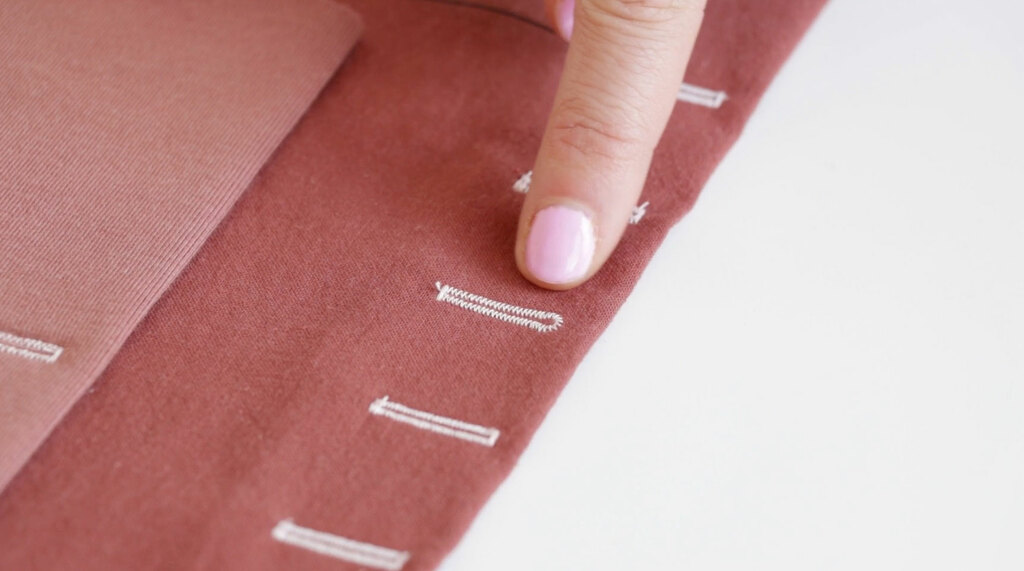
Keyhole buttonhole : These are best for heavier fabrics, jackets, or coats. The round end helps accommodate thick buttons.
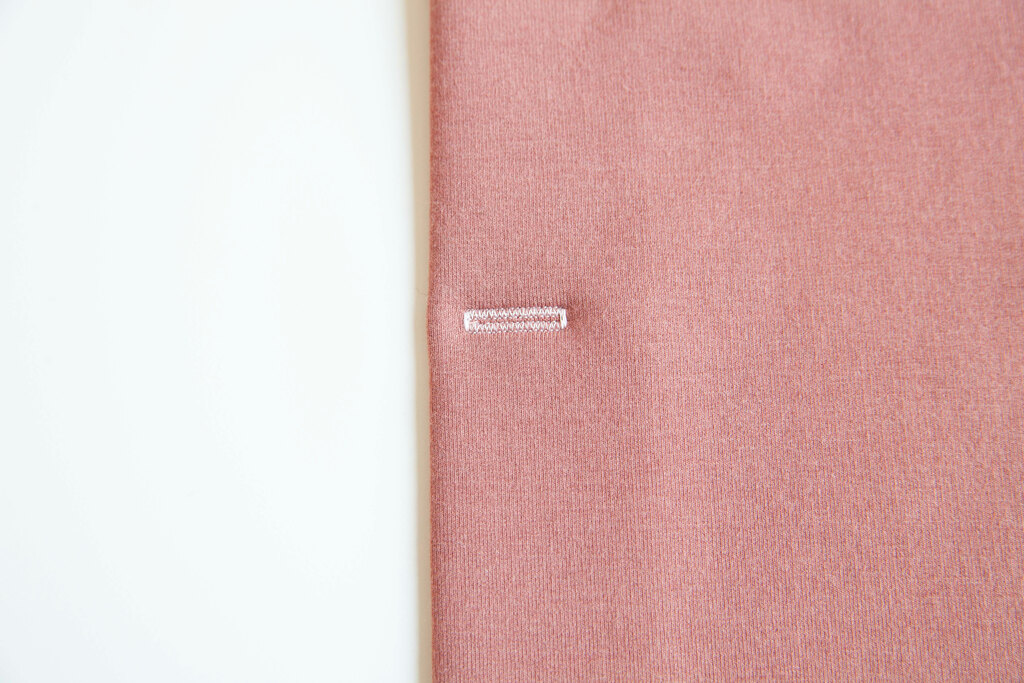
Stretch (or knit) buttonhole : This buttonhole is designed for knits and stretchy fabrics. It moves with the fabric. If your machine doesn’t have this function, increase the stitch length to add flexibility to the buttonhole.
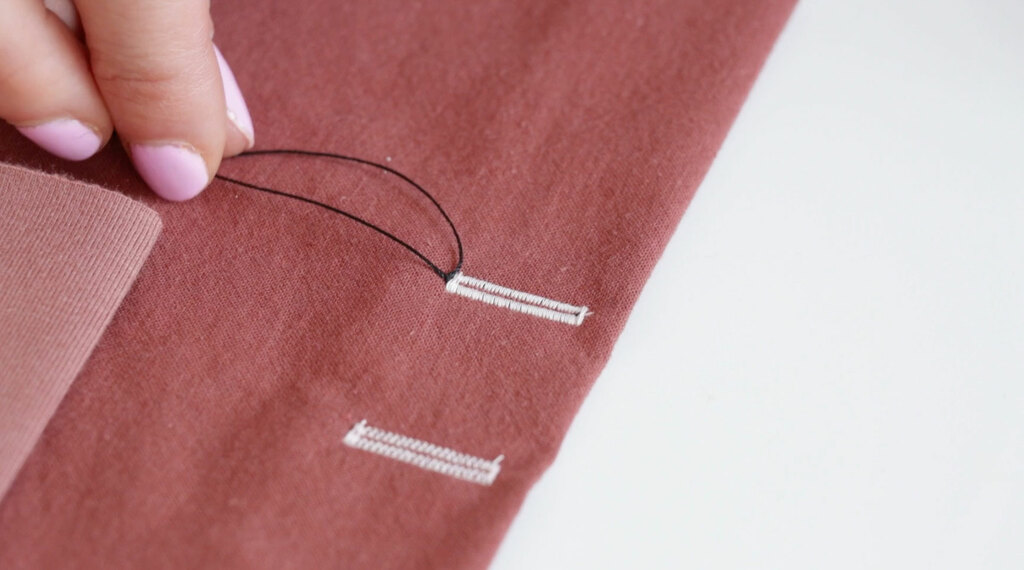
Corded buttonhole : This is a type of buttonhole reinforced with a cord to provide extra strength and durability. It’s particularly useful for fabrics that might fray easily, such as knits, lightweight wovens, or delicate fabrics.
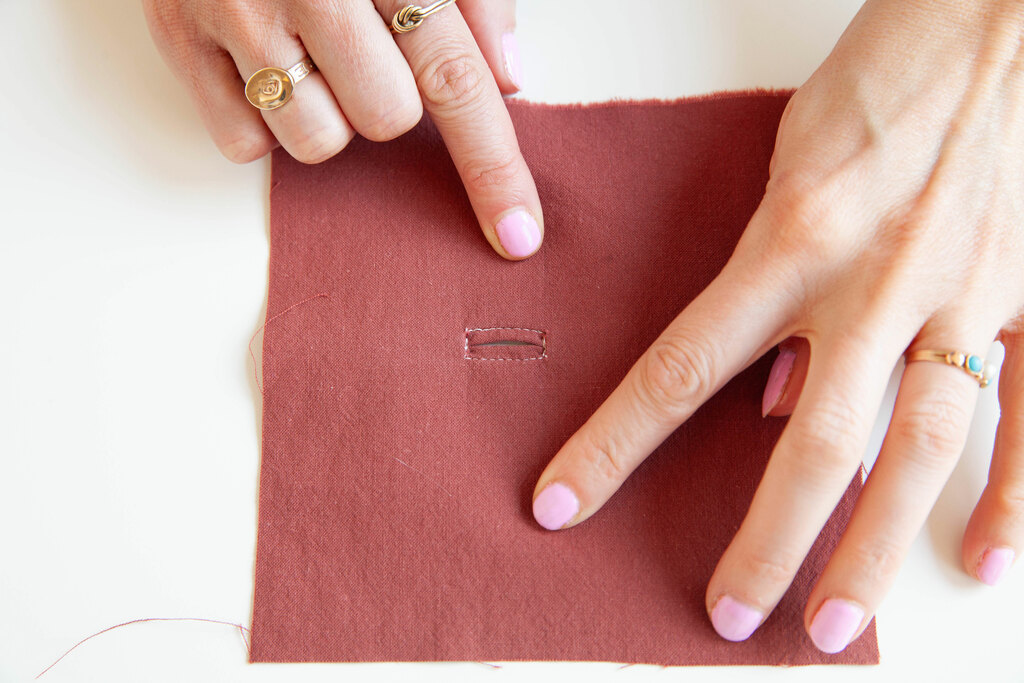
Bound buttonholes : You can create a bound buttonhole as a tailored option for outerwear or more formal garments. It’s almost like a little welt pocket. If you want a tutorial on bound buttonholes, let me know!
