Thank you for visiting nature.com. You are using a browser version with limited support for CSS. To obtain the best experience, we recommend you use a more up to date browser (or turn off compatibility mode in Internet Explorer). In the meantime, to ensure continued support, we are displaying the site without styles and JavaScript.
- View all journals
- Explore content
- About the journal
- Publish with us
- Sign up for alerts
- Review Article
- Open access
- Published: 05 April 2024

A systematic review of Stimulated Recall (SR) in educational research from 2012 to 2022
- Xuesong Zhai ORCID: orcid.org/0000-0002-4179-7859 1 , 2 na1 ,
- Xiaoyan Chu 1 na1 ,
- Minjuan Wang 3 , 4 ,
- Chin-Chung Tsai 5 ,
- Jyh-Chong Liang 5 &
- Jonathan Michael Spector 6
Humanities and Social Sciences Communications volume 11 , Article number: 489 ( 2024 ) Cite this article
1666 Accesses
2 Altmetric
Metrics details
- Science, technology and society
Stimulated Recall (SR) has long been used in educational settings as an approach of retrospection. However, with the fast growing of digital learning and advanced technologies in educational settings over the past decade, the extent to which stimulated recall has been effectively implemented by researchers remains minimal. This systematic review reveals that SR has been primarily employed to probe the patterns of participants’ thinking, to examine the effects of instructional strategies, and to promote metacognitive level. Notably, SR video stimuli have advanced, and the sources of stimuli have become more diverse, including the incorporation of physiological data. Additionally, researchers have applied various strategies, such as flexible intervals and questioning techniques, in SR interviews. Furthermore, this article discusses the relationships between different SR research items, including stimuli and learning contexts. The review and analysis also demonstrate that stimulated recall may be further enhanced by integrating multiple data sources, applying intelligent algorithms, and incorporating conversational agents enabled by generative artificial intelligence such as ChatGPT. This article provides a comprehensive analysis of SR studies in the realm of education and proposes a promising avenue for researchers to proactively apply stimulated recall in investigating educational issues in the digital era.
Similar content being viewed by others
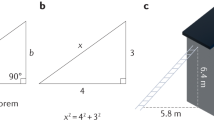
The science of effective learning with spacing and retrieval practice
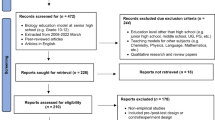
A meta-analysis to gauge the impact of pedagogies employed in mixed-ability high school biology classrooms
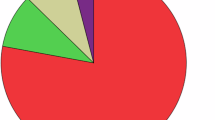
Exploring the impact of artificial intelligence on higher education: The dynamics of ethical, social, and educational implications
Introduction.
Stimulated Recall (SR) is an approach commonly used to prompt participants’ retrospection by employing diverse stimuli and interview strategies. This method is frequently applied to examine instructors’ and students’ reflections on their cognitive and affective responses during or after specific educational events or activities (Calderhead, 1981 ; Gass and Mackey, 2016 ). This type of SR represents an effective qualitative method for educational researchers to gather implicit data and has been broadly practiced to investigate various teaching and learning occurrences, including teacher cognition, study strategies, and language learning (Meade and McMeniman, 1992 ; Van der Kleij et al., 2017 ; White et al., 2016 ; Sundberg et al., 2018 ; Martinelle, 2018 ; Cao et al., 2019 ; Martinelle, 2020 ). Moreover, in addition to serving as a research tool to explore instructors’ and learners’ internal thoughts, several studies have innovatively implemented SR as a teaching and learning strategy to foster students’ metacognition (Zhai et al., 2018 ; Jensen, 2019 ). Nevertheless, although the purposes of SR-enabled research appear to be diverse, there are reasons to extend its use in more educational and research settings.
The vast technology integration into education has ushered in changes in the selection of stimuli and technologies for adopting SR in educational research (Gazdag et al., 2019 ). Technological advancement applied in teaching and learning settings have also expanded the sources of stimuli beyond traditional written notes, classroom photographs, and video recordings. Participants’ learning records on digital platforms and mobile devices can also be used as stimuli to evoke the memory of their own learning path (Koltovskaia, 2020 ; Lindfors et al., 2020 ). Furthermore, the shift of instructional environments from offline to online has rendered educational activities in physical scenes more static, lacking observable interactivity to generate an effective stimulus (Duo and Song, 2012 ; Gijselaers et al., 2016 ; Tan et al., 2021 ). Some studies have leveraged physiological feedback signals such as eye movement, setting position, and EEG data to provide valuable cues about changes in learners’ inner thoughts (Zhai et al., 2018 ; El and Windeatt, 2019 ). However, owing to the constantly evolving technological landscape in learning environments and pedagogical strategies, the question of whether traditional stimuli need to be improved and how to choose new stimuli remains unresolved (Wijayasundara, 2020 ).
When practicing interview strategies, researchers have exhibited distinctive tendencies in time arrangement and questioning techniques (Gass and Mackey, 2016 ). Even when the same stimuli were selected, the adoption of interview strategies varied across studies. Concerning the time arrangement of the interview, most researchers contend that participants should be presented with the stimuli and interviewed immediately after the instructional activity, while some researchers intentionally introduce an interval before further interviewing (Gass and Mackey, 2000 ; Kurki et al., 2016 ). In terms of questioning techniques, interviewers’ questions can be either entirely open-ended or focused, depending on the research design and educational settings. For instance, Heikonen et al., ( 2017 ) commenced with general questions and subsequently narrowed the question scope to explore student and instructors’ reflections on classroom incidents. In contrast, Hu and Gao ( 2020 ) posed rather specific questions on students’ responses to linguistic challenges in learning science through English. These disparities may be attributed to the distinct subjects and research questions that SR measures aim to address (Jackson and Cho, 2018 ; Tiainen et al., 2018 ).
In light of the ongoing developments in education and technology, it is worthwhile to conduct a meticulous review of the latest research on applying SR methods in education. Previous reviews were either outdated or narrow in scope. For instance, Keith’s ( 1988 ) review centered on studies that applied SR to investigate instructors’ cognitive processes, which, although valuable at the time, can only provide limited guide for current applications of SR in education. More recently, Gazdag et al., ( 2019 ) reviewed 35 articles on the use of Video Stimulated Recall (VSR) to enhance instructors’ reflective thinking. However, this study’s scope was confined to implementing VSR in teacher training and excluded studies in broader educational settings. Therefore, further studies are needed to comprehensively examine the application of SR across diverse contexts.
The present study offers a comprehensive review of research using SR in manifold teaching and learning contexts over the past decade. The investigation scrutinizes the characteristics of these studies, such as their research aims, stimuli, and interview strategies. It examines the interplay among these elements, including variations in the purposes of SR employment across disciplines. The ultimate goal of our study is to provide valuable insights for future applications of SR in education and also to aid researchers in exploring the external behaviors and internal thought processes of both instructors and students in a more effective manner.
Literature review
The theoretical foundation of sr in education.
SR is a research technique inspired by Dewey’s ( 1933 ) reflective thinking concept, which involves presenting participants with vivid prompts to evoke their memories of an original scenario (Bloom, 1953 ). Since its inception by researchers at Stanford University in 1970, SR has been an essential tool in pedagogical research and widely adopted to investigate various teaching and learning activities in educational research (Stough, 2001 ). Typically, SR comprises two stages: presenting stimuli and proposing recall questions (see Fig. 1 ) (Chu and Zhai, 2023 ). Researchers select specific artifacts, such as notes, audio or video recordings, that exhibit participants’ behavior or cognitive tasks as stimuli, followed by interviews that prompt participants to articulate their intrinsic thoughts, mental processes, or individual feelings at the moment when the stimuli were generated (Calderhead, 1981 ; Lyle, 2003 ).
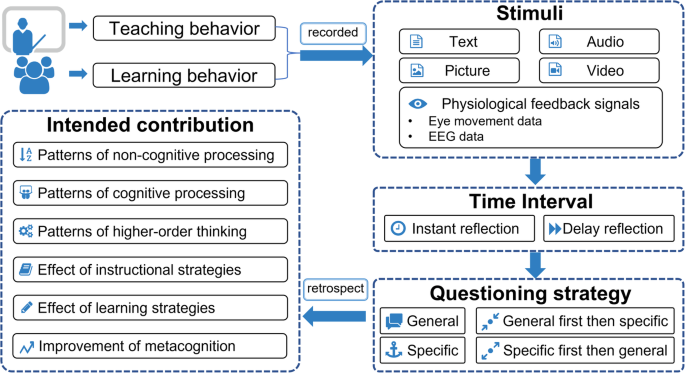
The figure shows the main stages of presenting stimuli and proposing recall questions when applying SR.
The theoretical basis of SR in educational research draws on the Retrocue Effect and the Cognitive Theory of Multimedia Learning (CTML) (Mayer and Moreno, 1998 ; Moreno and Mayer, 1999 ; Souza and Oberauer, 2016 ; Shepherdson et al., 2018 ). The Retrocue Effect, a cognitive psychology theory, suggests that an individual’s visual working memory is enhanced when their attention is directed toward prior information, even after a delay or distraction (Souza and Oberauer, 2016 ). Neuroscientific and biopsychological research both provide evidence supporting the protective effect of retroactive attentional focusing on working memory (Duarte et al., 2013 ; Schneider et al., 2017 ). According to this theory, retro cues, such as visual stimuli, improve the quality of retrieval and cognitive processes while also reducing cognitive load effects (Shepherdson et al., 2018 ). Based on this mechanism, SR can offer accurate and specific insights into an instructor or a learner’s thoughts and attitudes towards educational tasks.
In addition, the Cognitive Theory of Multimedia Learning (CTML) suggests that multimedia learning is most effective when information is presented in both visual and auditory formats, as learners are actively engaged in the learning process (Mayer and Moreno, 1998 ; Moreno and Mayer, 1999 ). As described in the CTML, learners have two separate channels for processing information: visual and verbal (Mayer, 2002 ; Mayer and Moreno, 2003 ). When multiple forms of stimuli are presented during the SR interview, instructors and learners become more cognizant of their prior experiences in each channel, which helps them articulate their thought processes in greater detail and enhances their retrospection of previous knowledge and cognition. In conclusion, the application of SR in educational research is rooted in the principles of the ICT and the CTML. Implementing SR provides researchers and practitioners with a valuable tool to gain insight into learners’ and instructors’ cognitive processes, ultimately leading to more effective teaching and learning.
The educational application using SR
The SR method is an effective technique used in qualitative educational research to gather data on instructors’ and learners’ thought patterns related to specific events. This method allows researchers to explore instructors’ and learners’ thinking and decision-making processes, making it a valuable tool for data collection (Nguyen et al., 2013 ; Bowles, 2018 ). The use of SR in educational research is critical for maintaining internal validity, as it provides introspective data. Additionally, SR has broad applicability and can be employed in various disciplines for a range of research aims (Meade and McMeniman, 1992 ; Kurki et al., 2016 ; Yu and Hu, 2017 ; Rietdijk et al., 2018 ; Martinelle, 2020 ). For instance, Yu and Hu ( 2017 ) used SR to probe second language learners’ intrinsic and personalized perceptions of peer feedback in collaborative writing assessment, by exploring students’ learning behaviors through interviews. Similarly, Kurki et al. ( 2016 ) and Rietdijk et al. ( 2018 ) tapped into SR to explore how instructors use various teaching strategies and their underlying beliefs, particularly concerning non-cognitive dimensions such as social and emotional factors.
Aside from its application as an educational research method, SR can also serve as an effective teaching and learning strategy. Instructors can use SR to assess what learners remember or may have overlooked to determine learning reinforcement strategies. SR enables learners to examine and articulate their thoughts through memory retrieval and thus elevating their thinking to a new level of expression. Therefore, SR can enhance learning rather than solely serving as a research approach (Smagorinsky, 1998 ; Egi, 2008 ). In addition, VSR is a valuable teacher training and development tool that includes video-supported reflection and questioning. This approach motivates instructors to reflect on themselves and their practice consciously, facilitates metacognitive reflection among preservice teachers, and provides reflective prompts for educational interactions (Geiger, Muir, and Lamb, 2016 ; Endacott, 2016 ).
While linguistics and teacher education are the primary application areas of SR, it is also used in other subjects, such as STEM education (Gass and Mackey, 2016 ; Al Mamun, Lawrie, and Wright, 2020 ; Schindler and Lilienthal, 2019 ). However, the purpose of applying SR varies depending on the subject and learning environment. Recent advances in instructional technologies have transformed teaching strategies and educational settings, yet the relationship between these elements and the principles of SR application in distinct contexts is still ambiguous.
S timuli and interview strategies in SR
The rapid diffusion of Information and Communication Technology (ICT) and the exponential growth of online learning have brought new challenges and opportunities for using SR in educational research and in teaching and learning. Integrating ICT into education requires a careful selection of stimuli that can adapt to the constantly evolving learning environments. When applied in physical environments, audio or video stimuli respond favorably to interactions between teachers and students, enabling subsequent interviews to investigate their inner impressions or perceptions (Chu and Zhai, 2023 ). In contrast, educational activities incorporating digital technologies are not easily observable, with instructional behaviors conducted through electronic devices and in video or audio conference systems. It is often challenging to find informative stimuli reflecting teacher-student interactions in digital settings.
Nevertheless, technological breakthroughs have enriched stimuli by expanding data collection channels and capacities at the same time. Through the integration of additional stimuli sources such as weblogs, computer screen captures, and biofeedback data, researchers are able to unearth information about learners’ inner workings. For example, Révész et al., ( 2017 ) gained a comprehensive picture of the L2 writing process and acquired a deeper understanding of implicit thinking using eye movement data-based stimuli. Overall, considering the diverse data collection methods and changing learning contexts, stimuli selection in SR in technology-enabled schooling still requires further clarification.
The interview stage is another crucial aspect of SR that distinguishes it from conventional memory recall. This stage emphasizes estimating internal thinking processes and determining how the method can encourage instructors’ and learners’ reflection and delve into their internal ideas. During the interview stage, researchers mainly acquire tacit data. Previous studies are inclined to perform interviews promptly after class and employ standard open-ended questions to encourage participants’ agency in reflecting on their experiences (Gass and Mackey, 2000 ; Chu and Zhai, 2023 ). However, some studies have chosen different approaches. For example, when investigating early childhood teachers’ instructional activities, behavior, and emotions, Kurki and his colleagues (2016) delayed inviting teachers to take part in the interview by two weeks. Additionally, researchers argue that, apart from using generic questions, incorporating specific follow-up questions that closely align with the research aim is equally crucial (Heikonen et al., 2017 ; Hu and Wu, 2020 ). Despite the significance and disparities in interview strategies, few studies have specifically analyzed this issue, and well-developed principles of organizing interviews and questions is absent.
SR has become an essential technique for examining cognition and behavioral patterns in education by activating instructors’ and students’ retrospection through stimuli and interviews. As SR has evolved and educational paradigms have transformed, the research purpose and critical steps, such as stimuli selection and interview strategies, of applying SR in educational research require further discussion. Education is a complex system with intertwined intrinsic elements such as discipline differences and learning environments (Jacobson and Wilensky, 2006 ; Jacobson et al., 2019 ), which can influence stimuli preference and the conduct of interviews.
Therefore, in order to provide insights for learning from past educational applications of SR and enhancing future developments, the present systematic review scrutinized the evolution of the SR method in educational research from 2012 to 2022. It aimed to elucidate what the contributions SR has made, how SR has been implemented, and the challenges and potentials it presents. To fulfill these objectives, we further proposed six specific coding items (see Table 1 ) to guide our content analysis coding procedure and decoding interpretation.
Guided by the aforementioned research questions, we systematically analyzed and interpreted studies related to SR from 2012 to 2022. Given the significant changes in teaching environments and research methods associated with the rapid development in educational technology, we believe that a 10-year time span can provide sufficient coverage of research in a variety of disciplines. We used qualitative content analysis to examine these studies, which consists of two steps: selecting papers for review and coding these papers by using an established coding scheme.
Paper selection
To guarantee the quality of selected, our research team reviewed well-recognized peer-reviewed articles in the Web of Science (WOS) core collection, Scopus, and IEEE Xplore. These databases contain reputable journals with recognized impact factors. The articles retrieved in WOS and Scopus can be further refined into social science or educational categories, allowing for more precise retrieval. Additionally, given the focus of this research on the use of technology in education, the IEEE database provides focused research in scientific and technical disciplines.
Two stages comprise the processes used to identify the research papers. In the first stage, the keyword “SR” was selected, and the discipline was refined to “education and educational research”. This process yielded 309 articles. In the second stage, the abstracts and full text of the chosen articles were manually and systematically screened by researchers to confirmed that they: (1) included the SR protocols, (2) prompted participants to reflect on their thinking process, (3) focused on research issues in the field of education, and (4) provided empirical evidence or evaluation rather than solely summarizing previous findings. For example, some articles merely reviewed others’ research on the SR method employed in teaching settings or using painting-based stimuli to spark students’ prior knowledge did not meet the inclusion criteria (Gazdag et al., 2019 ; Walan and Enochsson, 2019 ).
According to Golhasany and Harvey’s ( 2023 ) study, the coder should pose doctoral degrees or professorships in the relevant field, and each identified papers should be individually scrutinized by experts. Finally, three experts were selected to examine the sample pool: two of whom have doctoral degrees and professorships on learning technology, while the third have a doctoral degree in educational management and post-doctoral experience on learning technology. Moreover, to ensure there are no conflicts of interest, only one coder is involved in the authorship. The inter-coder reliability was assessed following a specific schedule: first, the coders independently examine the selected samples and provided their judgment. Then we use the Fleiss Kappa test in SPSS 26 to test the reliability. The results ranged from 0.874 to 0.973 indicating satisfactory inter-rater reliability and consistent coding for each item. Finally, we adopted the final coding results if all the experts or at least two of them agreed. Finally, the research team identified 257 representative papers as the research sample of this study. As recommended by Preferred Reporting Items for Systematic Reviews and Meta-Analyses (PRISMA, Page et al. 2021 ), we conducted the systematic review with a strong and thorough methodology. Figure 2 depicts the flow of this screening process, which is in accordance with Moher et al. ( 2009 ).
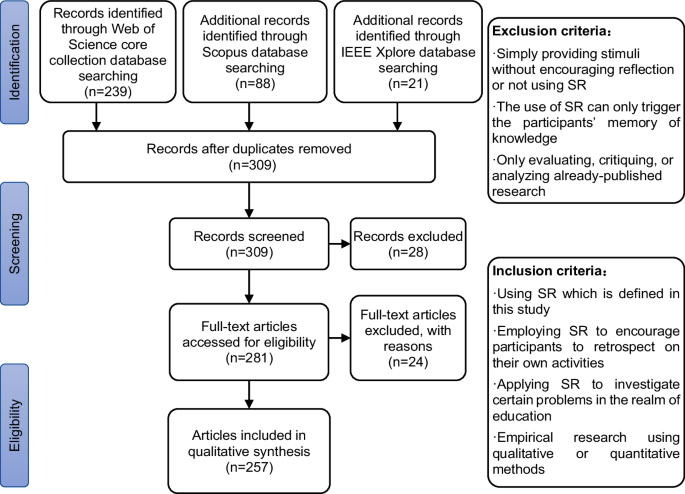
The diagram presents the systematic review flow according to PRISMA.
Coding procedure
The identified articles were systematically coded to carry out a precise and thorough examination of the utilization of SR in education. By adopting Gass and Mackey’s ( 2000 ) definition of SR, this study identifies its key components. They established a coding framework, including the research aim, stimuli, questioning technique, and questioning interval. Additionally, coding the learning subject and educational context helped clarify how to implement SR effectively in various situations. Table 2 illustrates the background information of SR research, such as the learning subject and educational context. Despite reviewing research involving instructors and students as subjects, this study did not differentiate between these two groups as the primary focus of SR is to investigate the participants’ consciousness and thinking behind their behavior, regardless of their roles. The table included in supplementary information described our data collection process.
The coding process involved identifying and extracting relevant data from the selected papers. Any discrepancies were resolved through discussion and consensus-reaching among the research team. We then analyzed the coded data and identified patterns and themes abiding by the content analysis method. The findings of this review are presented in the following sections, addressing the research questions outlined earlier.
Results and discussion
In accordance with the content analysis and coding criteria mentioned above, 257 papers were thoroughly examined. The following sections present the results and provide a corresponding discussion of the research questions.
RQ1: Research aims
The current literature reveal that SR is often applied to studying instructors’ and learners’ inner thoughts and ideas, prompting them to recall and comment on their thinking process. Furthermore, this approach can also examine the effect of teaching and learning strategies and to improve participants’ metacognitive skills. Because SR has long been used in educational settings, it is surprising that more substantial research has yet to be conducted on how it might be expanded and how to overcome its limitations such as time factors and distractions. Therefore, our work focuses on promoting the effectiveness of widespread application of SR in teaching and learning.
Exploring thought patterns
Exploring thought patterns is the primary focus of most educational research that uses SR. This includes investigating non-cognitive and cognitive processes, as well as higher-order thinking. As shown in Table 3 , over half (157 in total) of the research reviewed employed SR to achieve this objective. Eighty-two of the reviewed studies explored patterns of non-cognitive processing, such as motivation, emotions, and cultural orientation (Lichtinger and Kaplan, 2015 ; Ucan and Webb, 2015 ; Wilby et al., 2017 ). This method can also investigate the variables influencing the willingness to communicate or the ethical considerations of instructional practices in language learning (Rissanen et al., 2018 ; Chichon, 2019; Peng, 2020 ).
In addition, fifty-five studies explored patterns of cognitive processing, probing the epistemic thinking of diverse participants in various subjects, including language learning, STEM, and the arts (Bogard et al., 2013 ; dos Santos and Loveridge, 2014 ; Révész et al., 2017 ). Furthermore, some studies based on SR also obtained insight into both cognitive as well as non-cognitive processes through the integration of multimodal data (Lambert and Zhang, 2019 ). It is worth mentioning that a total of 11 papers explored both cognitive and non-cognitive thought processes with SR.
Finally, nine studies applied SR to investigate patterns of higher-order thinking, such as creative thinking and critical thinking, as well as the collaborative process (Rissanen et al., 2019 ; Schindler and Lilienthal, 2020 ; Łucznik and May, 2021 ). The application of SR in these studies allowed for a more comprehensive understanding of participants’ thinking processes and the factors that contribute to effective collaboration and higher-order thinking.
Investigating the effect of educational strategies
Another purpose for research employing SR is to investigate how participants’ learning processes and experiences are affected by instructional design or learning models. Specifically, 35 articles used SR to investigate the impact of specific learning strategies in educational settings, yet 54 studies examined instructional techniques. It seems that SR can facilitate investigating how instructors and students understand and apply newly adopted teaching or learning strategies.
For instance, SR has revealed the pedagogical knowledge base related to the use of dashboards and the provision of feedback by novice teachers (Karimi and Norouzi, 2017 ; Molenaar and Knoop-van Campen, 2018 ; Yu, 2021 ). In terms of the effectiveness of learning techniques, such as computer-enhanced self-directed learning, SR offers a more precise and comprehensive understanding from students’ viewpoints (White et al., 2016 ; Deng, 2020 ; Chu and Zhai, 2023 ).
Extensive empirical studies have shown that data acquired through SR can enhance the interpretability of single-outcome data, such as test results, and also produce more insightful information to evaluate and enhance strategies for improved learning outcomes for both instructors and students (Van der Kleij et al., 2017 ). In these studies, SR provided a deeper comprehension of how instructional design or learning strategies impact participants’ learning experiences and outcomes.
Improving metacognition
Nine articles took advantage of SR to improve participants’ metacognition. One example is using SR in online language learning, where learners can compare feedforward and eye-movement data to develop their metacognitive skills (Zhai et al., 2022 ). Metacognition refers to an individual’s awareness of their thinking processes and understanding of the underlying patterns (Flavell, 1979 ). Educational psychologists have widely acknowledged the significance of metacognition due to its substantial correlation with learners’ academic achievements.
Metacognitive activities usually occur during the self-reflection phase and involve the participants’ evaluation of their own cognition, understanding, and task performance. Using recorded learning processes as stimuli, participants are prompted to explain or evaluate their past behavior instead of simply recalling knowledge. Encouraging student participation in video-stimulated recall conversations enhances their self-reflection and improves their metacognitive skills by giving them a scaffold (Van der Kleij et al., 2017 ).
RQ2: Stimuli
Regarding the stimuli used to arouse recall, video recordings of the learning process have gained tremendous popularity. While some changes have occurred during the evolution of SR methods, such as the improvement of video stimuli and the integration of physiological feedback data.
Optimizing video stimuli
Video recordings are a widely popular type of stimuli in educational SR research, as evidenced by nearly 70% of the reviewed studies (173 articles) that utilized them. This prevalence of video stimuli has been noted in previous review research by Gazdag et al. ( 2019 ), which to some extent, explains the exclusive focus on video stimuli in his study. These recordings commonly consist of real-life scenes from classrooms, laboratories, and screen captures of technology-mediated learning settings. To serve as effective incentives for participants, video recordings should reflect the interactions between instructors and learners, and researchers ought to regulate their length to prevent participant weariness (Lee, 2020 ).
A number of enhancement options have been suggested by researchers. One technique is to use multiple cameras to record and display split-screen videos, providing various perspectives of the learning environment. For instance, Jackson and Cho ( 2018 ) produced a split-screen video recording of teachers’ and students’ simultaneous behaviors, enabling a more potent stimulus for supporting event recall, contextual and situational recall.
Additionally, some researchers have used head-mounted video cameras to record video from the participant’s perspective, visually reproducing original learning scenarios. Such an approach is beneficial in studies examining interpersonal communication, such as those exploring teacher-student interactions or teacher interventions in early childhood peer conflict (Agricola et al., 2021 ; Myrtil et al., 2021 ).
Utilizing biofeedback data
With more accurate and detailed data, biofeedback data (14 articles) is increasingly considered a stimulus choice for self-reflection. Currently, eye-tracking technology is the most commonly used physiological feedback technique. The Eye-Mind hypothesis suggests that eye movements correspond to mental operations, allowing researchers to infer cognitive processes from gaze patterns (Obersteiner and Tumpek, 2016 , p. 257). By combining eye-tracking data with self-reflection, potential ambiguity and uncertainty in eye-tracking techniques are reduced, giving a more thorough overview of the educational process for reflection (Schindler and Lilienthal, 2019 ; El and Windeatt, 2019 ; Chu and Zhai, 2023 ).
Moreover, other physiological indicators have served as informative stimuli in self-reflection. Zhai et al. ( 2018 ) found that online learners’ reading comprehension and cognitive abilities were significantly improve by using both eye-movement and EEG physiological signals as stimuli. Multiple physiological indicators can be included to provide a more thorough and accurate picture of the cognitive and affective states of learners during the learning process.
RQ3: Time factors
Considering time factors is crucial for the utilizing SR method in educational research. This is because time not only influences the selection and processing of stimuli but also has implications for the subsequent interviews. Specifically, enhancing the temporal properties involves both reducing the presentation time and increasing the span of information provided by the stimuli source. Moreover, it is essential to set appropriate time intervals to schedule the interviews. The reviewed literature suggests that the interview schedule may vary depending on the study.
Enhancing the temporal properties of stimuli
Presenting learners with stimuli is intended to assist them in reflecting on their previous learning activities. Nevertheless, if the presentation of stimulus sources persists for too long, it can also impose a heavier cognitive load on learners (Pratt and Martin, 2017 ). In general, stimuli sources in textual, image, and other static formats are more convenient due to their controllable presentation duration for participants. However, for classroom video recordings stimuli, direct video replay may take a considerable amount of time. Considering the time spent, one such approach involves selecting clips from full-length video footage, reducing the duration of the stimuli, and enabling participants to concentrate specifically on behaviors that are pertinent to the research aim (Määttä et al., 2016 ).
The time span of stimuli is also not limited to the classroom. As demonstrated in the 16 studies reviewed, combining multiple data sources has proved more effective. The integration of various materials, including text, video, and pictures, enhances the information capacity and authenticity of the recorded details. For instance, in limited interaction scenarios, researchers often use think-aloud methods, allowing participants to verbalize their thoughts, along with the videotapes, to augment the information provided (Kang and Pyun, 2013 ).
In addition, incorporating stimuli from multiple sources can encompass both subjective and objective aspects. Video recordings only capture a limited timeframe, while learning behaviors extend beyond the confines of the classroom. Cues to stimulate participants’ recall can also come from guide sheets, teacher preparation notes, and student class notes (Chu and Zhai, 2023 ). In an investigation on the use of metacognitive interventions in twenty-first century writing pedagogies, stimuli included a classroom tour video, a literacy autobiography, a teaching plan, and other instructional materials (Jensen, 2019 ).
Setting up flexible intervals
The time interval between in-class instructional activities and SR interviews generally varies across researchers. Among the 149 reviewed studies where the time interval was specified, the majority of the study (126) preferred instant reflection. Instant reflection involves conducting SR interviews as soon as participants finish their learning tasks, typically with only a 5- or 10-min interval or a slight delay according to the timetable for curriculum (White et al., 2016 ; Rassaei, 2015 ; Shintani, 2016 ; Fernandez, 2018 ).
A shorter time span makes sure that participants recollect the task’s cognitive processes more precisely, which improves the accuracy of the interview results (Gass and Mackey, 2000 ). Instant reflection is particularly valuable in studies that require precise information about the learners’ cognitive processes and strategies during the learning task.
However, some researchers (23 studies) purposefully extended the time interval between instruction and recall, for example, 2–4 weeks after the task was completed (Harvey et al., 2014 ). This design may optimize the study by allowing more time for the process of previously recorded raw data and footage (Nurmukhamedov and Kim, 2010 ; Kurki et al., 2016 ). Delayed interviews can also reduce research impact on participants by avoiding interference with subsequent teaching and learning activities (Dos Santos and Hentschke, 2011 ).
RQ4: Interview strategies
During the interview phase of SR, to better guide participants in autonomously reflecting on the teaching and learning process, researchers also need to pay attention to the use of strategies, including the openness and value-oriented nature of the questions.
Posing appropriate questions
SR interviews are utilized to encourage reflective thinking in participants within an open and dialogic environment through questioning strategies. Typically, this kind of interview consists of a succession of open-ended, introspective, and generic questions that do not require predetermined answers. This pattern has been observed in 88 reviewed studies, including research merely posing general questions, as well as those starting with general questions and then progressively narrows down the focus. During these interviews, researchers should take on the role of listeners, serving to train, facilitate, and illuminate while avoiding asking leading questions that could result in biased responses (Ramnarain and Modiba, 2013 ; Egi, 2008 ; Gass and Mackey, 2000 ; Sato, 2019 ). For instance, researchers should avoid yes-no questions that could encourage participants to react a specific way or provide presentational responses. This approach ensures that the purpose of the SR interview is maintained and that the risk of biased responses is minimized (Thararuedee and Wette, 2020 ; Rassaei, 2020 ;).
While questioning in SR interview should leave ample room for participants to retrospect, it must also address the research questions. Thus, 25 papers suggest that questions should be open-ended at the beginning but become increasingly specific as the interview progresses (Stolpe and Björklund, 2013 ). Researchers can use supplementary queries as prompts to ensure that the interview stays on topic and delves deeper into the research questions, depending on participants’ responses (Qiu and Lo, 2017 ; Qiu, 2020 ; Chu and Zhai, 2023 ).
Staying value-neutral in guiding
In addition to the scope of questioning, the neutrality and guidance provided by the interviewer are crucial. Participants receive training before the interview on how to reflect on previous cognitive processes, and the interviewer should remain as neutral as possible during the interview to capture retrospective thinking solely supported by the stimuli (Consuegra et al., 2016 ). If respondents feel that the questions are biased or contain value judgments, they may feel pressured to rationalize or make up explanations, leading to inaccurate reporting of their thoughts. Therefore, the interviewer must carefully design questions wording and adopt a supportive attitude that indicates curiosity in the descriptions provided by participants rather than making judgments (Wu, 2019 ; Schindler and Lilienthal, 2019 ).
RQ5: The relationship between different coding items
In addition to key application procedures such as research aims, stimuli, time factors, and interview strategies, the implementation of SR in educational research is also influenced by intrinsic factors within the educational context, such as learning subjects and educational context. The results of the review (see Table 3 ) indicate that SR is primarily employed within the fields of linguistics (115 articles) and teacher education (48 articles), with relatively fewer studies in areas such as the arts (9 articles). Over 75% of the articles still apply SR in physics learning environments, while nearly 20% explore the use of SR in online platforms.
To enhance the exploratory nature of the research discussion, the current study delved deeper into the intricate relationship between coding items. It is important to note that only outcomes warranting further exploration and discussion are presented in the subsequent section.
The relationship between research aims and learning subject
This bubble chart (Fig. 3 ) illustrates the connection between research aims and learning subjects, with the size of the bubbles indicating the number of relevant papers reviewed. Our current analysis aimed to explore whether SR is more suitable for investigating specific research questions in different disciplines.
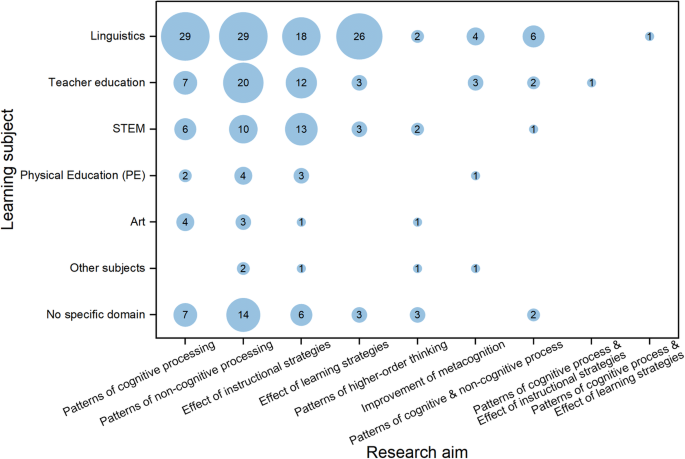
The relationship between research aims and learning subjects is depicted in this bubble chart, where the size of the bubbles represents the quantity of relevant studies.
Regarding research aims, SR was primarily used to investigate patterns of non-cognitive processing and the effect of instructional strategies across all subjects. In linguistics, researchers most frequently utilized SR to explore patterns of cognitive processing (29 articles), non-cognitive processing (29 articles), and learning strategies (26 articles). Another six studies focused on both cognitive and non-cognitive occurrences in linguistic teaching and learning. These findings are consistent with prior research highlighting the importance of non-cognitive factors (e.g., motivation) and learning strategies in language learning (Lin et al., 2017 ). Furthermore, 20 studies using SR investigated non-cognitive elements in teacher education contexts where teachers’ non-cognitive factors, such as intrinsic motivation, are strongly associated with their professional development (Maaranen et al., 2019 ).
In the realm of educational subjects, SR has also occupied a pivotal within the domain of STEM and art education research. Within the STEM disciplines, researchers have employed this methodology to probe the impact of pedagogical strategies (13 articles), non-cognitive processing (10 articles), and cognitive processing (6 articles). Intriguingly, SR has been invoked more frequently to investigate cognitive rather than non-cognitive factors within the sphere of art education (four articles versus three). This inclination may stem from the intricate nature that cognitive processing exhibits in artistic creation (Révész et al., 2017 ). Nonetheless, SR has demonstrated its utility as an effective facilitator, enabling arts educators to acquire profound insights into the cognitive aspects of art instruction and learning. For instance, dos Santos ( 2018 ) documented music teachers’ approaches to the instruction of rhythmic skills as stimuli, facilitating their reflection upon their cognitive processes and their utilization of their didactic content knowledge.
