- DOI: 10.1109/ACCESS.2021.3118541
- Corpus ID: 239037459

Video Processing using Deep learning Techniques: A Systematic Literature Review
- Vijeta Sharma , Manjari Gupta , +1 author Deepti Mishra
- Published in IEEE Access 2021
- Computer Science
Figures and Tables from this paper
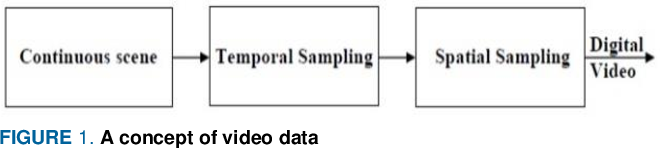
33 Citations
Video content analysis using deep learning models.
- Highly Influenced
Exploring Video Event Classification: Leveraging Two-Stage Neural Networks and Customized CNN Models with UCF-101 and CCV Datasets
Deep video stream information analysis and retrieval: challenges and opportunities, active learning for video classification with frame level queries, video crawling using deep learning, deep learning-based eye gaze estimation for automotive applications using knowledge distillation, enhanced video temporal segmentation using a siamese network with multimodal features, exploring the power of deep learning for seamless background audio generation in videos, a comprehensive analysis on unconstraint video analysis using deep learning approaches, deep-learning-based action and trajectory analysis for museum security videos, 140 references, a deep convolutional neural network for video sequence background subtraction, crowd video classification using convolutional neural networks, deep multi-view learning methods: a review, a survey on the new generation of deep learning in image processing, a short review of deep learning methods for understanding group and crowd activities, object detection with deep learning: a review, automatic soccer video event detection based on a deep neural network combined cnn and rnn, beyond short snippets: deep networks for video classification, enabling versatile analysis of large scale traffic video data with deep learning and hiveql, video scene parsing: an overview of deep learning methods and datasets, related papers.
Showing 1 through 3 of 0 Related Papers
Subscribe to the PwC Newsletter
Join the community, add a new evaluation result row, video generation.
307 papers with code • 15 benchmarks • 14 datasets
( Various Video Generation Tasks. Gif credit: MaGViT )
Benchmarks Add a Result
Trend | Dataset | Best Model | -->Paper | Code | Compare |
---|---|---|---|---|---|
W.A.L.T-XL (class-conditional) | -->|||||
MAGVIT | -->|||||
StyleSV (256x256) | -->|||||
Make-A-Video (ours) vs. CogVideo (Chinese) | -->|||||
TGAN-F | -->|||||
Imagen original (constant=6) | -->|||||
StyleSV (256x256) | -->|||||
TGANv2 (2020) | -->|||||
W.A.L.T-L | -->|||||
PG-SWGAN-3D | -->|||||
DVD-GAN | -->|||||
DVD-GAN | -->|||||
INR-V | -->|||||
StyleSV | -->|||||
VideoAssembler (Zero-Shot, 256x256, class-conditional) | -->

Most implemented papers
Gans trained by a two time-scale update rule converge to a local nash equilibrium.
Generative Adversarial Networks (GANs) excel at creating realistic images with complex models for which maximum likelihood is infeasible.
Everybody Dance Now

This paper presents a simple method for "do as I do" motion transfer: given a source video of a person dancing, we can transfer that performance to a novel (amateur) target after only a few minutes of the target subject performing standard moves.
Learning Temporal Coherence via Self-Supervision for GAN-based Video Generation
Additionally, we propose a first set of metrics to quantitatively evaluate the accuracy as well as the perceptual quality of the temporal evolution.
Consistency Models
Through extensive experiments, we demonstrate that they outperform existing distillation techniques for diffusion models in one- and few-step sampling, achieving the new state-of-the-art FID of 3. 55 on CIFAR-10 and 6. 20 on ImageNet 64x64 for one-step generation.
MoCoGAN: Decomposing Motion and Content for Video Generation
The proposed framework generates a video by mapping a sequence of random vectors to a sequence of video frames.
Video Diffusion Models
Generating temporally coherent high fidelity video is an important milestone in generative modeling research.
Temporal Generative Adversarial Nets with Singular Value Clipping
In this paper, we propose a generative model, Temporal Generative Adversarial Nets (TGAN), which can learn a semantic representation of unlabeled videos, and is capable of generating videos.
Stochastic Adversarial Video Prediction
However, learning to predict raw future observations, such as frames in a video, is exceedingly challenging -- the ambiguous nature of the problem can cause a naively designed model to average together possible futures into a single, blurry prediction.