You can sew all of these on a machine, but you can also hand sew a buttonhole, which is a beautiful couture detail for special projects.
Marking Your Buttonholes
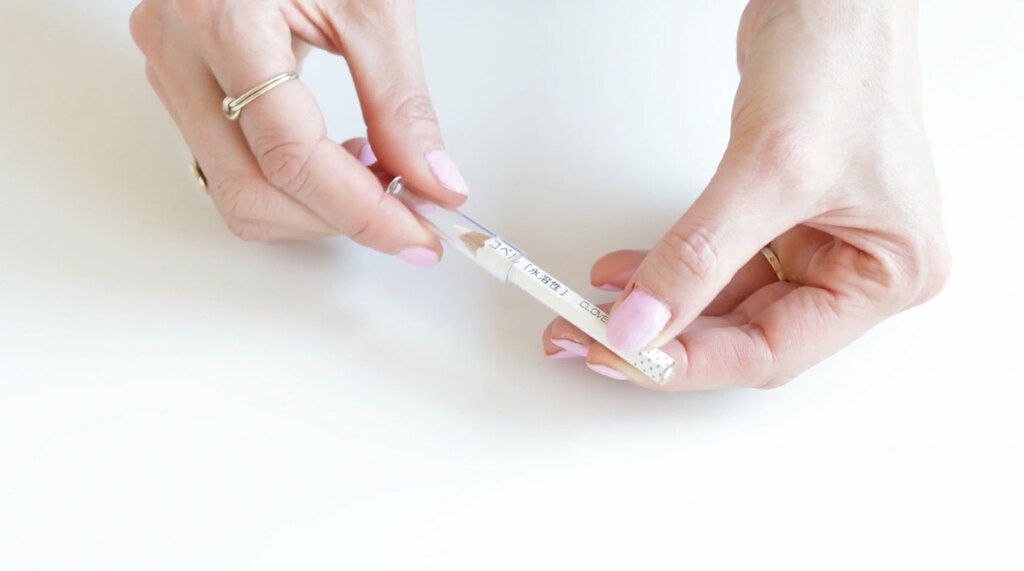
Most patterns will include buttonhole markings to match the recommended button size. If you're using a different size button, measure the button and add about 1/8 inch (3 mm) to the length to ensure it fits.
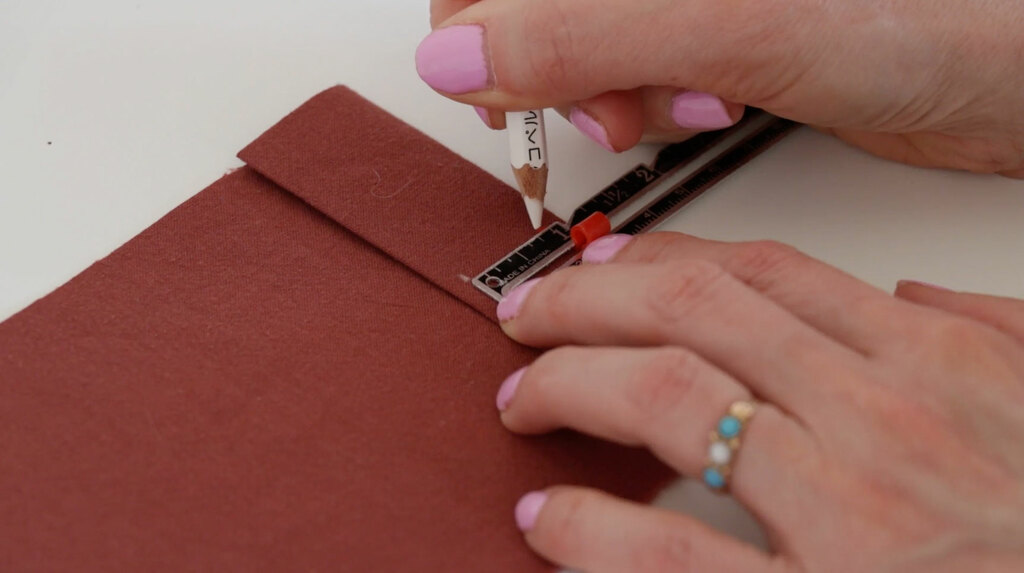
Use a water-soluble marker to mark the start and end points of each buttonhole. If you're adjusting button placement, consider using an expandable button gauge to ensure even spacing. Larger buttons may require you to move buttonholes further from the edge of the fabric, while smaller ones might need to be placed closer.
Stabilizing the Fabric
Stabilizing your fabric is key to long-lasting buttonholes. Most patterns call for interfacing in areas where buttonholes will go. Interfacing strengthens the fabric, making sure your buttonholes can withstand repeated wear.
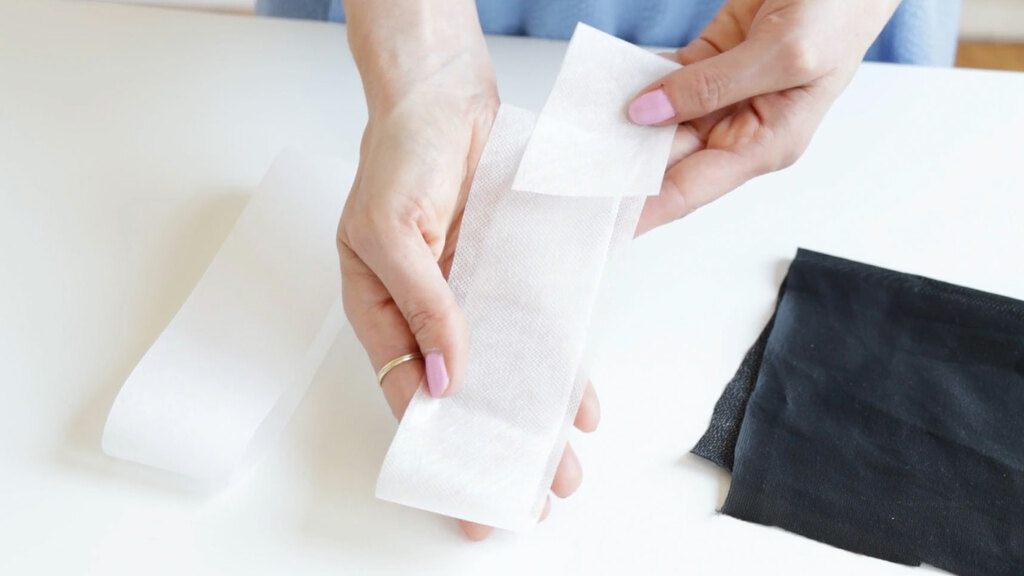
For lightweight or stretchy fabrics, consider using a wash-away or tear-away stabilizer in addition to interfacing. This prevents the fabric from puckering or shifting as you sew.
Setting Up Your Sewing Machine
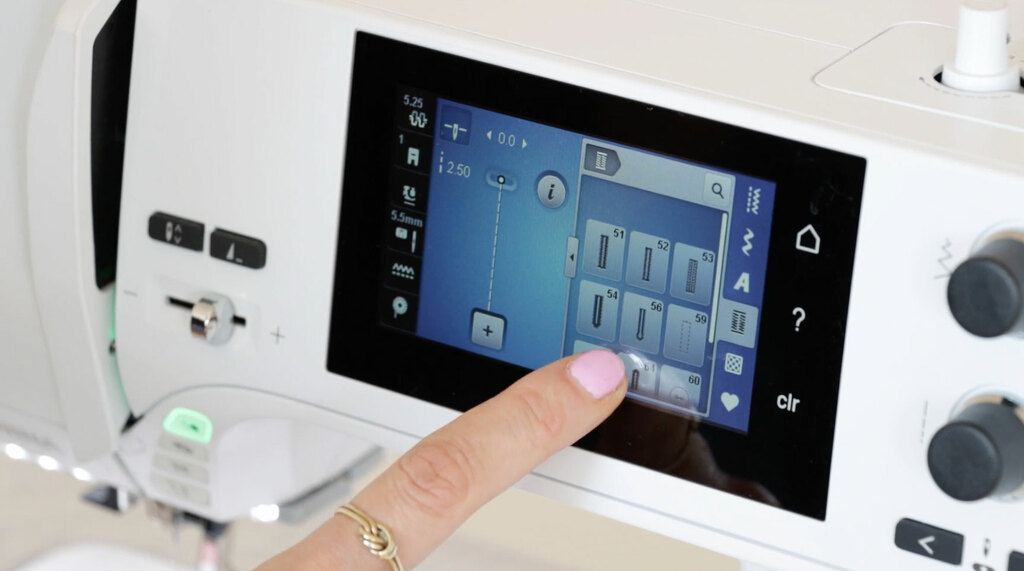
Once your fabric is marked and stabilized, it’s time to set up your machine:
Attach your buttonhole foot and select the stitch that matches the buttonhole style you want.