Linguistics and teacher education are two fields that more frequently took advantage of SR as a teaching strategy beyond research methods (Meade and McMeniman, 1992 ; Geiger et al., 2016 ; Sanchez and Grimshaw, 2019 ). Four articles in linguistics and three in teacher education explore using SR to improve participants’ metacognition. In particular, teacher’s professional development and language learning emphasize reflective practice and metacognition (Belvis et al., 2013 ; Zahid and Khanam, 2019 ). For example, research on teachers’ noticing highlights the importance of their cognition and behavior in classroom situations (Chan et al., 2021 ; Amador et al., 2021 ). In language learning, metacognitive awareness has been found to enhance foreign language writing ability, emphasizing the need for metacognitive strategies to improve learners’ skills (Ramadhanti and Yanda, 2021 ; Farahian, 2015 ). These requirements for introspective behavior and metacognition in language learning and teachers’ professional development align with the essential steps and reflective characteristics of SR.
The relationship between stimuli and educational context
The bubble diagram (Fig. 4 ) displays the stimuli and learning environment, with the size of the bubbles corresponding to the number of articles in the review. Our analysis aimed to scrutinize which stimuli are commonly adopted in different learning environments.
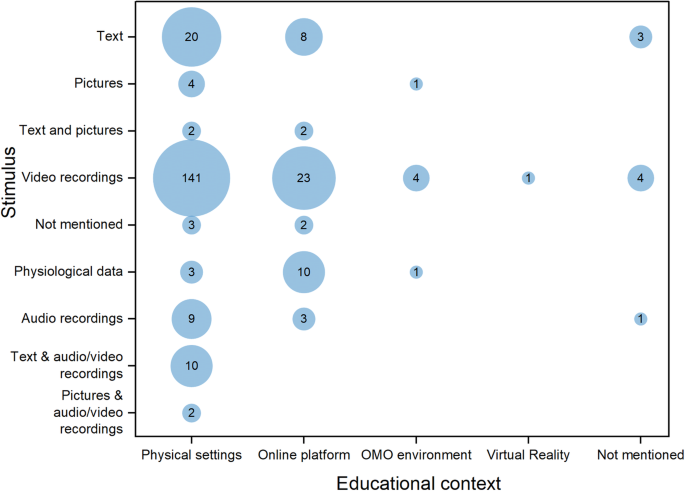
With the size of the bubbles indicating the number of articles in the review, the bubble diagram illustrates connection between learning environment and stimuli.
Firstly, video footage remains the dominant stimulus across various scenarios, with over half of the studies (141 articles) utilizing video in physical learning settings and 23 studies using video footage to stimulate reflection in digital learning platforms and OMO settings. However, there is a disparity in the type of videos used in these settings. Physical learning environments mostly relied on live-action videos that authentically recorded participants’ behaviors and interactions (Chan and Yung, 2015 ; Nyberg and Larsson, 2017 ), whereas digital learning environments utilized device screen recordings that captured participants’ operations on computer-supported learning platforms (Rassaei, 2013 ; Lee, 2020 ).
Secondly, alongside video recordings, physiological data of participants is most frequently used as a stimulus for SR research based on online platforms (10 articles). This trend is reasonable as screen recordings alone may not fully reflect students’ behavior, mainly if they do not perform mouse manipulation or keyboard input. For instance, eye-gaze behaviors provide direct and objective evidence, including fixation duration, fixation count, and scanning path, allowing for stronger conclusions about learners’ cognitive processes and learning strategies (Lai et al., 2013 ; Michel et al., 2020 ).
Thirdly, the diagram indicates that studies utilizing SR in physical environments are more mature and inclined to utilize multimodal stimuli. However, studies in online platforms, OMO environments, and VR environments are still limited, with predominantly homogeneous stimuli. Only one study explored students’ learning strategies in an English task using video footage as stimuli in a virtual reality setting (Park, 2018 ). Thus, more than relying on text, images, or audio and video alone as stimuli is required, and more physiological and multimodal stimuli should be employed in future teaching and learning environments.
The relationship between questioning strategy and research aim
The diagram presented here (Fig. 5 ) displays the questioning strategy and purpose of the study using the SR method, with the size of the bubbles representing the number of articles. Based on this information, our analysis aimed to explore whether the purpose of the study influenced questioning strategies.
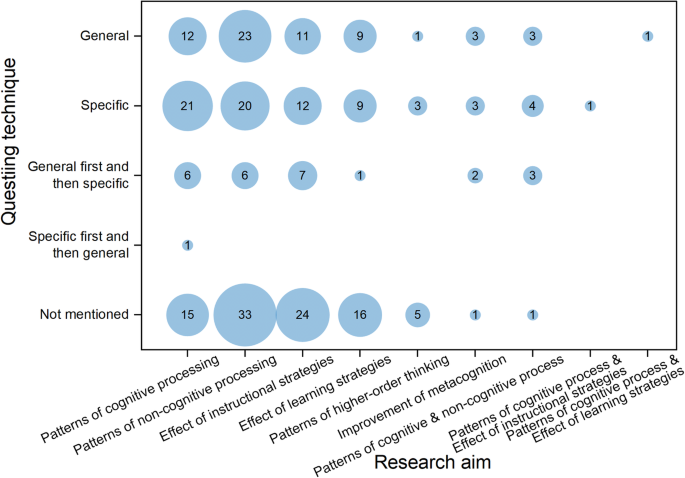
With the size of the bubbles signifying the number of articles, the diagram illustrates the SR questioning strategy and the research aim.
They were excluding articles that did not mention questioning techniques, 23 studies exploring non-cognitive processing utilized general questions during SR, outnumbering studies that implemented more focused questioning strategies (20 articles). This preference for general questioning may stem from the diverse and individualized nature of non-cognitive skills, which include motivation, responsibility, and perseverance (Smithers et al., 2018 ). Consequently, general questions are more appropriate as they allow participants to autonomously recall non-cognitive processing activities with the aid of stimulus materials. Moreover, reflection on non-cognitive processing is prone to interference from external factors. If interview questions are too directed towards the research objectives, they may interfere with the results.
In contrast, studies focusing on cognitive processing patterns predominantly utilized questioning sessions centered on research questions (21 articles), nearly double the number of studies using general questioning strategies (14 articles). Cognitive processing is often intimately related to the teaching or learning activity. Thus, researchers tend to focus their questioning on the learning activity that concerns the research goals. Notably, one article exploring cognitive patterns adopted a different questioning technique: focused first and then general. This article investigated what musicians learned when teaching older adults (Perkins et al., 2015 ). On the one hand, the research questions themselves were exploratory, and the researcher expected participants to provide more cognitive information. On the other hand, this phenomenon may also reflect the divergent and creative thinking of art learning, requiring questions that encourage participants to reflect freely after satisfying the research objectives.
RQ6: Potential improvements
In addition to exploring how effectively employ SR in education, the current review also points to possible future directions on SR research with existing models of computer-supported learning and technology-assisted instruction.
Enhancing the dependability of outcomes through the synthesis of multifaceted data sources
Table 3 shows that a mere fraction under 10% of the studies (16 articles) utilized multi-source stimuli. Indeed, the amalgamation of data derived from disparate stimuli can provide a complementary and robust scaffold for the outcomes of SR. This is attributable to the fact that the integration of heterogeneous types of stimuli broadens the information spectrum, providing participants with supplementary prompts that facilitate the recollection of cognitive processes. Such an approach diminishes the cognitive load on subjects, assists them in articulating more accurate reflections, and arguments the reliability of SR. Furthermore, this practice contributes to the transparency of educational research (D’Oca and Hrynaszkiewicz, 2015 ). For instance, combining video, audio, and text stimuli can offer a more comprehensive and nuanced understanding of learners’ cognitive processes and behaviors.
Additionally, using multimodal stimuli can help address the limitations of using a single type of stimuli and enhance the ecological validity of the study by better replicating real-world learning environments. Some researchers (e.g., Rankanen et al., 2022 ) conducted a study that employed both quantitative and qualitative methods to investigate the impact of non-instructional clay-making in art education on learners’ creative thinking and positive emotion stimulation. By combining multiple data sources, including physiological data such as heart rate variability (HRV) and electrocardiogram signals, this study provides a more detailed understanding of the art experience and the mechanisms at work in different art forms. Unlike previous research that relied solely on questionnaires, this study includes more objective and in-depth quantitative data analyses of art learning tasks. Additionally, the researchers used video-stimulated recall in addition to HRV data to provide a comprehensive perspective on the learners’ experience of non-instructional clay-making in art education. Including qualitative data can reveal the positive or negative value of the emotional experience of artmakers and provide a more nuanced understanding of the emotional complexity of art.
Strengthening the acquiring and processing of stimuli by adopting intelligent algorithms
The synthesis of the review indicates that over 70% of the studies (185 articles) employ video recordings as a singular or combined source of stimuli as depicted in Table 3 . Therefore, the employment of AI algorithms to refine the processing of video stimuli could markedly enhance the application of SR in education research.
Firstly, algorithm-supported techniques can assist in selecting the relevant learner interaction portion of video stimuli, thus shortening the length of SR and automatically extracting key information. Wass and Moskal ( 2017 ) proposed an automatic video annotation tool, which scaffolds more profound reflections and reduces the cognitive load in participating instructors and students. This intelligent excerpting and annotation process saves time and reduces labor, thus improving the efficiency of SR. Furthermore, algorithm-supported techniques can help to automate the coding and analysis of the data, reducing the potential for human error and increasing the reliability of the findings.
Intelligent algorithms can effectively address the challenge of identifying specific moments or events in classroom videos that are relevant to research questions and require meticulous observation, particularly in cases where the video playback duration is extended. Recent advances in computer vision and machine learning have made it possible to automatically extract valuable information from classroom videos, such as the head pose, gaze direction, and facial expressions of instructors and learners, as well as synchronous behaviors between neighboring students (Goldberg et al., 2021 ). Furthermore, the use of Convolutional Neural Networks (CNN) and Deep Neural Network (DNN) enables the analysis of audiovisual data to identify and annotate class environments, such as the teacher’s instructional strategies, student engagement, and classroom management (Ramakrishnan et al., 2023 ). The application of these intelligent algorithms has significant implications for using video recordings in SR, as they provide an accurate and comprehensive depiction of the classroom experience, enabling a more efficient analysis of video recordings in SR. By integrating intelligent algorithms, the effectiveness of retrospection can be enhanced, as algorithm-supported stimuli playback offers a reflective cognitive scaffolding beyond the mere recollection of the learning process.
Considering that many researchers have begun to incorporate physiological data as a source of stimuli (14 articles, as indicated in Table 3 ), the application of computer vision or machine learning algorithms could also be instrumental in capturing and analyzing learners’ physiological data in a lightweight manner, such as recognizing and analyzing their gestures and micro-expressions via webcam, which enriches the informativeness of stimuli (Zhai et al., 2022 ). Machine learning algorithms can now identify and analyze patterns in learner behavior that may not be apparent to human observers, providing a more nuanced understanding of cognitive processes. Moreover, intelligent algorithms enhance the reliability of findings and can also prevent the potential for the Hawthorne effect resulting from direct observation and data collection.
Facilitating the implementation interviews by using virtual agents powered by generative AI
Interviews are instrumental in the process of SR, with the majority of researchers opting to pose not merely general enquiries, but targeted ones (99 articles, as referenced in Table 3 ). This necessitated the undertaking of comprehensive interviews with each participant, a process that is notably time-intensive and requires substantial human endeavor. Future research could explore the use of virtual agents supported by generative AI technologies as an alternative approach to completing the questioning process of SR. Educational research has shown that intelligent agents positively affect learner motivation, academic performance, and cognitive load, making them ideal for training learners’ metacognitive abilities (Dinçer and Doğanay, 2017 ; Kautzmann and Jaques, 2019 ).
Intelligent conversational agents powered by natural language processing (NLP) and large language models (LLM) can replace researchers in providing participants with questions that prompt their recall and offer adaptive feedback based on their responses (Bozkurt, 2023 ). Employing intelligent agents to conduct interviews increases the number of subjects without increasing labor costs. For instance, OpenAI has developed several cutting-edge AI technologies, including the GPT series of language models such as ChatGPT, which can presumably be applied to provide personalized intelligent tutoring services in which feedback-enabled iterative learning occurs (Qadir, 2022 ). Furthermore, the LangChain architecture makes it easier to develop domain-specific agents. Such technologies provide tailored feedback to learners, enhancing their metacognitive awareness and learning outcomes. Additionally, recent advancements in generative AI have shown promising results in producing various forms of multimedia content, including text, images, videos, and 3D models (Gozalo-Brizuela and Garrido-Merchan, 2023 ). This ability to generate multimodal content aligns with the Cognitive Theory of Multimedia Learning, which emphasizes using multiple sensory channels to facilitate learning experiences (Mayer, 2002 ; Mayer and Moreno, 2003 ). By providing learners with diverse visual and auditory information, this technology can enhance the effectiveness of educational activities and promote reflection among instructors and students.
Nonetheless, using virtual agents in educational SR research raises ethical and privacy concerns that require attention in future studies. Firstly, the employment of generative AI in SR interviews involves communicating students’ sensitive data, including grades or personal information. Secondly, conversational virtual agents are trained on specific data, leading to the possibility of biased and discriminatory responses when posing SR questions. Therefore, SR researchers must utilize generative AI responsibly and ethically (Mhlanga, 2023 ).
Conclusions
This study reviews 257 empirical articles on using SR in education research from 2012 to 2022. The paper examines the changes and adaptations of the SR method in the present educational landscape, where virtual and online spaces are prevalent, and technological tools are increasingly involved in the teaching and learning process.
The revealed that researchers frequently employed SR to investigate participants’ internal viewpoints and thoughts, improving their metacognitive abilities. In terms of stimuli selection and processing, the commonly employed video stimuli undergo continuous advancements. Moreover, the sources of stimuli are becoming diverse, with the inclusion of physiological feedback data. Additionally, providing participants with space to respond to interview questions is crucial. Researchers should ensure the discussion does not deviate from the research questions and avoid influencing participants’ thoughts.
Furthermore, using technologies such as generative AI can enhance the reliability and generalizability of SR, and the study proposes suggestions for future research in result enhancement, stimuli optimization, and interview implementation. This study provides theoretical supplementation to manifesting the Retrocue Effect in educational settings. It strengthens the Cognitive Theory of Multimedia Learning (CTML) with specific pedagogical strategies by combining it with SR. From a practical perspective, the current research synthesizes current findings and can serve as a valuable reference for educators and researchers in this field.
As with any systematic review, the current research has limitations inherent to the selection and filtering process. Primarily, the sample size is restricted to articles available through the Web of Science, IEEE, and Scopus databases. There might be relevant and high-qualify studies published outside these three databases and are worthy studying. We hope future researchers can build on our research and offer a more comprehensive review of the use of SR in education.
In addition, education has now entered the era of Metaverse and artificial intelligence (Wang et al., 2022 ). How can instructors effectively apply SR in 3D virtual learning environments and in learning setting empowered by AI and AIGC (AI-generated content) remains a new territory for our continued research.
Data availability
All data generated or analyzed during this study are included in this published article.
Agricola BT, Prins FJ, van der Schaaf MF et al. (2021) Supervisor and student perspectives on undergraduate thesis supervision in higher education. Scand J Educ Res 65(5):877–897
Article Google Scholar
Al Mamun MA, Lawrie G, Wright T (2020) Instructional design of scaffolded online learning modules for self-directed and inquiry-based learning environments. Comput Educ 144:103695
Amador JM, Bragelman J, Superfine AC (2021) Prospective teachers’ noticing: a literature review of methodological approaches to support and analyze noticing. Teach Teach Educ 99:103256
Belvis E, Pineda P, Armengol C et al. (2013) Evaluation of reflective practice in teacher education. Eur J Teach Educ 36(3):279–292
Bloom BS (1953) Thought-processes in lectures and discussions. J Gen Educ 7(3):160–169
Google Scholar
Bogard T, Liu M, Chiang YHV (2013) Thresholds of knowledge development in complex problem solving: a multiple-case study of advanced learners’ cognitive processes. Educ Tech Res Dev 61(3):465–503
Bowles MA (2018) Introspective verbal reports: think-alouds and stimulated recall. In: Phakiti A, De Costa P, Plonsky L et al. (eds.) The Palgrave handbook of applied linguistics research methodology. Palgrave Macmillan, London, p 423-457
Bozkurt A (2023) Generative artificial intelligence (AI) powered conversational educational agents: the inevitable paradigm shift. Asian J Distance Educ 18(1):198–204
Calderhead J (1981) Stimulated recall: a method for research on teaching. Br J Educ Psychol 51(2):211–217
Cao Z, Yu S, Huang J (2019) A qualitative inquiry into undergraduates’ learning from giving and receiving peer feedback in L2 writing: Insights from a case study. Stud Educ Eval 63:102–112
Chan KKH, Yung BHW (2015) On-site pedagogical content knowledge development. Int J Sci Educ 37(8):1246–1278
Chan KKH, Xu L, Cooper R et al. (2021) Teacher noticing in science education: do you see what I see? Stud Sci Educ 57(1):1–44
Chu X, Zhai X (2023) A Systematic Review of Stimulated Recall (SR) in Education from 2012 to 2021. In: Shih JL et al. (eds.), Main Conference Proceedings (English Paper) of the 27th Global Chinese Conference on Computers in Education (GCCCE 2023) (pp. 100-108). China: Beijing Normal University. https://aic-fe.bnu.edu.cn/fj/lunwenji2023/EnglishPaper.pdf
Consuegra E, Engels N, Willegems V (2016) Using video-stimulated recall to investigate teacher awareness of explicit and implicit gendered thoughts on classroom interactions. Teach Teach 22(6):683–699
D’Oca G, Hrynaszkiewicz I (2015) Palgrave Communications’ commitment to promoting transparency and reproducibility in research. Palgr Commun. 1(1):1–3
Deng L (2020) Laptops and mobile phones at self-study time: examining the mechanism behind interruption and multitasking. Australas J Educ Technol 36(1):55–67
Dewey J (1933) How we think: a restatement of the relation of reflective thinking to the educative process. DC Heath
Dinçer S, Doğanay A (2017) The effects of multiple-pedagogical agents on learners’ academic success, motivation, and cognitive load. Comput Educ 111:74–100
dos Santos RAT (2018) Ways of using musical knowledge to think about one’s piano repertoire learning: three case studies. Music Educ Res 20(4):427–445
Dos Santos RAT, Hentschke L (2011) Praxis and poiesis in piano repertoire preparation. Music Educ Res 13(3):273–292
dos Santos S, Loveridge J (2014) Using video to promote early childhood teachers’ thinking and reflection. Teach Teach Educ 41:42–51
Duarte A, Hearons P, Jiang Y et al. (2013) Retrospective attention enhances visual working memory in the young but not the old: an ERP study. Psychophysiology 50(5):465–476
Article PubMed PubMed Central Google Scholar
Duo S, Song LX (2012) An e-learning system based on affective computing. Phys Procedia 24:1893–1898
Article ADS Google Scholar
Egi T (2008) Investigating stimulated recall as a cognitive measure: Reactivity and verbal reports in SLA research methodology. Lang Aware 17(3):212–228
El E, Windeatt S (2019) Eye tracking analysis of EAP Students’ regions of interest in computer-based feedback on grammar, usage, mechanics, style and organization and development. System 83:36–49
Endacott JL (2016) Using video-stimulated recall to enhance preservice-teacher reflection. N Educ 12(1):28–47
Farahian M (2015) Assessing EFL learners’ writing metacognitive awareness. J Lang Linguist Stud 11(2):39–51
Fernandez CJ (2018) Behind a spoken performance: test takers’ strategic reactions in a simulated part 3 of the IELTS speaking test. Lang Test Asia 8(1):18
Flavell JH (1979) Metacognition and cognitive monitoring:a new area of cognitive–developmental inquiry. Am Psychol 34(10):906
Gass SM, Mackey A (2000) Stimulated recall methodology in second language research. Routledge, New York
Gass SM, Mackey A (2016) Stimulated recall methodology in applied linguistics and L2 research. Routledge, New York
Book Google Scholar
Gazdag E, Nagy K, Szivák J (2019) “I Spy with My Little Eyes…” The use of video stimulated recall methodology in teacher training–The exploration of aims, goals and methodological characteristics of VSR methodology through systematic literature review. Int J Educ Res 95:60–75
Geiger V, Muir T, Lamb J (2016) Video-stimulated recall as a catalyst for teacher professional learning. J Math Teach Educ 19:457–475
Gijselaers HJ, Kirschner PA, Verboon P et al. (2016) Sedentary behavior and not physical activity predicts study progress in distance education. Learn Individ Differ 49:224–229
Goldberg P, Sümer Ö, Stürmer K et al. (2021) Attentive or not? Toward a machine learning approach to assessing students’ visible engagement in classroom instruction. Educ Psychol Rev 33:27–49
Golhasany H, Harvey B (2023) Capacity development for knowledge mobilization: a scoping review of the concepts and practices. Hum Soc Sci Commun 10(1):1–12
Gozalo-Brizuela R, Garrido-Merchan EC (2023) ChatGPT is not all you need. A State-of-the-Art Review of large Generative AI models. arXiv. https://arxiv.org/abs/2301.04655
Harvey W, Wilkinson S, Pressé C et al. (2014) Children say the darndest things: physical activity and children with attention-deficit hyperactivity disorder. Phys Educ Sport Pedag 19(2):205–220
Heikonen L, Toom A, Pyhältö K et al. (2017) Student-teachers’ strategies in classroom interaction in the context of the teaching practicum. J Educ Teach 43(5):534–549
Hu J, Gao X (2020) Appropriation of resources by bilingual students for self-regulated learning of science. Int J Biling Educ Biling 23(5):567–583
Hu J, Wu P (2020) Understanding English language learning in tertiary English-medium instruction contexts in China. System 93:102305
Jackson DO, Cho M (2018) Language teacher noticing: a socio-cognitive window on classroom realities. Lang Teach Res 22(1):29–46
Jacobson MJ, Wilensky U (2006) Complex systems in education: scientific and educational importance and implications for the learning sciences. J Learn Sci 15(1):11–34
Jacobson MJ, Levin JA, Kapur M (2019) Education as a complex system: conceptual and methodological implications. Educ Res 48(2):112–119
Jensen A (2019) Fostering preservice teacher agency in 21st century writing instruction. Engl Teach Pract Crit 18(3):298–311
Kang YS, Pyun DO (2013) Mediation strategies in L2 writing processes: a case study of two Korean language learners. Lang Cult Curric 26(1):52–67
Karimi MN, Norouzi M (2017) Scaffolding teacher cognition: changes in novice L2 teachers’ pedagogical knowledge base through expert mentoring initiatives. System 65:38–48
Kautzmann TR, Jaques PA (2019) Effects of adaptive training on metacognitive knowledge monitoring ability in computer-based learning. Comput Educ 129:92–105
Keith MJ (1988) Stimulated recall and teachers’ thought processes: a critical review of the methodology and an alternative perspective. Paper presented at the 17th Annual Meeting of the Mid-South Educational Research Association, Louisville, KY, 9−11
Koltovskaia S (2020) Student engagement with automated written corrective feedback (AWCF) provided by Grammarly: a multiple case study. Assess 44:100450
Kurki K, Järvenoja H, Järvelä S et al. (2016) How teachers co-regulate children’s emotions and behaviour in socio-emotionally challenging situations in day-care settings. Int J Educ Res 76:76–88
Lai ML, Tsai MJ, Yang FY et al. (2013) A review of using eye-tracking technology in exploring learning from 2000 to 2012. Educ Res Rev 10:90–115
Lambert C, Zhang G (2019) Engagement in the use of English and Chinese as foreign languages: the role of learner‐generated content in instructional task design. Mod Lang J 103(2):391–411
Lee C (2020) A study of adolescent English learners’ cognitive engagement in writing while using an automated content feedback system. Comput Assist Lang. Learn 33(1-2):26–57
Lichtinger E, Kaplan A (2015) Employing a case study approach to capture motivation and self-regulation of young students with learning disabilities in authentic educational contexts. Metacogn Learn 10(1):119–149
Lin CH, Zhang Y, Zheng B (2017) The roles of learning strategies and motivation in online language learning: a structural equation modeling analysis. Comput Educ 113:75–85
Lindfors M, Bodin M, Simon S (2020) Unpacking students’ epistemic cognition in a physics problem‐solving environment. J Res Sci Teach 57(5):695–732
Łucznik K, May J (2021) Measuring individual and group flow in collaborative improvisational dance. Think Skills Creat 40:100847
Lyle J (2003) Stimulated recall: a report on its use in naturalistic research. Br Educ Res J 29(6):861–878
Maaranen K, Kynäslahti H, Byman R et al. (2019) Teacher education matters: finnish teacher educators’ concerns, beliefs, and values. Eur J Teach Educ 42(2):211–227
Määttä E, Mykkänen A, Järvelä S (2016) Elementary schoolchildrenas self-and social perceptions of success. J Res Child Educ 30(2):170–184
Martinelle R (2018) Video-stimulated recall: aiding teacher practice. Educ Leadersh 76(3):55
Martinelle R (2020) Using video-stimulated recall to understand the reflections of ambitious social studies teachers. J Soc Stud Res 44(3):307–322
Mayer RE, Moreno R (1998) A cognitive theory of multimedia learning: Implications for design principles. J Educ Psychol 91(2):358–368
Mayer RE, Moreno R (2003) Nine ways to reduce cognitive load in multimedia learning. Educ Psychol 38(1):43–52
Mayer RE (2002) Multimedia learning. In: Brian HR (ed.) Psychology of learning and motivation, vol 41. Academic Press, Cambridge, p 85-139
Meade P, McMeniman M (1992) Stimulated recall—An effective methodology for examining successful teaching in science. Aust Educ Res 19(3):1–18
Mhlanga D (2023) Open AI in Education, the Responsible and Ethical Use of ChatGPT Towards Lifelong Learning. In: Mhlanga D (ed.) FinTech and Artificial Intelligence for Sustainable Development. Sustainable Development Goals Series. Palgrave Macmillan, Cham, pp 387-409
Michel M, Révész A, Lu X et al. (2020) Investigating L2 writing processes across independent and integrated tasks: a mixed-methods study. Second Lang Res 36(3):307–334
Moher D, Liberati A, Tetzlaff J et al. (2009) Preferred reporting items for systematic reviews and meta-analyses: the PRISMA statement. Ann Intern Med 151(4):264–269
Article PubMed Google Scholar
Molenaar I, Knoop-van Campen CA (2018) How teachers make dashboard information actionable. IEEE Trans Learn Technol 12(3):347–355
Moreno R, Mayer RE (1999) Cognitive principles of multimedia learning: the role of modality and contiguity. J Educ Psychol 91(2):358
Myrtil MJ, Lin TJ, Chen J et al. (2021) Pros and (con) flict: using head-mounted cameras to identify teachers’ roles in intervening in conflict among preschool children. Early Child Res Q 55:230–241
Nguyen NT, McFadden A, Tangen D et al. (2013) Video-stimulated recall interviews in qualitative research. Paper presented at the Annual Meeting of the Australian Association for Research in Education (AARE), Adelaide, Australia, 1-5 December 2013
Nurmukhamedov U, Kim SH (2010) ‘Would you perhaps consider…’: hedged comments in ESL writing. ELT J 64(3):272–282
Nyberg G, Larsson H (2017) Physical education teachers’ content knowledge of movement capability. J Teach Phys Educ 36(1):61–69
Obersteiner A, Tumpek C (2016) Measuring fraction comparison strategies with eye-tracking. ZDM 48(3):255–266
Page MJ, McKenzie JE, Bossuyt PM et al. (2021) The PRISMA 2020 statement: an updated guideline for reporting systematic reviews. Int J Surg 88:105906
Park M (2018) Innovative assessment of aviation English in a virtual world: windows into cognitive and metacognitive strategies. ReCALL 30(2):196–213
Peng JE (2020) Teacher interaction strategies and situated willingness to communicate. ELT J 74(3):307–317
Perkins R, Aufegger L, Williamon A (2015) Learning through teaching: exploring what conservatoire students learn from teaching beginner older adults. Int J Music Educ 33(1):80–90
Pratt SM, Martin AM (2017) The differential impact of video-stimulated recall and concurrent questioning methods on beginning readers’ verbalization about self-monitoring during oral reading. Read Psychol 38(5):439–485
Qadir J (2022) Engineering education in the era of ChatGPT: promise and pitfalls of generative AI for education. In: 2023 IEEE Global Engineering Education Conference (EDUCON), Kuwait, Kuwait, 1-4 May 2023
Qiu X (2020) Functions of oral monologic tasks: effects of topic familiarity on L2 speaking performance. Lang Teach Res 24(6):745–764
Qiu X, Lo YY (2017) Content familiarity task repetition and Chinese EFL learners’ engagement in second language use. Lang Teach Res 21(6):681–698
Ramadhanti D, Yanda DP (2021) Students’ metacognitive awareness and its impact on writing skill. Int J Lang Educ 5(3):193–206
Ramakrishnan A, Zylich B, Ottmar E et al. (2023) Toward automated classroom observation: multimodal machine learning to estimate class positive climate and negative climate. IEEE Trans Affect Comput 14(1):664–679
Ramnarain UD, Modiba M (2013) Critical friendship collaboration and trust as a basis for self-determined professional development: a case of science teaching. Int J Sci Educ 35(1):65–85
Rankanen M, Leinikka M, Groth C et al. (2022) Physiological measurements and emotional experiences of drawing and clay forming. Arts Psychother 79:101899
Rassaei E (2013) Corrective feedback, learners’ perceptions, and second language development. System 41(2):472–483
Rassaei E (2015) Journal writing as a means of enhancing EFL learners’ awareness and effectiveness of recasts. Linguist Educ 32:118–130
Rassaei E (2020) The separate and combined effects of recasts and textual enhancement as two focus on form techniques on L2 development. System 89:102193
Révész A, Kourtali NE, Mazgutova D (2017) Effects of task complexity on L2 writing behaviors and linguistic complexity. Lang Learn 67(1):208–241
Rietdijk S, van Weijen D, Janssen T et al. (2018) Teaching writing in primary education: classroom practice, time, teachers’ beliefs and skills. J Educ Psychol 110(5):640
Rissanen I, Kuusisto E, Hanhimäki E et al. (2018) The implications of teachers’ implicit theories for moral education: a case study from Finland. J Moral Educ 47(1):63–77
Rissanen I, Kuusisto E, Tuominen M et al. (2019) In search of a growth mindset pedagogy: a case study of one teacher’s classroom practices in a Finnish elementary school. Teach Teach Educ 77:204–213
Sanchez HS, Grimshaw T (2019) Stimulated recall. In: McKinley J, Rose H (eds.) The Routledge handbook of research methods in applied linguistics. Routledge, Abingdon, pp 312-323
Sato R (2019) Fluctuations in an EFL teacher’s willingness to communicate in an English-medium lesson: an observational case study in Japan. Innov Lang Learn Teach 13(2):105–117
Schindler M, Lilienthal AJ (2019) Domain-specific interpretation of eye tracking data: towards a refined use of the eye-mind hypothesis for the field of geometry. Educ Stud Math 101(1):123–139
Schindler M, Lilienthal AJ (2020) Students’ creative process in mathematics: insights from eye-tracking-stimulated recall interview on students’ work on multiple solution tasks. Int J Sci Math Educ 18(8):1565–1586
Schneider D, Barth A, Getzmann S et al. (2017) On the neural mechanisms underlying the protective function of retroactive cuing against perceptual interference: evidence by event-related potentials of the EEG. Biol Psychol 124:47–56
Shepherdson P, Oberauer K, Souza AS (2018) Working memory load and the retro-cue effect: a diffusion model account. J Exp Psychol Hum Percept Perform 44(2):286
Shintani N (2016) The effects of computer-mediated synchronous and asynchronous direct corrective feedback on writing: a case study. Comput Assist Lang Learn 29(3):517–538
Smagorinsky P (1998) Thinking and speech and protocol analysis. Mind Cult Act 5(3):157–177
Smithers LG, Sawyer AC, Chittleborough CR et al. (2018) A systematic review and meta-analysis of effects of early life non-cognitive skills on academic, psychosocial, cognitive and health outcomes. Nat Hum Behav 2(11):867–880
Souza AS, Oberauer K (2016) In search of the focus of attention in working memory: 13 years of the retro-cue effect. Atten Percept Psycho 78:1839–1860
Stolpe K, Björklund L (2013) Students’ long-term memories from an ecology field excursion: retelling a narrative as an interplay between implicit and explicit memories. Scand J Educ Res 57(3):277–291
Stough LM (2001) Using Stimulated Recall in Classroom Observation and Professional Development. Paper presented at the Annual Meeting of the American. Educational Research Association, Seattle, WA, 10-14 April 2001
Sundberg B, Areljung S, Due K et al. (2018) Opportunities for and obstacles to science in preschools: views from a community perspective. Int J Sci Educ 40(17):2061–2077
Tan ST, Tan CX, Tan SS (2021) Physical activity, sedentary behavior, and weight status of university students during the covid-19 lockdown: a cross-national comparative study. Int J Environ Res Public Health 18(13):7125
Article CAS PubMed PubMed Central Google Scholar
Thararuedee P, Wette R (2020) Attending to learners’ affective needs: teachers’ knowledge and practices. System 95:102375
Tiainen O, Korkeamäki RL, Dreher MJ (2018) Becoming reflective practitioners: a case study of three beginning pre-service teachers. Scand J Educ Res 62(4):586–600
Ucan S, Webb M (2015) Social regulation of learning during collaborative inquiry learning in science: how does it emerge and what are its functions? Int J Sci Educ 37(15):2503–2532
Van der Kleij F, Adie L, Cumming J (2017) Using video technology to enable student voice in assessment feedback. Br J Educ Technol 48(5):1092–1105
Walan S, Enochsson AB (2019) The potential of using a combination of storytelling and drama, when teaching young children science. Eur Early Child Educ Res J 27(6):821–836
Wang M, Yu H, Bell Z et al. (2022) Constructing an Edu-Metaverse ecosystem: a New and innovative framework. IEEE Trans Learn Technol 15(6):685–696
Wass R, Moskal ACM (2017) What can Interpersonal Process Recall (IPR) offer academic development? Int J Acad Dev 22(4):293–306
White C, Direnzo R, Bortolotto C (2016) The learner-context interface: emergent issues of affect and identity in technology-mediated language learning spaces. System 62:3–14
Wijayasundara M (2020) Integration of ICT in teaching and learning in schools. Int J Res 1(10):198–209
Wilby KJ, Govaerts MJ, Austin Z et al. (2017) Exploring the influence of cultural orientations on assessment of communication behaviours during patient-practitioner interactions. BMC Med Educ 17(1):1–10
Wu SC (2019) Researching children’s learning and play in a Chinese context: children’s perspectives on their play and learning. Eur Early Child Educ Res J 27(4):551–565
Yu S (2021) Giving genre-based peer feedback in academic writing: sources of knowledge and skills, difficulties and challenges. Assess Eval High Educ 46(1):36–53
Yu S, Hu G (2017) Understanding university students’ peer feedback practices in EFL writing: Insights from a case study. Assess Writ 33:25–35
Zahid M, Khanam A (2019) Effect of reflective teaching practices on the performance of prospective teachers. Turk Online J Educ Technol 18(1):32–43
Zhai X, Fang Q, Dong Y et al. (2018) The effects of biofeedback‐based stimulated recall on self‐regulated online learning: a gender and cognitive taxonomy perspective. J Comput Assist Learn 34(6):775–786
Zhai X, Chu X, Meng N et al. (2022) The effect of multi-mode stimuli of feedforward and eye tracking on metacognition—an exploratory study using digital dictionaries. Educ Technol Soc 25(1):213–227
Zhai X, Xu J, Chen NS et al. (2022) The Syncretic Effect of Dual-Source Data on Affective Computing in Online Learning Contexts: A Perspective From Convolutional Neural Network With Attention Mechanism. J Educ Comput Res 61(2):466–493
Download references
Acknowledgements
This research is funded by the National Science and Technology Major Project (Grant No. 2022ZD0115904), 2021 National Natural Science Foundation of China (Grant No. 62177042), and 2024 Zhejiang Provincial Natural Science Foundation of China (Grant No. Y24F020039).
Author information
These authors contributed equally: Xuesong Zhai, Xiaoyan Chu.
Authors and Affiliations
College of Education, Zhejiang University, Hangzhou, China
Xuesong Zhai & Xiaoyan Chu
Hangzhou International Urbanology Research Center & Zhejiang Urban Governance Studies Center, Hangzhou, China
Xuesong Zhai
Department of Mathematics and Information Technology, The Education University of Hong Kong, Hong Kong SAR, China
Minjuan Wang
Learning Design and Technology, San Diego State University, San Diego, CA, USA
Program of Learning Sciences and Institute for Research Excellence in Learning Sciences, National Taiwan Normal University, Taipei, Taiwan
Chin-Chung Tsai & Jyh-Chong Liang
Department of Learning Technologies, University of North Texas, Denton, TX, USA
Jonathan Michael Spector
You can also search for this author in PubMed Google Scholar
Contributions
All authors have contributed to material preparation and data analysis. Conceptualization, design and data collection: XS Zhai, and XY Chu. Methodology: All. Original draft: XS Zhai and XY Chu. Second draft: MJ Wang. Third draft: CC Tsai and JC Liang. Final round revision and quality check: JM Spector. All authors discussed the results and reviewed, edited, and approved the final version of the manuscript.
Corresponding authors
Correspondence to Xiaoyan Chu or Minjuan Wang .
Ethics declarations
Competing interests.
The author declares no competing interests.
Ethical approval
Ethical approval was not required as the study did not involve human participants.
Informed consent
This article does not contain any studies with human participants performed by any of the authors.
Additional information
Publisher’s note Springer Nature remains neutral with regard to jurisdictional claims in published maps and institutional affiliations.
Supplementary information
Rights and permissions.
Open Access This article is licensed under a Creative Commons Attribution 4.0 International License, which permits use, sharing, adaptation, distribution and reproduction in any medium or format, as long as you give appropriate credit to the original author(s) and the source, provide a link to the Creative Commons licence, and indicate if changes were made. The images or other third party material in this article are included in the article’s Creative Commons licence, unless indicated otherwise in a credit line to the material. If material is not included in the article’s Creative Commons licence and your intended use is not permitted by statutory regulation or exceeds the permitted use, you will need to obtain permission directly from the copyright holder. To view a copy of this licence, visit http://creativecommons.org/licenses/by/4.0/ .
Reprints and permissions
About this article
Cite this article.
Zhai, X., Chu, X., Wang, M. et al. A systematic review of Stimulated Recall (SR) in educational research from 2012 to 2022. Humanit Soc Sci Commun 11 , 489 (2024). https://doi.org/10.1057/s41599-024-02987-6
Download citation
Received : 01 June 2023
Accepted : 25 March 2024
Published : 05 April 2024
DOI : https://doi.org/10.1057/s41599-024-02987-6
Share this article
Anyone you share the following link with will be able to read this content:
Sorry, a shareable link is not currently available for this article.
Provided by the Springer Nature SharedIt content-sharing initiative
Quick links
- Explore articles by subject
- Guide to authors
- Editorial policies