Collaborative Neural Rendering using Anime Character Sheets
Drawing images of characters with desired poses is an essential but laborious task in anime production.
Unsupervised Learning for Physical Interaction through Video Prediction
A core challenge for an agent learning to interact with the world is to predict how its actions affect objects in its environment.
IEEE Account
- Change Username/Password
- Update Address
Purchase Details
- Payment Options
- Order History
- View Purchased Documents
Profile Information
- Communications Preferences
- Profession and Education
- Technical Interests
- US & Canada: +1 800 678 4333
- Worldwide: +1 732 981 0060
- Contact & Support
- About IEEE Xplore
- Accessibility
- Terms of Use
- Nondiscrimination Policy
- Privacy & Opting Out of Cookies
A not-for-profit organization, IEEE is the world's largest technical professional organization dedicated to advancing technology for the benefit of humanity. © Copyright 2024 IEEE - All rights reserved. Use of this web site signifies your agreement to the terms and conditions.
This website uses cookies to ensure you get the best experience. Learn more about DOAJ’s privacy policy.
Hide this message
You are using an outdated browser. Please upgrade your browser to improve your experience and security.
The Directory of Open Access Journals
Quick search, ictact journal on image and video processing ijivp.
0976-9099 (Print) / 0976-9102 (Online)
- ISSN Portal
Publishing with this journal
There are no publication fees ( article processing charges or APCs ) to publish with this journal.
Look up the journal's:
- Aims & scope
- Instructions for authors
- Editorial Board
- Double anonymous peer review
Expect on average 8 weeks from submission to publication.
Best practice
This journal began publishing in open access in 2010 . What does DOAJ define as Open Accesss?
This journal uses a CC BY-NC-SA license.
Attribution Non-Commercial Share Alike
→ Look up their open access statement and their license terms .
The author does not retain unrestricted copyrights and publishing rights.
Journal metadata
Publisher ICT Academy of Tamil Nadu , India Manuscripts accepted in English
LCC subjects Look up the Library of Congress Classification Outline Technology: Electrical engineering. Electronics. Nuclear engineering: Telecommunication Medicine: Medicine (General): Computer applications to medicine. Medical informatics Keywords computer vision medical imaging image and video processing video segmentation and analysis computer graphics and visualization pattern recognition
WeChat QR code
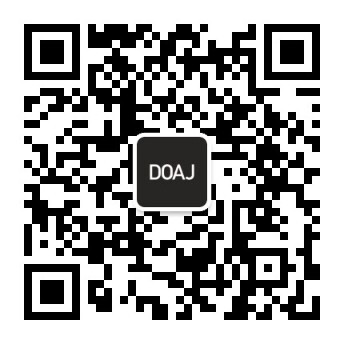
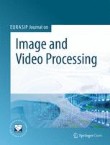
- Search by keyword
- Search by citation
Page 1 of 19
PointPCA: point cloud objective quality assessment using PCA-based descriptors
Point clouds denote a prominent solution for the representation of 3D photo-realistic content in immersive applications. Similarly to other imaging modalities, quality predictions for point cloud contents are ...
- View Full Text
Hybrid model-based early diagnosis of esophageal disorders using convolutional neural network and refined logistic regression
Accurate diagnosis of the stage of esophageal disorders is crucial in the treatment planning for patients with esophageal cancer and in improving the 5-year survival rate. The progression of esophageal cancer ...
Compressed point cloud classification with point-based edge sampling
3D point cloud data, as an immersive detailed data source, has been increasingly used in numerous applications. To deal with the computational and storage challenges of this data, it needs to be compressed bef...
Evaluation of the use of box size priors for 6D plane segment tracking from point clouds with applications in cargo packing
This paper addresses the problem of 6D pose tracking of plane segments from point clouds acquired from a mobile camera. This is motivated by manual packing operations, where an opportunity exists to enhance pe...
Remote expert viewing, laboratory tests or objective metrics: which one(s) to trust?
We present a study on the validity of quality assessment in the context of the development of visual media coding schemes. The work is motivated by the need for reliable means for decision-taking in standardiz...
Impact of LiDAR point cloud compression on 3D object detection evaluated on the KITTI dataset
The rapid growth on the amount of generated 3D data, particularly in the form of Light Detection And Ranging (LiDAR) point clouds (PCs), poses very significant challenges in terms of data storage, transmission...