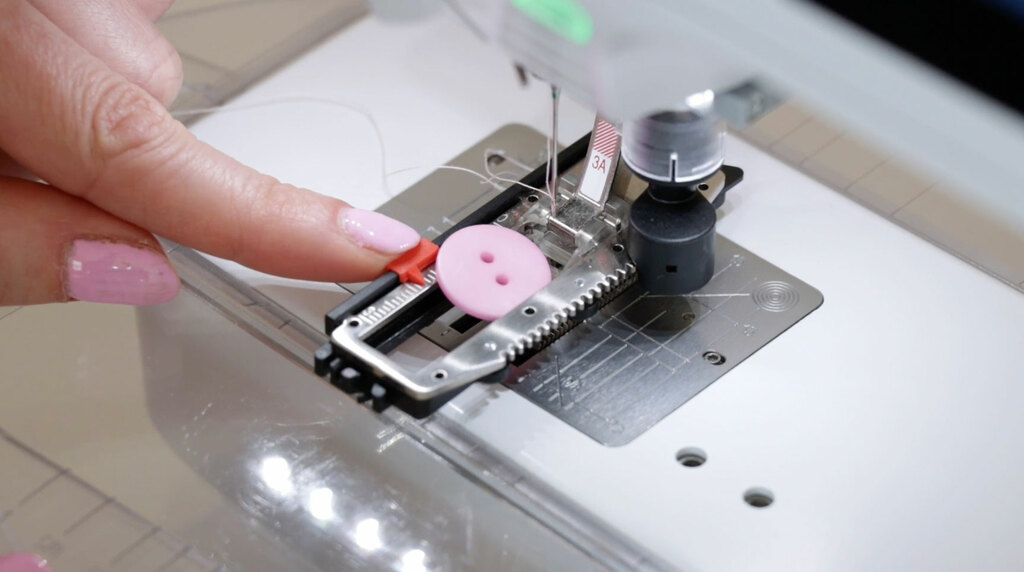
Use the buttonhole foot’s slider to mark the length of the buttonhole.
Follow your machine’s manual for specific settings. Each machine has its own instructions for creating buttonholes, so it’s worth taking a moment to check.
Here's an important tip: Never start a buttonhole on your actual garment without testing it first. Use a scrap piece of fabric that matches your project, including any interfacing or stabilizer.
Test your buttonhole and make sure:
- Your button fits easily through the hole.
- There are no skipped stitches or tension issues.
Adjust the buttonhole length if needed before sewing on your garment.
Creating the Buttonhole
To sew the buttonhole, start stitching at the top of your marked line.
Sew continuously to avoid stopping or pausing mid-stitch.
Once you reach the end of the buttonhole, use the backstitch function on your machine to finish it off.
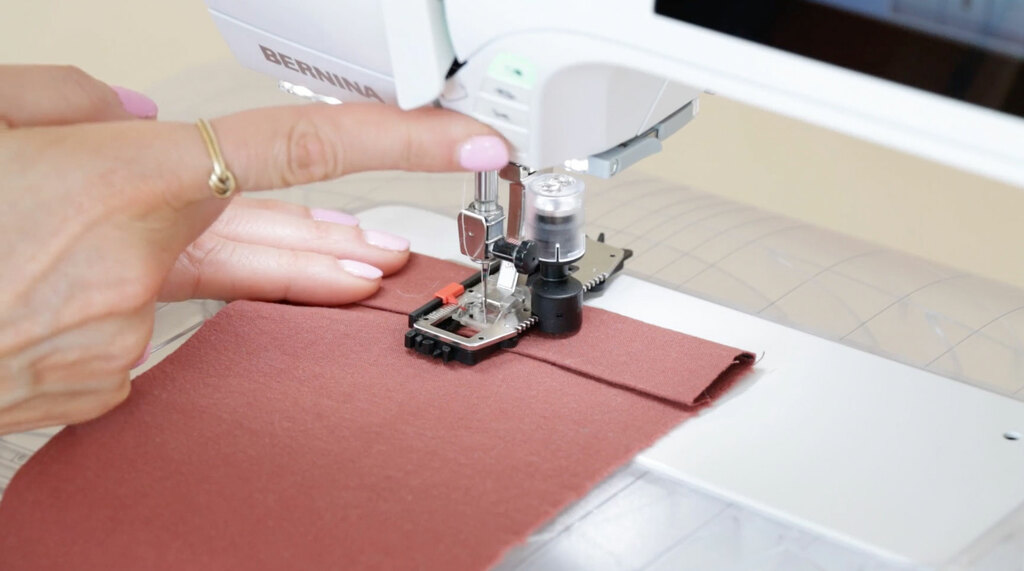
Repeat for the remaining buttonholes, following the same process.
Cutting the Buttonhole
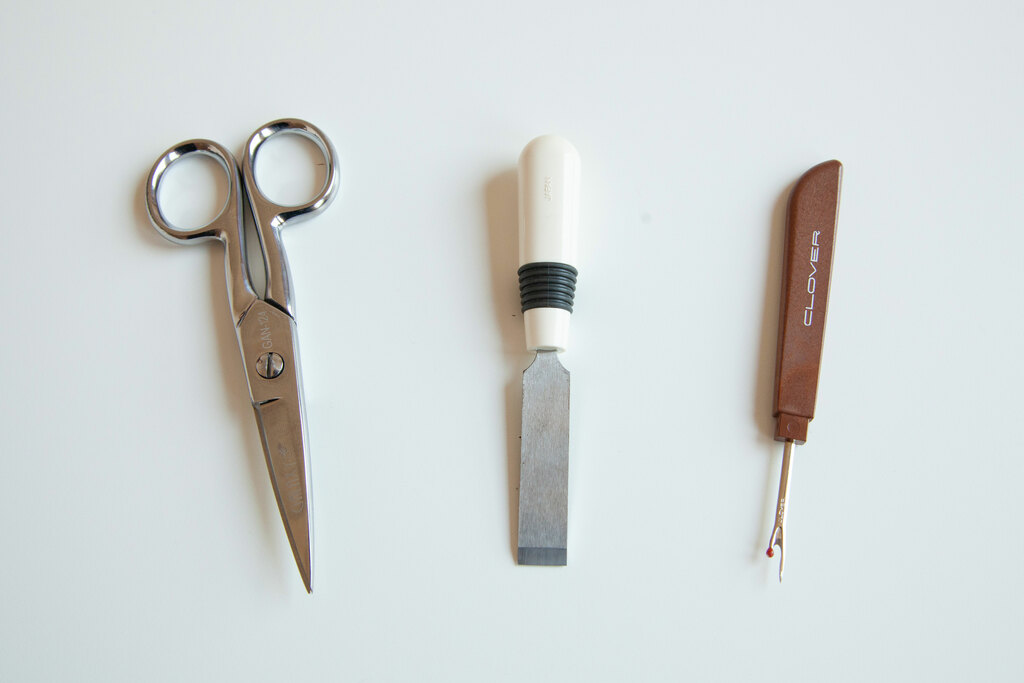
Cutting your buttonhole is a delicate step. If you use sharp scissors or a seam ripper, place pins at both ends of the buttonhole to prevent overcutting. Start from the center and carefully cut towards the ends.
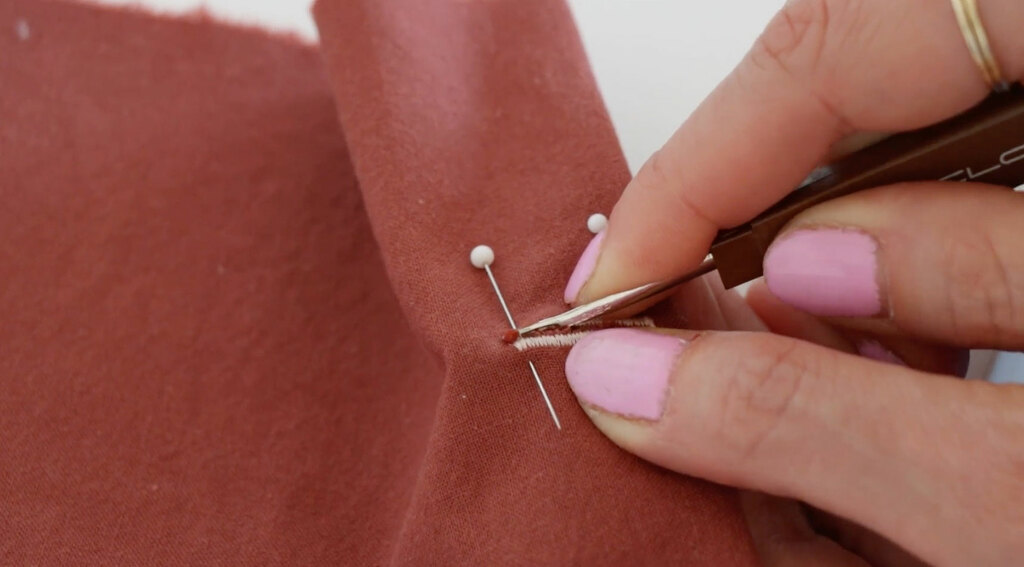
If you use a buttonhole cutter, just place your garment over a cutting mat and chisel it.