Select either 'Selected records' or 'All records' and then click 'Export to CSV' to begin exporting.

Welcome to the International Database of Education Systematic Reviews.
IDESR is a database of published systematic reviews in Education and a clearinghouse for protocol registration of ongoing and planned systematic reviews. From this page you can:
- dive straight in and search the database
- register a protocol for a planned systematic review
- read more about IDESR ,
- explore the rationale for IDESR
- meet the team behind the IDESR project.
IDESR is now in its second phase of development and is accepting registrations of review protocols for all areas of education . Please check the inclusion criteria and submission guidance here. The library arm will continue to focus on published reviews in Language Education for the time being.
Search IDESR

The IDESR Team are grateful to the John Fell Fund and the Department of Education at the University of Oxford for their support of this pilot phase of IDESR. We believe it will provide proof of concept to inform future funding bids to enable the expansion of IDESR to include systematic reviews from all sub-fields of Education.
Keep up to date with developments at IDESR, including when we will be accepting protocol registrations from fields outside Language Education by following the IDESR blog and on Twitter @idesr_org .

This is the management page visible only to Gordon at the moment
Log in with your IDESR password

Are you registered yet? You can do this here .
Reset your IDESR password
Email address
Register with IDESR
Affiliation
IDESR search
- To search for all records use * as your search term
- To search for truncated words add * to the end of the word, e.g. to search for words that start with caption use caption*
- To search for an exact term use double quotes, e.g. "Captioned Video for L2 listening and vocabulary learning : A meta-analysis."

Type | Title | -->
---|
Record details
Before you register your review, inclusion criteria for idesr protocols.
IDESR accepts registrations of protocols for systematic reviews in all fields of education.
We operationalise systematic reviews as follows:
Systematic reviews are rigorous, transparent and replicable reviews of research literature. High quality systematic reviews aim to identify all relevant published and unpublished research reports on a given topic and provide an unbiased summary of totality of that evidence.
Systematic reviews select for inclusion reports of studies using a series of transparent and replicable steps. These include applying a predetermined systematic search strategy and assessment of the eligibility of studies for inclusion using clear inclusion/exclusion criteria.
The quality of each study included in the review should be assessed for trustworthiness. The criteria by which trustworthiness is assessed is informed by the types of study eligible for the review. For example, reviews of experiments might use the Cochrane Risk of Bias Tool , or Gorard's Sieve . Reviews of qualitative studies might use the Quality in Qualitative Evaluation Tool ; observational studies might use the Newcastle-Ottawa Scale .
The assembled body of evidence should be synthesised using narrative and/or statistical synthesis (meta-analysis) and interpreted taking into account the quality of the studies included.
For more information about the characteristics of high quality systematic reviews, reference should be made to PRISMA (the Preferred Reporting Items for Systematic Reviews and Meta-analyses) at www.prisma-statement.org.
Note: Although the terms are often used interchangeably, a meta-analysis is not a systematic review, it is a statistical technique. A systematic review may incorporate a meta-analysis, but to be considered a systematic review, the study must adhere to the methodological characteristics summarised above.
Protocol Registration
Before embarking on a systematic review, it is good scientific practice to prepare a protocol detailing the steps that will be taken to reduce biases in preparing the review, and to make this protocol publicly available. This helps to guard against poor scientific practices, such as outcome switching, selective reporting, and failure to publish. In addition, protocol registration helps to reduce unnecessary duplication of effort (by allowing prospective reviewers to check if other researchers have already embarked on a review addressing the same or a similar topic), and to foster collaboration (by alerting reviewers to other groups interested in the same or similar topics). It also gives reviewers an opportunity to demonstrate their commitment to open science. This is one of the key objectives of the IDESR Registry.
How to register your protocol
Before you start.
Check IDESR and other relevant databases for systematic reviews that have already been published or that are ongoing or proposed. Satisfy yourself that your proposed review does not unnecessarily duplicate work that has been or is being done.
You should have a complete or near complete protocol. If you intend to have your protocol peer-reviewed, this should be done before you begin the registration process. Protocols should be prepared in accordance with PRISMA-P (the PRISMA extension for protocols for systematic reviews), available here .
Confirm that work on the review has not progressed beyond the search phase. We understand that reviewers may have piloted their search strategy and may have screened some of the records returned in the pilot to help refine their inclusion/exclusion criteria before they are ready to submit a protocol registration. Thus, we do not insist that no work has been undertaken in the preparation of the review prior to registration. However, pre-registration is only helpful in reducing the potential for bias if it happens before the main bulk of the work on the review begins. That said, sometimes during the process of conducting a systematic review, new information comes to light and changes to the protocol are deemed necessary. In such cases, an update to the protocol should be submitted to IDESR. This will be date-stamped and published alongside the original protocol. This provides an audit trail for the review, keeping methodological choices open and transparent.
Protocols for completed and/or published systematic reviews should not be registered.
Protocols registered on IDESR should not be registered elsewhere. To maintain the integrity of the protocol registration process, only one version of a protocol registration should exist.
Submissions to IDESR must be in English. Though great fans of multilingualism, the IDESR team is not able to process applications in languages other than English. However, search terms may be in any language, and authors can include a link to different language versions of the protocol if they wish .
Registering your protocol
To register the protocol for your systematic review, first set up an IDESR account. You can do this here .
Once you have set up your account you will be able to access the protocol registration form. Completion is straightforward and involves entering the relevant information from your protocol into the appropriate fields on the form. A blank version of the form is available here ( PDF / Word ) to assist with preparing your draft protocol before finalising it for upload.
Once you have completed all the fields and clicked 'submit', you will receive a confirmation email and your application will be sent to one of the IDESR team for review. Once it has been reviewed you will receive an email telling you either a) that the application has been accepted for publication; b) that further information is required before your submission can be accepted; or c) that the application has been rejected (stating the reasons for this).
Note: IDESR is a clearinghouse for registrations of systematic review protocols, not an arbiter of the necessity or merit of any individual review, nor a judge of a review's methodological quality. These are matters for the review team, its funders, and the body providing peer review of the research. The IDESR team is responsible only for confirming that a protocol registration reflects the minimum criteria for systematic review protocols, as laid out in PRISMA-P, and to make a permanent, publicly available record of those which do. The decision to accept or reject your application will be made on the basis of these criteria only.
Access to your application will be suspended while it is being processed.
Once accepted, your registration will be converted to a PDF file and added to the IDESR Registry, and it will be made available to view by all users of the site.
Once published on the IDESR website, you will be able to update your registration if necessary. All updates will be date stamped to provide an audit trail.
On completion of your review you should update the record to say that the review is complete and to provide information about where it has been published. Bibliographic information about your published review will be added to the IDESR Library and will include a link back to your IDESR protocol.
Authors of published protocols retain the copyright to their words and grant IDESR exclusive rights to publish them in the form of an IDESR protocol registration. Authors agree to these being published under a Creative Commons Attribution-NonCommercial-NoDerivs licence. This license allows others to download your works and share them with others as long as they credit you, but they can’t change them in any way or use them commercially.
Eligibility criteria for inclusion in IDESR library
Lorem ipsum dolor sit amet, consectetur adipiscing elit, sed do eiusmod tempor incididunt ut labore et dolore magna aliqua. Ut enim ad minim veniam, quis nostrud exercitation ullamco laboris nisi ut aliquip ex ea commodo consequat. Duis aute irure dolor in reprehenderit in voluptate velit esse cillum dolore eu fugiat nulla pariatur. Excepteur sint occaecat cupidatat non proident, sunt in culpa qui officia deserunt mollit anim id est laborum.
Your registered records
Tell us about a systematic review that we don't have.
We have searched extensively for systematic reviews in language education, but inevitably we may have missed some. If you know of a systematic review that we don’t have, we want to know about it. First, please check the IDESR database by searching by author name, keyword or title. If the record does not exist in the IDESR database, please use the form below to let us know. Please complete as many fields as possible.
We operationalise systematic reviews as literature reviews that have a methods section. At minimum, the methods section should describe the way that literature was searched for and what the inclusion criteria were. Ideally, a systematic review will adhere to all defining criteria laid out in the PRISMA statement.
Abstract (if available)
About idesr.
The International Database of Education Systematic Reviews (IDESR) is a free-at-the-point-of-access electronic database of published systematic reviews in the field of Education and an online space where planned and ongoing Education systematic reviews can be registered, searched for and viewed.
IDESR is currently in its first phase of development, focusing on cataloguing and registering systematic reviews from one sub-field of Education: language education. This phase of the project has been generously supported by a John Fell Fund award and will serve as proof of concept for future funding bids to support the expansion of IDESR to include systematic reviews from all sub-fields of Education.
Ethical and useful research should build on what is already known, and systematic reviews of existing research are key in this regard. Systematic reviews aim to locate, critically assess, and synthesise the totality of reliable evidence relevant to a particular topic or question. When looking to inform educational policy, decision makers frequently rely on systematic reviews for an up-to-date, quality-assessed overview of available evidence. Teachers, teacher educators and publishers also look to systematic reviews for digestible, evidence-based guidance, seeking to underpin pedagogy and materials development with substantiated insights and approaches, a practice which is ultimately beneficial to their learners and so to society at large. Systematic reviews are thus a crucial element of the knowledge base on which the field of Education is founded. Despite their importance, however, locating systematic reviews in Education can be difficult and time-consuming. A dedicated database of Education systematic reviews is needed to simplify the location of Systematic Reviews in Education.
In addition to a database of completed systematic reviews, Education lacks a platform through which to register ongoing and planned systematic reviews. Prospective registration provides transparency by providing a permanent record of planned systematic reviews, irrespective of whether they are eventually published, and helps to detect and address publication bias (with underreporting of 'unflattering' or 'unexciting reviews'). Prospective registration helps researchers and other users to assess the published versions of systematic reviews against their protocols, and helping them to identify instances of poor practice, e.g., outcome switching. Prospective registration also helps those commissioning or planning systematic reviews to identify whether reviews on their chosen topic are already in preparation, thus helping to avoid duplication of effort, and facilitating collaboration. While registries for planned systematic reviews exist in other disciplines (e.g., PROSPERO for health-related reviews), until IDESR there has been no such registry in Education.
IDESR is coordinated by Dr Hamish Chalmers of the Department of Education, University of Oxford. Initial set up of IDESR was supported by Dr Jessica Briggs Baffoe-Djan and Jessica Brown (IDESR Research Assistant), and the IDESR advisory group as listed below.