Subjective performance evaluation of bitrate allocation strategies for MPEG and JPEG Pleno point cloud compression
The recent rise in interest in point clouds as an imaging modality has motivated standardization groups such as JPEG and MPEG to launch activities aiming at developing compression standards for point clouds. L...
Adaptive bridge model for compressed domain point cloud classification
The recent adoption of deep learning-based models for the processing and coding of multimedia signals has brought noticeable gains in performance, which have established deep learning-based solutions as the un...
Learning-based light field imaging: an overview
Conventional photography can only provide a two-dimensional image of the scene, whereas emerging imaging modalities such as light field enable the representation of higher dimensional visual information by cap...
Cartoon copyright recognition method based on character personality action
Aiming at the problem of cartoon piracy and plagiarism, this paper proposes a method of cartoon copyright recognition based on character personality actions. This method can be used to compare the original car...
4AC-YOLOv5: an improved algorithm for small target face detection
In real scenes, small target faces often encounter various conditions, such as intricate background, occlusion and scale change, which leads to the problem of omission or misdetection of face detection results...
Analysis of thermal videos for detection of lie during interrogation
The lie-detection tests are traditionally carried out by well-trained experts using polygraph machines. However, it is time-consuming, invasive, and, overall, a cumbersome process, not admissible by the court ...
Semi-automated computer vision-based tracking of multiple industrial entities: a framework and dataset creation approach
This contribution presents the TOMIE framework (Tracking Of Multiple Industrial Entities), a framework for the continuous tracking of industrial entities (e.g., pallets, crates, barrels) over a network of, in ...
Fast CU size decision and intra-prediction mode decision method for H.266/VVC
H.266/Versatile Video Coding (VVC) is the most recent video coding standard developed by the Joint Video Experts Team (JVET). The quad-tree with nested multi-type tree (QTMT) architecture that improves the com...
Assessment framework for deepfake detection in real-world situations
Detecting digital face manipulation in images and video has attracted extensive attention due to the potential risk to public trust. To counteract the malicious usage of such techniques, deep learning-based de...
Edge-aware nonlinear diffusion-driven regularization model for despeckling synthetic aperture radar images
Speckle noise corrupts synthetic aperture radar (SAR) images and limits their applications in sensitive scientific and engineering fields. This challenge has attracted several scholars because of the wide dema...
Multimodal few-shot classification without attribute embedding
Multimodal few-shot learning aims to exploit complementary information inherent in multiple modalities for vision tasks in low data scenarios. Most of the current research focuses on a suitable embedding space...
Secure image transmission through LTE wireless communications systems
Secure transmission of images over wireless communications systems can be done using RSA, the most known and efficient cryptographic algorithm, and OFDMA, the most preferred signal processing choice in wireles...
An optimized capsule neural networks for tomato leaf disease classification
Plant diseases have a significant impact on leaves, with each disease exhibiting specific spots characterized by unique colors and locations. Therefore, it is crucial to develop a method for detecting these di...
Multi-layer features template update object tracking algorithm based on SiamFC++
SiamFC++ only extracts the object feature of the first frame as a tracking template, and only uses the highest level feature maps in both the classification branch and the regression branch, so that the respec...
Robust steganography in practical communication: a comparative study
To realize the act of covert communication in a public channel, steganography is proposed. In the current study, modern adaptive steganography plays a dominant role due to its high undetectability. However, th...
Multi-attention-based approach for deepfake face and expression swap detection and localization
Advancements in facial manipulation technology have resulted in highly realistic and indistinguishable face and expression swap videos. However, this has also raised concerns regarding the security risks assoc...
Semantic segmentation of textured mosaics
This paper investigates deep learning (DL)-based semantic segmentation of textured mosaics. Existing popular datasets for mosaic texture segmentation, designed prior to the DL era, have several limitations: (1...
Comparison of synthetic dataset generation methods for medical intervention rooms using medical clothing detection as an example
The availability of real data from areas with high privacy requirements, such as the medical intervention space is low and the acquisition complex in terms of data protection. To enable research for assistance...
Phase congruency based on derivatives of circular symmetric Gaussian function: an efficient feature map for image quality assessment
Image quality assessment (IQA) has become a hot issue in the area of image processing, which aims to evaluate image quality automatically by a metric being consistent with subjective evaluation. The first stag...