Reinforcing the Buttonhole
Here are a few tips for reinforcing your buttonholes to make sure they last:
- After you sew, press the stitches gently with an iron.
- Apply fray check to the edges before cutting and it will secure your buttonhole and keep the threads from coming loose. Avoid putting fray check on any water-soluble markings, because it will seal the marking and make it permanent. So, rinse of the markings before applying fray check.
- Add bar tacks at the ends for extra strength, especially on thick fabrics.
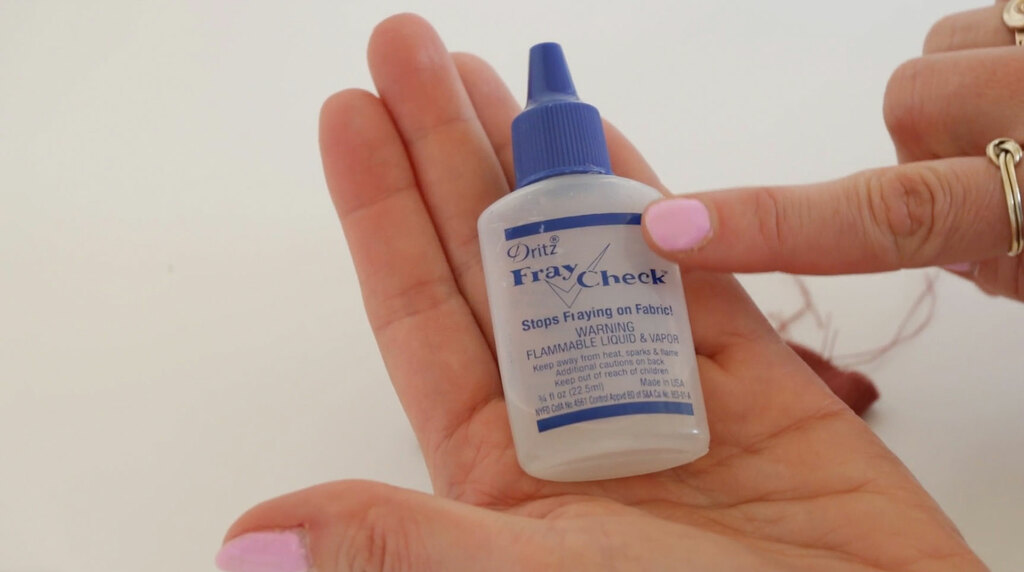
Fixing Common Buttonhole Issues
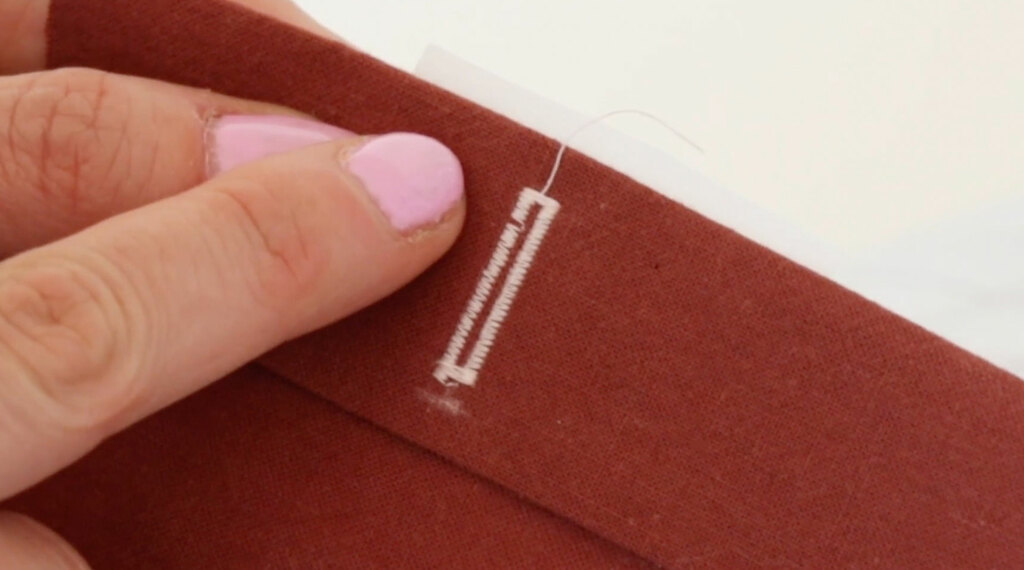
- pply fray check to the edges before cutting and it will secure your buttonhole and keep the threads from coming loose. Avoid putting fray check on any water-soluble markings, because it will seal the marking and make it permanent. So, rinse of the markings before applying fray check.
Final Tip: Using Buttonhole Gimp
Want to create a super stable buttonhole? Try using buttonhole gimp. It’s a thicker thread that reinforces the buttonhole, helping it hold its shape. You can loop the gimp onto your buttonhole foot, and your machine will stitch over it, giving you a sharp, defined finish. This is especially useful for tailored garments or stretchy fabrics.
Read this article and watch the video to learn how to use it: How to Sew Tricky Buttonholes .
Now that you’ve got all the tips you need, you’re ready to start sewing buttonholes with confidence!
Join the Conversation
Sign in or become a seamwork member to comment on this article., you may also like.
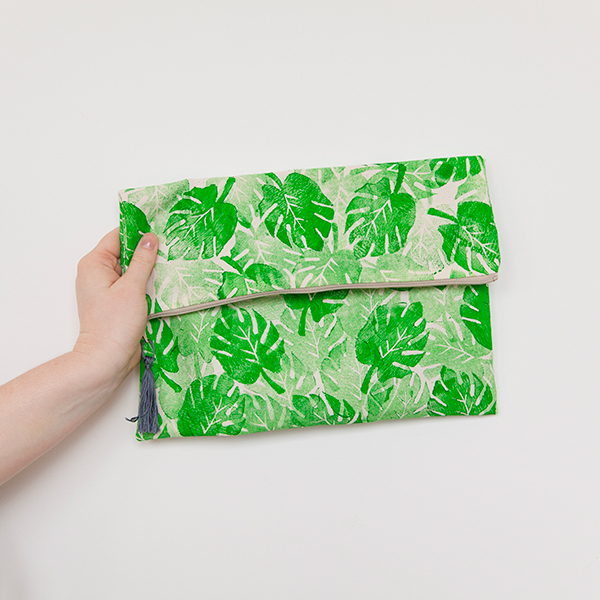
Tutorials & Techniques
Creating block printed textiles.
Add a graphic pop of color to your next project with block printed fabric, by Haley Glenn.
April 30, 2017 0
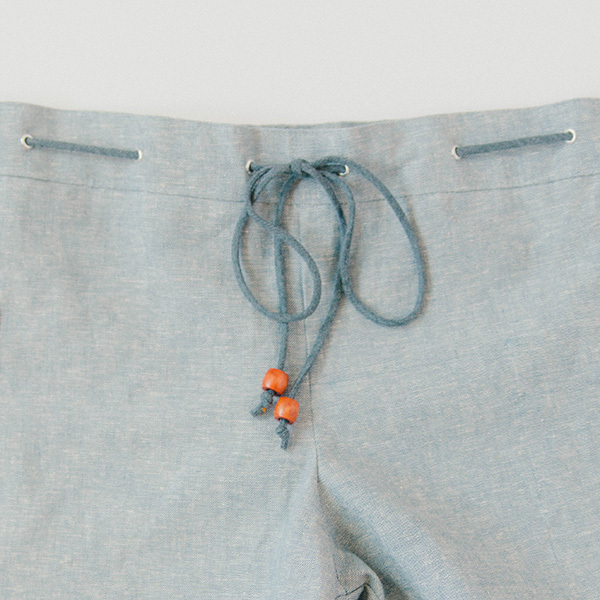
A Guide to Drawstrings
A simple detail that offers a range of design possibilities.