The IDESR website was built and is maintained by Gordon Dooley and Metaxis Software Design .
If you have any questions or feedback about IDESR, please contact [email protected]
Below is a list of your records. Records that have been submitted are locked until an administrator has approved or rejected them. Records that have been published may not be edited but may be updated.
Title | Status | Action |
---|
For any questions about this site please contact Dr Hamish Chalmers [email protected]
IDESR disclaimer
Disclaimer To the extent permitted by law, IDESR provides this website and its contents on an "as is" basis and makes no representation or warranty of any kind regarding this website or any information, content, products or services on it. IDESR does not represent or warrant that the information accessible via this website is accurate, complete or current. In no circumstances, to the extent permitted by law, shall IDESR or any of its officers or employees be liable for any loss, additional costs or damage (howsoever arising) suffered as a result of any use of this website or its contents.
Links to third party information This website includes links to third party web sites. These links are used to provide further information and are not intended to signify that IDESR endorses such websites and/or their content. IDESR takes no responsibility for any loss or damage suffered as a result of using the information published on any of the pages of the linked web sites.
Published Protocols This website publishes protocol registrations submitted by users of the IDESR platform. IDESR takes no responsibility for the information contained in those protocols and consequently, IDESR does not and cannot guarantee the accuracy of such information.
Privacy poicy
IDESR is committed to protecting your privacy, as an IDESR account holder and/or user of our website. This privacy policy explains how we collect and use personal data we collect from you, or that you provide.
IDESR is housed at The Department of Education, University of Oxford. The key personnel at IDESR are named on the Team page of the IDESR website. The IDESR website was built and is maintained by Metaxis Software Design , and is stored on servers owned by Metaxis.
The IDESR blog, linked to from this website but not part of it, is housed on Wordpress.com and is covered by Wordpress's privacy policy .
The information we collect
Idesr account holders.
When users set up an IDESR account for the purpose of registering protocols of planned and ongoing systematic reviews, we ask for first and last names, email address, institutional affiliation, and geographical location. When an account holder submits a protocol registration form, we ask for the name, institutional affiliation, email address and physical mailing address of the main contact/corresponding author. We also ask for the names, institutional affiliations, and email addresses of any additional authors.
All IDESR users
We use Google Analytics to provide information about site usage. Information such as your IP address and your usage of our website is automatically collected each time you visit.
Our websites use cookies – small text files that are placed on your machine to help the websites provide a better user experience. In general, cookies are used to retain user preferences, store information for things like your protocol registrations, and provide anonymised tracking data to third party applications like Google Analytics. As a rule, cookies will make your browsing experience better. However, you may prefer to disable cookies on this site and on others. The most effective way to do this is to disable cookies in your browser. We suggest consulting the Help section of your browser or taking a look at the About Cookies website which offers guidance for all modern browsers.
How do we use your data?
We use the lawful bases of consent, contract and legitimate interests to process your personal data.
We may use your personal information to:
- Administer and communicate with you for any reason related to your IDESR account
- Send you email reminders when updates to any protocols you have registered are due
- Notify you of any changes to our services
- Respond to any enquiries made by you
We may use personal information to:
- Understand how the IDESR website is used by its visitors, via information collected by third party services like Google Analytics
- Maintain the safety and security of our websites and other online platforms, and to prevent fraud
Sharing your information
We may share your personal information with:
- Any member of IDESR operational staff. The type of personal data shared will be relevant to the purpose for which the data is used. For example, to communicate with you via email to tell you the outcome of a protocol registration application.
- Providers of IT services for administration of our websites and management of our internal systems.
- Analytics and search engine providers such as Google Analytics, to help us to improve our website and your user experience.
- Regulators, financial organisations, fraud detection and crime prevention agencies. This will be in order to comply with any legal obligations or mandatory reporting requirements.
In addition, accepted protocol registrations will be published on the IDESR website and will include the information provided by the IDESR account holder about the main contact/corresponding author and any additional authors. Published protocol registrations are freely available to view and download by any user of the IDESR website.
In addition, anonymised analytics information (for example geographical locations of users of the site, pages views, length of time on the site, etc.) may be used to provide usage reports for IDESR, its funders, and in any applications for funding support in the future. These anonymised data may also be used in scholarly publications about the IDESR project.
Where is your personal data is stored?
IDESR is based in the UK and is currently bound by regulations applying to members of the EEA (European Economic Area). We may transfer, store and process the data we collect from you at a destination outside of the EEA. Where it is necessary to do so, we will take all steps reasonably necessary to ensure that appropriate safeguards are in place to treat your data securely and in accordance with this policy. After 31 December 2020 we will review our privacy policy in the light of any changes to the UK's relationship with the EEA.
Information you provide is stored on our in-house servers and with third-party cloud providers. This policy covers processing once your information has been received by IDESR and does not cover any processing which may be carried out by your internet service provider (ISP). Any transmission of data via the internet is not completely secure and at your own risk. We recommend that you keep any passwords issued for access to our website and your personal information confidential.
Retention of your information
Unless we inform you otherwise, we will retain your personal information as follows:
- For as long as is required to provide services you have requested as an IDESR account holder.
- For as long as is necessary for our own legitimate interests (such as investigating misuse of the IDESR platform)
- For retention periods in line with legal and regulatory requirements or guidance.
- Accepted protocol registrations, including the personal data contained therein, are published permanently.
Your rights
You have several rights regarding the collection and use of your personal data. These include, but are not limited to a right to:
- Be informed about the collection and processing of your personal data
- Access to your personal information and how we process it
- Object to the processing of your personal information
- Rectification of any inaccurate personal information or have incomplete information completed
- Erasure of personal information if we no longer have a lawful basis for retaining or processing it
- Data portability – obtain and re-use your data in a commonly used, machine-readable format
- Withdraw consent
- Object to automated decision making and profiling
These do not apply in all circumstances. If you wish to use them, we will explain whether they are applicable in that instance.
You have the right to lodge a complaint with the Information Commissioner's Office (ICO) . It is usually expected that you would raise your concern with us, in the first instance.
Other websites
This privacy policy only covers our websites. Any other websites which are linked to from our websites have their own privacy policies which may differ from ours. We do not accept any responsibility or liability for these policies.
Updates to this Privacy Policy
We may change this privacy policy from time to time in response to changes in the law and/or how we use your personal information. We recommend that you review the contents of this privacy policy regularly. Your continued use of the websites after changes are posted constitutes your acceptance of this agreement as modified.
If you have any questions, comments, requests or complaints about this privacy policy or how we treat your personal data, then please contact the editor of IDESR Dr Hamish Chalmers, in the first instance:
Department of Education University of Oxford 15 Norham Gardens Oxford OX2 6PY
+44 (0)1865 284091
Subject access requests are free. We will respond to your request within one month.
If you believe that we have not met our obligations, you are entitled to contact the Information Commissioner's Office (ICO) .
This site uses cookies for navigation purposes only. Please see our Privacy policy for more details.
- Review article
- Open access
- Published: 28 October 2019
Systematic review of research on artificial intelligence applications in higher education – where are the educators?
- Olaf Zawacki-Richter ORCID: orcid.org/0000-0003-1482-8303 1 ,
- Victoria I. Marín ORCID: orcid.org/0000-0002-4673-6190 1 ,
- Melissa Bond ORCID: orcid.org/0000-0002-8267-031X 1 &
- Franziska Gouverneur 1
International Journal of Educational Technology in Higher Education volume 16 , Article number: 39 ( 2019 ) Cite this article
322k Accesses
912 Citations
244 Altmetric
Metrics details
According to various international reports, Artificial Intelligence in Education (AIEd) is one of the currently emerging fields in educational technology. Whilst it has been around for about 30 years, it is still unclear for educators how to make pedagogical advantage of it on a broader scale, and how it can actually impact meaningfully on teaching and learning in higher education. This paper seeks to provide an overview of research on AI applications in higher education through a systematic review. Out of 2656 initially identified publications for the period between 2007 and 2018, 146 articles were included for final synthesis, according to explicit inclusion and exclusion criteria. The descriptive results show that most of the disciplines involved in AIEd papers come from Computer Science and STEM, and that quantitative methods were the most frequently used in empirical studies. The synthesis of results presents four areas of AIEd applications in academic support services, and institutional and administrative services: 1. profiling and prediction, 2. assessment and evaluation, 3. adaptive systems and personalisation, and 4. intelligent tutoring systems. The conclusions reflect on the almost lack of critical reflection of challenges and risks of AIEd, the weak connection to theoretical pedagogical perspectives, and the need for further exploration of ethical and educational approaches in the application of AIEd in higher education.
Introduction
Artificial intelligence (AI) applications in education are on the rise and have received a lot of attention in the last couple of years. AI and adaptive learning technologies are prominently featured as important developments in educational technology in the 2018 Horizon report (Educause, 2018 ), with a time to adoption of 2 or 3 years. According to the report, experts anticipate AI in education to grow by 43% in the period 2018–2022, although the Horizon Report 2019 Higher Education Edition (Educause, 2019 ) predicts that AI applications related to teaching and learning are projected to grow even more significantly than this. Contact North, a major Canadian non-profit online learning society, concludes that “there is little doubt that the [AI] technology is inexorably linked to the future of higher education” (Contact North, 2018 , p. 5). With heavy investments by private companies such as Google, which acquired European AI start-up Deep Mind for $400 million, and also non-profit public-private partnerships such as the German Research Centre for Artificial Intelligence Footnote 1 (DFKI), it is very likely that this wave of interest will soon have a significant impact on higher education institutions (Popenici & Kerr, 2017 ). The Technical University of Eindhoven in the Netherlands, for example, recently announced that they will launch an Artificial Intelligence Systems Institute with 50 new professorships for education and research in AI. Footnote 2
The application of AI in education (AIEd) has been the subject of research for about 30 years. The International AIEd Society (IAIED) was launched in 1997, and publishes the International Journal of AI in Education (IJAIED), with the 20th annual AIEd conference being organised this year. However, on a broader scale, educators have just started to explore the potential pedagogical opportunities that AI applications afford for supporting learners during the student life cycle.
Despite the enormous opportunities that AI might afford to support teaching and learning, new ethical implications and risks come in with the development of AI applications in higher education. For example, in times of budget cuts, it might be tempting for administrators to replace teaching by profitable automated AI solutions. Faculty members, teaching assistants, student counsellors, and administrative staff may fear that intelligent tutors, expert systems and chat bots will take their jobs. AI has the potential to advance the capabilities of learning analytics, but on the other hand, such systems require huge amounts of data, including confidential information about students and faculty, which raises serious issues of privacy and data protection. Some institutions have recently been established, such as the Institute for Ethical AI in Education Footnote 3 in the UK, to produce a framework for ethical governance for AI in education, and the Analysis & Policy Observatory published a discussion paper in April 2019 to develop an AI ethics framework for Australia. Footnote 4
Russel and Norvig ( 2010 ) remind us in their leading textbook on artificial intelligence, “All AI researchers should be concerned with the ethical implications of their work” (p. 1020). Thus, we would like to explore what kind of fresh ethical implications and risks are reflected by the authors in the field of AI enhanced education. The aim of this article is to provide an overview for educators of research on AI applications in higher education. Given the dynamic development in recent years, and the growing interest of educators in this field, a review of the literature on AI in higher education is warranted.
Specifically, this paper addresses the following research questions in three areas, by means of a systematic review (see Gough, Oliver, & Thomas, 2017 ; Petticrew & Roberts, 2006 ):
How have publications on AI in higher education developed over time, in which journals are they published, and where are they coming from in terms of geographical distribution and the author’s disciplinary affiliations?
How is AI in education conceptualised and what kind of ethical implications, challenges and risks are considered?
What is the nature and scope of AI applications in the context of higher education?
The field AI originates from computer science and engineering, but it is strongly influenced by other disciplines such as philosophy, cognitive science, neuroscience, and economics. Given the interdisciplinary nature of the field, there is little agreement among AI researchers on a common definition and understanding of AI – and intelligence in general (see Tegmark, 2018 ). With regard to the introduction of AI-based tools and services in higher education, Hinojo-Lucena, Aznar-Díaz, Cáceres-Reche, and Romero-Rodríguez ( 2019 ) note that “this technology [AI] is already being introduced in the field of higher education, although many teachers are unaware of its scope and, above all, of what it consists of” (p. 1). For the purpose of our analysis of artificial intelligence in higher education, it is desirable to clarify terminology. Thus, in the next section, we explore definitions of AI in education, and the elements and methods that AI applications might entail in higher education, before we proceed with the systematic review of the literature.
AI in education (AIEd)
The birth of AI goes back to the 1950s when John McCarthy organised a two-month workshop at Dartmouth College in the USA. In the workshop proposal, McCarthy used the term artificial intelligence for the first time in 1956 (Russel & Norvig, 2010 , p. 17):
The study [of artificial intelligence] is to proceed on the basis of the conjecture that every aspect of learning or any other feature of intelligence can in principle be so precisely described that a machine can be made to simulate it. An attempt will be made to find how to make machines use language, form abstractions and concepts, solve kinds of problems now reserved for humans, and improve themselves.
Baker and Smith ( 2019 ) provide a broad definition of AI: “Computers which perform cognitive tasks, usually associated with human minds, particularly learning and problem-solving” (p. 10). They explain that AI does not describe a single technology. It is an umbrella term to describe a range of technologies and methods, such as machine learning, natural language processing, data mining, neural networks or an algorithm.
AI and machine learning are often mentioned in the same breath. Machine learning is a method of AI for supervised and unsupervised classification and profiling, for example to predict the likelihood of a student to drop out from a course or being admitted to a program, or to identify topics in written assignments. Popenici and Kerr ( 2017 ) define machine learning “as a subfield of artificial intelligence that includes software able to recognise patterns, make predictions, and apply newly discovered patterns to situations that were not included or covered by their initial design” (p. 2).
The concept of rational agents is central to AI: “An agent is anything that can be viewed as perceiving its environment through sensors and acting upon that environment through actuators” (Russel & Norvig, 2010 , p. 34). The vacuum-cleaner robot is a very simple form of an intelligent agent, but things become very complex and open-ended when we think about an automated taxi.
Experts in the field distinguish between weak and strong AI (see Russel & Norvig, 2010 , p. 1020) or narrow and general AI (see Baker & Smith, 2019 , p. 10). A philosophical question remains whether machines will be able to actually think or even develop consciousness in the future, rather than just simulating thinking and showing rational behaviour. It is unlikely that such strong or general AI will exist in the near future. We are therefore dealing here with GOFAI (“ good old-fashioned AI ”, a term coined by the philosopher John Haugeland, 1985 ) in higher education – in the sense of agents and information systems that act as if they were intelligent.
Given this understanding of AI, what are potential areas of AI applications in education, and higher education in particular? Luckin, Holmes, Griffiths, and Forcier ( 2016 ) describe three categories of AI software applications in education that are available today: a) personal tutors, b) intelligent support for collaborative learning, and c) intelligent virtual reality.
Intelligent tutoring systems (ITS) can be used to simulate one-to-one personal tutoring. Based on learner models, algorithms and neural networks, they can make decisions about the learning path of an individual student and the content to select, provide cognitive scaffolding and help, to engage the student in dialogue. ITS have enormous potential, especially in large-scale distance teaching institutions, which run modules with thousands of students, where human one-to-one tutoring is impossible. A vast array of research shows that learning is a social exercise; interaction and collaboration are at the heart of the learning process (see for example Jonassen, Davidson, Collins, Campbell, & Haag, 1995 ). However, online collaboration has to be facilitated and moderated (Salmon, 2000 ). AIEd can contribute to collaborative learning by supporting adaptive group formation based on learner models, by facilitating online group interaction or by summarising discussions that can be used by a human tutor to guide students towards the aims and objectives of a course. Finally, also drawing on ITS, intelligent virtual reality (IVR) is used to engage and guide students in authentic virtual reality and game-based learning environments. Virtual agents can act as teachers, facilitators or students’ peers, for example, in virtual or remote labs (Perez et al., 2017 ).
With the advancement of AIEd and the availability of (big) student data and learning analytics, Luckin et al. ( 2016 ) claim a “[r] enaissance in assessment” (p. 35). AI can provide just-in-time feedback and assessment. Rather than stop-and-test, AIEd can be built into learning activities for an ongoing analysis of student achievement. Algorithms have been used to predict the probability of a student failing an assignment or dropping out of a course with high levels of accuracy (e.g. Bahadır, 2016 ).
In their recent report, Baker and Smith ( 2019 ) approach educational AI tools from three different perspectives; a) learner-facing, b) teacher-facing, and c) system-facing AIEd. Learner-facing AI tools are software that students use to learn a subject matter, i.e. adaptive or personalised learning management systems or ITS. Teacher-facing systems are used to support the teacher and reduce his or her workload by automating tasks such as administration, assessment, feedback and plagiarism detection. AIEd tools also provide insight into the learning progress of students so that the teacher can proactively offer support and guidance where needed. System-facing AIEd are tools that provide information for administrators and managers on the institutional level, for example to monitor attrition patterns across faculties or colleges.
In the context of higher education, we use the concept of the student life-cycle (see Reid, 1995 ) as a framework to describe the various AI based services on the broader institutional and administrative level, as well as for supporting the academic teaching and learning process in the narrower sense.
The purpose of a systematic review is to answer specific questions, based on an explicit, systematic and replicable search strategy, with inclusion and exclusion criteria identifying studies to be included or excluded (Gough, Oliver & Thomas, 2017 ). Data is then coded and extracted from included studies, in order to synthesise findings and to shine light on their application in practice, as well as on gaps or contradictions. This contribution maps 146 articles on the topic of artificial intelligence in higher education.
Search strategy
The initial search string (see Table 1 ) and criteria (see Table 2 ) for this systematic review included peer-reviewed articles in English, reporting on artificial intelligence within education at any level, and indexed in three international databases; EBSCO Education Source, Web of Science and Scopus (covering titles, abstracts, and keywords). Whilst there are concerns about peer-review processes within the scientific community (e.g., Smith, 2006 ), articles in this review were limited to those published in peer-reviewed journals, due to their general trustworthiness in academia and the rigorous review processes undertaken (Nicholas et al., 2015 ). The search was undertaken in November 2018, with an initial 2656 records identified.
After duplicates were removed, it was decided to limit articles to those published during or after 2007, as this was the year that iPhone’s Siri was introduced; an algorithm-based personal assistant, started as an artificial intelligence project funded by the US Defense Advanced Research Projects Agency (DARPA) in 2001, turned into a company that was acquired by Apple Inc. It was also decided that the corpus would be limited to articles discussing applications of artificial intelligence in higher education only.
Screening and inter-rater reliability
The screening of 1549 titles and abstracts was carried out by a team of three coders and at this first screening stage, there was a requirement of sensitivity rather than specificity, i.e. papers were included rather than excluded. In order to reach consensus, the reasons for inclusion and exclusion for the first 80 articles were discussed at regular meetings. Twenty articles were randomly selected to evaluate the coding decisions of the three coders (A, B and C) to determine inter-rater reliability using Cohen’s kappa (κ) (Cohen, 1960 ), which is a coefficient for the degree of consistency among raters, based on the number of codes in the coding scheme (Neumann, 2007 , p. 326). Kappa values of .40–.60 are characterised as fair, .60 to .75 as good, and over .75 as excellent (Bakeman & Gottman, 1997 ; Fleiss, 1981 ). Coding consistency for inclusion or exclusion of articles between rater A and B was κ = .79, between rater A and C it was κ = .89, and between rater B and C it was κ = .69 (median = .79). Therefore, inter-rater reliability can be considered as excellent for the coding of inclusion and exclusion criteria.
After initial screening, 332 potential articles remained for screening on full text (see Fig. 1 ). However, 41 articles could not be retrieved, either through the library order scheme or by contacting authors. Therefore, 291 articles were retrieved, screened and coded, and following the exclusion of 149 papers, 146 articles remained for synthesis. Footnote 5
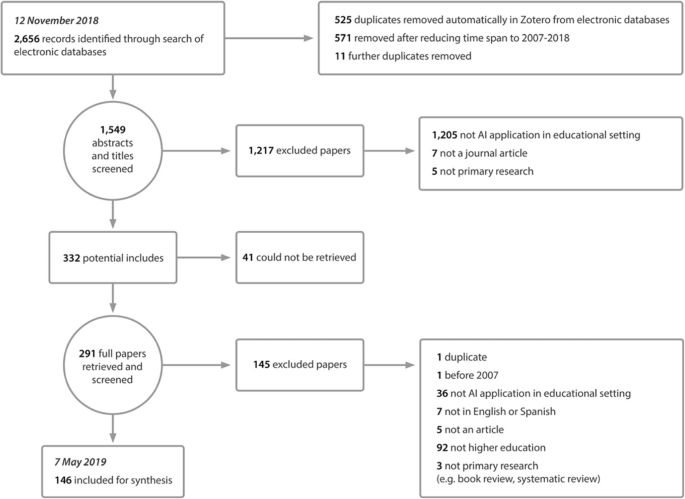
PRISMA diagram (slightly modified after Brunton & Thomas, 2012 , p. 86; Moher, Liberati, Tetzlaff, & Altman, 2009 , p. 8)
Coding, data extraction and analysis
In order to extract the data, all articles were uploaded into systematic review software EPPI Reviewer Footnote 6 and a coding system was developed. Codes included article information (year of publication, journal name, countries of authorship, discipline of first author), study design and execution (empirical or descriptive, educational setting) and how artificial intelligence was used (applications in the student life cycle, specific applications and methods). Articles were also coded on whether challenges and benefits of AI were present, and whether AI was defined. Descriptive data analysis was carried out with the statistics software R using the tidyr package (Wickham & Grolemund, 2016 ).
Limitations
Whilst this systematic review was undertaken as rigorously as possible, each review is limited by its search strategy. Although the three educational research databases chosen are large and international in scope, by applying the criteria of peer-reviewed articles published only in English or Spanish, research published on AI in other languages were not included in this review. This also applies to research in conference proceedings, book chapters or grey literature, or those articles not published in journals that are indexed in the three databases searched. In addition, although Spanish peer-reviewed articles were added according to inclusion criteria, no specific search string in the language was included, which narrows down the possibility of including Spanish papers that were not indexed with the chosen keywords. Future research could consider using a larger number of databases, publication types and publication languages, in order to widen the scope of the review. However, serious consideration would then need to be given to project resources and the manageability of the review (see Authors, in press).
Journals, authorship patterns and methods
Articles per year.
There was a noticeable increase in the papers published from 2007 onwards. The number of included articles grew from six in 2007 to 23 in 2018 (see Fig. 2 ).
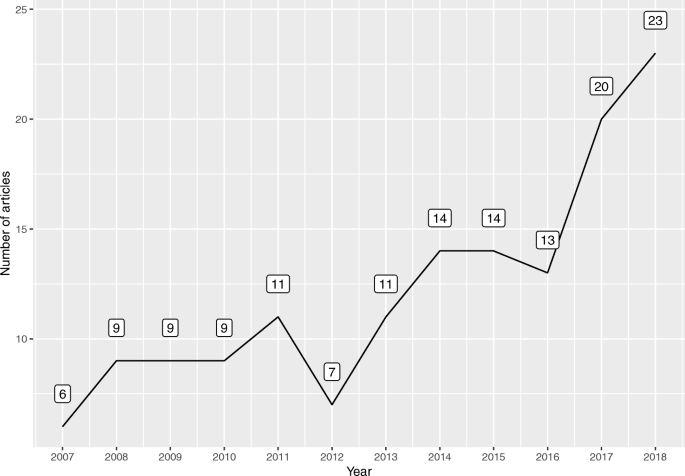
Number of included articles per year ( n = 146)
The papers included in the sample were published in 104 different journals. The greatest number of articles were published in the International Journal of Artificial Intelligence in Education ( n = 11) , followed by Computers & Education ( n = 8) , and the International Journal of Emerging Technologies in Learning ( n = 5) . Table 3 lists 19 journals that published at least two articles on AI in higher education from 2007 to 2018.
For the geographical distribution analysis of articles, the country of origin of the first author was taken into consideration ( n = 38 countries). Table 4 shows 19 countries that contributed at least two papers, and it reveals that 50% of all articles come from only four countries: USA, China, Taiwan, and Turkey.
Author affiliations
Again, the affiliation of the first author was taken into consideration (see Table 5 ). Researchers working in departments of Computer Science contributed by far the greatest number of papers ( n = 61) followed by Science, Technology, Engineering and Mathematics (STEM) departments ( n = 29). Only nine first authors came from an Education department, some reported dual affiliation with Education and Computer Science ( n = 2), Education and Psychology ( n = 1), or Education and STEM ( n = 1).
Thus, 13 papers (8.9%) were written by first authors with an Education background. It is noticeable that three of them were contributed by researchers from the Teachers College at Columbia University, New York, USA (Baker, 2016 ; Paquette, Lebeau, Beaulieu, & Mayers, 2015 ; Perin & Lauterbach, 2018 ) – and they were all published in the same journal, i.e. the International Journal of Artificial Intelligence in Education .
Thirty studies (20.5%) were coded as being theoretical or descriptive in nature. The vast majority of studies (73.3%) applied quantitative methods, whilst only one (0.7%) was qualitative in nature and eight (5.5%) followed a mixed-methods approach. The purpose of the qualitative study, involving interviews with ESL students, was to explore the nature of written feedback coming from an automated essay scoring system compared to a human teacher (Dikli, 2010 ). In many cases, authors employed quasi-experimental methods, being an intentional sample divided into the experimental group, where an AI application (e.g. an intelligent tutoring system) was applied, and the control group without the intervention, followed by pre- and posttest (e.g. Adamson, Dyke, Jang, & Rosé, 2014 ).
Understanding of AI and critical reflection of challenges and risks
There are many different types and levels of AI mentioned in the articles, however only five out of 146 included articles (3.4%) provide an explicit definition of the term “Artificial Intelligence”. The main characteristics of AI, described in all five studies, are the parallels between the human brain and artificial intelligence. The authors conceptualise AI as intelligent computer systems or intelligent agents with human features, such as the ability to memorise knowledge, to perceive and manipulate their environment in a similar way as humans, and to understand human natural language (see Huang, 2018 ; Lodhi, Mishra, Jain, & Bajaj, 2018 ; Welham, 2008 ). Dodigovic ( 2007 ) defines AI in her article as follows (p. 100):
Artificial intelligence (AI) is a term referring to machines which emulate the behaviour of intelligent beings [ … ] AI is an interdisciplinary area of knowledge and research, whose aim is to understand how the human mind works and how to apply the same principles in technology design. In language learning and teaching tasks, AI can be used to emulate the behaviour of a teacher or a learner [ … ] . (p. 100)
Dodigovic is the only author who gives a definition of AI, and comes from an Arts, Humanities and Social Science department, taking into account aspects of AI and intelligent tutors in second language learning.
A stunningly low number of authors, only two out of 146 articles (1.4%), critically reflect upon ethical implications, challenges and risks of applying AI in education. Li ( 2007 ) deals with privacy concerns in his article about intelligent agent supported online learning:
Privacy is also an important concern in applying agent-based personalised education. As discussed above, agents can autonomously learn many of students’ personal information, like learning style and learning capability. In fact, personal information is private. Many students do not want others to know their private information, such as learning styles and/or capabilities. Students might show concern over possible discrimination from instructors in reference to learning performance due to special learning needs. Therefore, the privacy issue must be resolved before applying agent-based personalised teaching and learning technologies. (p. 327)
Another challenge of applying AI is mentioned by Welham ( 2008 , p. 295) concerning the costs and time involved in developing and introducing AI-based methods that many public educational institutions cannot afford.
AI applications in higher education
As mentioned before, we used the concept of the student life-cycle (see Reid, 1995 ) as a framework to describe the various AI based services at the institutional and administrative level (e.g. admission, counselling, library services), as well as at the academic support level for teaching and learning (e.g. assessment, feedback, tutoring). Ninety-two studies (63.0%) were coded as relating to academic support services and 48 (32.8%) as administrative and institutional services; six studies (4.1%) covered both levels. The majority of studies addressed undergraduate students ( n = 91, 62.3%) compared to 11 (7.5%) focussing on postgraduate students, and another 44 (30.1%) that did not specify the study level.
The iterative coding process led to the following four areas of AI applications with 17 sub-categories, covered in the publications: a) adaptive systems and personalisation, b) assessment and evaluation, c) profiling and prediction, and d) intelligent tutoring systems. Some studies addressed AI applications in more than one area (see Table 6 ).
The nature and scope of the various AI applications in higher education will be described along the lines of these four application categories in the following synthesis.
Profiling and prediction
The basis for many AI applications are learner models or profiles that allow prediction, for example of the likelihood of a student dropping out of a course or being admitted to a programme, in order to offer timely support or to provide feedback and guidance in content related matters throughout the learning process. Classification, modelling and prediction are an essential part of educational data mining (Phani Krishna, Mani Kumar, & Aruna Sri, 2018 ).
Most of the articles (55.2%, n = 32) address issues related to the institutional and administrative level, many (36.2%, n = 21) are related to academic teaching and learning at the course level, and five (8.6%) are concerned with both levels. Articles dealing with profiling and prediction were classified into three sub-categories; admission decisions and course scheduling ( n = 7), drop-out and retention ( n = 23), and student models and academic achievement ( n = 27). One study that does not fall into any of these categories is the study by Ge and Xie ( 2015 ), which is concerned with forecasting the costs of a Chinese university to support management decisions based on an artificial neural network.
All of the 58 studies in this area applied machine learning methods, to recognise and classify patterns, and to model student profiles to make predictions. Thus, they are all quantitative in nature. Many studies applied several machine learning algorithms (e.g. ANN, SVM, RF, NB; see Table 7 ) Footnote 7 and compared their overall prediction accuracy with conventional logistic regression. Table 7 shows that machine learning methods outperformed logistic regression in all studies in terms of their classification accuracy in percent. To evaluate the performance of classifiers, the F1-score can also be used, which takes into account the number of positive instances correctly classified as positive, the number of negative instances incorrectly classified as positive, and the number of positive instances incorrectly classified as negative (Umer et al., 2017 ; for a brief overview of measures of diagnostic accuracy, see Šimundić, 2009 ). The F1-score ranges between 0 and 1 with its best value at 1 (perfect precision and recall). Yoo and Kim ( 2014 ) reported high F1-scores of 0.848, 0.911, and 0.914 for J48, NB, and SVM, in a study to predict student’s group project performance from online discussion participation.
Admission decisions and course scheduling
Chen and Do ( 2014 ) point out that “the accurate prediction of students’ academic performance is of importance for making admission decisions as well as providing better educational services” (p. 18). Four studies aimed to predict whether or not a prospective student would be admitted to university. For example, Acikkar and Akay ( 2009 ) selected candidates for a School of Physical Education and Sports in Turkey based on a physical ability test, their scores in the National Selection and Placement Examination, and their graduation grade point average (GPA). They used the support vector machine (SVM) technique to classify the students and where able to predict admission decisions on a level of accuracy of 97.17% in 2006 and 90.51% in 2007. SVM was also applied by Andris, Cowen, and Wittenbach ( 2013 ) to find spatial patterns that might favour prospective college students from certain geographic regions in the USA. Feng, Zhou, and Liu ( 2011 ) analysed enrolment data from 25 Chinese provinces as the training data to predict registration rates in other provinces using an artificial neural network (ANN) model. Machine learning methods and ANN are also used to predict student course selection behaviour to support course planning. Kardan, Sadeghi, Ghidary, and Sani ( 2013 ) investigated factors influencing student course selection, such as course and instructor characteristics, workload, mode of delivery and examination time, to develop a model to predict course selection with an ANN in two Computer Engineering and Information Technology Masters programs. In another paper from the same author team, a decision support system for course offerings was proposed (Kardan & Sadeghi, 2013 ). Overall, the research shows that admission decisions can be predicted at high levels of accuracy, so that an AI solution could relieves the administrative staff and allows them to focus on the more difficult cases.
Drop-out and retention
Studies pertaining to drop-out and retention are intended to develop early warning systems to detect at-risk students in their first year (e.g., Alkhasawneh & Hargraves, 2014 ; Aluko, Adenuga, Kukoyi, Soyingbe, & Oyedeji, 2016 ; Hoffait & Schyns, 2017 ; Howard, Meehan, & Parnell, 2018 ) or to predict the attrition of undergraduate students in general (e.g., Oztekin, 2016 ; Raju & Schumacker, 2015 ). Delen ( 2011 ) used institutional data from 25,224 students enrolled as Freshmen in an American university over 8 years. In this study, three classification techniques were used to predict dropout: ANN, decision trees (DT) and logistic regression. The data contained variables related to students’ demographic, academic, and financial characteristics (e.g. age, sex, ethnicity, GPA, TOEFL score, financial aid, student loan, etc.). Based on a 10-fold cross validation, Delen ( 2011 ) found that the ANN model worked best with an accuracy rate of 81.19% (see Table 7 ) and he concluded that the most important predictors of student drop-out are related to the student’s past and present academic achievement, and whether they receive financial support. Sultana, Khan, and Abbas ( 2017 , p. 107) discussed the impact of cognitive and non-cognitive features of students for predicting academic performance of undergraduate engineering students. In contrast to many other studies, they focused on non-cognitive variables to improve prediction accuracy, i.e. time management, self-concept, self-appraisal, leadership, and community support.
Student models and academic achievement
Many more studies are concerned with profiling students and modelling learning behaviour to predict their academic achievements at the course level. Hussain et al. ( 2018 ) applied several machine learning algorithms to analyse student behavioural data from the virtual learning environment at the Open University UK, in order to predict student engagement, which is of particular importance at a large scale distance teaching university, where it is not possible to engage the majority of students in face-to-face sessions. The authors aim to develop an intelligent predictive system that enables instructors to automatically identify low-engaged students and then to make an intervention. Spikol, Ruffaldi, Dabisias, and Cukurova ( 2018 ) used face and hand tracking in workshops with engineering students to estimate success in project-based learning. They concluded that results generated from multimodal data can be used to inform teachers about key features of project-based learning activities. Blikstein et al. ( 2014 ) investigated patterns of how undergraduate students learn computer programming, based on over 150,000 code transcripts that the students created in software development projects. They found that their model, based on the process of programming, had better predictive power than the midterm grades. Another example is the study of Babić ( 2017 ), who developed a model to predict student academic motivation based on their behaviour in an online learning environment.
The research on student models is an important foundation for the design of intelligent tutoring systems and adaptive learning environments.
- Intelligent tutoring systems
All of the studies investigating intelligent tutoring systems (ITS) ( n = 29) are only concerned with the teaching and learning level, except for one that is contextualised at the institutional and administrative level. The latter presents StuA , an interactive and intelligent student assistant that helps newcomers in a college by answering queries related to faculty members, examinations, extra curriculum activities, library services, etc. (Lodhi et al., 2018 ).
The most common terms for referring to ITS described in the studies are intelligent (online) tutors or intelligent tutoring systems (e.g., in Dodigovic, 2007 ; Miwa, Terai, Kanzaki, & Nakaike, 2014 ), although they are also identified often as intelligent (software) agents (e.g., Schiaffino, Garcia, & Amandi, 2008 ), or intelligent assistants (e.g., in Casamayor, Amandi, & Campo, 2009 ; Jeschike, Jeschke, Pfeiffer, Reinhard, & Richter, 2007 ). According to Welham ( 2008 ), the first ITS reported was the SCHOLAR system, launched in 1970, which allowed the reciprocal exchange of questions between teacher and student, but not holding a continuous conversation.
Huang and Chen ( 2016 , p. 341) describe the different models that are usually integrated in ITS: the student model (e.g. information about the student’s knowledge level, cognitive ability, learning motivation, learning styles), the teacher model (e.g. analysis of the current state of students, select teaching strategies and methods, provide help and guidance), the domain model (knowledge representation of both students and teachers) and the diagnosis model (evaluation of errors and defects based on domain model).
The implementation and validation of the ITS presented in the studies usually took place over short-term periods (a course or a semester) and no longitudinal studies were identified, except for the study by Jackson and Cossitt ( 2015 ). On the other hand, most of the studies showed (sometimes slightly) positive / satisfactory preliminary results regarding the performance of the ITS, but they did not take into account the novelty effect that a new technological development could have in an educational context. One study presented negative results regarding the type of support that the ITS provided (Adamson et al., 2014 ), which could have been more useful if it was more adjusted to the type of (in this case, more advanced) learners.
Overall, more research is needed on the effectiveness of ITS. The last meta-analysis of 39 ITS studies was published over 5 years ago: Steenbergen-Hu and Cooper ( 2014 ) found that ITS had a moderate effect of students’ learning, and that ITS were less effective that human tutoring, but ITS outperformed all other instruction methods (such as traditional classroom instruction, reading printed or digital text, or homework assignments).
The studies addressing various ITS functions were classified as follows: teaching course content ( n = 12), diagnosing strengths or gaps in students’ knowledge and providing automated feedback ( n = 7), curating learning materials based on students’ needs ( n = 3), and facilitating collaboration between learners ( n = 2).
Teaching course content
Most of the studies ( n = 4) within this group focused on teaching Computer Science content (Dobre, 2014 ; Hooshyar, Ahmad, Yousefi, Yusop, & Horng, 2015 ; Howard, Jordan, di Eugenio, & Katz, 2017 ; Shen & Yang, 2011 ). Other studies included ITS teaching content for Mathematics (Miwa et al., 2014 ), Business Statistics and Accounting (Jackson & Cossitt, 2015 ; Palocsay & Stevens, 2008 ), Medicine (Payne et al., 2009 ) and writing and reading comprehension strategies for undergraduate Psychology students (Ray & Belden, 2007 ; Weston-Sementelli, Allen, & McNamara, 2018 ). Overall, these ITS focused on providing teaching content to students and, at the same time, supporting them by giving adaptive feedback and hints to solve questions related to the content, as well as detecting students’ difficulties/errors when working with the content or the exercises. This is made possible by monitoring students’ actions with the ITS.
In the study by Crown, Fuentes, Jones, Nambiar, and Crown ( 2011 ), a combination of teaching content through dialogue with a chatbot, that at the same time learns from this conversation - defined as a text-based conversational agent -, is described, which moves towards a more active, reflective and thinking student-centred learning approach. Duffy and Azevedo ( 2015 ) present an ITS called MetaTutor, which is designed to teach students about the human circulatory system, but it also puts emphasis on supporting students’ self-regulatory processes assisted by the features included in the MetaTutor system (a timer, a toolbar to interact with different learning strategies, and learning goals, amongst others).