June 30, 2015 0
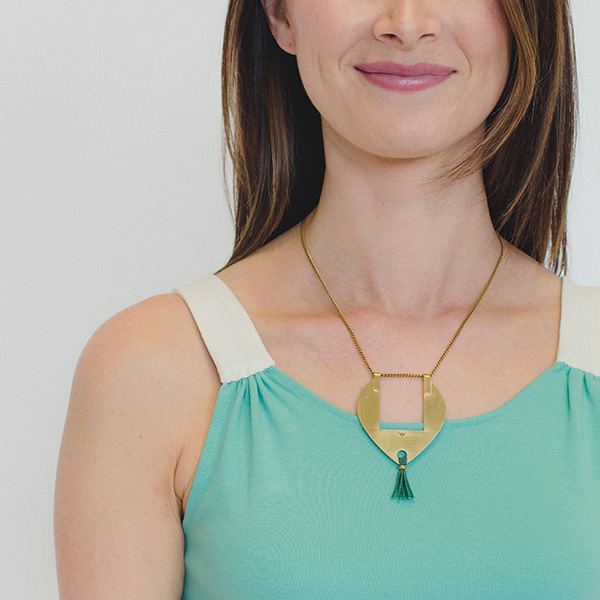
Sewing Knits Without a Serger
Simple tips for using your home sewing machine to sew knit fabrics. By Haley Glenn.
May 31, 2015 0
Explore more seamwork, seamwork members have access to hundreds of patterns, with two new patterns available each month. membership starts at just $15 per month, get inspired & stay in the know:.
Evaluation of Reservoir Porosity and Permeability from Well Log Data Based on an Ensemble Approach: A Comprehensive Study Incorporating Experimental, Simulation, and Fieldwork Data
- Original Paper
- Published: 18 September 2024
Cite this article
- Edwin E. Nyakilla ORCID: orcid.org/0000-0002-7402-2611 1 , 2 ,
- Sun Guanhua 1 , 2 ,
- Hao Hongliang 2 ,
- Grant Charles 3 ,
- Mouigni B. Nafouanti 3 ,
- Emanuel X. Ricky 3 ,
- Selemani N. Silingi 3 , 4 ,
- Elieneza N. Abelly 3 ,
- Eric R. Shanghvi 3 ,
- Safi Naqibulla 3 ,
- Mbega R. Ngata 3 ,
- Erasto Kasala 3 ,
- Melckzedeck Mgimba 5 ,
- Alaa Abdulmalik 3 ,
- Fatna A. Said 7 ,
- Mbula N. Nadege 3 ,
- Johnson J. Kasali 6 &
Permeability and porosity are key parameters in reservoir characterization for understanding hydrocarbon flow behavior. While traditional laboratory core analysis is time-consuming, machine learning has emerged as a valuable tool for more efficient and accurate estimation. This paper proposes an ensemble technique called adaptive boosting (AdaBoost) for porosity and permeability estimation, utilizing methods such as support vector machine (SVM), Gaussian process regression (GPR), multivariate analysis, and backpropagation neural network (BPNN) for prediction based on well logs. Performance evaluation metrics including root mean square error, mean square error, and coefficient of determination ( R 2 ) were used to compare the models. The results demonstrate that AdaBoost outperformed GPR, SVM, and BPNN models in terms of processing time and accuracy, achieving R 2 values of 0.980 and 0.962 for permeability and porosity during training, respectively, and 0.960 and 0.951 during testing, respectively. This study highlights AdaBoost as a robust and accurate technique that can enhance reservoir characterization.
This is a preview of subscription content, log in via an institution to check access.
Access this article
Subscribe and save.
- Get 10 units per month
- Download Article/Chapter or eBook
- 1 Unit = 1 Article or 1 Chapter
- Cancel anytime
Price includes VAT (Russian Federation)
Instant access to the full article PDF.
Rent this article via DeepDyve
Institutional subscriptions
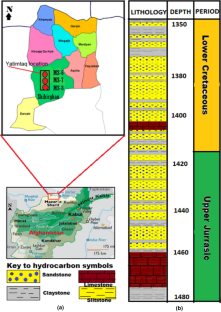
Explore related subjects
- Artificial Intelligence
Data and Materials Availability
All the data and materials used here are published with this paper and will be available upon request.
Adegbite, J. O., Belhaj, H., & Bera, A. (2021). Investigations on the relationship among the porosity, permeability and pore throat size of transition zone samples in carbonate reservoirs using multiple regression analysis, artificial neural network and adaptive neuro-fuzzy interface system. Petroleum Research . https://doi.org/10.1016/j.ptlrs.2021.05.005
Article Google Scholar
Adeniran, A. A., Adebayo, A. R., Salami, H. O., Yahaya, M. O., & Abdulraheem, A. (2019). A competitive ensemble model for permeability prediction in heterogeneous oil and gas reservoirs. Applied Computing and Geosciences, 1 , 100004.
Ahmadi, M. A., & Chen, Z. (2019). Comparison of machine learning methods for estimating permeability and porosity of oil reservoirs via petro-physical logs. Petroleum, 5 , 271–284.
Ahmadi, M., Naderpour, H., & Kheyroddin, A. (2017). ANN model for predicting the compressive strength of circular steel-confined concrete. International Journal of Civil Engineering, 15 , 213–221.
Ahmadi, M. A., Zendehboudi, S., Lohi, A., Elkamel, A., Chatzis, I., Ali Ahmadi, M., Zendehboudi, S., Lohi, A., Elkamel, A., & Chatzis, I. (2013). Reservoir permeability prediction by neural networks combined with hybrid genetic algorithm and particle swarm optimization. Geophysical Prospecting, 61 , 582–598.
Al-Anazi, A., & Gates, I. D. (2010). A support vector machine algorithm to classify lithofacies and model permeability in heterogeneous reservoirs. Engineering Geology, 114 , 267–277.
Al-Anazi, A. F., & Gates, I. D. (2012a). Support vector regression to predict porosity and permeability: Effect of sample size. Computers & Geosciences, 39 , 64–76.
Ali Ahmadi, M., Zendehboudi, S., Lohi, A., Elkamel, A., & Chatzis, I. (2013). Reservoir permeability prediction by neural networks combined with hybrid genetic algorithm and particle swarm optimization. Geophysical Prospecting, 61 , 582–598.
Aljuboori, F. A., Lee, J. H., Elraies, K. A., & Stephen, K. D. (2021). Using statistical approaches in permeability prediction in highly heterogeneous carbonate reservoirs. Carbonates and Evaporites, 36 , 1–14.
Al-Mohair, H. K., Saleh, J. M., & Suandi, S. A. (2015). Hybrid human skin detection using neural network and K-means clustering technique. Applied Soft Computing, 33 , 337–347.
Al-Mudhafar, W.J., (2020). Integrating electrofacies and well logging data into regression and machine learning approaches for improved permeability estimation in a carbonate reservoir in a giant southern Iraqi oil field, In Offshore technology conference. OnePetro.
Al-Rikaby, A. S., & Al-Jawad, M. S. (2024). Identification of reservoir flow zone & permeability estimation. Egyptian Journal of Petroleum, 33 , 1–21.
Amour, F., & Nick, H. M. (2021). Porosity and permeability variability across a chalk reservoir in the Danish North Sea: Quantitative impacts of depositional and diagenetic processes. Engineering Geology, 285 , 106059.
Asante-Okyere, S., Shen, C., Ziggah, Y. Y., Rulegeya, M. M., & Zhu, X. (2020a). A novel hybrid technique of integrating gradient-boosted machine and clustering algorithms for lithology classification. Natural Resources Research, 29 , 2257–2273.
Asante-Okyere, S., Shen, C., Ziggah, Y. Y., Rulegeya, M. M., & Zhu, X. (2020b). Principal component analysis (PCA) based hybrid models for the accurate estimation of reservoir water saturation. Computers & Geosciences, 145 , 104555.
Babadagli, T., & Al-Salmi, S. (2004). A review of permeability-prediction methods for carbonate reservoirs using well-log data. SPE Reservoir Evaluation and Engineering, 7 , 75–88.
Article CAS Google Scholar
Bashir, Y., Siddiqui, N. A., Morib, D. L., Babasafari, A. A., Ali, S. H., Imran, Q. S., & Karaman, A. (2024). Cohesive approach for determining porosity and P-impedance in carbonate rocks using seismic attributes and inversion analysis. Journal of Petroleum Exploration and Production Technology, 14 (5), 1173–1187.
Bolandi, V., Kadkhodaie, A., & Farzi, R. (2017). Analyzing organic richness of source rocks from well log data by using SVM and ANN classifiers: A case study from the Kazhdumi formation, the Persian Gulf basin, offshore Iran. Journal of Petroleum Science and Engineering, 151 , 224–234.