Diagnosing strengths or gaps in student knowledge, and providing automated feedback
In most of the studies ( n = 4) of this group, ITS are presented as a rather one-way communication from computer to student, concerning the gaps in students’ knowledge and the provision of feedback. Three examples in the field of STEM have been found: two of them where the virtual assistance is presented as a feature in virtual laboratories by tutoring feedback and supervising student behaviour (Duarte, Butz, Miller, & Mahalingam, 2008 ; Ramírez, Rico, Riofrío-Luzcando, Berrocal-Lobo, & Antonio, 2018 ), and the third one is a stand-alone ITS in the field of Computer Science (Paquette et al., 2015 ). One study presents an ITS of this kind in the field of second language learning (Dodigovic, 2007 ).
In two studies, the function of diagnosing mistakes and the provision of feedback is accomplished by a dialogue between the student and the computer. For example, with an interactive ubiquitous teaching robot that bases its speech on question recognition (Umarani, Raviram, & Wahidabanu, 2011 ), or with the tutoring system, based on a tutorial dialogue toolkit for introductory college Physics (Chi, VanLehn, Litman, & Jordan, 2011 ). The same tutorial dialogue toolkit (TuTalk) is the core of the peer dialogue agent presented by Howard et al. ( 2017 ), where the ITS engages in a one-on-one problem-solving peer interaction with a student and can interact verbally, graphically and in a process-oriented way, and engage in collaborative problem solving instead of tutoring. This last study could be considered as part of a new category regarding peer-agent collaboration.
Curating learning materials based on student needs
Two studies focused on this kind of ITS function (Jeschike et al., 2007 ; Schiaffino et al., 2008 ), and a third one mentions it in a more descriptive way as a feature of the detection system presented (Hall Jr & Ko, 2008 ). Schiaffino et al. ( 2008 ) present eTeacher as a system for personalised assistance to e-learning students by observing their behaviour in the course and generating a student’s profile. This enables the system to provide specific recommendations regarding the type of reading material and exercises done, as well as personalised courses of action. Jeschike et al. ( 2007 ) refers to an intelligent assistant contextualised in a virtual laboratory of statistical mechanics, where it presents exercises and the evaluation of the learners’ input to content, and interactive course material that adapts to the learner.
Facilitating collaboration between learners
Within this group we can identify only two studies: one focusing on supporting online collaborative learning discussions by using academically productive talk moves (Adamson et al., 2014 ); and the second one, on facilitating collaborative writing by providing automated feedback, generated automatic questions, and the analysis of the process (Calvo, O’Rourke, Jones, Yacef, & Reimann, 2011 ). Given the opportunities that the applications described in these studies afford for supporting collaboration among students, more research in this area would be desireable.
The teachers’ perspective
As mentioned above, Baker and Smith ( 2019 , p.12) distinguish between student and teacher-facing AI. However, only two included articles in ITS focus on the teacher’s perspective. Casamayor et al. ( 2009 ) focus on assisting teachers with the supervision and detection of conflictive cases in collaborative learning. In this study, the intelligent assistant provides the teachers with a summary of the individual progress of each group member and the type of participation each of them have had in their work groups, notification alerts derived from the detection of conflict situations, and information about the learning style of each student-logging interactions, so that the teachers can intervene when they consider it convenient. The other study put the emphasis on the ITS sharing teachers’ tutoring tasks by providing immediate feedback (automating tasks), and leaving the teachers the role of providing new hints and the correct solution to the tasks (Chou, Huang, & Lin, 2011 ). The study of Chi et al. ( 2011 ) also mentions the ITS purpose to share teacher’s tutoring tasks. The main aim in any of these cases is to reduce teacher’s workload. Furthermore, many of the learner-facing studies deal with the teacher-facing functions too, although they do not put emphasis on the teacher’s perspective.
Assessment and evaluation
Assessment and evaluation studies also largely focused on the level of teaching and learning (86%, n = 31), although five studies described applications at the institutional level. In order to gain an overview of student opinion about online and distance learning at their institution, academics at Anadolu University (Ozturk, Cicek, & Ergul, 2017 ) used sentiment analysis to analyse mentions by students on Twitter, using Twitter API Twython and terms relating to the system. This analysis of publicly accessible data, allowed researchers insight into student opinion, which otherwise may not have been accessible through their institutional LMS, and which can inform improvements to the system. Two studies used AI to evaluate student Prior Learning and Recognition (PLAR); Kalz et al. ( 2008 ) used Latent Semantic Analysis and ePortfolios to inform personalised learning pathways for students, and Biletska, Biletskiy, Li, and Vovk ( 2010 ) used semantic web technologies to convert student credentials from different institutions, which could also provide information from course descriptions and topics, to allow for easier granting of credit. The final article at the institutional level (Sanchez et al., 2016 ) used an algorithm to match students to professional competencies and capabilities required by companies, in order to ensure alignment between courses and industry needs.
Overall, the studies show that AI applications can perform assessment and evaluation tasks at very high accuracy and efficiency levels. However, due to the need to calibrate and train the systems (supervised machine learning), they are more applicable to courses or programs with large student numbers.
Articles focusing on assessment and evaluation applications of AI at the teaching and learning level, were classified into four sub-categories; automated grading ( n = 13), feedback ( n = 8), evaluation of student understanding, engagement and academic integrity ( n = 5), and evaluation of teaching ( n = 5).
Automated grading
Articles that utilised automated grading, or Automated Essay Scoring (AES) systems, came from a range of disciplines (e.g. Biology, Medicine, Business Studies, English as a Second Language), but were mostly focused on its use in undergraduate courses ( n = 10), including those with low reading and writing ability (Perin & Lauterbach, 2018 ). Gierl, Latifi, Lai, Boulais, and Champlain’s ( 2014 ) use of open source Java software LightSIDE to grade postgraduate medical student essays resulted in an agreement between the computer classification and human raters between 94.6% and 98.2%, which could enable reducing cost and the time associated with employing multiple human assessors for large-scale assessments (Barker, 2011 ; McNamara, Crossley, Roscoe, Allen, & Dai, 2015 ). However, they stressed that not all writing genres may be appropriate for AES and that it would be impractical to use in most small classrooms, due to the need to calibrate the system with a large number of pre-scored assessments. The benefits of using algorithms that find patterns in text responses, however, has been found to lead to encouraging more revisions by students (Ma & Slater, 2015 ) and to move away from merely measuring student knowledge and abilities by multiple choice tests (Nehm, Ha, & Mayfield, 2012 ). Continuing issues persist, however, in the quality of feedback provided by AES (Dikli, 2010 ), with Barker ( 2011 ) finding that the more detailed the feedback provided was, the more likely students were to question their grades, and a question was raised over the benefits of this feedback for beginning language students (Aluthman, 2016 ).
Articles concerned with feedback included a range of student-facing tools, including intelligent agents that provide students with prompts or guidance when they are confused or stalled in their work (Huang, Chen, Luo, Chen, & Chuang, 2008 ), software to alert trainee pilots when they are losing situation awareness whilst flying (Thatcher, 2014 ), and machine learning techniques with lexical features to generate automatic feedback and assist in improving student writing (Chodorow, Gamon, & Tetreault, 2010 ; Garcia-Gorrostieta, Lopez-Lopez, & Gonzalez-Lopez, 2018 ; Quixal & Meurers, 2016 ), which can help reduce students cognitive overload (Yang, Wong, & Yeh, 2009 ). The automated feedback system based on adaptive testing reported by Barker ( 2010 ), for example, not only determines the most appropriate individual answers according to Bloom’s cognitive levels, but also recommends additional materials and challenges.
Evaluation of student understanding, engagement and academic integrity
Three articles reported on student-facing tools that evaluate student understanding of concepts (Jain, Gurupur, Schroeder, & Faulkenberry, 2014 ; Zhu, Marquez, & Yoo, 2015 ) and provide personalised assistance (Samarakou, Fylladitakis, Früh, Hatziapostolou, & Gelegenis, 2015 ). Hussain et al. ( 2018 ) used machine learning algorithms to evaluate student engagement in a social science course at the Open University, including final results, assessment scores and the number of clicks that students make in the VLE, which can alert instructors to the need for intervention, and Amigud, Arnedo-Moreno, Daradoumis, and Guerrero-Roldan ( 2017 ) used machine learning algorithms to check academic integrity, by assessing the likelihood of student work being similar to their other work. With a mean accuracy of 93%, this opens up possibilities of reducing the need for invigilators or to access student accounts, thereby reducing concerns surrounding privacy.
Evaluation of teaching
Four studies used data mining algorithms to evaluate lecturer performance through course evaluations (Agaoglu, 2016 ; Ahmad & Rashid, 2016 ; DeCarlo & Rizk, 2010 ; Gutierrez, Canul-Reich, Ochoa Zezzatti, Margain, & Ponce, 2018 ), with Agaoglu ( 2016 ) finding, through using four different classification techniques, that many questions in the evaluation questionnaire were irrelevant. The application of an algorithm to evaluate the impact of teaching methods in a differential equations class, found that online homework with immediate feedback was more effective than clickers (Duzhin & Gustafsson, 2018 ). The study also found that, whilst previous exam results are generally good predictors for future exam results, they say very little about students’ expected performance in project-based tasks.
Adaptive systems and personalisation
Most of the studies on adaptive systems (85%, n = 23) are situated at the teaching and learning level, with four cases considering the institutional and administrative level. Two studies explored undergraduate students’ academic advising (Alfarsi, Omar, & Alsinani, 2017 ; Feghali, Zbib, & Hallal, 2011 ), and Nguyen et al. ( 2018 ) focused on AI to support university career services. Ng, Wong, Lee, and Lee ( 2011 ) reported on the development of an agent-based distance LMS, designed to manage resources, support decision making and institutional policy, and assist with managing undergraduate student study flow (e.g. intake, exam and course management), by giving users access to data across disciplines, rather than just individual faculty areas.
There does not seem to be agreement within the studies on a common term for adaptive systems, and that is probably due to the diverse functions they carry out, which also supports the classification of studies. Some of those terms coincide in part with the ones used for ITS, e.g. intelligent agents (Li, 2007 ; Ng et al., 2011 ). The most general terms used are intelligent e-learning system (Kose & Arslan, 2016 ), adaptive web-based learning system (Lo, Chan, & Yeh, 2012 ), or intelligent teaching system (Yuanyuan & Yajuan, 2014 ). As in ITS, most of the studies either describe the system or include a pilot study but no longer-term results are reported. Results from these pilot studies are usually reported as positive, except in Vlugter, Knott, McDonald, and Hall ( 2009 ), where the experimental group that used the dialogue-based computer assisted language-system scored lower than the control group in the delayed post-tests.
The 23 studies focused on teaching and learning can be classified into five sub-categories; teaching course content ( n = 7), recommending/providing personalised content ( n = 5), supporting teachers in learning and teaching design ( n = 3), using academic data to monitor and guide students ( n = 2), and supporting representation of knowledge using concept maps ( n = 2). However, some studies were difficult to classify, due to their specific and unique functions; helping to organise online learning groups with similar interests (Yang, Wang, Shen, & Han, 2007 ), supporting business decisions through simulation (Ben-Zvi, 2012 ), or supporting changes in attitude and behaviour for patients with Anorexia Nervosa, through embodied conversational agents (Sebastian & Richards, 2017 ). Aparicio et al. ( 2018 ) present a study where no adaptive system application was analysed, rather students’ perceptions of the use of information systems in education in general - and biomedical education in particular - were analysed, including intelligent information access systems .
The disciplines that are taught through adaptive systems are diverse, including environmental education (Huang, 2018 ), animation design (Yuanyuan & Yajuan, 2014 ), language learning (Jia, 2009 ; Vlugter et al., 2009 ), Computer Science (Iglesias, Martinez, Aler, & Fernandez, 2009 ) and Biology (Chaudhri et al., 2013 ). Walsh, Tamjidul, and Williams ( 2017 ), however, present an adaptive system based on machine learning-human machine learning symbiosis from a descriptive perspective, without specifying any discipline.
Recommending/providing personalised content
This group refers to adaptive systems that deliver customised content, materials and exercises according to students’ behaviour profiling in Business and Administration studies (Hall Jr & Ko, 2008 ) and Computer Science (Kose & Arslan, 2016 ; Lo et al., 2012 ). On the other hand, Tai, Wu, and Li ( 2008 ) present an e-learning recommendation system for online students to help them choose among courses, and Torres-Díaz, Infante Moro, and Valdiviezo Díaz ( 2014 ) emphasise the usefulness of (adaptive) recommendation systems in MOOCs to suggest actions, new items and users, according to students’ personal preferences.
Supporting teachers in learning and teaching design
In this group, three studies were identified. One study puts the emphasis on a hybrid recommender system of pedagogical patterns, to help teachers define their teaching strategies, according to the context of a specific class (Cobos et al., 2013 ), and another study presents a description of a metadata-based model to implement automatic learning designs that can solve detected problems (Camacho & Moreno, 2007 ). Li’s ( 2007 ) descriptive study argues that intelligent agents save time for online instructors, by leaving the most repetitive tasks to the systems, so that they can focus more on creative work.
Using academic data to monitor and guide students
The adaptive systems within this category focus on the extraction of student academic information to perform diagnostic tasks, and help tutors to offer a more proactive personal guidance (Rovira, Puertas, & Igual, 2017 ); or, in addition to that task, include performance evaluation and personalised assistance and feedback, such as the Learner Diagnosis, Assistance, and Evaluation System based on AI (StuDiAsE) for engineering learners (Samarakou et al., 2015 ).
Supporting representation of knowledge in concept maps
To help build students’ self-awareness of conceptual structures, concept maps can be quite useful. In the two studies of this group, an expert system was included, e.g. in order to accommodate selected peer ideas in the integrated concept maps and allow teachers to flexibly determine in which ways the selected concept maps are to be merged ( ICMSys ) (Kao, Chen, & Sun, 2010 ), or to help English as a Foreign Language college students to develop their reading comprehension through mental maps of referential identification (Yang et al., 2009 ). This latter system also includes system-guided instruction, practice and feedback.
Conclusions and implications for further educational research
In this paper, we have explored the field of AIEd research in terms of authorship and publication patterns. It is evident that US-American, Chinese, Taiwanese and Turkish colleagues (accounting for 50% of the publications as first authors) from Computer Science and STEM departments (62%) dominate the field. The leading journals are the International Journal of Artificial Intelligence in Education , Computers & Education , and the International Journal of Emerging Technologies in Learning .
More importantly, this study has provided an overview of the vast array of potential AI applications in higher education to support students, faculty members, and administrators. They were described in four broad areas (profiling and prediction, intelligent tutoring systems, assessment and evaluation, and adaptive systems and personalisation) with 17 sub-categories. This structure, which was derived from the systematic review, contributes to the understanding and conceptualisation of AIEd practice and research.
On the other hand, the lack of longitudinal studies and the substantial presence of descriptive and pilot studies from the technological perspective, as well as the prevalence of quantitative methods - especially quasi-experimental methods - in empirical studies, shows that there is still substantial room for educators to aim at innovative and meaningful research and practice with AIEd that could have learning impact within higher education, e.g. adopting design-based approaches (Easterday, Rees Lewis, & Gerber, 2018 ). A recent systematic literature review on personalisation in educational technology coincided with the predominance of experiences in technological developments, which also often used quantitative methods (Bartolomé, Castañeda, & Adell, 2018 ). Misiejuk and Wasson ( 2017 , p. 61) noted in their systematic review on Learning Analytics that “there are very few implementation studies and impact studies” (p. 61), which is also similar to the findings in the present article.
The full consequences of AI development cannot yet be foreseen today, but it seems likely that AI applications will be a top educational technology issue for the next 20 years. AI-based tools and services have a high potential to support students, faculty members and administrators throughout the student lifecycle. The applications that are described in this article provide enormous pedagogical opportunities for the design of intelligent student support systems, and for scaffolding student learning in adaptive and personalized learning environments. This applies in particular to large higher education institutions (such as open and distance teaching universities), where AIEd might help to overcome the dilemma of providing access to higher education for very large numbers of students (mass higher education). On the other hand, it might also help them to offer flexible, but also interactive and personalized learning opportunities, for example by relieving teachers from burdens, such as grading hundreds or even thousands of assignments, so that they can focus on their real task: empathic human teaching.
It is crucial to emphasise that educational technology is not (only) about technology – it is the pedagogical, ethical, social, cultural and economic dimensions of AIEd we should be concerned about. Selwyn ( 2016 , p. 106) writes:
The danger, of course, lies in seeing data and coding as an absolute rather than relative source of guidance and support. Education is far too complex to be reduced solely to data analysis and algorithms. As with digital technologies in general, digital data do not offer a neat technical fix to education dilemmas – no matter how compelling the output might be.
We should not strive for what is technically possible, but always ask ourselves what makes pedagogical sense. In China, systems are already being used to monitor student participation and expressions via face recognition in classrooms (so called Intelligent Classroom Behavior Management System, Smart Campus Footnote 8 ) and display them to the teacher on a dashboard. This is an example of educational surveillance, and it is highly questionable whether such systems provide real added value for a good teacher who should be able to capture the dynamics in a learning group (online and in an on-campus setting) and respond empathically and in a pedagogically meaningful way. In this sense, it is crucial to adopt an ethics of care (Prinsloo, 2017 ) to start thinking on how we are exploring the potential of algorithmic decision-making systems that are embedded in AIEd applications. Furthermore, we should also always remember that AI systems “first and foremost, require control by humans. Even the smartest AI systems can make very stupid mistakes. […] AI Systems are only as smart as the date used to train them” (Kaplan & Haenlein, 2019 , p. 25). Some critical voices in educational technology remind us that we should go beyond the tools, and talk again about learning and pedagogy, as well as acknowledging the human aspects of digital technology use in education (Castañeda & Selwyn, 2018 ). The new UNESCO report on challenges and opportunities of AIEd for sustainable development deals with various areas, all of which have an important pedagogical, social and ethical dimension, e.g. ensuring inclusion and equity in AIEd, preparing teachers for AI-powered education, developing quality and inclusive data systems, or ethics and transparency in data collection, use and dissemination (Pedró, Subosa, Rivas, & Valverde, 2019 ).
That being said, a stunning result of this review is the dramatic lack of critical reflection of the pedagogical and ethical implications as well as risks of implementing AI applications in higher education. Concerning ethical implications, privacy issues were also noted to be rarely addressed in empirical studies in a recent systematic review on Learning Analytics (Misiejuk & Wasson, 2017 ). More research is needed from educators and learning designers on how to integrate AI applications throughout the student lifecycle, to harness the enormous opportunities that they afford for creating intelligent learning and teaching systems. The low presence of authors affiliated with Education departments identified in our systematic review is evidence of the need for educational perspectives on these technological developments.
The lack of theory might be a syndrome within the field of educational technology in general. In a recent study, Hew, Lan, Tang, Jia, and Lo ( 2019 ) found that more than 40% of articles in three top educational technology journals were wholly a-theoretical. The systematic review by Bartolomé et al. ( 2018 ) also revealed this lack of explicit pedagogical perspectives in the studies analysed. The majority of research included in this systematic review is merely focused on analysing and finding patterns in data to develop models, and to make predictions that inform student and teacher facing applications, or to support administrative decisions using mathematical theories and machine learning methods that were developed decades ago (see Russel & Norvig, 2010 ). This kind of research is now possible through the growth of computing power and the vast availability of big digital student data. However, at this stage, there is very little evidence for the advancement of pedagogical and psychological learning theories related to AI driven educational technology. It is an important implication of this systematic review, that researchers are encouraged to be explicit about the theories that underpin empirical studies about the development and implementation of AIEd projects, in order to expand research to a broader level, helping us to understand the reasons and mechanisms behind this dynamic development that will have an enormous impact on higher education institutions in the various areas we have covered in this review.
Availability of data and materials
The datasets used and/or analysed during the current study (the bibliography of included studies) are available from the corresponding author upon request.
https://www.dfki.de/en/web/ (accessed 22 July, 2019)
https://www.tue.nl/en/news/news-overview/11-07-2019-tue-announces-eaisi-new-institute-for-intelligent-machines/ (accessed 22 July, 2019)
http://instituteforethicalaiineducation.org (accessed 22 July, 2019)
https://apo.org.au/node/229596 (accessed 22 July, 2019)
A file with all included references is available at: https://www.researchgate.net/publication/ 335911716_AIED-Ref (CC-0; DOI: https://doi.org/10.13140/RG.2.2.13000.88321 )
https://eppi.ioe.ac.uk/cms/er4/ (accessed July 22, 2019)
It is beyond the scope of this article to discuss the various machine learning methods for classification and prediction. Readers are therefore encouraged to refer to the literature referenced in the articles that are included in this review (e.g. Delen, 2010 and Umer, Susnjak, Mathrani, & Suriadi, 2017 ).
https://www.businessinsider.de/china-school-facial-recognition-technology-2018-5?r=US&IR=T (accessed July 5, 2019)
Acikkar, M., & Akay, M. F. (2009). Support vector machines for predicting the admission decision of a candidate to the School of Physical Education and Sports at Cukurova University. Expert Systems with Applications , 36 (3 PART 2), 7228–7233. https://doi.org/10.1016/j.eswa.2008.09.007 .
Article Google Scholar
Adamson, D., Dyke, G., Jang, H., & Rosé, C. P. (2014). Towards an agile approach to adapting dynamic collaboration support to student needs. International Journal of Artificial Intelligence in Education , 24 (1), 92–124. https://doi.org/10.1007/s40593-013-0012-6 .
Agaoglu, M. (2016). Predicting instructor performance using data mining techniques in higher education. IEEE Access , 4 , 2379–2387. https://doi.org/10.1109/ACCESS.2016.2568756 .
Ahmad, H., & Rashid, T. (2016). Lecturer performance analysis using multiple classifiers. Journal of Computer Science , 12 (5), 255–264. https://doi.org/10.3844/fjcssp.2016.255.264 .
Alfarsi, G. M. S., Omar, K. A. M., & Alsinani, M. J. (2017). A rule-based system for advising undergraduate students. Journal of Theoretical and Applied Information Technology , 95 (11) Retrieved from http://www.jatit.org .
Alkhasawneh, R., & Hargraves, R. H. (2014). Developing a hybrid model to predict student first year retention in STEM disciplines using machine learning techniques. Journal of STEM Education: Innovations & Research , 15 (3), 35–42 https://core.ac.uk/download/pdf/51289621.pdf .
Google Scholar
Aluko, R. O., Adenuga, O. A., Kukoyi, P. O., Soyingbe, A. A., & Oyedeji, J. O. (2016). Predicting the academic success of architecture students by pre-enrolment requirement: Using machine-learning techniques. Construction Economics and Building , 16 (4), 86–98. https://doi.org/10.5130/AJCEB.v16i4.5184 .
Aluthman, E. S. (2016). The effect of using automated essay evaluation on ESL undergraduate students’ writing skill. International Journal of English Linguistics , 6 (5), 54–67. https://doi.org/10.5539/ijel.v6n5p54 .
Amigud, A., Arnedo-Moreno, J., Daradoumis, T., & Guerrero-Roldan, A.-E. (2017). Using learning analytics for preserving academic integrity. International Review of Research in Open and Distance Learning , 18 (5), 192–210. https://doi.org/10.19173/irrodl.v18i5.3103 .
Andris, C., Cowen, D., & Wittenbach, J. (2013). Support vector machine for spatial variation. Transactions in GIS , 17 (1), 41–61. https://doi.org/10.1111/j.1467-9671.2012.01354.x .
Aparicio, F., Morales-Botello, M. L., Rubio, M., Hernando, A., Muñoz, R., López-Fernández, H., … de Buenaga, M. (2018). Perceptions of the use of intelligent information access systems in university level active learning activities among teachers of biomedical subjects. International Journal of Medical Informatics , 112 (December 2017), 21–33. https://doi.org/10.1016/j.ijmedinf.2017.12.016 .
Babić, I. D. (2017). Machine learning methods in predicting the student academic motivation. Croatian Operational Research Review , 8 (2), 443–461. https://doi.org/10.17535/crorr.2017.0028 .
Article MathSciNet Google Scholar
Bahadır, E. (2016). Using neural network and logistic regression analysis to predict prospective mathematics teachers’ academic success upon entering graduate education. Kuram ve Uygulamada Egitim Bilimleri , 16 (3), 943–964. https://doi.org/10.12738/estp.2016.3.0214 .
Bakeman, R., & Gottman, J. M. (1997). Observing interaction - an introduction to sequential analysis . Cambridge: Cambridge University Press.
Book Google Scholar
Baker, R. S. (2016). Stupid Tutoring Systems, Intelligent Humans. International Journal of Artificial Intelligence in Education , 26 (2), 600–614. https://doi.org/10.1007/s40593-016-0105-0 .
Baker, T., & Smith, L. (2019). Educ-AI-tion rebooted? Exploring the future of artificial intelligence in schools and colleges. Retrieved from Nesta Foundation website: https://media.nesta.org.uk/documents/Future_of_AI_and_education_v5_WEB.pdf
Barker, T. (2010). An automated feedback system based on adaptive testing: Extending the model. International Journal of Emerging Technologies in Learning , 5 (2), 11–14. https://doi.org/10.3991/ijet.v5i2.1235 .
Barker, T. (2011). An automated individual feedback and marking system: An empirical study. Electronic Journal of E-Learning , 9 (1), 1–14 https://www.learntechlib.org/p/52053/ .
Bartolomé, A., Castañeda, L., & Adell, J. (2018). Personalisation in educational technology: The absence of underlying pedagogies. International Journal of Educational Technology in Higher Education , 15 (14). https://doi.org/10.1186/s41239-018-0095-0 .
Ben-Zvi, T. (2012). Measuring the perceived effectiveness of decision support systems and their impact on performance. Decision Support Systems , 54 (1), 248–256. https://doi.org/10.1016/j.dss.2012.05.033 .
Biletska, O., Biletskiy, Y., Li, H., & Vovk, R. (2010). A semantic approach to expert system for e-assessment of credentials and competencies. Expert Systems with Applications , 37 (10), 7003–7014. https://doi.org/10.1016/j.eswa.2010.03.018 .
Blikstein, P., Worsley, M., Piech, C., Sahami, M., Cooper, S., & Koller, D. (2014). Programming pluralism: Using learning analytics to detect patterns in the learning of computer programming. Journal of the Learning Sciences , 23 (4), 561–599. https://doi.org/10.1080/10508406.2014.954750 .
Brunton, J., & Thomas, J. (2012). Information management in systematic reviews. In D. Gough, S. Oliver, & J. Thomas (Eds.), An introduction to systematic reviews , (pp. 83–106). London: SAGE.
Calvo, R. A., O’Rourke, S. T., Jones, J., Yacef, K., & Reimann, P. (2011). Collaborative writing support tools on the cloud. IEEE Transactions on Learning Technologies , 4 (1), 88–97 https://www.learntechlib.org/p/73461/ .
Camacho, D., & Moreno, M. D. R. (2007). Towards an automatic monitoring for higher education learning design. International Journal of Metadata, Semantics and Ontologies , 2 (1), 1. https://doi.org/10.1504/ijmso.2007.015071 .
Casamayor, A., Amandi, A., & Campo, M. (2009). Intelligent assistance for teachers in collaborative e-learning environments. Computers & Education , 53 (4), 1147–1154. https://doi.org/10.1016/j.compedu.2009.05.025 .
Castañeda, L., & Selwyn, N. (2018). More than tools? Making sense of he ongoing digitizations of higher education. International Journal of Educational Technology in Higher Education , 15 (22). https://doi.org/10.1186/s41239-018-0109-y .
Chaudhri, V. K., Cheng, B., Overtholtzer, A., Roschelle, J., Spaulding, A., Clark, P., … Gunning, D. (2013). Inquire biology: A textbook that answers questions. AI Magazine , 34 (3), 55–55. https://doi.org/10.1609/aimag.v34i3.2486 .
Chen, J.-F., & Do, Q. H. (2014). Training neural networks to predict student academic performance: A comparison of cuckoo search and gravitational search algorithms. International Journal of Computational Intelligence and Applications , 13 (1). https://doi.org/10.1142/S1469026814500059 .
Chi, M., VanLehn, K., Litman, D., & Jordan, P. (2011). Empirically evaluating the application of reinforcement learning to the induction of effective and adaptive pedagogical strategies. User Modeling and User-Adapted Interaction , 21 (1), 137–180. https://doi.org/10.1007/s11257-010-9093-1 .
Chodorow, M., Gamon, M., & Tetreault, J. (2010). The utility of article and preposition error correction systems for English language learners: Feedback and assessment. Language Testing , 27 (3), 419–436. https://doi.org/10.1177/0265532210364391 .
Chou, C.-Y., Huang, B.-H., & Lin, C.-J. (2011). Complementary machine intelligence and human intelligence in virtual teaching assistant for tutoring program tracing. Computers & Education , 57 (4), 2303–2312 https://www.learntechlib.org/p/167322/ .
Cobos, C., Rodriguez, O., Rivera, J., Betancourt, J., Mendoza, M., León, E., & Herrera-Viedma, E. (2013). A hybrid system of pedagogical pattern recommendations based on singular value decomposition and variable data attributes. Information Processing and Management , 49 (3), 607–625. https://doi.org/10.1016/j.ipm.2012.12.002 .
Cohen, J. (1960). A coefficient of agreement for nominal scales. Educational and Psychological Measurement , 20 , 37–46. https://doi.org/10.1177/001316446002000104 .
Contact North. (2018). Ten facts about artificial intelligence in teaching and learning. Retrieved from https://teachonline.ca/sites/default/files/tools-trends/downloads/ten_facts_about_artificial_intelligence.pdf
Crown, S., Fuentes, A., Jones, R., Nambiar, R., & Crown, D. (2011). Anne G. Neering: Interactive chatbot to engage and motivate engineering students. Computers in Education Journal , 21 (2), 24–34.
DeCarlo, P., & Rizk, N. (2010). The design and development of an expert system prototype for enhancing exam quality. International Journal of Advanced Corporate Learning , 3 (3), 10–13. https://doi.org/10.3991/ijac.v3i3.1356 .
Delen, D. (2010). A comparative analysis of machine learning techniques for student retention management. Decision Support Systems , 49 (4), 498–506. https://doi.org/10.1016/j.dss.2010.06.003 .
Delen, D. (2011). Predicting student attrition with data mining methods. Journal of College Student Retention: Research, Theory and Practice , 13 (1), 17–35. https://doi.org/10.2190/CS.13.1.b .
Dikli, S. (2010). The nature of automated essay scoring feedback. CALICO Journal , 28 (1), 99–134. https://doi.org/10.11139/cj.28.1.99-134 .
Dobre, I. (2014). Assessing the student′s knowledge in informatics discipline using the METEOR metric. Mediterranean Journal of Social Sciences , 5 (19), 84–92. https://doi.org/10.5901/mjss.2014.v5n19p84 .
Dodigovic, M. (2007). Artificial intelligence and second language learning: An efficient approach to error remediation. Language Awareness , 16 (2), 99–113. https://doi.org/10.2167/la416.0 .
Duarte, M., Butz, B., Miller, S., & Mahalingam, A. (2008). An intelligent universal virtual laboratory (UVL). IEEE Transactions on Education , 51 (1), 2–9. https://doi.org/10.1109/SSST.2002.1027009 .
Duffy, M. C., & Azevedo, R. (2015). Motivation matters: Interactions between achievement goals and agent scaffolding for self-regulated learning within an intelligent tutoring system. Computers in Human Behavior , 52 , 338–348. https://doi.org/10.1016/j.chb.2015.05.041 .
Duzhin, F., & Gustafsson, A. (2018). Machine learning-based app for self-evaluation of teacher-specific instructional style and tools. Education Sciences , 8 (1). https://doi.org/10.3390/educsci8010007 .
Easterday, M. W., Rees Lewis, D. G., & Gerber, E. M. (2018). The logic of design research. Learning: Research and Practice , 4 (2), 131–160. https://doi.org/10.1080/23735082.2017.1286367 .
EDUCAUSE. (2018). Horizon report: 2018 higher education edition. Retrieved from EDUCAUSE Learning Initiative and The New Media Consortium website: https://library.educause.edu/~/media/files/library/2018/8/2018horizonreport.pdf
EDUCAUSE. (2019). Horizon report: 2019 higher education edition. Retrieved from EDUCAUSE Learning Initiative and The New Media Consortium website: https://library.educause.edu/-/media/files/library/2019/4/2019horizonreport.pdf
Feghali, T., Zbib, I., & Hallal, S. (2011). A web-based decision support tool for academic advising. Educational Technology and Society , 14 (1), 82–94 https://www.learntechlib.org/p/52325/ .
Feng, S., Zhou, S., & Liu, Y. (2011). Research on data mining in university admissions decision-making. International Journal of Advancements in Computing Technology , 3 (6), 176–186. https://doi.org/10.4156/ijact.vol3.issue6.21 .
Fleiss, J. L. (1981). Statistical methods for rates and proportions . New York: Wiley.
MATH Google Scholar
Garcia-Gorrostieta, J. M., Lopez-Lopez, A., & Gonzalez-Lopez, S. (2018). Automatic argument assessment of final project reports of computer engineering students. Computer Applications in Engineering Education, 26(5), 1217–1226. https://doi.org/10.1002/cae.21996
Ge, C., & Xie, J. (2015). Application of grey forecasting model based on improved residual correction in the cost estimation of university education. International Journal of Emerging Technologies in Learning , 10 (8), 30–33. https://doi.org/10.3991/ijet.v10i8.5215 .
Gierl, M., Latifi, S., Lai, H., Boulais, A., & Champlain, A. (2014). Automated essay scoring and the future of educational assessment in medical education. Medical Education , 48 (10), 950–962. https://doi.org/10.1111/medu.12517 .
Gough, D., Oliver, S., & Thomas, J. (2017). An introduction to systematic reviews , (2nd ed., ). Los Angeles: SAGE.
Gutierrez, G., Canul-Reich, J., Ochoa Zezzatti, A., Margain, L., & Ponce, J. (2018). Mining: Students comments about teacher performance assessment using machine learning algorithms. International Journal of Combinatorial Optimization Problems and Informatics , 9 (3), 26–40 https://ijcopi.org/index.php/ojs/article/view/99 .
Hall Jr., O. P., & Ko, K. (2008). Customized content delivery for graduate management education: Application to business statistics. Journal of Statistics Education , 16 (3). https://doi.org/10.1080/10691898.2008.11889571 .
Haugeland, J. (1985). Artificial intelligence: The very idea. Cambridge, Mass.: MIT Press
Hew, K. F., Lan, M., Tang, Y., Jia, C., & Lo, C. K. (2019). Where is the “theory” within the field of educational technology research? British Journal of Educational Technology , 50 (3), 956–971. https://doi.org/10.1111/bjet.12770 .
Hinojo-Lucena, F.-J., Aznar-Díaz, I., Cáceres-Reche, M.-P., & Romero-Rodríguez, J.-M. (2019). Artificial intelligence in higher education: A bibliometric study on its impact in the scientific literature. Education Sciences , 9 (1), 51. https://doi.org/10.3390/educsci9010051 .
Hoffait, A.-S., & Schyns, M. (2017). Early detection of university students with potential difficulties. Decision Support Systems , 101 , 1–11. https://doi.org/10.1016/j.dss.2017.05.003 .
Hooshyar, D., Ahmad, R., Yousefi, M., Yusop, F., & Horng, S. (2015). A flowchart-based intelligent tutoring system for improving problem-solving skills of novice programmers. Journal of Computer Assisted Learning , 31 (4), 345–361. https://doi.org/10.1111/jcal.12099 .
Howard, C., Jordan, P., di Eugenio, B., & Katz, S. (2017). Shifting the load: A peer dialogue agent that encourages its human collaborator to contribute more to problem solving. International Journal of Artificial Intelligence in Education , 27 (1), 101–129. https://doi.org/10.1007/s40593-015-0071-y .
Howard, E., Meehan, M., & Parnell, A. (2018). Contrasting prediction methods for early warning systems at undergraduate level. Internet and Higher Education , 37 , 66–75. https://doi.org/10.1016/j.iheduc.2018.02.001 .
Huang, C.-J., Chen, C.-H., Luo, Y.-C., Chen, H.-X., & Chuang, Y.-T. (2008). Developing an intelligent diagnosis and assessment e-Learning tool for introductory programming. Educational Technology & Society , 11 (4), 139–157 https://www.jstor.org/stable/jeductechsoci.11.4.139 .
Huang, J., & Chen, Z. (2016). The research and design of web-based intelligent tutoring system. International Journal of Multimedia and Ubiquitous Engineering , 11 (6), 337–348. https://doi.org/10.14257/ijmue.2016.11.6.30 .
Huang, S. P. (2018). Effects of using artificial intelligence teaching system for environmental education on environmental knowledge and attitude. Eurasia Journal of Mathematics, Science and Technology Education , 14 (7), 3277–3284. https://doi.org/10.29333/ejmste/91248 .
Hussain, M., Zhu, W., Zhang, W., & Abidi, S. M. R. (2018). Student engagement predictions in an e-Learning system and their impact on student course assessment scores. Computational Intelligence and Neuroscience . https://doi.org/10.1155/2018/6347186 .
Iglesias, A., Martinez, P., Aler, R., & Fernandez, F. (2009). Reinforcement learning of pedagogical policies in adaptive and intelligent educational systems. Knowledge-Based Systems , 22 (4), 266–270 https://e-archivo.uc3m.es/bitstream/handle/10016/6502/reinforcement_aler_KBS_2009_ps.pdf?sequence=1&isAllowed=y .
Jackson, M., & Cossitt, B. (2015). Is intelligent online tutoring software useful in refreshing financial accounting knowledge? Advances in Accounting Education: Teaching and Curriculum Innovations , 16 , 1–19. https://doi.org/10.1108/S1085-462220150000016001 .
Jain, G. P., Gurupur, V. P., Schroeder, J. L., & Faulkenberry, E. D. (2014). Artificial intelligence-based student learning evaluation: A concept map-based approach for analyzing a student’s understanding of a topic. IEEE Transactions on Learning Technologies , 7 (3), 267–279. https://doi.org/10.1109/TLT.2014.2330297 .
Jeschike, M., Jeschke, S., Pfeiffer, O., Reinhard, R., & Richter, T. (2007). Equipping virtual laboratories with intelligent training scenarios. AACE Journal , 15 (4), 413–436 h ttps://www.learntechlib.org/primary/p/23636/ .
Jia, J. (2009). An AI framework to teach English as a foreign language: CSIEC. AI Magazine , 30 (2), 59–59. https://doi.org/10.1609/aimag.v30i2.2232 .
Jonassen, D., Davidson, M., Collins, M., Campbell, J., & Haag, B. B. (1995). Constructivism and computer-mediated communication in distance education. American Journal of Distance Education , 9 (2), 7–25. https://doi.org/10.1080/08923649509526885 .
Kalz, M., van Bruggen, J., Giesbers, B., Waterink, W., Eshuis, J., & Koper, R. (2008). A model for new linkages for prior learning assessment. Campus-Wide Information Systems , 25 (4), 233–243. https://doi.org/10.1108/10650740810900676 .
Kao, Chen, & Sun (2010). Using an e-Learning system with integrated concept maps to improve conceptual understanding. International Journal of Instructional Media , 37 (2), 151–151.
Kaplan, A., & Haenlein, M. (2019). Siri, Siri, in my hand: Who’s the fairest in the land? On the interpretations, illustrations, and implications of artificial intelligence. Business Horizons , 62 (1), 15–25. https://doi.org/10.1016/j.bushor.2018.08.004 .
Kardan, A. A., & Sadeghi, H. (2013). A decision support system for course offering in online higher education institutes. International Journal of Computational Intelligence Systems , 6 (5), 928–942. https://doi.org/10.1080/18756891.2013.808428 .
Kardan, A. A., Sadeghi, H., Ghidary, S. S., & Sani, M. R. F. (2013). Prediction of student course selection in online higher education institutes using neural network. Computers and Education , 65 , 1–11. https://doi.org/10.1016/j.compedu.2013.01.015 .
Kose, U., & Arslan, A. (2016). Intelligent e-Learning system for improving students’ academic achievements in computer programming courses. International Journal of Engineering Education , 32 (1, A), 185–198.
Li, X. (2007). Intelligent agent-supported online education. Decision Sciences Journal of Innovative Education , 5 (2), 311–331. https://doi.org/10.1111/j.1540-4609.2007.00143.x .
Lo, J. J., Chan, Y. C., & Yeh, S. W. (2012). Designing an adaptive web-based learning system based on students’ cognitive styles identified online. Computers and Education , 58 (1), 209–222. https://doi.org/10.1016/j.compedu.2011.08.018 .
Lodhi, P., Mishra, O., Jain, S., & Bajaj, V. (2018). StuA: An intelligent student assistant. International Journal of Interactive Multimedia and Artificial Intelligence , 5 (2), 17–25. https://doi.org/10.9781/ijimai.2018.02.008 .
Luckin, R., Holmes, W., Griffiths, M., & Forcier, L. B. (2016). Intelligence unleashed - an argument for AI in education. Retrieved from http://discovery.ucl.ac.uk/1475756/
Ma, H., & Slater, T. (2015). Using the developmental path of cause to bridge the gap between AWE scores and writing teachers’ evaluations. Writing & Pedagogy , 7 (2), 395–422. https://doi.org/10.1558/wap.v7i2-3.26376 .
McNamara, D. S., Crossley, S. A., Roscoe, R. D., Allen, L. K., & Dai, J. (2015). A hierarchical classification approach to automated essay scoring. Assessing Writing , 23 , 35–59. https://doi.org/10.1016/j.asw.2014.09.002 .
Misiejuk, K., & Wasson, B. (2017). State of the field report on learning analytics. SLATE report 2017–2 . Bergen: Centre for the Science of Learning & Technology (SLATE) Retrieved from http://bora.uib.no/handle/1956/17740 .
Miwa, K., Terai, H., Kanzaki, N., & Nakaike, R. (2014). An intelligent tutoring system with variable levels of instructional support for instructing natural deduction. Transactions of the Japanese Society for Artificial Intelligence , 29 (1), 148–156. https://doi.org/10.1527/tjsai.29.148 .
Moher, D., Liberati, A., Tetzlaff, J., & Altman, D. G. (2009). Preferred reporting items for systematic reviews and meta-analyses: The PRISMA statement. BMJ , 339 , b2535. https://doi.org/10.1136/bmj.b2535 Clinical Research Ed.
Nehm, R. H., Ha, M., & Mayfield, E. (2012). Transforming biology assessment with machine learning: Automated scoring of written evolutionary explanations. Journal of Science Education and Technology , 21 (1), 183–196. https://doi.org/10.1007/s10956-011-9300-9 .
Neumann, W. L. (2007). Social research methods: Qualitative and quantitative approaches . Boston: Pearson.
Ng, S. C., Wong, C. K., Lee, T. S., & Lee, F. Y. (2011). Design of an agent-based academic information system for effective education management. Information Technology Journal , 10 (9), 1784–1788. https://doi.org/10.3923/itj.2011.1784.1788 .
Nguyen, J., Sánchez-Hernández, G., Armisen, A., Agell, N., Rovira, X., & Angulo, C. (2018). A linguistic multi-criteria decision-aiding system to support university career services. Applied Soft Computing Journal , 67 , 933–940. https://doi.org/10.1016/j.asoc.2017.06.052 .
Nicholas, D., Watkinson, A., Jamali, H. R., Herman, E., Tenopir, C., Volentine, R., … Levine, K. (2015). Peer review: still king in the digital age. Learned Publishing , 28 (1), 15–21. https://doi.org/10.1087/20150104 .
Oztekin, A. (2016). A hybrid data analytic approach to predict college graduation status and its determinative factors. Industrial Management and Data Systems , 116 (8), 1678–1699. https://doi.org/10.1108/IMDS-09-2015-0363 .
Ozturk, Z. K., Cicek, Z. I. E., & Ergul, Z. (2017). Sentiment analysis: An application to Anadolu University. Acta Physica Polonica A , 132 (3), 753–755. https://doi.org/10.12693/APhysPolA.132.753 .
Palocsay, S. W., & Stevens, S. P. (2008). A study of the effectiveness of web-based homework in teaching undergraduate business statistics. Decision Sciences Journal of Innovative Education , 6 (2), 213–232. https://doi.org/10.1111/j.1540-4609.2008.00167.x .
Paquette, L., Lebeau, J. F., Beaulieu, G., & Mayers, A. (2015). Designing a knowledge representation approach for the generation of pedagogical interventions by MTTs. International Journal of Artificial Intelligence in Education , 25 (1), 118–156 https://www.learntechlib.org/p/168275/ .
Payne, V. L., Medvedeva, O., Legowski, E., Castine, M., Tseytlin, E., Jukic, D., & Crowley, R. S. (2009). Effect of a limited-enforcement intelligent tutoring system in dermatopathology on student errors, goals and solution paths. Artificial Intelligence in Medicine , 47 (3), 175–197. https://doi.org/10.1016/j.artmed.2009.07.002 .
Pedró, F., Subosa, M., Rivas, A., & Valverde, P. (2019). Artificial intelligence in education: Challenges and opportunities for sustainable development . Paris: UNESCO.
Perez, S., Massey-Allard, J., Butler, D., Ives, J., Bonn, D., Yee, N., & Roll, I. (2017). Identifying productive inquiry in virtual labs using sequence mining. In E. André, R. Baker, X. Hu, M. M. T. Rodrigo, & B. du Boulay (Eds.), Artificial intelligence in education , (vol. 10,331, pp. 287–298). https://doi.org/10.1007/978-3-319-61425-0_24 .
Chapter Google Scholar
Perin, D., & Lauterbach, M. (2018). Assessing text-based writing of low-skilled college students. International Journal of Artificial Intelligence in Education , 28 (1), 56–78. https://doi.org/10.1007/s40593-016-0122-z .
Petticrew, M., & Roberts, H. (2006). Systematic reviews in the social sciences: A practical guide . Malden; Oxford: Blackwell Pub.
Phani Krishna, K. V., Mani Kumar, M., & Aruna Sri, P. S. G. (2018). Student information system and performance retrieval through dashboard. International Journal of Engineering and Technology (UAE) , 7 , 682–685. https://doi.org/10.14419/ijet.v7i2.7.10922 .
Popenici, S., & Kerr, S. (2017). Exploring the impact of artificial intelligence on teaching and learning in higher education. Research and Practice in Technology Enhanced Learning . https://doi.org/10.1186/s41039-017-0062-8 .
Prinsloo, P. (2017). Fleeing from Frankenstein’s monster and meeting Kafka on the way: Algorithmic decision-making in higher education. E-Learning and Digital Media , 14 (3), 138–163. https://doi.org/10.1177/2042753017731355 .
Quixal, M., & Meurers, D. (2016). How can writing tasks be characterized in a way serving pedagogical goals and automatic analysis needs? Calico Journal , 33 (1), 19–48. https://doi.org/10.1558/cj.v33i1.26543 .
Raju, D., & Schumacker, R. (2015). Exploring student characteristics of retention that lead to graduation in higher education using data mining models. Journal of College Student Retention: Research, Theory and Practice , 16 (4), 563–591. https://doi.org/10.2190/CS.16.4.e .
Ramírez, J., Rico, M., Riofrío-Luzcando, D., Berrocal-Lobo, M., & Antonio, A. (2018). Students’ evaluation of a virtual world for procedural training in a tertiary-education course. Journal of Educational Computing Research , 56 (1), 23–47. https://doi.org/10.1177/0735633117706047 .
Ray, R. D., & Belden, N. (2007). Teaching college level content and reading comprehension skills simultaneously via an artificially intelligent adaptive computerized instructional system. Psychological Record , 57 (2), 201–218 https://opensiuc.lib.siu.edu/cgi/viewcontent.cgi?referer=https://www.google.com/&httpsredir=1&article=1103&context=tpr .
Reid, J. (1995). Managing learner support. In F. Lockwood (Ed.), Open and distance learning today , (pp. 265–275). London: Routledge.
Rovira, S., Puertas, E., & Igual, L. (2017). Data-driven system to predict academic grades and dropout. PLoS One , 12 (2), 1–21. https://doi.org/10.1371/journal.pone.0171207 .
Russel, S., & Norvig, P. (2010). Artificial intelligence - a modern approach . New Jersey: Pearson Education.
Salmon, G. (2000). E-moderating - the key to teaching and learning online , (1st ed., ). London: Routledge.
Samarakou, M., Fylladitakis, E. D., Früh, W. G., Hatziapostolou, A., & Gelegenis, J. J. (2015). An advanced eLearning environment developed for engineering learners. International Journal of Emerging Technologies in Learning , 10 (3), 22–33. https://doi.org/10.3991/ijet.v10i3.4484 .
Sanchez, E. L., Santos-Olmo, A., Alvarez, E., Huerta, M., Camacho, S., & Fernandez-Medina, E. (2016). Development of an expert system for the evaluation of students’ curricula on the basis of competencies. Future Internet , 8 (2). https://doi.org/10.3390/fi8020022 .
Schiaffino, S., Garcia, P., & Amandi, A. (2008). eTeacher: Providing personalized assistance to e-learning students. Computers & Education , 51 (4), 1744–1754. https://doi.org/10.1016/j.compedu.2008.05.008 .
Sebastian, J., & Richards, D. (2017). Changing stigmatizing attitudes to mental health via education and contact with embodied conversational agents. Computers in Human Behavior , 73 , 479–488. https://doi.org/10.1016/j.chb.2017.03.071 .
Selwyn, N. (2016). Is technology good for education? Cambridge, UK: Malden, MA : Polity Press.
Shen, V. R. L., & Yang, C.-Y. (2011). Intelligent multiagent tutoring system in artificial intelligence. International Journal of Engineering Education , 27 (2), 248–256.
Šimundić, A.-M. (2009). Measures of diagnostic accuracy: Basic definitions. Journal of the International Federation of Clinical Chemistry and Laboratory Medicine , 19 (4), 203–2011 https://www.ncbi.nlm.nih.gov/pubmed/27683318 .
Smith, R. (2006). Peer review: a flawed process at the heart of science and journals. Journal of the Royal Society of Medicine , 99 , 178–182. https://doi.org/10.1258/jrsm.99.4.178 .
Spikol, D., Ruffaldi, E., Dabisias, G., & Cukurova, M. (2018). Supervised machine learning in multimodal learning analytics for estimating success in project-based learning. Journal of Computer Assisted Learning , 34 (4), 366–377. https://doi.org/10.1111/jcal.12263 .
Sreenivasa Rao, K., Swapna, N., & Praveen Kumar, P. (2018). Educational data mining for student placement prediction using machine learning algorithms. International Journal of Engineering and Technology (UAE) , 7 (1.2), 43–46. https://doi.org/10.14419/ijet.v7i1.2.8988 .
Steenbergen-Hu, S., & Cooper, H. (2014). A meta-analysis of the effectiveness of intelligent tutoring systems on college students’ academic learning. Journal of Educational Psychology , 106 (2), 331–347. https://doi.org/10.1037/a0034752 .
Sultana, S., Khan, S., & Abbas, M. (2017). Predicting performance of electrical engineering students using cognitive and non-cognitive features for identification of potential dropouts. International Journal of Electrical Engineering Education , 54 (2), 105–118. https://doi.org/10.1177/0020720916688484 .
Tai, D. W. S., Wu, H. J., & Li, P. H. (2008). Effective e-learning recommendation system based on self-organizing maps and association mining. Electronic Library , 26 (3), 329–344. https://doi.org/10.1108/02640470810879482 .
Tegmark, M. (2018). Life 3.0: Being human in the age of artificial intelligence . London: Penguin Books.
Teshnizi, S. H., & Ayatollahi, S. M. T. (2015). A comparison of logistic regression model and artificial neural networks in predicting of student’s academic failure. Acta Informatica Medica, 23(5), 296-300. https://doi.org/10.5455/aim.2015.23.296-300
Thatcher, S. J. (2014). The use of artificial intelligence in the learning of flight crew situation awareness in an undergraduate aviation programme. World Transactions on Engineering and Technology Education , 12 (4), 764–768 https://www.semanticscholar.org/paper/The-use-of-artificial-intelligence-in-the-learning-Thatcher/758d3053051511cde2f28fc6b2181b8e227f8ea2 .
Torres-Díaz, J. C., Infante Moro, A., & Valdiviezo Díaz, P. (2014). Los MOOC y la masificación personalizada. Profesorado , 18 (1), 63–72 http://www.redalyc.org/articulo.oa?id=56730662005 .
Umarani, S. D., Raviram, P., & Wahidabanu, R. S. D. (2011). Speech based question recognition of interactive ubiquitous teaching robot using supervised classifier. International Journal of Engineering and Technology , 3 (3), 239–243 http://www.enggjournals.com/ijet/docs/IJET11-03-03-35.pdf .
Umer, R., Susnjak, T., Mathrani, A., & Suriadi, S. (2017). On predicting academic performance with process mining in learning analytics. Journal of Research in Innovative Teaching , 10 (2), 160–176. https://doi.org/10.1108/JRIT-09-2017-0022 .
Vlugter, P., Knott, A., McDonald, J., & Hall, C. (2009). Dialogue-based CALL: A case study on teaching pronouns. Computer Assisted Language Learning , 22 (2), 115–131. https://doi.org/10.1080/09588220902778260 .
Walsh, K., Tamjidul, H., & Williams, K. (2017). Human machine learning symbiosis. Journal of Learning in Higher Education , 13 (1), 55–62 http://cs.uno.edu/~tamjid/pub/2017/JLHE.pdf .
Welham, D. (2008). AI in training (1980–2000): Foundation for the future or misplaced optimism? British Journal of Educational Technology , 39 (2), 287–303. https://doi.org/10.1111/j.1467-8535.2008.00818.x .
Weston-Sementelli, J. L., Allen, L. K., & McNamara, D. S. (2018). Comprehension and writing strategy training improves performance on content-specific source-based writing tasks. International Journal of Artificial Intelligence in Education , 28 (1), 106–137. https://doi.org/10.1007/s40593-016-0127-7 .
Wickham, H., & Grolemund, G. (2016). R for data science: Import, tidy, transform, visualize, and model data , (1st ed., ). Sebastopol: O’Reilly.
Yang, F., Wang, M., Shen, R., & Han, P. (2007). Community-organizing agent: An artificial intelligent system for building learning communities among large numbers of learners. Computers & Education , 49 (2), 131–147. https://doi.org/10.1016/j.compedu.2005.04.019 .
Yang, Y. F., Wong, W. K., & Yeh, H. C. (2009). Investigating readers’ mental maps of references in an online system. Computers and Education , 53 (3), 799–808. https://doi.org/10.1016/j.compedu.2009.04.016 .
Yoo, J., & Kim, J. (2014). Can online discussion participation predict group project performance? Investigating the roles of linguistic features and participation patterns. International Journal of Artificial Intelligence in Education , 24 (1), 8–32 https://www.learntechlib.org/p/155243/ .
Yuanyuan, J., & Yajuan, L. (2014). Development of an intelligent teaching system based on 3D technology in the course of digital animation production. International Journal of Emerging Technologies in Learning , 9 (9), 81–86. https://doi.org/10.3991/ijet.v11i09.6116 .
Zhu, W., Marquez, A., & Yoo, J. (2015). “Engineering economics jeopardy!” Mobile app for university students. Engineering Economist , 60 (4), 291–306. https://doi.org/10.1080/0013791X.2015.1067343 .
Download references
Acknowledgements
Not applicable.
This study received no external funding.
Author information
Authors and affiliations.
Faculty of Education and Social Sciences, University of Oldenburg, Ammerländer Heerstr. 138, 26129, Oldenburg, Germany
Olaf Zawacki-Richter, Victoria I. Marín, Melissa Bond & Franziska Gouverneur
You can also search for this author in PubMed Google Scholar
Contributions
The authors declare that each author has made a substantial contribution to this article, has approved the submitted version of this article and hast agreed to be personally accountable for the author’s own contributions. In particular, OZR as the leading author, has made a major contribution to the conception and design of the research; the data collection, screening of abstracts and full papers, the analysis, synthesis and interpretation of data; VIM has made a major contribution to the data collection, screening of abstracts and full papers, the analysis, synthesis and interpretation of data; MB has made a major contribution to the data collection, screening of full papers, the analysis, synthesis and interpretation of data; as a native speaker of English she was also responsible for language editing; FG has made a major contribution to the data collection, and the screening of abstracts and full papers. She calculated Cohen’s kappa values of interrater reliability.
Authors’ information
Dr. Olaf Zawacki-Richter is a Professor of Educational Technology in the Faculty of Education and Social Sciences at the University of Oldenburg in Germany. He is the Director of the Center for Open Education Research (COER) and the Center for Lifelong Learning (C3L).
Dr. Victoria I. Marín is a Post-doctoral Researcher in the Faculty of Education and Social Sciences / Center for Open Education Research (COER) at the University of Oldenburg in Germany.
Melissa Bond is a PhD candidate and Research Associate in the Faculty of Education and Social Sciences / Center for Open Education Research (COER) at the University of Oldenburg in Germany.
Franziska Gouverneur is a Masters student and Research Assistant in the Faculty of Education and Social Sciences / Center for Open Education Research (COER) at the University of Oldenburg in Germany.
Corresponding author
Correspondence to Olaf Zawacki-Richter .
Ethics declarations
Competing interests.
The authors declare that they have no competing interests.
Additional information
Publisher’s note.
Springer Nature remains neutral with regard to jurisdictional claims in published maps and institutional affiliations.
Rights and permissions
Open Access This article is distributed under the terms of the Creative Commons Attribution 4.0 International License ( http://creativecommons.org/licenses/by/4.0/ ), which permits unrestricted use, distribution, and reproduction in any medium, provided you give appropriate credit to the original author(s) and the source, provide a link to the Creative Commons license, and indicate if changes were made.
Reprints and permissions
About this article
Cite this article.
Zawacki-Richter, O., Marín, V.I., Bond, M. et al. Systematic review of research on artificial intelligence applications in higher education – where are the educators?. Int J Educ Technol High Educ 16 , 39 (2019). https://doi.org/10.1186/s41239-019-0171-0
Download citation
Received : 26 July 2019
Accepted : 01 October 2019
Published : 28 October 2019
DOI : https://doi.org/10.1186/s41239-019-0171-0
Share this article
Anyone you share the following link with will be able to read this content:
Sorry, a shareable link is not currently available for this article.
Provided by the Springer Nature SharedIt content-sharing initiative
- Artificial intelligence
- Higher education
- Machine learning
- Systematic review
ODU Digital Commons
- < Previous
Home > Education > Teaching & Learning > Faculty Pubs > 253
Teaching & Learning Faculty Publications
A systematic review of educational online peer-review and assessment systems: charting the landscape.
Dmytro Babik , James Madison University Edward Gehringer , North Carolina State University Jennifer Kidd , Old Dominion University Follow Kristine Sunday , Old Dominion University Follow David Tinapple , Arizona State University Steven Gilbert , The TLT Group
Document Type
Publication date.
10.1007/s11423-024-10349-x
Publication Title
Educational Technology Research & Development
Over the past two decades, there has been an explosion of innovation in software tools that encapsulate and expand the capabilities of the widely used student peer assessment. While the affordances and pedagogical impacts of traditional in-person, "paper-and-pencil" peer assessment have been studied extensively and are relatively well understood, computerized (online) peer assessment introduced not only shifts in scalability and efficiency, but also entirely new capabilities and forms of social learning interactions, instructor leverage, and distributed cognition, that still need to be researched and systematized. Despite the ample research on traditional peer assessment and evidence of its efficacy, common vocabulary and shared understanding of online peer-assessment system design, including the variety of methods, techniques, and implementations, is still missing. We present key findings of a comprehensive survey based on a systematic research framework for examining and generalizing affordances and constraints of online peer-assessment systems. This framework (a) provides a foundation of a design-science metatheory of online peer assessment, (b) helps structure the discussion of user needs and design options, and (c) informs educators and system design practitioners. We identified two major themes in existing and potential research—orientation towards scaffolded learning vs. exploratory learning and system maturity. We also outlined an agenda for future studies.
© The Authors 2024.
This article is licensed under a Creative Commons Attribution 4.0 International (CC BY 4.0) License, which permits use, sharing, adaptation, distribution and reproduction in any medium or format, as long as you give appropriate credit to the original author(s) and the source, provide a link to the Creative Commons license, and indicate if changes were made. The images or other third party material in this article are included in the article’s Creative Commons license, unless indicated otherwise in a credit line to the material. If material is not included in the article’s Creative Commons license and your intended use is not permitted by statutory regulation or exceeds the permitted use, you will need to obtain permission directly from the copyright holder.
Original Publication Citation
Babik, D., Gehringer, E., Kidd, J., Sunday, K., Tinapple, D., & Gilbert, S. (2024). A systematic review of educational online peer-review and assessment systems: Charting the landscape. Educational Technology Research & Development , 72 (3), 1653-1689. https://doi.org/10.1007/s11423-024-10349-x
Repository Citation
Babik, Dmytro; Gehringer, Edward; Kidd, Jennifer; Sunday, Kristine; Tinapple, David; and Gilbert, Steven, "A Systematic Review of Educational Online Peer-Review and Assessment Systems: Charting the Landscape" (2024). Teaching & Learning Faculty Publications . 253. https://digitalcommons.odu.edu/teachinglearning_fac_pubs/253
Included in
Educational Assessment, Evaluation, and Research Commons , Educational Technology Commons , Instructional Media Design Commons
Advanced Search
- Notify me via email or RSS
- Collections
- Disciplines
- Author Guidelines
- Submit Works
- Department of Teaching & Learning
- Other Digital Collections
- ODU Libraries
- Old Dominion University
Home | About | FAQ | My Account | Accessibility Statement
Privacy Copyright
- Open access
- Published: 02 August 2024
Moral courage level of nurses: a systematic review and meta-analysis
- Hang Li 1 na1 ,
- JuLan Guo 1 , 2 na1 ,
- ZhiRong Ren 1 , 3 na1 ,
- Dingxi Bai 1 ,
- Jing Yang 1 ,
- Wei Wang 1 ,
- Qing Yang 1 ,
- Chaoming Hou 1 &
- Jing Gao 1
BMC Nursing volume 23 , Article number: 530 ( 2024 ) Cite this article
71 Accesses
Metrics details
Moral distress occurs in daily nursing work and plagues nurses. Improving the level of moral courage is one of the main strategies to reduce moral distress, and low levels of moral courage may lead to nurse burnout, increased turnover, and reduced quality of care.
Nine electronic databases in Chinese and English were searched for the level of moral courage among nurses, including PubMed, Web of Science, EMBASE, CINAHL, CNKI, Wan fang, Wei pu, CBM and Cochrane Library, for the period from the date of database creation to April 5, 2023. The Agency for Healthcare Research and Quality (AHRQ) was used to assess the methodological quality of the included studies, followed the Preferred Reporting Items for Systematic Reviews and Meta-Analyses (PRISMA) guidelines and the Meta-analysis and Systematic Reviews of Observational Studies guidelines, and data from the included studies were meta-analyzed in STATA version 15 using a fixed-effects model.
Seventeen cross-sectional studies of moderate or high quality met the eligibility criteria and involved 7718 nurses, and the Nurses’ Moral Courage Scale (NMCS) was used to measure the self-assessed moral courage level of nurses. Eleven of these studies reported total scores for nurses’ moral courage, and the meta-analysis results showed a pooled mean score of 78.94 (95% CI: 72.17, 85.72); Fourteen studies reported mean entry scores for nurses’ moral courage, and the meta-analysis results showed a pooled mean score of 3.93 (95% CI: 3.64, 4.23).
The results of the meta-analysis showed that nurses’ moral courage levels were in the medium to high range, among the nurses who seemed to be male, non-nursing managers, high school education, had not experienced ethical issues, and considering resignation had lower levels of moral courage. The results of the meta-analysis may provide some reference for nursing managers and even hospital administrators to develop strategies to optimize nursing quality.
Peer Review reports
Introduction
Nursing is embedded in ethical and moral concerns [ 1 , 2 ], The survey showed that 67.7% of nurses experience moral distress [ 3 ], and that distress is more frequent and more serious for nurses than for other healthcare workers because they have more contact time with patients, so the frequency of moral distress is relatively high [ 4 ], especially during disease pandemics, which creat more ethical issues and distress for nurses, increasing their moral suffering [ 5 , 6 ]. Research suggests that moral distress negatively affects nurses, for example, when nurses are in a chronic moral distress, it decreases their job satisfaction and increases turnover rates [ 7 ]; it can also lead to empathic fatigue [ 8 ], burnout [ 9 ], and an increased rate of medication errors among nurses [ 10 ].Therefore healthcare organizations must recognize the negative effects of moral distress on nurses and take proactive measures in order to mitigate its impact on both individuals and patient outcomes.
Improving the level of moral courage is one of the main strategies to reduce the frequency of moral distress [ 11 ]. Moral courage is the courage to act in accordance with moral principles in the face of moral conflict, even though one may experience negative consequences [ 12 ], and in the field of nursing, moral courage defined as the nurse’s ability to adhere to professional ethical guidelines and to act in strict compliance with those guidelines, even if there is a foreseeable or real negative impact on yourself as a result [ 13 ]. Research [ 14 ]shows that nurses with higher level of moral courage experience lower frequencies of moral distress. High level of moral courage enables nurses to effectively respond to challenging situations and uphold their professional values. Additionally, high moral courage enables them to openly oppose unethical practices, protect patients’ rights and make the right decisions. Low level of moral courage may lead to nurses being unable to adhere to ethical principles, leading to an increase in the frequency of moral distress, thereby reducing the quality of care, and ultimately leading to unethical behavior [ 15 ]. As the backbone of the healthcare system, nurses require a supportive environment to meet their needs [ 14 ].
Encouragingly, scholars are increasingly paying attention to nurses’ current level of moral courage. Therefore, the number of studies on this topic is gradually increasing. However, it is worth noting that there is a wide range of opinions regarding the level of moral courage exhibited by nurses. Tang et al. [ 16 ] surveyed 331 psychiatric nurses in a hospital in Henan Province and the study showed that the moral level of nurses was at a higher level. Other studies have reached different conclusions, for example, Gan et al. [ 17 ] surveyed 368 junior nurses in a hospital in Harbin and showed that nurses’ moral courage was at a moderate to low level, and Nora Hauhio et al. [ 18 ] surveyed 482 registered nurses in a hospital in Finland and showed that nurses’ moral courage was at a moderate to high level, which may be related to the survey area, sample size, and the nurse’s work environment, work experience, and education level [ 13 , 19 , 20 ]. Although different studies have drawn different opinions and conclusions, one thing is still certain - nurses are an indispensable part of maintaining ethical standards in the medical field. Their role cannot be overemphasized, as they are often at the forefront of patient care and promotion. Therefore, we must study the current situation of nurses’ moral courage so that we can identify areas for improvement to enhance their level of moral courage. This not only helps to reduce the ethical distress faced by nurses, but also helps to improve the overall quality of care [ 21 ].
To date, our search of major databases revealed that there are no meta-analyses of nurses’ levels of moral courage, indicating a lack of evidence-based evidence in this area. Therefore, the purpose of this review is to understand the level of moral courage of nurses by pooling studies which using NMCS.
Design and registration
The Systematic review and Meta-analysis followed the Preferred Reporting Items for Systematic Reviews and Meta-Analyses (PRISMA) guidelines [ 22 ] and the Meta-analysis and Systematic Reviews of Observational Studies guidelines [ 23 ], it can enhance the clarity and organization of reports, so the systematic reviews and meta-analysis reports will not miss important information, thus providing high-quality evidence for evidence-based decisions. This systematic review and meta-analysis have been registered on PROSPERO website (Registration number: CRD42023414565).
Search strategy
The studies were searched in nine electronic databases in English and Chinese (PubMed, Web of Science, EMBASE, CINAHL, CNKI, Wan fang, Weipu, CBM and Cochrane Library), for the period from the date of database creation to April 5, 2023. A combination of Mesh terms and free terms was used for the literature search. The Mesh terms included “Nurses”, “Nurse”, “Nursing Personnel”, “Registered Nurses”, and “Moral Courage”. To ensure the comprehensiveness of the literature search, references cited in the literature were manually searched to find the research that may be included in the literature. We will also seek the help of an experienced librarian to refine the search strategy for each database. For full text not available or only abstracts or unpublished documents, we will email the corresponding author or first author for help. (Supplementary Table 1 )
Eligibility criteria
Inclusion criteria:
The research subjects included in the study are nurses.
The Nurses’ Moral Courage Scale was developed by Numminen et al. [ 24 ] in 2018 to assess the level of moral courage.
It is a quantitative study that can extract the mean ± standard deviation of the total score of the scale or the mean ± standard deviation of the mean score of each item.
Observational studies (cross-sectional, case-control, cohort studies).
Exclusion criteria:
Unable to extract mean ± standard deviation of scale scores.
Secondary research (Meta-analysis, Systematic evaluation, Review, etc.).
Full text was not available.
Quality assessment
Since all studies included in this review are cross-sectional the Agency for Healthcare Research and Quality (AHRQ) was used to assess methodological quality [ 25 ], which is currently an excellent tool for assessing the quality of cross-sectional studies [ 26 ]. It is also one of the widely accepted tools for assessing the quality of cross-sectional studies, and the AHRQ is available at http://www.ncbi.nlm.nih.gov/books/NBK35156/ [ 27 ]. The AHRQ has 11 items and assigns a score of 1 when assessing individual items for “yes” and 0 points otherwise. The total score is 0 to 3 for low quality, 4 to 7 for moderate quality, and 8 to 11 for high quality. This study quality was assessed by the LH reviewer and then checked by the reviewer BDX, and any discrepancies were resolved through discussion. (Supplementary Table 2 )
Data extraction
Two researchers (LH and FH) independently selected the literature in EndNote X9, extracted the data, and cross-checked according to search strategies and inclusion criteria. In case of any disagreement, a third researcher (BDX) was consulted for resolution. The main data were extracted in Microsoft Office Excel, including: Study, Country, Study design, Total sample, Number of Male, Number of Female, Age, Moral courage score, Average score of entries. (Table 1 )
Data synthesis
All included studies used a consistent measurement instrument, so meta-analysis was used to synthesize the quantitative data. Mean scores and standard deviations of NMCS scale scores were pooled across studies using Stata15 software, and the pooled mean scores were expressed as weighted effect sizes and 95% confidence intervals (CI). Between-study heterogeneity was assessed using the Cochran Q chi-square test and the I 2 statistic, with I 2 values of 25%, 50%, and 75% for low, moderate, and high heterogeneity, respectively. When I 2 > 50% and p < 0.05, moderate or high heterogeneity was indicated and a random effects model was used for analysis; otherwise, a fixed effects model was used [ 28 ]. In addition, pre-defined subgroup analyses were used to explore the effects of gender, whether or not they were nurse leaders, education level, on the level of nurse ethics, and whether or not they were experiencing ethical problems or related knowledge. Egger’s test was used to identify publication bias, with P > 0.05 indicating a low likelihood of publication bias [ 29 ]. If publication bias exists, correction is made by haircutting.
Study screening & selection process
417 literature were obtained through database search, 3 literature obtained by tracing the included references, obtained a total of 420 literature. According to the inclusion and exclusion criteria, 181 obviously irrelevant literature were excluded from the initial screening; after reading the full text and re-screening, 40 literature with inconsistent study subjects, study content, study design, outcome indicators, non-English and Chinese, non-accessible full text were excluded, and 17 [ 16 , 17 , 18 , 30 , 31 , 32 , 33 , 34 , 35 , 36 , 37 , 38 , 39 , 40 , 41 , 42 , 43 ] citations were finally included. (Fig. 1 )
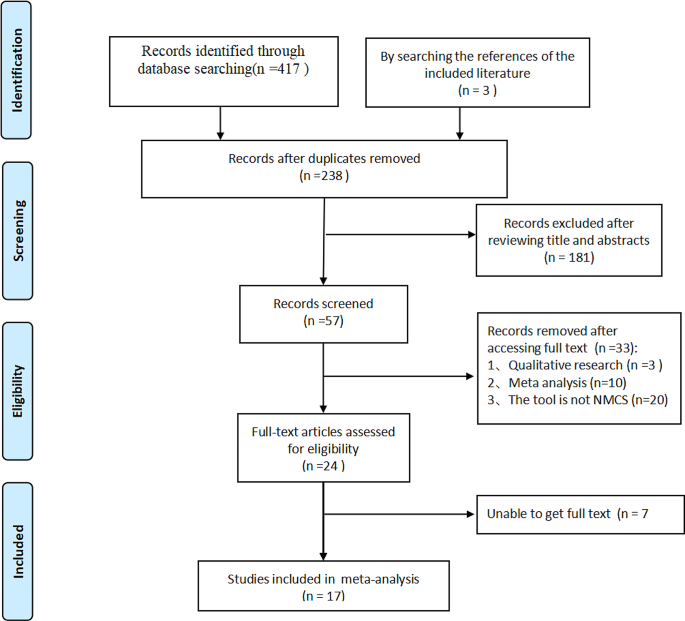
The literature screening flow chart
Study description
A total of seventeen studies involving 7718 nurses were included in this review, eleven of these studies reported total scores for nurses’ moral courage, fourteen studies reported mean entry scores for nurses’ moral courage, we separately merged the mean standard deviation of the total score of moral courage level and the average score of each item to better review the current status of nurses’ moral courage level. The included studies were all published between 2020 and 2023; a few studies (n = 5) were conducted in European countries (Finland, Turkey, Belgium), while the majority (n = 11) were conducted in China, and all studies were cross-sectional. The included studies all used the Nurse Moral Courage Scale developed by Numminen et al [ 24 ]. The scale consists of 21 items in 4 dimensions, namely moral integrity (7 items), commitment to good care (5 items), compassion and true presence (5 items), and moral responsibility (4 items). The Likert 5-point scale was used, with scores ranging from 1 to 5 on a scale of “not at all consistent with me” to “completely consistent with me”, and scores ranging from 21 to 105. Thirteen of the studies further reported mean scores and standard deviations for their four dimensions. (Table 1 )
Nurses’ moral courage
Eleven of these studies reported total scores for nurses’ moral courage, Fourteen studies reported mean entry scores for nurses’ moral courage, and the meta-analysis found that the total scores for nurses’ moral courage ( Q = 4.00, I 2 = 0.0%, p = 0.947), mean entry scores for nurses’ moral courage ( Q = 4.07, I 2 = 0.0%, p = 0.99), and fixed-effects models were used to pool effect sizes. The meta-analysis results showed a pooled mean score were 78.94 (95% CI: 72.17, 85.72), 3.93 (95% CI: 3.64, 4.23). (Figures 2 and 3 )
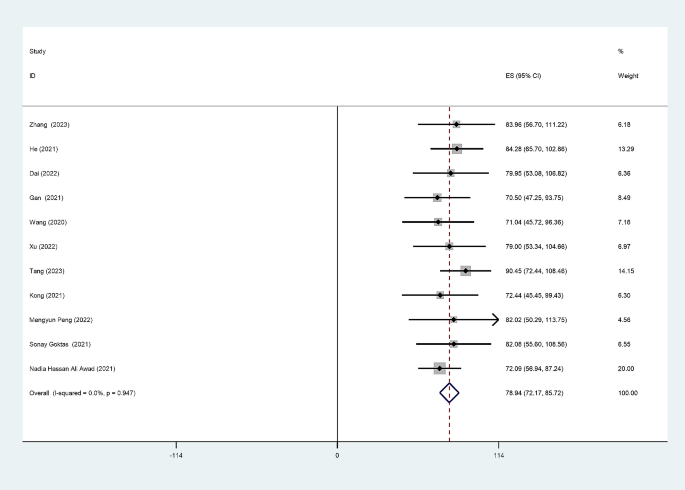
Forest plot of pooled mean scores for total score of moral courage level
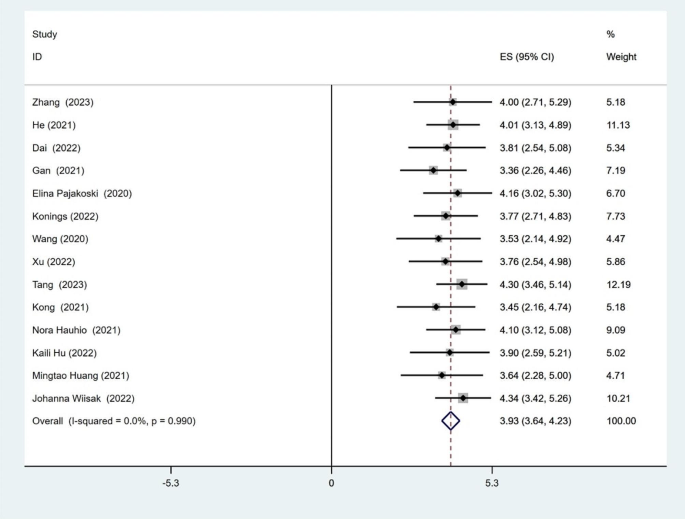
Forest plot of pooled mean scores for average score of entries
A total of thirteen studies were included for the analysis of the four dimensions. The meta-analysis found that compassion and true presence ( Q = 4.16, I 2 = 0.0%, p = 0.998), commitment to good care ( Q = 4.63, I 2 = 0.0%, p = 0.969), moral integrity ( Q = 2.81, I 2 = 0.0%, p = 0.997), and moral responsibility ( Q = 2.65, I 2 = 0.0%, p = 0.998) was homogeneous, and fixed-effects models were used to pool effect sizes, with a pooled mean scores were 3.84 (95% CI: 3.46, 4.21), 3.76 (95% CI: 3.40, 4.12), 3.89 (95% CI: 3.54, 4.24), and 3.84 (95% CI: 3.46, 4.21) respectively. (Supplementary Figs. 1 – 4 )
Subgroup analyses of moral courage for nurses
The subgroup analysis revealed relatively high level of moral courage among female nurses, nurses with higher education, nurse leaders, nurses who had experienced moral issues or were knowledgeable about them, and nurses who had never considered leaving their jobs. (Table 2 )
Quality appraisal
Eight of the seventeen cross-sectional studies had High methodological quality (AHRQ scores of 8), and nine had moderate methodological quality (AHRQ scores of 6–7). The risk of bias for included studies was mainly from item 2 (The inclusion and exclusion criteria for exposed and unexposed subjects were not listed, or reference was made to previous publications), item 7 (No explanation was given for any patients excluded from the analysis), item 9 (There was no explanation on how to handle missing data in the analysis) and item 11 (The percentage of patients who did not have clear expected follow-up and did not receive incomplete data or follow-up), and all included studies were included in the meta-analysis because they were of moderate to high quality. (Supplementary Table 2 )
Sensitivity analysis/ risk of publication bias
The funnel plot distribution is symmetrical (Figs. 4 and 5 ), and sensitivity analysis revealed no significant differences between the results and the overall comprehensive estimate, indicating that the meta-analysis findings are relatively stable and reliable (Supplementary Figs. 5 – 6 ). Egger’s test result was 0.533 ( p = 0.993) for the total score of moral courage level for nurses. Therefore, there was no significant publication bias. Egger’s test result was 0.042 ( p = 0.009) for the mean entry scores for nurses’ moral courage level, in this regard, we performed the cut-and-patch method and the results showed that P = 0.99. (Supplementary Figs. 7 – 9 )
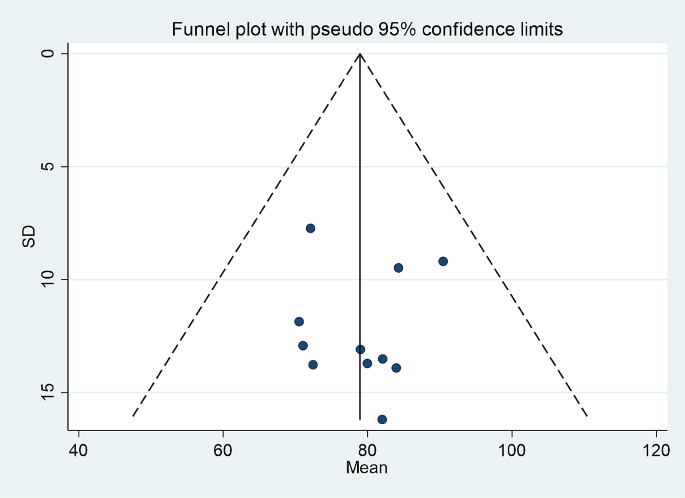
The publication bias in the estimated aggregate average score of moral courage evaluated by the funnel plot
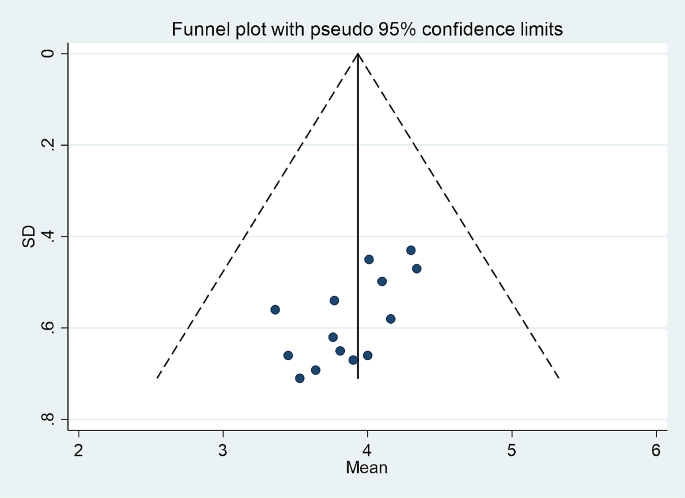
The publication bias of the average score of moral courage items evaluated through a funnel plot
The Agency for Healthcare Research and Quality (AHRQ) has recommended quality evaluation criteria for observational studies [ 25 ], which assess the risk of bias in 5 domains: selection bias, implementation bias, follow-up bias, measurement bias, and reporting bias. In the cross-sectional studies included in our review, their scores range from 6 to 8, indicating a higher quality of inclusion in the study, the main problem was that the studies lacked exclusion criteria, did not explain the reasons for excluding patients, and did not explain how the analysis handled the missing data. Studies that are rated as high quality are mainly due to their emphasis on sample size and study quality, meaning that they describe any assessments performed to ensure quality and explain the reasons for excluding any patients from the analysis. Therefore, future researchers should pay attention to the above problems when conducting cross-sectional studies.
To our knowledge, this is the first quantitative meta-analysis of nurses’ level of moral courage. In our meta-analysis, we analyzed the four dimensions of the Nurses’ Moral Courage Scale and found that mean scores of all four dimensions were in the moderate to high range. Subgroup analyses to further explore how gender, level of education, ethical experience and related knowledge, and whether resignation was considered affected nurses’ levels of moral courage. By doing so, we aimed to provide a more nuanced understanding of this critical aspect of nursing practice.
This review find that the level of moral courage of nurses is at a medium to high range, the results of this review are similar to the findings of Dai [ 32 ] and Xu [ 36 ]. This is an encouraging finding as it suggests that many nurses possess the necessary qualities to provide exceptional care for their patients. The four areas of nurses’ moral courage are moral responsibility, compassion and true presence, moral integrity and commitment to good care. These are important components of effective nursing practices, reflecting a deep commitment to the profession. However, there is always room for improvement. Although the current level of moral courage among nurses is commendable, we believe that with the continuous efforts and support of healthcare organizations, this can be further strengthened. By creating an environment that encourages ethical decision-making and prioritizes patient centered care, we can take our nurses to new heights of excellence. In summary, although there is still work to be done to comprehensively improve the moral courage level of nurses, this review provides optimistic reasons for the future of nursing practice.
With continuous attention to these key areas, including moral responsibility, compassion and true presence, moral integrity and commitment to good care, we can continue to build a good medical system.When the dimensions were analyzed and compared, the highest scores were found for moral integrity, Similar to the results of the study by Hu et al. [ 39 ].Which focuses on adherence to the basic principles and values of the profession and health care, especially in situations where there is a risk of negative consequences for others [ 44 ]. The fact that nurses scored high on this dimension indicates their unwavering commitment to upholding ethical guidelines and demonstrates their courage and ability to act accordingly; commitment to good care is relatively low, similar to the results of Xu et al. [ 36 ], Koning et al. [ 34 ]. The main content of this dimension refers to nurses’ courage to defend the good rights of patients in the case of insufficient resources or professional competence, compromise or coercive practices that threaten the good care of patients [ 44 ]. The low score of this dimension indicates that nurses’ courage to defend the good rights of patients in the case of insufficient resources or professional competence, compromise or coercive practices that threaten the good care of patients Inadequate.
Interestingly, we found that female nurses exhibit higher moral courage than male nurses. This discovery led us to explore the potential reasons for this disparity, assuming that it may be related to the professional identity of male nurses. Our analysis reveals a positive correlation between professional identity and job engagement, indicating that those with stronger professional identity are more likely to participate in their work [ 45 ]. However, we also found that male nurses with lower professional identity often exhibit less work enthusiasm, which in turn affects their moral courage. This is an important insight as it emphasizes the need for healthcare organizations to cultivate strong professional identities among all staff, especially male nurses, who may face unique challenges in establishing themselves in a predominantly female field. In a study on the moral courage level of Argentine doctors, we also found that men have lower level of moral courage than women [ 46 ]. Subgroup analysis revealed that the higher the level of education, the higher the level of moral courage, which may be related to the fact that nurses with higher education have a higher level of professional knowledge and a better judgment of the treatment and care plan for patients; Meanwhile, our subgroup analysis revealed a large difference in the level of moral courage between nurse leaders and clinical nurses. This may be related to the fact that the professional role of the nurse leader needs to deal with complex nurse-patient and health care relationships on a daily basis, and that he or she has a wider range of interactions at work, has more power, and thus has relatively higher moral courage [ 32 ]; Compared to nurses who have experienced moral distress and related knowledge, inexperienced nurses have relatively low levels of moral courage, which may increase with work experience, repeated confrontation with moral challenges, and learning from this may increase with experience, repeated confrontation with ethical challenges, and learning from ethical behavior [ 47 ], for example, the relatively high level of moral courage among nurses compared to graduating nursing students may be related to the environment in which the graduating nurses are placed and their age. Clinical nurses often encounter moral dilemmas in their work, which may be associated with their increasing level of moral courage as they gain experience [ 48 ]; The higher level of moral courage among nurses who had never considered leaving compared to those who had considered leaving may be related to job dissatisfaction among nurses who considered leaving, this result is similar to the view of Khodaveisi M et al. [ 15 ].
Overall, nurses have played a valuable role in promoting ethical practices in the medical environment. They are firmly committed to upholding ethical principles, which not only benefits individual patients but also contributes to building a more just and equitable medical environment. This review suggests that the moral courage level of nurses still needs to be further improved. Therefore, it is imperative that nursing managers and hospital administrators recognize the crucial role of moral courage in the nursing profession. Nurses are often faced with moral distress that require them to make difficult decisions, and having a high level of moral courage can greatly impact their ability to act ethically. To this end, we recommend that senior nurses take an active role in mentoring junior nurses and providing guidance on how to navigate complex ethical situations. By sharing their own experiences and offering support, they can help prevent junior nurses from encountering similar challenges in the future. Additionally, experienced nurses should be encouraged to lead ethics lectures and discussions within their departments. This will not only improve the moral sensitivity of all nurses but also foster a culture of open communication where ethical concerns can be addressed openly and honestly [ 49 ]. Ultimately, by prioritizing the development of moral courage among its nursing staff, hospitals can ensure that patients receive care that is both compassionate and ethically sound.
Limitations
There are certain limitations to this review. First, the included studies were cross-sectional in design, therefore, no causal relationship can be inferred from the observed association and inevitably had design flaws. Second, the scales we included were patient self-reported outcome scales, which are somewhat subjective. Third, we did not search the gray literature base and may have missed those unpublished papers. Fourth, in the meta-analysis, scales not developed by Numminen et al. were excluded, which may bias the integration results. Finally, more of the included studies were conducted in China ( n = 11), thus, the scope of our study may have been limited.
Clinical implication
This meta-analysis delves into the key topic of nurses’ moral courage. By incorporating relevant literature, this review reveals the current status of nurses’ moral courage level and provides valuable insights for nursing managers and hospital managers. The findings of this meta-analysis have profound implications for healthcare organizations. By better understanding the factors that contribute to moral courage, hospitals can develop effective management strategies to improve ethical practices and strengthen patient care. A key suggestion is to create a positive work environment that supports professional ethics. When nurses feel supported by colleagues and superiors, they are more likely to demonstrate moral courage in challenging situations. Conversely, it can also provide better care for patients and improve the overall quality of care [ 50 ]. In addition to creating a supportive workplace culture, hospitals should also prioritize providing relevant training and education around ethical issues. Overall, this meta-analysis represents an important step forward in understanding the moral courage of nurses. By taking action based on these findings, hospitals can create a more ethical workplace culture that benefits both patients and nurses.
This review find that the level of moral courage of nurses is at a medium to high range, the level of moral courage was lower among nurses who were male, non-nursing managers, had lower education, had not experienced ethical issues, and were considering resignation. These subgroup analysis results indicate that there is still room for improvement in cultivating an environment where all nurses have the right to act on behalf of the best interests of patients. So it is recommended that nursing managers as well as hospital administrators take appropriate measures to create a good working environment for nurses and improve their level of moral courage in order to improve the quality of care.
Data availability
All the data are available from the corresponding author up on a reasonable request.
Haahr A, Norlyk A, Martinsen B, Dreyer P. Nurses experiences of ethical dilemmas: a review. Nurs Ethics. 2020;27(1):258–72.
Article PubMed Google Scholar
Jena LK, Sarkar J, Goyal S. Sense of courage: the mediating role of courage between emotional reflexivity and work-life integration among nurses in Indian hospitals. Int J Nurs Sci. 2021;8(3):318–24.
PubMed PubMed Central Google Scholar
Grosek Š, Kučan R, Grošelj J, Oražem M, Grošelj U, Erčulj V, Lajovic J, Borovečki A, Ivanc B. The first nationwide study on facing and solving ethical dilemmas among healthcare professionals in Slovenia. PLoS ONE. 2020;15(7):e0235509.
Article CAS PubMed PubMed Central Google Scholar
Hally SM, Settle M, Nelson BD. Relationship between Moral distress and intent to leave a position among neonatal intensive care nurses. Adv Neonatal Care. 2021;21(6):E191–8.
Huang L, Lei W, Xu F, Liu H, Yu L. Emotional responses and coping strategies in nurses and nursing students during Covid-19 outbreak: a comparative study. PLoS ONE. 2020;15(8):e0237303.
Lake ET, Narva AM, Holland S, et al. Hospital nurses’ moral distress and mental health during COVID-19. J Adv Nurs. 2022;78(3):799–809.
Ulrich C, O’Donnell P, Taylor C, Farrar A, Danis M, Grady C. Ethical climate, ethics stress, and the job satisfaction of nurses and social workers in the United States. Soc Sci Med. 2007;65(8):1708–19.
Article PubMed PubMed Central Google Scholar
Maiden J, Georges JM, Connelly CD. Moral distress, compassion fatigue, and perceptions about medication errors in certified critical care nurses. Dimens Crit Care Nurs. 2011 Nov-Dec;30(6):339–45.
Mason VM, Leslie G, Clark K, Lyons P, Walke E, Butler C, Griffin M. Compassion fatigue, moral distress, and work engagement in surgical intensive care unit trauma nurses: a pilot study. Dimens Crit Care Nurs. 2014 Jul-Aug;33(4):215–25.
Malliarou M, Nikolentzos A, Papadopoulos D, Bekiari T, Sarafis P. ICU nurse’s Moral Distress as an Occupational Hazard threatening Professional Quality of Life in the time of Pandemic COVID 19. Mater Sociomed. 2021;33(2):88–93.
Namadi F, Shahbaz A, Jasemi M. Nurses’ lived experiences of Moral courage inhibitors: a qualitative descriptive study. SAGE Open Nurs. 2023;9:23779608231157326.
Jiang Fubin, Wang Zhen. A review of research on the formation and effects of moral courage in the workplace. J Manage 2023,20(01):149–58.
Numminen O, Repo H, Leino-Kilpi H. Moral courage in nursing: a concept analysis. Nurs Ethics. 2017;24(8):878–91.
Safarpour H, Ghazanfarabadi M, Varasteh S, Bazyar J, Fuladvandi M, Malekyan L. The Association between Moral Distress and Moral Courage in nurses: a cross-sectional study in Iran. Iran J Nurs Midwifery Res. 2020;25(6):533–8.
Khodaveisi M, Oshvandi K, Bashirian S, Khazaei S, Gillespie M, Masoumi SZ, Mohammadi F. Moral courage, moral sensitivity and safe nursing care in nurses caring of patients with COVID-19. Nurs Open. 2021;8(6):3538–46.
Tang YC, Wang CS, Zuo ZM, Lv LP, Zhu WZ, Tian YP. Analysis of the current situation and influencing factors of moral courage among psychiatric nurses[J]. Gen Pract Nurs. 2023;21(06):848–52.
Google Scholar
Gan L, Li H, Liu XQ. A study on the current situation and influencing factors of professional deviant behaviors of junior nurses[J]. J Nurs 2021,36(22):12–5.
Hauhio N, Leino-Kilpi H, Katajisto J, Numminen O. Nurses’ self-assessed moral courage and related socio-demographic factors. Nurs Ethics 2021 Nov-Dec;28(7–8):1402–15.
Escolar-Chua RL. Moral sensitivity, moral distress, and moral courage among baccalaureate Filipino nursing students. Nurs Ethics. 2018;25(4):458–69.
Pajakoski E, Rannikko S, Leino-Kilpi H, Numminen O. Moral courage in nursing - an integrative literature review. Nurs Health Sci. 2021;23(3):570–85.
Santos RPD, Garros D, Carnevale F. Difficult decisions in pediatric practice and moral distress in the intensive care unit. Rev Bras Ter Intensiva 2018 Apr-Jun;30(2):226–32.
Page MJ, McKenzie JE, Bossuyt PM, Boutron I, Hoffmann TC, Mulrow CD et al. The PRISMA 2020 statement: an updated guideline for reporting systematic reviews BMJ. 2021; 372:n71.
Stroup DF, Berlin JA, Morton SC, Olkin I, Williamson GD, Rennie D, Moher D, Becker BJ, Sipe TA, Thacker SB. Meta-analysis of observational studies in epidemiology: a proposal for reporting. Meta-analysis of Observational studies in Epidemiology (MOOSE) group. JAMA. 2000;283(15):2008–12.
Article CAS PubMed Google Scholar
Numminen O, Katajisto J, Leino-Kilpi H. Development and validation of Nurses’ Moral Courage Scale. Nurs Ethics 2019 Nov-Dec;26(7–8):2438–55.
Rostom A, Dubé C, Cranney A, Saloojee N, Sy R, Garritty C, Sampson M, Zhang L, Yazdi F, Mamaladze V, Pan I, McNeil J, Moher D, Mack D, Patel D. Celiac disease. Evid Rep Technol Assess (Summ). 2004;(104):1–6.
Zeng X, Zhang Y, Kwong JS, Zhang C, Li S, Sun F, Niu Y, Du L. The methodological quality assessment tools for preclinical and clinical studies, systematic review and meta-analysis, and clinical practice guideline: a systematic review. J Evid Based Med. 2015;8(1):2–10.
Rostom A, Dubé C, Cranney A et al. Celiac Disease. Rockville (MD): Agency for Healthcare Research and Quality (US); 2004 Sep. (evidence Reports/Technology assessments, No. 104.) Appendix D. Quality Assessment Forms.
Higgins JP, Thompson SG, Deeks JJ, Altman DG. Measuring inconsistency in meta-analyses. BMJ. 2003;327(7414):557–60.
Egger M, Davey Smith G, Schneider M, Minder C. Bias in meta-analysis detected by a simple, graphical test. BMJ. 1997;315(7109):629–34.
Zhang YD, Du AP, Tian Y. Analysis of the current situation and influencing factors of moral courage of ICU nurses[J]. J Nurs 2023,38(02):51–4.
He J, Yang X, Xia HY, Xiang XY, Han WL, Wang DH. Analysis of the current situation and influencing factors of moral courage among 693 clinical nurses in a secondary hospital[J]. J Nurs 2021,28(18):41–5.
Dai HM, Li Y, Wu HY. Study on the correlation between moral courage and moral dilemma of nursing staff [J]. J Nurs. 2022;37(21):53–7.
Pajakoski E, Rannikko S, Leino-Kilpi H, Eliisa, Löyttyniemi. Olivia Numminen. Nurses’ moral courage in Finnish older people care: a cross-sectional study[J]. Nordic J Nurs Res. 2023;43(1):20571585231162807.
Konings KJ, Gastmans C, Numminen OH, Claerhout R, Aerts G, Leino-Kilpi H, de Casterlé. BD. Measuring nurses’ moral courage: an explorative study. Nurs Ethics. 2022;29(1):114–130.
Wang SY, Jiang WB, Liu C, Shang QW, Yang HP, Zhang Y, Wei LL. A study on the moral courage of nurses and its influencing factors [J]. Chin J Nurs Educ. 2022;19(08):732–6.
Xu J, Han L, Wang J, Pao YJ, Deng JQ, Huang ZW. Analysis of moral courage and influencing factors of psychiatric nurses [J]. J Nurs. 2022;37(16):51–3.
Linshu K, Jingyan W, Lina D, et al. Investigation on the Moral courage of clinical nurses in geriatric hospitals [J]. J Hosp Manage People’s Liberation Army. 2021;28(04):383–5.
Peng M, Saito S, Guan H, Li B. Moral distress, moral courage, and career identity among nurses: a cross-sectional study. Nurs Ethics 2022 Dec 22:9697330221140512.
Hu K, Liu J, Zhu L, Zhou Y. Clinical nurses’ moral courage and related factors: an empowerment perspective. BMC Nurs. 2022;21(1):321.
Goktas S, Aktug C, Gezginci E. Evaluation of moral sensitivity and moral courage in intensive care nurses in Turkey during the COVID-19 pandemic. Nurs Crit Care. 2023;28(2):261–71.
Ali Awad NH, Al-Anwer Ashour HM. Crisis, ethical leadership and moral courage: ethical climate during COVID-19. Nurs Ethics. 2022;29(6):1441–56.
Huang M, Dong W, Zhao Q, Mo N. Factors associated with the moral courage of nurses in China: a cross-sectional study. Nurs Open. 2023 Feb 22.
Wiisak J, Suhonen R, Leino-Kilpi H. Whistle-blowers - morally courageous actors in health care? Nurs Ethics. 2022;29(6):1415–29.
Wang SY, Wei LL, Zhang Y, Li T, Jiang WB, Yang HP, Chen K, Wang SY, Chen QQ. Sinicization and reliability testing of the Nurse Moral courage Scale[J]. J Nurs 2019,34(21):92–5.
Wu C, Fu MM, Cheng SZ, Lin YW, Yan JR, Wu J, Zhang XY, Cao BH, Du J, Lang HJ. Career identity and career success among Chinese male nurses: the mediating role of work engagement. J Nurs Manag. 2022;30(7):3350–9.
Borracci RA, Ciambrone G, Gallesio JMA. Correlation between moral courage score and social desirability score of the medical residents and fellows in Argentina. J Educ Eval Health Prof. 2020;17:6.
Ren P, Yin F, Jiang XR, Luo YY, An D. Research progress of moral courage of nurses[J]. Gen Pract Nurs 2021,19(35):4951–4.
Koskinen S, Pajakoski E, Fuster P, Ingadottir B, Löyttyniemi E, Numminen O, Salminen L, Scott PA, Stubner J, Truš M, Leino-Kilpi H, ProCompNurse Consortium. Analysis of graduating nursing students’ moral courage in six European countries. Nurs Ethics. 2021;28(4):481–97.
Ko HK, Tseng HC, Chin CC, Hsu MT. Phronesis of nurses: a response to moral distress. Nurs Ethics. 2020;27(1):67–76.
Fradelos C, Alexandropoulou E, Kontopoulou CA, Alikari L, Papagiannis V, Tsaras D, Papathanasiou K. The effect of hospital ethical climate on nurses’ work-related quality of life: a cross-sectional study. Nurs Forum. 2022;57(2):244–51.
Article Google Scholar
Download references
Acknowledgements
We would like to express our gratitude to all respondents included in this meta-analysis.
The author did not receive any financial support in writing or publishing the article.
Author information
Hang Li, JuLan Guo, and ZhiRong Ren contributed equally to this work.
Authors and Affiliations
Chengdu University of Traditional Chinese Medicine, Chengdu, Sichuan, China
Hang Li, JuLan Guo, ZhiRong Ren, Dingxi Bai, Jing Yang, Wei Wang, Han Fu, Qing Yang, Chaoming Hou & Jing Gao
Hospital of Chengdu University of Traditional Chinese Medicine, Chengdu, Sichuan, China
The Affiliated Fifth People’s Hospital of Chengdu University of Traditional Chinese Medicine, Chengdu, Sichuan, China
ZhiRong Ren
You can also search for this author in PubMed Google Scholar
Contributions
1.Hang Li: Topic concept, literature search, data analysis, and article writing. 2.JuLan Guo: Topic concept, language check, and Revised guidance. 3.ZhiRong Ren: Topic concept, PRISMA and MOOSE checklist combed, and Revised guidance. 4.Dingxi Bai: Topic concept, literature search, data analysis, and article writing. 5.Jing Yang: Topic concept, literature search, data analysis, and article writing. 6.Wei Wang: Data processing, analyzing data. 7.Han Fu: Data processing, analyzing data. 8.Qing Yang: Data processing, analyzing data. 9.Chaoming Hou: Revise and review articles. 10.Jing Gao: Revise and review articles.
Corresponding authors
Correspondence to Chaoming Hou or Jing Gao .
Ethics declarations
Ethics approval and consent to participate.
Not applicable.
Consent for publication
Conflict of interest.
The authors declare that they have no competing interests in this paper’s publication.
Additional information
Publisher’s note.
Springer Nature remains neutral with regard to jurisdictional claims in published maps and institutional affiliations.
Electronic supplementary material
Below is the link to the electronic supplementary material.
Supplementary Material 1
Rights and permissions.
Open Access This article is licensed under a Creative Commons Attribution 4.0 International License, which permits use, sharing, adaptation, distribution and reproduction in any medium or format, as long as you give appropriate credit to the original author(s) and the source, provide a link to the Creative Commons licence, and indicate if changes were made. The images or other third party material in this article are included in the article’s Creative Commons licence, unless indicated otherwise in a credit line to the material. If material is not included in the article’s Creative Commons licence and your intended use is not permitted by statutory regulation or exceeds the permitted use, you will need to obtain permission directly from the copyright holder. To view a copy of this licence, visit http://creativecommons.org/licenses/by/4.0/ . The Creative Commons Public Domain Dedication waiver ( http://creativecommons.org/publicdomain/zero/1.0/ ) applies to the data made available in this article, unless otherwise stated in a credit line to the data.
Reprints and permissions
About this article
Cite this article.
Li, H., Guo, J., Ren, Z. et al. Moral courage level of nurses: a systematic review and meta-analysis. BMC Nurs 23 , 530 (2024). https://doi.org/10.1186/s12912-024-02082-w
Download citation
Received : 24 July 2023
Accepted : 10 June 2024
Published : 02 August 2024
DOI : https://doi.org/10.1186/s12912-024-02082-w
Share this article
Anyone you share the following link with will be able to read this content:
Sorry, a shareable link is not currently available for this article.
Provided by the Springer Nature SharedIt content-sharing initiative
- Moral courage
- Moral distress
- Meta-analysis
BMC Nursing
ISSN: 1472-6955
- General enquiries: [email protected]
- Medical Science Nursing
Advancing nursing education in Brazil: A systematic literature review of curricular evolution and emerging challenges
- Nurse Education Today 139(2):106228
- 139(2):106228