Bom, C. R., Valentín, M. B., Fraga, B. M. O., Campos, J., Coutinho, B., Dias, L. O., Faria, E. L., de Albuquerque, M. P. M. P., de Albuquerque, M. P. M. P., & Correia, M. D. (2021). Bayesian deep networks for absolute permeability and porosity uncertainty prediction from image borehole logs from brazilian carbonate reservoirs. Journal of Petroleum Science and Engineering, 201 , 108361.
Bramer, M. (2016). Data for data mining. Principles of data mining (pp. 9–19). Springer.
Chapter Google Scholar
Cabrera, D., & Samaniego, F. (2021). Experimental permeability tensor for fractured carbonate rocks. Rock Mechanics and Rock Engineering, 54 , 1171–1191.
Chen, S., Gu, C., Lin, C., Wang, Y., & Hariri-Ardebili, M. A. (2020). Prediction, monitoring, and interpretation of dam leakage flow via adaptative kernel extreme learning machine. Measurement, 166 , 108161.
Chen, W., & Li, Y. (2020). GIS-based evaluation of landslide susceptibility using hybrid computational intelligence models. Catena, 195 , 104777.
D’Haen, J., Van den Poel, D., & Thorleuchter, D. (2013). Predicting customer profitability during acquisition: Finding the optimal combination of data source and data mining technique. Expert Systems with Applications, 40 , 2007–2012.
Davari, M. A., Senemari, S., Alimoradi, A., & Safavi, S. J. (2024). Permeability prediction from log data using machine learning methods. Journal of Petroleum Geomechanics . https://doi.org/10.22107/JPG.2024.426878.1220
Dev, V. A., & Eden, M. R. (2019). Formation lithology classification using scalable gradient boosted decision trees. Computers & Chemical Engineering, 128 , 392–404.
Edwards, D. S., Struckmeyer, H. I. M., Bradshaw, M. T., & Skinner, J. E. (1999). Geochemical characteristics of Australia’s southern margin petroleum systems. APPEA J., 39 , 297–321.
Farouk, S., Sen, S., Ganguli, S. S., Abuseda, H., & Debnath, A. (2021). Petrophysical assessment and permeability modeling utilizing core data and machine learning approaches-A study from the Badr El Din-1 field, Egypt. Marine and Petroleum Geology, 133 , 105265.
Feng, D. C., Liu, Z. T., Wang, X. D., Chen, Y., Chang, J. Q., Wei, D. F., & Jiang, Z. M. (2020). Machine learning-based compressive strength prediction for concrete: An adaptive boosting approach. Construction and Building Materials, 230 , 117000.
Freund, Y., & Schapire, R. E. (1997). A decision-theoretic generalization of on-line learning and an application to boosting. Journal of Computer and System Sciences, 55 , 119–139.
Gan, L., Wang, Y., Luo, X., Zhang, M., Li, X., Dai, X., & Yang, H. (2019). A permeability prediction method based on pore structure and lithofacies. Petroleum Exploration and Development, 46 , 935–942.
Gholizadeh, P., & Esmaeili, B. (2016). Applying classification trees to analyze electrical contractors’ accidents. Construction Research Congress, 2016 , 2699–2708.
Google Scholar
Gu, Y., Bao, Z., & Cui, G. (2018). Permeability prediction using hybrid techniques of continuous restricted Boltzmann machine, particle swarm optimization and support vector regression. Journal of Natural Gas Science and Engineering, 59 , 97–115.
Han, H., Shi, B., & Zhang, L. (2021). Prediction of landslide sharp increase displacement by SVM with considering hysteresis of groundwater change. Engineering Geology, 280 , 105876.
Hidayat, F., & Astsauri, T. M. S. (2021). Applied random forest for parameter sensitivity of low salinity water injection (LSWI) implementation on carbonate reservoir. Alexandria Engineering Journal . https://doi.org/10.1016/j.aej.2021.06.096
Humadi, J. (2024). Predicting reservoir or non-reservoir formations by calculating permeability and porosity in an Iraqi oil field. Journal of Chemical and Petroleum Engineering., 58 , 115–129.
Izenman, A. J. (2008). Modern multivariate statistical techniques. Regression Classification, and Manifold Learning, 10 , 970–978.
Jia, W., Zhao, D., Shen, T., Ding, S., Zhao, Y., & Hu, C. (2015). An optimized classification algorithm by BP neural network based on PLS and HCA. Applied Intelligence, 43 , 176–191.
Kadhim, F.S., Imran, A.L.A.M., Rasool, M.Y.F., 2020. Using NMR, core analysis, and well logging data to predict permeability of carbonate reservoirs: a case study, In: IOP conference series: Materials science and engineering. IOP Publishing, p. 12071.
Kaloop, M. R., Kumar, D., Samui, P., Hu, J. W., & Kim, D. (2020). Compressive strength prediction of high-performance concrete using gradient tree boosting machine. Construction and Building Materials, 264 , 120198.
Kohavi, R., 1995. A study of cross-validation and bootstrap for accuracy estimation and model selection, In: Ijcai. Montreal, Canada, pp. 1137–1145.
Leisi, A., Aftab, S., & Manaman, N. S. (2024). Poro-acoustic impedance (PAI) as a new and robust seismic inversion attribute for porosity prediction and reservoir characterization. Journal of Applied Geophysics, 223 , 105351.
Li, J., Tang, T., Yu, S., & Yu, P. (2024). A machine learning based-method to generate random circle-packed porous media with the desired porosity and permeability. Advances in Water Resources, 185 , 104631.
Liao, K. W., Fan, J. C., & Huang, C. L. (2011). An artificial neural network for groutability prediction of permeation grouting with microfine cement grouts. Computers and Geotechnics, 38 , 978–986.
Liu, J. J., & Liu, J. C. (2022). Permeability predictions for tight sandstone reservoir using explainable machine learning and particle swarm optimization. Geofluids, 2022 , 2263329.
Liu, X., Han, G., Wang, E., Wang, S., & Nawnit, K. (2018). Multiscale hierarchical analysis of rock mass and prediction of its mechanical and hydraulic properties. Journal of Rock Mechanics and Geotechnical Engineering, 10 , 694–702.
Mahdy, A., Zakaria, W., Helmi, A., Helaly, A. S., & Mahmoud, A. M. E. (2024). Machine learning approach for core permeability prediction from well logs in Sandstone Reservoir, Mediterranean Sea, Egypt. Journal of Applied Geophysics, 220 , 105249.
Mangione, A., Lewis, H., Geiger, S., Jiang, Z., Couples, G. D., Buckman, J., Beavington-Penney, S., & Hall, S. A. (2021). Estimation of pre-dolomitisation porosity and permeability of a nummulitic carbonate reservoir rock using the multi-component architecture method (MCAM). Marine and Petroleum Geology, 132 , 105196.
Matveev, M. Y., Endruweit, A., Long, A. C., Iglesias, M. A., & Tretyakov, M. V. (2021). Bayesian inversion algorithm for estimating local variations in permeability and porosity of reinforcements using experimental data. Composites Part A, Applied Science and Manufacturing, 143 , 106323.
Mohammadian, E., Kheirollahi, M., Liu, B., Ostadhassan, M., & Sabet, M. (2022). A case study of petrophysical rock typing and permeability prediction using machine learning in a heterogenous carbonate reservoir in Iran. Science and Reports, 12 , 1–15.
Moussa, T., Elkatatny, S., Mahmoud, M., & Abdulraheem, A. (2018). Development of new permeability formulation from well log data using artificial intelligence approaches. Journal of Energy Resources Technology, 140 , 072903.
Mulashani, A. K., Shen, C., Nkurlu, B. M., Mkono, C. N., & Kawamala, M. (2021). Enhanced group method of data handling (GMDH) for permeability prediction based on the modified levenberg marquardt technique from well log data. Energy, 239 , 121915.
Nady, M. M. E., Lotfy, N. M., Ramadan, F. S., & Hammad, M. M. (2015). Evaluation of organic matters, hydrocarbon potential and thermal maturity of source rocks based on geochemical and statistical methods: Case study of source rocks in Ras Gharib oilfield, central Gulf of Suez, Egypt. Egyptian Journal of Petroleum, 24 , 203–211.
Nyakilla, E. E., Jun, G., Kasimu, N. A., Robert, E. F., Innocent, N., Mohamedy, T., & Shaame, M. (2022). Application of machine learning in the prediction of compressive, and shear bond strengths from the experimental data in oil well cement at 80 °C. Ensemble trees boosting approach. Construction and Building Materials, 317 , 125778.