- Federal University of Minas Gerais
- This person is not on ResearchGate, or hasn't claimed this research yet.
Discover the world's research
- 25+ million members
- 160+ million publication pages
- 2.3+ billion citations
No full-text available

To read the full-text of this research, you can request a copy directly from the authors.

- Mauricio Viana Gomes de Oliveira

- Int J Consum Stud

- Stefano Bresciani

- María Antonia Jiménez-Gómez
- María Dolores Bardallo-Porras
- Vilanice Alves de Araújo-Püschel
- Camila Ester Fuentes Olmos
- Jeferson Rodrigues

- Taka Oguisso
- Almerinda Moreira

- REV LAT-AM ENFERM
- Silvia Helena De Bortoli Cassiani

- Nathaly Rozo Gutiérrez

- Rosa Zarate

- REV ESC ENFERM USP
- Cilene Aparecida Cosíardi Ide

- Regina Aurora Trino Romano
- Luiza Maria Piazzi Papa
- Gertrudes Teixeira Lopes
- Joise Magarão Queiroz Silva

- Ellen Márcia P. Peixoto
- Sonia Silva
- Maria Auxiliadora Córdova Christófaro

- Liliane Passarelli Rodrigues

- Kaneji Shiratori
- Maria Regina Lemes de Sordi
- Maria Inês Brandão Bocardi

- Kurcgant Paulina
- NURS EDUC TODAY
- Prinaz Jahan Peyma

- Beatriz de Castro Magalhães
- Caik Ferreira Silva

- Grayce Alencar Albuquerque

- ARCH PSYCHIAT NURS
- Sérgia Cristina Cavalcante Pereira

- J INFORMETR

- Silvana Sidney
- Costa Santos

- Vendrúscolo
- Recruit researchers
- Join for free
- Login Email Tip: Most researchers use their institutional email address as their ResearchGate login Password Forgot password? Keep me logged in Log in or Continue with Google Welcome back! Please log in. Email · Hint Tip: Most researchers use their institutional email address as their ResearchGate login Password Forgot password? Keep me logged in Log in or Continue with Google No account? Sign up
This paper is in the following e-collection/theme issue:
Published on 31.7.2024 in Vol 13 (2024)
Effectiveness of Mobile App Interventions to Improve Periodontal Health: Protocol for a Systematic Review and Meta-Analysis
Authors of this article:

- Reem Musa, BDS, MSc ;
- Dalia Elamin, BDS, MSc ;
- Robert Barrie, BChD, MChD, MPA, PhD ;
- Faheema Kimmie-Dhansay, BSc, BChD, PgDipl, MSc, PhD
Department of Community Dentistry, Faculty of Dentistry, University of Western Cape, Cape Town, South Africa
Corresponding Author:
Reem Musa, BDS, MSc
Department of Community Dentistry
Faculty of Dentistry
University of Western Cape
Fransie, Francie Van Zijl Dr
Cape Town, 7500
South Africa
Phone: 27 0847177976
Email: [email protected]
Background: Periodontal health plays a key role as a shared reference point for evaluating periodontal diseases and identifying significant treatment outcomes. Providing adequate instruction and enhancing the motivation of patients to maintain proper oral hygiene are crucial factors for successful periodontal treatment, with self-performed regular oral hygiene identified as a critical factor in improving the outcomes of treatment for periodontal diseases. Recently, mobile health (mHealth) solutions, especially mobile apps, have emerged as valuable tools for self-management in chronic diseases such as periodontal disease, providing essential health education and monitoring capabilities. However, the use of mHealth apps for periodontal health is complex owing to various interacting components such as patient behavior, socioeconomic status, and adherence to oral hygiene practices. Existing literature has indicated positive effects of mHealth on oral health behaviors, knowledge, attitude, practice, plaque index score, and gingivitis reduction. However, there has been no systematic review of mobile apps specifically targeting patients with periodontal disease. Understanding the design and impact of mHealth apps is crucial for creating high-quality apps.
Objective: The aim of this systematic review and meta-analysis is to evaluate the effectiveness of existing mobile apps in promoting periodontal health.
Methods: A comprehensive search strategy will be performed in multiple electronic databases (PubMed, EBSCOhost, CINAHL Plus, Dentistry & Oral Sciences, ScienceDirect, Scopus, and Cochrane Central Register of Controlled Trials) with the following keywords in the title/abstract: “mobile application,” “mobile health,” “mHealth,” “telemedicine,” “periodontal health,” “periodontitis,” and “text message.” Only randomized controlled trials will be included that assessed the following outcomes to measure periodontal health improvement: gingival index, bleeding index, periodontal pocket depth, and clinical attachment loss. Covidence will be used for data collection, and a PRISMA (Preferred Reporting Items for Systematic reviews and Meta-Analyses) flowchart will be used to describe the selection process of the included, identified, and excluded studies. The Confidence in Network Meta-Analysis approach will be used for meta-analysis of the extracted data from the included studies.
Results: This review will not require ethical approval since no primary data will be included. As of July 2024, a total of 83 articles retrieved from various databases have been imported to Covidence with 13 articles deemed eligible for inclusion in the review. The review is currently ongoing and is expected to be complete by the end of 2024 with the results published in early 2025.
Conclusions: This systematic review and meta-analysis will contribute to developing mobile apps with enhanced criteria to improve periodontal clinical outcomes. The review emphasizes the importance of mHealth and preventing periodontal disease, which can set the stage for informed global health care strategies.
Trial Registration: PROSPERO CRD42022340827; https://www.crd.york.ac.uk/prospero/display_record.php?RecordID=340827
International Registered Report Identifier (IRRID): DERR1-10.2196/50479
Introduction
Periodontal health is essential to overall oral health and well-being [ 1 ]. Periodontal health is generally defined as the absence of inflammatory periodontal disease [ 2 ], encompassing the health of the surrounding support system for the tooth, which includes the gingiva, periodontal ligament, and alveolar bone [ 3 ]. A standard definition of periodontal health is crucial for identifying a reference point to determine and assess treatment outcomes [ 2 ]. Building upon this understanding, periodontal health is classified into the following 4 levels, as proposed by Lang and Bartold [ 2 ], based on various factors such as the condition of the periodontium, the ability to control modifying factors, predisposing factors, and treatment outcomes:
- Pristine periodontal health, representing the absence of clinical inflammation, usually with no bleeding on probing, bone loss, or attachment loss involvement, making it difficult to measure clinically.
- Clinical periodontal health (intact periodontium), referring to minimal or absent gingival inflammation, which is clinically detectable with bleeding on probing.
- Periodontal disease with stability in the reduced periodontium, defined as major plaque-associated periodontal diseases such as periodontitis, with the condition being effectively treated and no apparent worsening of clinical signs despite a reduced periodontium.
- Periodontal disease in remission or under control, characterized by a reduction in the severity of symptoms, although they may not be resolved entirely.
Furthermore, the two primary periodontal conditions, gingivitis and periodontitis, play significant roles in oral health. Gingivitis, the mildest form of periodontal disease, is characterized by localized, reversible inflammation of the gingiva caused by dental plaque [ 4 ], whereas periodontitis is a microbe-associated, host-mediated inflammatory condition leading to progressive attachment loss [ 5 ]. Chronic conditions such as periodontal disease and dental caries can cause severe pain in advanced stages and may result in challenges with speaking and eating [ 6 ]. Additionally, there is a strong association between oral diseases and noncommunicable conditions such as diabetes [ 7 ] and cardiovascular disease [ 8 ].
In response to the evolving understanding of periodontal diseases, a global workshop was convened in 2017 to develop a new classification system to simplify diagnosing and treating these diseases in general dental practice [ 9 ]. This updated classification system addresses the form, severity, and extent of periodontitis and formally incorporates recognized risk factors and their associations with systemic diseases. Critical components of this classification system include staging, which categorizes the severity and extent of the disease, and grading, which predicts future risks and potential health impacts. This classification system allows for precise and focused definitions of periodontal health and gingivitis as follows: (1) patients with an intact periodontium, (2) patients with a reduced periodontium due to causes other than periodontitis, and (3) patients with a reduced periodontium due to periodontitis [ 9 ].
The previous classification was formally introduced in 1999 [ 10 ]; the notable distinction between the two classifications was the use of the term “periodontitis” instead of “aggressive and chronic periodontitis” [ 9 ]. Furthermore, smoking and diabetes were identified as major potential risk factors that could influence the staging of periodontal disease in the new classification [ 9 ].
As part of an accurate diagnosis and appropriate treatment strategy, having clear and focused definitions in a classification system is fundamental in promoting periodontal health. This understanding is vital as neglecting periodontal health can lead to significant conditions such as periodontal disease, bone loss, and ultimately tooth loss [ 10 ]. A combination of effective oral hygiene practices, routine dental examinations, and a healthy lifestyle is essential to maintain optimal periodontal health. Daily brushing and flossing help eliminate plaque, while professional scaling can remove calculus buildup [ 1 ]. The importance of effective self-performed oral hygiene in preventing oral diseases is well-established [ 11 ], including the integration of mobile health (mHealth) interventions to promote the self-management of oral health [ 12 ].
Indeed, the combination of mHealth technology in periodontal care has significantly impacted the field, offering a promising avenue for promoting oral health awareness and empowering patients to take control of their periodontal health [ 13 , 14 ]. mHealth is a medical and public health practice supported by mobile devices such as mobile phones, patient monitoring devices, personal digital assistants, and other wireless devices [ 15 ]. These mobile apps gather users’ health data, offer customized guidance, and facilitate remote patient data monitoring, thereby fostering collaborative relationships between dental professionals and individuals [ 16 ]. The increasing interest in chronic disease management has created opportunities to enhance self-management capabilities [ 17 ].
In light of these developments, Toniazzo et al [ 12 ] performed a systematic review to summarize the role of mHealth in improving oral health, with the measured outcomes being the plaque index and gingival index (GI). The review findings demonstrated a significant improvement in dental plaque and gingivitis resulting from the use of mobile apps, suggesting that these tools may enhance oral health behaviors. However, the lack of regulation during app development increases the risk of patients accessing inaccurate information [ 18 ]. Therefore, this review is designed as a preliminary step prior to the development of an mHealth-promotion app. To our knowledge, no other systematic review has been performed to specifically evaluate mobile apps targeting patients with periodontal disease.
Overall, integrating mHealth technology in periodontal care presents exciting opportunities for advancing oral health promotion and disease management. By embracing these innovations, dental professionals can enhance patient care and improve oral health outcomes while maintaining a comprehensive understanding of periodontal diseases.
Review Objective
The aim of this systematic review and meta-analysis is to evaluate the effectiveness of existing mobile apps or text messages designed to enhance periodontal health among patients compared to the outcomes of patients without any mobile app or digital intervention to support their treatment.
Study Registration and Framework
This systematic review has been registered in PROSPERO under registration number CRD42022340827.
The Participants, Interventions, Comparators, Outcomes, and Study design (PICOS) framework is used to guide the eligibility criteria [ 19 , 20 ]. Participants include all patients, regardless of age or sex, with or without periodontal disease, except those who cannot participate independently. The intervention of interest is the use of mobile apps available on Android or iOS devices and mobile phones for sending SMS text messages or web-based interventions. As comparators , this review considers studies that compare the intervention to standard or usual care such as a placebo or control group without using mobile technology. The main outcome is improvement in the periodontal health of participants based on signs of progress in the following indicators: GI, bleeding index (BI), clinical attachment loss (CAL), and periodontal probing depth (PPD). All of these periodontal clinical parameters (described in further detail below) are considered crucial for assessing periodontal health because they provide valuable information about the condition of the gingiva and surrounding tissues [ 2 ].
Outcome Measures
Gingival index.
The GI is a clinical examination tool used to assess the condition of the gingival tissue and differentiate the lesion severity. To perform the examination, the alterations in 4 areas around the tooth are located. Each area (mesial, distal, buccal, palatine, or lingual) is assigned a score ranging from 0 to 3. The overall score is calculated by summing the scores from the 4 areas and dividing the total by 4. [ 21 ]: a total score of 0 indicates a normal gingiva; a score of 1 represents mild inflammation, characterized by a slight color change and edema with no bleeding on probing; a score of 2 signifies moderate inflammation, characterized by redness and edema; and a score of 3 indicates severe inflammation marked by pronounced redness, edema, ulceration, and a tendency toward spontaneous bleeding [ 21 , 22 ].
Bleeding Index
The BI is a tool to assess and reflect the histological and bacterial factors associated with the severity of periodontal diseases [ 23 ]. The BI is typically determined using a Williams periodontal probe inserted into the gingival crevice to a depth of approximately 2 millimeters. The probe is angled at approximately 60° to the tooth’s longitudinal axis. The presence or absence of bleeding is then assessed within 30 seconds after probing [ 24 ].
Clinical Attachment Loss
CAL is one of the most important tools to measure periodontitis, which is crucial in diagnosing and managing periodontal diseases. CAL is calculated as the distance from the cementoenamel junction or the border of restoration to the bottom of the periodontal pocket [ 25 ].
Periodontal Probing Depth
PPD represents a deepened gingival sulcus around a tooth at the gingival margin due to pathological causes. The PPD is an important marker to indicate the presence of inflammation or infection and to monitor the progress of periodontal treatment [ 26 ]. The values are recorded in millimeters at 6 sites per tooth (mesio-buccal, mid-buccal, disto-buccal, mesio-lingual, mid-lingual, and disto-lingual) to measure the distance between the base of the pocket and the gingival margin [ 25 ].
Inclusion and Exclusion Criteria
All published randomized controlled trials (RCTs) published in English will be included in the review. Studies aimed at improving tooth mobility and the grey literature will be excluded from this review. There will be no limitations on the time frame for measuring the outcome of the intervention.
Search Strategy for Identification of Potential Studies
The search strategy for identifying potential studies will be designed by 3 team members (RM, DE, and FKD) to ensure a comprehensive exploration of eligible studies. This will involve performing a thorough search across various electronic databases, including PubMed, EBSCOhost, Dentistry & Oral Sciences, ScienceDirect, CINAHL Plus, and Scopus. The search will use combinations of Medical Subject Headings (MeSH) key terms in the title or abstract, including “mobile applications,” “periodontal health,” “periodontitis,” “mHealth,” “smartphone application,” and “app-based intervention.” These terms will be combined using Boolean operators such as “AND” and “OR” to capture relevant studies. Additionally, databases such as the Cochrane Central Register of Controlled Trials, International Clinical Trials Registry Platform [ 27 ], and ClinicalTrials.gov [ 28 ] will be searched. A final rerun of the search will be performed before the final meta-analysis to include any additional studies. An example of the search strategy for PubMed is provided in Multimedia Appendix 1 .
Study Selection Process
Two independent reviewers (RM and DE) will review all included articles. The selection will be based on the eligibility criteria and discrepancies will be resolved through consultation with a third reviewer (FKD). The identified articles will be imported to Covidence software [ 29 ], which is a web software system that assesses the data management process by reviewers during the systematic review process. The screening process will be performed independently by the two reviewers in 2 stages: (1) screening the abstract and (2) screening the full texts. Duplicate records will be removed automatically by Covidence.
Ongoing trials with no results will be flagged and recorded in an “Ongoing studies” table. Articles not meeting the eligibility criteria will be excluded and listed in a “Characteristics of excluded studies” table along with the reasons for exclusion. The study selection process will be described in a PRISMA (Preferred Reporting Items for Systematic reviews and Meta-Analyses) flowchart [ 30 ]. This protocol adheres to the PRISMA Protocols checklist (see Multimedia Appendix 2 ).
Data Extraction and Management
Two independent reviewers (RM and DE) will extract data into Covidence using a specific form designed for this purpose. Data categories to be extracted include the field of research, sample size, setting, authors, publication year, countries, number of participants, app name and platform, app purpose, intervention period, and outcomes. Any disagreements will be resolved through discussion between the two reviewers. In cases where disagreements are unresolved, the third reviewer (FKD) will be consulted to reach a consensus through discussion.
Assessment of Risk of Bias of Included Studies
Two independent reviewers (RM and DE) will assess the methodological quality of the included RCTs using the Cochrane Risk of Bias tool [ 31 ]. Any disagreements with quality ratings will be settled by consensus-based discussion.
The following domains for the risk of bias will be assessed for all included studies: random sequence generation (selection bias); allocation concealment (selection bias); blinding (performance bias and detection bias), separated for blinding of participants and personnel and blinding of outcome assessment; incomplete outcome data (attrition bias); selective reporting (reporting bias); and other bias [ 31 ].
The risk of bias for each domain will be categorized as high, low, or unclear. The risk of bias assessment outcomes will be documented in a table with a justification of the decision. A description of the criteria for a decision of “low risk,” “high risk,” or “unclear risk” will be documented [ 31 ].
Meta-Analysis
Measures of treatment effect.
If there is a probability that data are skewed with a fixed upper and lower limit, a “rule of thumb” calculation will be used to detect the distribution of the data. This calculation will follow the method proposed by Higgins et al [ 31 ], who suggested that if the SD doubled is greater than the mean, the assumption that the data are normally distributed cannot be made (ie, that the mean is at the center of the distribution). If the outcomes are dichotomous data, the data will be presented as risk ratios, odds ratios, or risk difference values. Data that include continuous outcomes will be presented as means or standardized mean differences. All significance tests will be judged at the 5% α level with 95% CIs. Time-to-event outcomes will be reported as hazard ratios and 95% CIs [ 31 ].
Assessment of Heterogeneity
The heterogeneity of results among studies will be interpreted by considering the variation of participant characteristics (eg, age) and trial characteristics such as randomization and allocation concealment in the different studies. Heterogeneity will be generally assessed by visually inspecting the forest plots to detect the proximity of the point estimates and the overlapping of CIs. Specifically, heterogeneity will be examined using the I ² statistic with a P value threshold of <.10, which quantifies inconsistency across studies to assess its impact on the meta-analysis. The I 2 statistic will be classified as follows: 0%-40%, negligible to minor heterogeneity; 30%-60%, moderate heterogeneity; 50%-90%, substantial heterogeneity; and 75%-100%, considerable heterogeneity.
Heterogeneity will further be investigated using sensitivity analysis. This involves assessing the robustness of the findings to different decisions or ranges of values made during the study. This process helps ascertain whether the findings are dependent on specific decisions or if they are robust and reliable [ 32 , 33 ]. If heterogeneity is found to be significant, the Mantel-Haenszel method will be used for the fixed-effects model. The random-effects model will be selected if I ²≥50% or P <.10.
Assessment of Reporting Biases
When the outcomes are reported in 10 or more studies, a funnel plot will be constructed to investigate the possibility of reporting bias according to the guidelines outlined in the Cochrane Handbook for Systematic Reviews of Interventions [ 31 ].
Confidence of Study Findings
The results of the meta-analysis will be analyzed using the Confidence In Network Meta-Analysis (CINeMA) tool if there are more than 2 interventions included in the analysis; CINeMA is a software based on the framework developed by Salanti [ 34 ] and refined by Nikolakopoulou [ 35 - 37 ]. This framework’s efficiency lies in combining direct and indirect evidence from included studies in a meta-analysis when comparing more than one intervention.
Specifically, the CINeMA framework evaluates network meta-analysis through the following 6 domains: (1) within-study bias, (2) reporting bias, (3) indirectness, (4) imprecision, (5) heterogeneity, and (6) incoherence. The evaluation of each domain determines the level of concern, categorized as having no, some, or major concerns. These judgments across the domains are combined into a single confidence rating of “high,” “moderate,” “low,” or “very low.”
CINeMA serves as an alternative to the GRADE (Grading of Recommendations Assessment, Development, and Evaluation) approach, which has traditionally been used to assess confidence in the outcomes of systematic reviews and meta-analyses [ 33 ].
Data will be narratively synthesized when a meta-analysis is unsuitable according to the nature of included studies.
Sensitivity Analysis
A sensitivity analysis will be performed to determine the possible effect of loss to follow-up on the effect estimates for the primary outcomes. The results of the sensitivity analysis will be matched to the overall findings. However, for continuous data, the sensitivity analysis will be aligned with the methods described by Ebrahim et al [ 38 , 39 ]. These methods involve 5 sources of data for imputing means for participants with missing data: (1) best mean score among intervention arms, (2) best mean score among control arms, (3) mean score of the same trial among the control arms, (4) worst mean score among intervention arms, and (5) worst mean score among control arms [ 38 ].
Dissemination of Findings and Data Sharing
All data, regardless of publication quality, will be included in the review. If study details cannot be obtained, the librarian will be consulted. If the study remains unobtainable, it will not be included in the meta-analysis.
Of the 159,214 publications identified, 83 were imported into Covidence to remove duplicates and perform the screening. This was followed by full-text reviewing and data extraction, resulting in 13 included articles. CINeMA analysis of the data is underway. This review is expected to be completed by the end of 2024, and we plan to publish the results in a peer-reviewed journal.
The significance of this systematic review and meta-analysis lies in its contribution to the development of mobile apps with enhanced criteria specifically aimed at improving periodontal clinical outcomes. To enhance the quality of new apps, a comprehensive understanding of the design and impact of mHealth apps is essential in improving treatment outcomes.
Using mHealth apps to improve periodontal health represents a complex intervention due to the numerous interacting components involved (eg, patient behaviors, socioeconomic status, and adherence to oral hygiene practices). The existing literature in this field has mainly focused on promoting oral health, hygiene, practices, and adherence to orthodontic treatment.
In a previous systematic review, Toniazzo et al [ 12 ] evaluated the effects of mobile apps on oral hygiene, revealing significant improvements in reducing dental plaque and gingivitis and in promoting better oral health behaviors. Another systematic review on the impact of digital media for promoting oral health showed improved knowledge, attitude, practice, and plaque index scores, along with overall gingivitis reduction [ 40 ].
Chen et al [ 41 ] performed a systematic review with a thematic focus on the prevention of dental caries through mobile apps aiming to improve oral hygiene, encourage adequate fluoride usage, and regulate dietary intake, while addressing adherence to orthodontic treatment. However, the authors concluded that dental caries outcomes management could have been more precise. In another systematic review and meta-analysis, Al-Moghrabi et al [ 42 ] assessed behavioral changes in orthodontic patients, covering aspects such as adherence to appliance use, oral health–related behaviors, hygiene levels, periodontal outcomes, appointment attendance, and knowledge, finding very low to moderate evidence supporting the effect of digital health; the authors thus recommended designing a well-constructed mobile app to enhance orthodontic outcomes.
Limiting our review to studies published in the English language may introduce bias. Nevertheless, we acknowledge that studies with significant findings are often published in English to enhance their visibility and citation potential. Furthermore, this review exclusively includes RCTs and we are excluding the grey literature. An important recommendation for future systematic reviews will be to assess the applicability and feasibility of the mHealth apps.
Finally, the broader implication of this systematic review and meta-analysis is its pivotal contribution to advancing the global integration of mobile apps for oral health by highlighting the positive impact of these tools on periodontal outcomes and emphasizing the importance of periodontal health management. These findings can help to enhance our understanding of mHealth interventions, elevate the quality of life among the target population, and set the stage for informed health care strategies in periodontal care.
Acknowledgments
All authors declared that they had insufficient or no funding to support open access publication of this manuscript, including from affiliated organizations or institutions, funding agencies, or other organizations. JMIR Publications provided article processing fee (APF) support for the publication of this article.
Conflicts of Interest
None declared.
Search strategy sample from PubMed.
PRIMSA-P (Preferred Reporting Items for Systematic reviews and Meta-Analyses Protocols) checklist.
- Petersen PE, Ogawa H. Strengthening the prevention of periodontal disease: the WHO approach. J Periodontol. Dec 2005;76(12):2187-2193. [ CrossRef ] [ Medline ]
- Lang NP, Bartold PM. Periodontal health. J Periodontol. Jun 2018;89(Suppl 1):S9-S16. [ CrossRef ] [ Medline ]
- Dentino AR, Kassab MM, Renner EJ. Prevention of periodontal diseases. Dent Clin North Am. Jul 2005;49(3):573-594. [ CrossRef ] [ Medline ]
- Pihlstrom BL, Michalowicz BS, Johnson NW. Periodontal diseases. Lancet. Nov 19, 2005;366(9499):1809-1820. [ CrossRef ] [ Medline ]
- Tonetti MS, Greenwell H, Kornman KS. Staging and grading of periodontitis: framework and proposal of a new classification and case definition. J Periodontol. Jun 2018;89(Suppl 1):S159-S172. [ CrossRef ] [ Medline ]
- Worthington H, MacDonald L, Poklepovic Pericic T, Sambunjak D, Johnson TM, Imai P, et al. Home use of interdental cleaning devices, in addition to toothbrushing, for preventing and controlling periodontal diseases and dental caries. Cochrane Database Syst Rev. Apr 10, 2019;4(4):CD012018. [ FREE Full text ] [ CrossRef ] [ Medline ]
- Preshaw PM, Bissett SM. Periodontitis and diabetes. Br Dent J. Oct 11, 2019;227(7):577-584. [ CrossRef ] [ Medline ]
- Zardawi F, Gul S, Abdulkareem A, Sha A, Yates J. Association between periodontal disease and atherosclerotic cardiovascular diseases: revisited. Front Cardiovasc Med. Jan 15, 2020;7:625579. [ FREE Full text ] [ CrossRef ] [ Medline ]
- Dietrich T, Ower P, Tank M, West NX, Walter C, Needleman I, et al. British Society of Periodontology. Periodontal diagnosis in the context of the 2017 classification system of periodontal diseases and conditions - implementation in clinical practice. Br Dent J. Jan 11, 2019;226(1):16-22. [ CrossRef ] [ Medline ]
- Könönen E, Gursoy M, Gursoy U. Periodontitis: a multifaceted disease of tooth-supporting tissues. J Clin Med. Jul 31, 2019;8(8):1135. [ FREE Full text ] [ CrossRef ] [ Medline ]
- Williams K, Mithani S, Sadeghi G, Palomo L. Effectiveness of oral hygiene instructions given in computer-assisted format versus a self-care instructor. Dent J. Jan 10, 2018;6(1):2. [ FREE Full text ] [ CrossRef ] [ Medline ]
- Toniazzo MP, Nodari D, Muniz FWMG, Weidlich P. Effect of mHealth in improving oral hygiene: a systematic review with meta-analysis. J Clin Periodontol. Mar 19, 2019;46(3):297-309. [ CrossRef ] [ Medline ]
- Dicianno BE, Parmanto B, Fairman AD, Crytzer TM, Yu DX, Pramana G, et al. Perspectives on the evolution of mobile (mHealth) technologies and application to rehabilitation. Phys Ther. Mar 2015;95(3):397-405. [ FREE Full text ] [ CrossRef ] [ Medline ]
- Bene BA, O'Connor S, Mastellos N, Majeed A, Fadahunsi KP, O'Donoghue J. Impact of mobile health applications on self-management in patients with type 2 diabetes mellitus: protocol of a systematic review. BMJ Open. Jun 25, 2019;9(6):e025714. [ FREE Full text ] [ CrossRef ] [ Medline ]
- WHO Global Observatory for eHealth. mHealth: new horizons for health through mobile technologies: second global survey on eHealth. World Health Organization. 2011. URL: https://iris.who.int/handle/10665/44607 [accessed 2024-07-10]
- Aitkin M. Patient apps for improved healthcare: from novelty to mainstream. IMS Institute for Healthcare Informatics. Oct 2013. URL: https://ignacioriesgo.es/wp-content/uploads/2014/03/iihi_patient_apps_report_editora_39_2_1.pdf [accessed 2024-07-10]
- United Nations Foundation, Vodafone Foundation. mHealth for development: the opportunity of mobile technology for healthcare in the developing world. reliefweb. 2009. URL: https://reliefweb.int/report/world/mhealth-development-opportunity-mobile-technology-healthcare-developing-world [accessed 2024-07-10]
- Sharif MO, Alkadhimi A. Patient focused oral hygiene apps: an assessment of quality (using MARS) and knowledge content. Br Dent J. Sep 13, 2019;227(5):383-386. [ CrossRef ] [ Medline ]
- Schardt C, Adams MB, Owens T, Keitz S, Fontelo P. Utilization of the PICO framework to improve searching PubMed for clinical questions. BMC Med Inform Decis Mak. Jun 15, 2007;7(1):16. [ FREE Full text ] [ CrossRef ] [ Medline ]
- Liberati A, Altman DG, Tetzlaff J, Mulrow C, Gøtzsche PC, Ioannidis JPA, et al. The PRISMA statement for reporting systematic reviews and meta-analyses of studies that evaluate health care interventions: explanation and elaboration. Ann Intern Med. Aug 18, 2009;151(4):W65-W94. [ FREE Full text ] [ CrossRef ] [ Medline ]
- Löe H. The gingival index, the plaque index and the retention index systems. J Periodontol. 1967;38(6):Suppl:610-Suppl:616. [ CrossRef ] [ Medline ]
- Rathee M, Jain P. Gingivitis. StatPearls [Internet]. 2024. URL: https://pubmed.ncbi.nlm.nih.gov/32491354/ [accessed 2024-07-10]
- Greenstein G. The role of bleeding upon probing in the diagnosis of periodontal disease. A literature review. J Periodontol. Dec 1984;55(12):684-688. [ CrossRef ] [ Medline ]
- Van der Weijden GA, Timmerman MF, Nijboer A, Reijerse E, Van der Velden U. Comparison of different approaches to assess bleeding on probing as indicators of gingivitis. J Clin Periodontol. Oct 13, 1994;21(9):589-594. [ CrossRef ] [ Medline ]
- Lindhe J, Socransky SS, Nyman S, Haffajee A, Westfelt E. "Critical probing depths" in periodontal therapy. J Clin Periodontol. Jul 14, 1982;9(4):323-336. [ CrossRef ] [ Medline ]
- Listgarten MA. Periodontal probing: what does it mean? J Clin Periodontol. Jun 14, 1980;7(3):165-176. [ CrossRef ] [ Medline ]
- International Clinical Trials Registry Platform. World Health Organization. URL: https://trialsearch.who.int/ [accessed 2024-07-10]
- ClinicalTrials.gov. URL: https://clinicaltrials.gov/ [accessed 2024-07-10]
- Babineau J. Product review: Covidence (systematic review software). J Can Health Libr Assoc. Aug 01, 2014;35(2):68. [ CrossRef ]
- Kahale LA, Elkhoury R, El Mikati I, Pardo-Hernandez H, Khamis AM, Schünemann HJ, et al. Tailored PRISMA 2020 flow diagrams for living systematic reviews: a methodological survey and a proposal. F1000Res. 2021;10:192. [ FREE Full text ] [ CrossRef ] [ Medline ]
- Higgins JPT, Savović J, Page MJ, Elbers RG, Sterne JAC. Chapter 8: Assessing risk of bias in a randomized trial. Cochrane Handbook for Systematic Reviews of Interventions. 2019. URL: https://training.cochrane.org/handbook/current/chapter-08 [accessed 2024-07-10]
- Deeks JJ, Bossuyt PM, Leeflang MM, Takwoingi Y. Cochrane Handbook for Systematic Reviews of Diagnostic Test Accuracy. URL: https://training.cochrane.org/handbook-diagnostic-test-accuracy [accessed 2024-07-10]
- Higgins JPT, Thomas J, Chandler J, Cumpston M, Li T, Page MJ, et al. Cochrane Handbook for Systematic Reviews of Interventions. London. The Cochrane Collaboration; 2019.
- Salanti G, Del Giovane C, Chaimani A, Caldwell DM, Higgins JPT. Evaluating the quality of evidence from a network meta-analysis. PLoS One. Jul 3, 2014;9(7):e99682. [ FREE Full text ] [ CrossRef ] [ Medline ]
- Papakonstantinou T, Nikolakopoulou A, Higgins JPT, Egger M, Salanti G. CINeMA: software for semiautomated assessment of the confidence in the results of network meta-analysis. Campbell Syst Rev. Mar 11, 2020;16(1):e1080. [ FREE Full text ] [ CrossRef ] [ Medline ]
- Nikolakopoulou A. Assessing confidence in the results of network meta-analysis (CINeMA). BioRxiv. Preprint posted online on April 5, 2019 [ CrossRef ]
- Nikolakopoulou A, Higgins JPT, Papakonstantinou T, Chaimani A, Del Giovane C, Egger M, et al. CINeMA: an approach for assessing confidence in the results of a network meta-analysis. PLoS Med. Apr 3, 2020;17(4):e1003082. [ CrossRef ] [ Medline ]
- Ebrahim S, Akl EA, Mustafa RA, Sun X, Walter SD, Heels-Ansdell D, et al. Addressing continuous data for participants excluded from trial analysis: a guide for systematic reviewers. J Clin Epidemiol. Sep 2013;66(9):1014-1021. [ CrossRef ] [ Medline ]
- Ebrahim S, Johnston BC, Akl EA, Mustafa RA, Sun X, Walter SD, et al. Addressing continuous data measured with different instruments for participants excluded from trial analysis: a guide for systematic reviewers. J Clin Epidemiol. May 2014;67(5):560-570. [ CrossRef ] [ Medline ]
- Sharma S, Mohanty V, Balappanavar AY, Chahar P, Rijhwani K. Role of digital media in promoting oral health: a systematic review. Cureus. Sep 2022;14(9):e28893. [ FREE Full text ] [ CrossRef ] [ Medline ]
- Chen R, Santo K, Wong G, Sohn W, Spallek H, Chow C, et al. Mobile apps for dental caries prevention: systematic search and quality evaluation. JMIR Mhealth Uhealth. Jan 13, 2021;9(1):e19958. [ FREE Full text ] [ CrossRef ] [ Medline ]
- Al-Moghrabi D, Alkadhimi A, Tsichlaki A, Pandis N, Fleming PS. The influence of mobile applications and social media-based interventions in producing behavior change among orthodontic patients: A systematic review and meta-analysis. Am J Orthod Dentofacial Orthop. Mar 2022;161(3):338-354. [ CrossRef ] [ Medline ]
Abbreviations
bleeding index |
clinical attachment loss |
Confidence In Network Meta-Analysis |
gingival index |
Grading of Recommendations Assessment, Development, and Evaluation |
Medical Subject Heading |
mobile health |
Participants, Interventions, Comparators, Outcomes, and Study design |
periodontal pocket depth |
Preferred Reporting Items for Systematic reviews and Meta-Analyses |
randomized controlled trial |
Edited by A Mavragani; submitted 04.07.23; peer-reviewed by MA Rusandi; comments to author 09.11.23; revised version received 22.02.24; accepted 11.03.24; published 31.07.24.
©Reem Musa, Dalia Elamin, Robert Barrie, Faheema Kimmie-Dhansay. Originally published in JMIR Research Protocols (https://www.researchprotocols.org), 31.07.2024.
This is an open-access article distributed under the terms of the Creative Commons Attribution License (https://creativecommons.org/licenses/by/4.0/), which permits unrestricted use, distribution, and reproduction in any medium, provided the original work, first published in JMIR Research Protocols, is properly cited. The complete bibliographic information, a link to the original publication on https://www.researchprotocols.org, as well as this copyright and license information must be included.

IMAGES
VIDEO
COMMENTS
Review of Educational Research. The Review of Educational Research (RER) publishes critical, integrative reviews of research literature bearing on education, including conceptualizations, interpretations, and syntheses of literature and scholarly work in a field broadly relevant to … | View full journal description.
A review of reviews (sometimes called 'overviews' or 'umbrella' reviews) is a tertiary level of analysis. It is a systematic map and/or synthesis of previous reviews. The 'data' for reviews of reviews are previous reviews rather than primary research studies (see for example Newman et al. ( 2018 ).
In this open access edited volume, international researchers of the field describe and discuss the systematic review method in its application to research in education. Alongside fundamental methodical considerations, reflections and practice examples are included and provide an introduction and overview on systematic reviews in education research.
In this open access edited volume, international researchers of the field describe and discuss the systematic review method in its application to research in education. Alongside fundamental ...
Educational Research Review. Volume 34, November 2021, 100411. Teacher well-being: A systematic review of the research literature from the year 2000-2019 ... A systematic review following the PRISMA-statement was applied to peer-reviewed papers published between the years 2000-2019 and a total of 98 studies were included in the final analysis ...
The theoretical basis of SR in educational research draws on the Retrocue Effect and the Cognitive Theory of Multimedia Learning (CTML) (Mayer and Moreno, 1998; Moreno and Mayer, 1999; Souza and ...
Research with systematic review is a research design to systematically synthesize existing research evidence by searching research articles, critical review (critical appraisal), and synthesis of ...
IDESR is a database of published systematic reviews in Education and a clearinghouse for protocol registration of ongoing and planned systematic reviews. From this page you can: meet the team behind the IDESR project. IDESR is now in its second phase of development and is accepting registrations of review protocols for all areas of education.
education research to share their experiences as worked examples, and to reect on the promises and pitfalls in each step of the review process. We hope that these examples will be particularly helpful and can serve as a kind of roadmap for col-leagues who are conducting a systematic review for the rst time. Part I: Methodological considerations
Systematic reviews are still a controversial topic in some quarters, with the arguments for and against their use being well-rehearsed. In an attempt to advance a more nuanced approach to thinking about systematic reviewing, this paper illustrates the wide range of theoretical perspectives, methodologies and purposes that underpin the vast range of systematic review approaches now available ...
The primary role of educational research, ... Through a systematic review of all RCTs conducted in education from 1980 to 2016, this article seeks to assess these four criticisms against the actual evidence. Following an outline of the methods used for this systematic review, the article sets out the key findings and then returns to these four ...
Overviews, or syntheses of research syntheses, have become a popular approach to synthesizing the rapidly expanding body of research and systematic reviews. Despite their popularity, few guidelines exist and the state of the field in education is unclear.
ure repositories yielded 25 total education-related over-views. Our analysis revealed that many commonly reported aspects of sys-tematic reviews, such as the. search, screen, and coding procedures, were regularly unreported. Only a handful of overview authors discussed the syn-thesis technique and few.
The purpose of a systematic review is to answer specific questions, based on an explicit, systematic and replicable search strategy, with inclusion and exclusion criteria identifying studies to be included or excluded (Gough, Oliver & Thomas, 2017).Data is then coded and extracted from included studies, in order to synthesise findings and to shine light on their application in practice, as ...
Overviews, or syntheses of research syntheses, have become a popular approach to synthesizing the rapidly expanding body of research and systematic reviews. Despite their popularity, few guidelines exist and the state of the field in education is unclear. The purpose of this study is to describe the prevalence and current state of overviews of education research and to provide further guidance ...
The Best of Two Worlds: A Systematic Review on Combining Real and Virtual Experiments in Science Education. Salome Wörner. Jochen Kuhn. Katharina Scheiter. Preview abstract. Open Access Research article First published April 6, 2022 pp. 911-952. xml PDF / EPUB.
The interdisciplinary and multidisciplinary characteristics of education research make it difficult for researchers to identify the overall development of specific topics within the field. In this paper, we first collect core papers from the field of education. Then, we construct keyword co-occurrence networks using these papers.
Educational Research Review is an international journal addressed to researchers and various agencies interested in the review of studies and theoretical papers in education at any level. The journal accepts high quality articles that are solving educational research problems by using a review approach. ... A systematic review. Fan Ouyang ...
Abstract There has been a recent increase in interest in the research review as a method of presenting cumulative data about the effects of educational policies and practices. This is part of a wider movement in 'evidence-informed policy-making' espoused by the current Government. In part, the interest as been sparked by the perceived success of the Cochrane Collaboration in medicine ...
Aim and research question. This systematic literature review (Hart, Citation 2018) addresses the abovementioned gap, with the aim of contributing knowledge about research into the decision-making tasks undertaken by educational superintendents, and understanding the characteristics of superintendents' duties as decision-makers at the administrative level.
We present key findings of a comprehensive survey based on a systematic research framework for examining and generalizing affordances and constraints of online peer-assessment systems. This framework (a) provides a foundation of a design-science metatheory of online peer assessment, (b) helps structure the discussion of user needs and design ...
The Agency for Healthcare Research and Quality (AHRQ) has recommended quality evaluation criteria for observational studies [], which assess the risk of bias in 5 domains: selection bias, implementation bias, follow-up bias, measurement bias, and reporting bias.In the cross-sectional studies included in our review, their scores range from 6 to 8, indicating a higher quality of inclusion in the ...
Systematic phonics instruction helped children learn to read better than all forms of control group instruction, including whole language. In sum, systematic phonics instruction proved effective and should be implemented as part of literacy programs to teach beginning reading as well as to prevent and remediate reading difficulties.
To commemorate the inaugural annual special issue on systematic literature reviews in the International Journal of Consumer Studies, the editors have pooled their expertise and experience of ...
MacDonncha, Ciaran: Department of Physical Education and Sport Sciences, University of Limerick, Limerick, Ireland. Adamakis, Manolis: School of Physical Education and Sport Science, National and Kapodistrian University of Athens, Athens, Greece. Source. Adolescent Research Review, Vol 9(2), Jun 2024, 365. ISSN. 2363-8354(Electronic); 2363-8346 ...
European Early Childhood Education Research Journal List of Issues Latest Articles ... A systematic review of English-language empirical research published between 2018 and 2022 was conducted to identify the features and impacts of CPD approaches that have a significant influence on the transformation of ECEC practices and contexts. The ...
The purpose of this study is to explore the relationship between empathy and psychological capital through a systematic literature review. Outlining the concept of empathy, the components and measurement of psychological capital, and current research on the relationship between these two constructs, it examines the theoretical frameworks that explain the interaction between empathy and ...
The Effects of Educational Technology Interventions on Literacy in Elementary School: A Meta-Analysis. Rebecca D. Silverman. Kristin Keane. Elena Darling-Hammond. Saurabh Khanna. Preview abstract. Educational technology is ubiquitous, and educational technology interventions often target literacy.
education research to share their experiences as worked examples, and to reect on the promises and pitfalls in each step of the review process. We hope that these examples will be particularly helpful and can serve as a kind of roadmap for col-leagues who are conducting a systematic review for the rst time. Part I: Methodological considerations
The review is currently ongoing and is expected to be complete by the end of 2024 with the results published in early 2025. Conclusions: This systematic review and meta-analysis will contribute to developing mobile apps with enhanced criteria to improve periodontal clinical outcomes.