Nyakilla, E. E., Silingi, S. N., Shen, C., Jun, G., Mulashani, A. K., & Chibura, P. E. (2021). Evaluation of source rock potentiality and prediction of total organic carbon using well log data and inte- grated methods of multivariate analysis, machine learning, and geochemical analysis. Natural Resources Research . https://doi.org/10.1007/s11053-021-09988-1
Otchere, D. A. (2024). Fundamental error in tree-based machine learning model selection for reservoir characterisation. Energy Geosci., 5 , 100229.
Otchere, D. A., Ganat, T. O. A., Gholami, R., & Lawal, M. (2021). A novel custom ensemble learning model for an improved reservoir permeability and water saturation prediction. J. Nat. Gas Sci. Eng., 91 , 103962.
Pan, S., Horsfield, B., Zou, C., Yang, Z., & Gao, D. (2017). Statistical analysis as a tool for assisting geochemical interpretation of the upper Triassic Yanchang formation, Ordos Basin, Central China. International Journal of Coal Geology, 173 , 51–64.
Pearson, K. (1901). LIII. On lines and planes of closest fit to systems of points in space. London, Edinburgh. Dublin Philos. Mag. J. Sci., 2 , 559–572.
Pham, B. T., Jaafari, A., Phong, T. V., Mafi-Gholami, D., Amiri, M., Van Tao, N., Duong, V. H., & Prakash, I. (2021a). Naïve Bayes ensemble models for groundwater potential mapping. Ecol. Inform., 64 , 101389.
Pham, B. T., Nguyen, M. D., Nguyen-Thoi, T., Ho, L. S., Koopialipoor, M., Kim Quoc, N., Armaghani, D. J., & Le, H. V. (2021b). A novel approach for classification of soils based on laboratory tests using Adaboost, tree and ANN modeling. Transportation Geotechnics, 27 , 100508.
Pitombo, C. S., de Souza, A. D., & Lindner, A. (2017). Comparing decision tree algorithms to estimate intercity trip distribution. Transportation Research Part C: Emerging Technologies, 77 , 16–32.
Qian, C., Yang, S., Wang, Y., Wu, C., & Zhang, Y. (2021). Prediction and modeling of petrophysical parameters of deep-buried, low permeability glutenite reservoirs in Yubei area, Turpan-Hami Basin, China. Journal of Petroleum Science and Engineering, 207 , 109154.
Qian, J., Yan, Y., Wang, Y., Liu, Y., & Luo, Q. (2024). Effect of scale and matrix porosity on the relationship between permeability and resistivity in fracture-matrix system. Journal of Hydrology, 629 , 130600.
Rafik, B., & Kamel, B. (2017). Prediction of permeability and porosity from well log data using the nonparametric regression with multivariate analysis and neural network, Hassi R’Mel Field. Algeria. Egypt. J. Pet., 26 , 763–778.
Rao, H., Shi, X., Rodrigue, A. K., Feng, J., Xia, Y., Elhoseny, M., Yuan, X., & Gu, L. (2019). Feature selection based on artificial bee colony and gradient boosting decision tree. Applied Soft Computing, 74 , 634–642.
Röding, M., Ma, Z., & Torquato, S. (2020). Predicting permeability via statistical learning on higher—order microstructural information. Scientific Reports . https://doi.org/10.1038/s41598-020-72085-5
Sun, Y., Pang, S., Zhang, J., & Zhang, Y. (2024). Porosity prediction through well logging data: A combined approach of convolutional neural network and transformer model (CNN-transformer). Physics of Fluids, 36 , 026604.
Tan, X. H., Jiang, L., Li, X. P., Li, Y. Y., & Zhang, K. (2017). A complex model for the permeability and porosity of porous media. Chemical Engineering Science, 172 , 230–238.
Tian, J., Qi, C., Sun, Y., Yaseen, Z. M., & Pham, B. T. (2021). Permeability prediction of porous media using a combination of computational fluid dynamics and hybrid machine learning methods. Engineering Computations, 37 , 3455–3471.
Tong, Z., Meng, Y., Zhang, J., Wu, Y., Li, Z., Wang, D., Li, X., & Ou, G. (2024). Coal structure identification based on geophysical logging data: Insights from wavelet transform (WT) and particle Swarm optimization support vector machine (PSO-SVM) algorithms. International Journal of Coal Geology, 282 , 104435.
Urang, J. G., Ebong, E. D., Akpan, A. E., & Akaerue, E. I. (2020). A new approach for porosity and permeability prediction from well logs using artificial neural network and curve fitting techniques: A case study of Niger Delta, Nigeria. Journal of Applied Geophysics, 183 , 104207.
Vapnik, V. N. (1995). The nature of statistical learning theory . Springer.
Book Google Scholar
Wang, B., Wang, Z., Shen, B., Tang, D., Wu, Y., Wu, B., Li, S., & Zhang, J. (2024a). Evaluation of saturation interpretation methods for ultra-low permeability argillaceous sandstone gas reservoirs: A case study of the Huangliu formation in the Dongfang area. Processes, 12 , 271.
Wang, L., & Zhang, Y. (2024). Interpreting correlations in stress-dependent permeability, porosity, and compressibility of rocks: A viewpoint from finite strain theory. International Journal for Numerical and Analytical Methods in Geomechanics, 48 , 2000–2019.
Wang, Z., Fang, H., Sang, S., Guo, J., Yu, S., Liu, H., & Xu, H. (2024b). Comprehensive analysis of connectivity and permeability of a pore-fracture structure in low permeability seam of Huainan–Huaibei coalfield. ACS Omega, 9 , 15357–15371.
Wu, G., Lü, Z. T., & Wu, Z. S. (2006). Strength and ductility of concrete cylinders confined with FRP composites. Construction and Building Materials, 20 , 134–148.
Wu, J., Xu, H., Xiong, B., Fang, C., Wang, S., Zong, P., Liu, D., & Xin, F. (2024). A new method for investigating the impact of temperature on in-situ reservoir properties using high-temperature AFM. Geothermics, 120 , 103006.
Wu, X., Kumar, V., Quinlan, J. R., Ghosh, J., Yang, Q., Motoda, H., McLachlan, G. J., Ng, A., Liu, B., & Philip, S. Y. (2008). Top 10 algorithms in data mining. Knowledge and Information Systems, 14 , 1–37.
Yalamanchi, P., & Datta Gupta, S. (2024). Estimation of pore structure and permeability in tight carbonate reservoir based on machine learning (ML) algorithm using SEM images of Jaisalmer sub-basin, India. Scientific Repoorts, 14 , 930.
Yasuda, T., Ookawara, S., Yoshikawa, S., & Matsumoto, H. (2021). Machine learning and data-driven characterization framework for porous materials: Permeability prediction and channeling defect detection. Chemical Engineering Journal, 420 , 130069.
Zaremotlagh, S., Hezarkhani, A., & Sadeghi, M. (2016). Detecting homogenous clusters using whole-rock chemical compositions and REE patterns: A graph-based geochemical approach. Journal of Geochemical Exploration, 170 , 94–106.
Zhang, J., Wang, R., Jia, A., & Feng, N. (2024). Optimization and Application of XGBoost Logging Prediction Model for Porosity and Permeability Based on K-means Method. Applied Sciences, 14 , 3956.
Zhong, Z., Carr, T. R., Wu, X., & Wang, G. (2019). Application of a convolutional neural network in permeability prediction: A case study in the Jacksonburg-Stringtown oil field, West Virginia, USA. Geophysics, 84 , B363–B373.
Zhou, D., Tang, Y., Zhou, W., Wu, Z., Wu, Y., Yan, G., Huang, Z., Wang, H., Li, Z., Li, Y., (2024). Study on 4D geomechanical modelling for fault critical re-active stress evaluation in underground gas storage, In: International petroleum technology conference. IPTC, p. D021S049R007.
Zhuang, X. Y., Chen, L., Komarneni, S., Zhou, C. H., Tong, D. S., Yang, H. M., Yu, W. H., & Wang, H. (2016). Fly ash-based geopolymer: clean production, properties and applications. Journal of Cleaner Production, 125 , 253–267.
Download references
Acknowledgments
This work was supported by the Natural Science Foundation of Hubei (Three Gorges Innovation Development Joint Fund grant No. 2022CFD031), this work was supported by Peking University Ordos Energy Research Institute, Huineng Kechuang Building, Minzu Road, Kangbashi District, Ordos City, Inner Mongolia, and the National Science Foundation for Young Scientists of China (12302507). Finally, we would like to express our sincere thanks and gratitude to all reviewers and editors for their time end efforts toward raising this work to the publication standards.
Author information
Authors and affiliations.
State Key Laboratory of Geomechanics and Geotechnical Engineering, Institute of Rock and Soil Mechanics, Chinese Academy of Sciences, Wuhan, 430071, China
Edwin E. Nyakilla & Sun Guanhua
Peking University Ordos Energy Research Institute, Huineng Kechuang Building, Minzu Road, Kangbashi District, Ordos City, 017010, Inner Mongolia Autonomous Region, China
Edwin E. Nyakilla, Sun Guanhua, Hao Hongliang & Li Dan
Department of Petroleum Geology School of Earth Resources, China University of Geosciences, Wuhan, 430074, China
Grant Charles, Mouigni B. Nafouanti, Emanuel X. Ricky, Selemani N. Silingi, Elieneza N. Abelly, Eric R. Shanghvi, Safi Naqibulla, Mbega R. Ngata, Erasto Kasala, Alaa Abdulmalik & Mbula N. Nadege
Department of Geology, Earth Sciences Institute of Shinyanga, (ESIS), P.O. Box 1016, Shinyanga, Tanzania
Selemani N. Silingi
Mbeya University of Science and Technology (MUST), P.O. Box 131, Mbeya, Tanzania
Melckzedeck Mgimba
Colledge of Petroleum Engineering, China University of Petroleum (Beijing), Beijing, 102249, China
Johnson J. Kasali
School of Petroleum Engineering, China University of Petroleum (East China), Qingdao, 266580, China
Fatna A. Said
You can also search for this author in PubMed Google Scholar
Corresponding authors
Correspondence to Edwin E. Nyakilla , Sun Guanhua or Hao Hongliang .
Ethics declarations
Conflict of interest.
The authors declare there is no conflict of interest.
Rights and permissions
Springer Nature or its licensor (e.g. a society or other partner) holds exclusive rights to this article under a publishing agreement with the author(s) or other rightsholder(s); author self-archiving of the accepted manuscript version of this article is solely governed by the terms of such publishing agreement and applicable law.
Reprints and permissions
About this article
Nyakilla, E.E., Guanhua, S., Hongliang, H. et al. Evaluation of Reservoir Porosity and Permeability from Well Log Data Based on an Ensemble Approach: A Comprehensive Study Incorporating Experimental, Simulation, and Fieldwork Data. Nat Resour Res (2024). https://doi.org/10.1007/s11053-024-10402-9
Download citation
Received : 25 March 2024
Accepted : 27 August 2024
Published : 18 September 2024
DOI : https://doi.org/10.1007/s11053-024-10402-9
Share this article
Anyone you share the following link with will be able to read this content:
Sorry, a shareable link is not currently available for this article.
Provided by the Springer Nature SharedIt content-sharing initiative
- Machine learning
- multivariate analysis
- permeability
- Find a journal
- Publish with us
- Track your research

COMMENTS
Abstract. This paper is concerned with experimental analysis of the behaviour of mechanisms of sewing. machines. There has been carried out mapping of the so und field in the vicinit y of the ...
Sewing machines play a vital role in family economic status. Apart from the garment industry sewing machine was majorly used in the domestic sector. ... In this many research papers were reviewed and from which we got to know variously ergonomically study techniques which had been done at the industrial level to reduce occupational health ...
Sewing equipment and work aids. P. Jana, in Garment Manufacturing Technology, 2015 11.1 Introduction. Sewing is the process of stitch formation involving needle and thread. Although hand sewing was prevalent during the Paleolithic era, most sewing processes could be performed by machines by about 1900 (Glock and Kunz, 2000).This chapter will discuss different features and functions of sewing ...
The first functional sewing machine was invented by the French tailor, Barthélemy Thimonnier in 1830. The invention sparked fear among other tailors for their livelihood, and Barthélemy Thimonnier's workshop was burnt down. After 16 years, Elias Howe, the American inventor, patented an improved sewing machine in 1846.
A. Regional attainment of 0.2% Per Capita Sewing Machine Diffusion and Per Capita Income 1850-1920. B. Regional attainment of 1.0% Per Capita Sewing Machine Diffusion and Per Capita Income 1866-1925.
This study attempts to explore the female life history embedded in the sewing machines. This study examines the experience of women in Taiwan of different periods to use sewing machines through the historical studies and qualitative research, which can be used as historical supplement of women users and relevant products that have long been neglected in design, but also reflect numerous ...
This study aimed to establish an automatic sewing process for garment production according to textile properties. An automatic feeding system and a self-made template were introduced to an industrial sewing machine. Two types of stitches were performed on fabrics with various physical properties and surface roughness using this automatic sewing machine. The appearance, stitch length and width ...
Based on the sewing thread 40s count, many factors affect the level of seam strength. These are knitted fabrics structure and properties, stress location of a garment, sewing thread type and construction, sewing machine tension, sewing needle type, stitch and seam types and stitch density influenced by the seam strength and seam slippage.
The aim of this paper is to investigate and interpret of the definition of sewing, the process starting from the invention of sewing machines, their historical development and impacts towards women. Down from the times of the invention of the first sewing machine, machines had developed rapidly and had started to serve a reply to functionality ...
Figure 4 shows the evolution of the needle Jerk, for the extreme optimal solutions S1, S2 and S3 as a function of the input link angle. We present also, in the same figure, the evolution of the needle jerk, obtained by the sewing machine Juki 8700. One can note that by using the S1 design vector, we decrease the needle jerk down by 35% compared to the one for the Juki 8700.
Findings. Sewing damages such as needle cut and other sewing damages/defects are studied mostly in woven fabric. There are very few studies conducted on knitted fabric sewing damages/defects. The sewing damage problems do not have single solution that is capable of removing these damages in fabric. All the determined and affecting parameters ...
ON Mar. 8, M. Herriott, the former premier of France, unveiled a monument at Lyons to Barth©lemy Thimonnier, the inventor of the sewing machine. Thimonnier applied for his first patent in collaboration with Auguste Ferrand, of the School of Mines, St. Étienne, on April 12, 1830, and the erection of the monument was the outcome of the celebration last year of the centenary of that event. The ...
The Sewing Machine War exhibited all of these phenomena, proving that these are hoary issues in patent law. The denouement of this patent thicket in the Sewing Machine Combination of 1856, the first privately formed patent pool, further challenges the conventional wisdom that patent thickets are best solved through public-ordering regimes that ...
Discover the world's research. 25+ million members; ... inserted into the needle bar o f an industrial sewing machine ... paper, it is followed ISO 13935-1 (2014) ...
Processing textile materials is generally very difficult due to the flexible nature of the material. In industries using sewing as assembly process, most processes rely on human labor, being difficult or even impossible to automate. The relations between machine configuration and adjustment, material properties, and the resulting product quality are also complex. This paper describes current ...
The relations between machine configuration and adjustment, material properties, and the resulting product quality are also complex. This paper describes current work using an instrumented lockstitch sewing machine to study the dynamics and variations of one of the important process parameters during high-speed sewing of shirts: thread tensions.
The benefits of TPM implementation in the sewing process are also well recognized. Unproductive times and non-value added activities were identified and their countermeasures were made to increase the efficiency of the sewing machines of the footwear industry (Reyes et al., 2018). The improvement in the equipment utilization in the ...
Abstract. Purpose The purpose of this review paper is to define the dominating factors (such as fiber, yarn, fabric structure, sewing thread, sewing needle and machine parameters) that affect the ...
Before you start, make sure you have the right tools. A good setup makes all the difference when it comes to smooth buttonhole sewing. You'll need: A sewing machine with a buttonhole foot (some machines have automatic buttonholes, while others require manual stitching) A water-soluble fabric marker; Interfacing or stabilizer
2.1 Proposed Methodology. The following methodology was proposed in order to study the impact of the application of IoT devices in the apparel industry. A sewing machine (HAITRAL HT-CS141WPU) was used to implement three sensors to monitor the variables: motor temperature, needle bar vibration, and foot pedal force (Fig. 1).The motor temperature was monitored to estimate whether there was ...
Sewing is a complex and non-linear dynamic operation process, posing challenges in achieving effective collaboration between robots and sewing machines (Schrimpf et al. 2013). The parameters such as the speed of the sewing machine, the needle distance, and the pressure of the press foot will also affect the operating state of the robot.
This machine learning model decides where to split based on random selection of features. Random forest tree methods have a uniqueness where each split can be done through scrutiny of different features. This paper proposes the ensemble machine learning based random de-correlated extra decision tree model for the forest cover type prediction.
This paper examines the integration of generative artificial intelligence (AI) in doctoral writing pedagogy. It explores how AI augments traditional teaching and composition processes, fosters a new paradigm of cognitive engagement and collaborative academic writing, and the broader ethical and social implications of human-AI writing in doctoral writing pedagogy. A community-engaged ...
Permeability and porosity are key parameters in reservoir characterization for understanding hydrocarbon flow behavior. While traditional laboratory core analysis is time-consuming, machine learning has emerged as a valuable tool for more efficient and accurate estimation. This paper proposes an ensemble technique called adaptive boosting (AdaBoost) for porosity and permeability estimation ...