Correction: Printing and scanning investigation for image counter forensics
The original article was published in EURASIP Journal on Image and Video Processing 2022 2022 :2
An early CU partition mode decision algorithm in VVC based on variogram for virtual reality 360 degree videos
360-degree videos have become increasingly popular with the application of virtual reality (VR) technology. To encode such kind of videos with ultra-high resolution, an efficient and real-time video encoder be...
Learning a crowd-powered perceptual distance metric for facial blendshapes
It is known that purely geometric distance metrics cannot reflect the human perception of facial expressions. A novel perceptually based distance metric designed for 3D facial blendshape models is proposed in ...
Studies in differentiating psoriasis from other dermatoses using small data set and transfer learning
Psoriasis is a common skin disorder that should be differentiated from other dermatoses if an effective treatment has to be applied. Regions of Interests, or scans for short, of diseased skin are processed by ...
Heterogeneous scene matching based on the gradient direction distribution field
Heterogeneous scene matching is a key technology in the field of computer vision. The image rotation problem is popular and difficult in the field of heterogeneous scene matching. In this paper, a heterogeneou...
FitDepth: fast and lite 16-bit depth image compression algorithm
This article presents a fast parallel lossless technique and a lossy image compression technique for 16-bit single-channel images. Nowadays, such techniques are “a must” in robotics and other areas where sever...
Vehicle logo detection using an IoAverage loss on dataset VLD100K-61
Vehicle Logo Detection (VLD) is of great significance to Intelligent Transportation Systems (ITS). Although many methods have been proposed for VLD, it remains a challenging problem. To improve the VLD accurac...
Correction: Research on application of multimedia image processing technology based on wavelet transform
The original article was published in EURASIP Journal on Image and Video Processing 2019 2019 :24
Correction: Geolocation of covert communication entity on the Internet for post-steganalysis
The original article was published in EURASIP Journal on Image and Video Processing 2020 2020 :15
Reversible designs for extreme memory cost reduction of CNN training
Training Convolutional Neural Networks (CNN) is a resource-intensive task that requires specialized hardware for efficient computation. One of the most limiting bottlenecks of CNN training is the memory cost a...
Data and image storage on synthetic DNA: existing solutions and challenges
Storage of digital data is becoming challenging for humanity due to the relatively short life-span of storage devices. Furthermore, the exponential increase in the generation of digital data is creating the ne...
Retraction Note: Research on path guidance of logistics transport vehicle based on image recognition and image processing in port area
A novel secured euclidean space points algorithm for blind spatial image watermarking.
Digital raw images obtained from the data set of various organizations require authentication, copyright protection, and security with simple processing. New Euclidean space point’s algorithm is proposed to au...
Retraction Note: Research on professional talent training technology based on multimedia remote image analysis
Retraction note: analysis of sports image detection technology based on machine learning, retraction note: research on image correction method of network education assignment based on wavelet transform, retraction note: performance analysis of ethylene-propylene diene monomer sound-absorbing materials based on image processing recognition, retraction note to: translation analysis of english address image recognition based on image recognition, retraction note: image processing algorithm of hartmann method aberration automatic measurement system with tensor product model, retraction note to: research on english translation distortion detection based on image evolution, retraction note: a method for spectral image registration based on feature maximum submatrix, fine-grained precise-bone age assessment by integrating prior knowledge and recursive feature pyramid network.
Bone age assessment (BAA) evaluates individual skeletal maturity by comparing the characteristics of skeletal development to the standard in a specific population. The X-ray image examination for bone age is t...
Palpation localization of radial artery based on 3-dimensional convolutional neural networks
Palpation localization is essential for detecting physiological parameters of the radial artery for pulse diagnosis of Traditional Chinese Medicine (TCM). Detecting signal or applying pressure at the wrong loc...
Weakly supervised spatial–temporal attention network driven by tracking and consistency loss for action detection
This study proposes a novel network model for video action tube detection. This model is based on a location-interactive weakly supervised spatial–temporal attention mechanism driven by multiple loss functions...
Performance analysis of different DCNN models in remote sensing image object detection
In recent years, deep learning, especially deep convolutional neural networks (DCNN), has made great progress. Many researchers use different DCNN models to detect remote sensing targets. Different DCNN models...
- Aims and Scope
- Editorial Board
- Sign up for article alerts and news from this journal
- Follow us on Twitter
- Follow us on Facebook
Webinar Series
Learn more about the EURASIP Journal on Image and Video Processing free monthly webinar series
Affiliated with
Academia.edu no longer supports Internet Explorer.
To browse Academia.edu and the wider internet faster and more securely, please take a few seconds to upgrade your browser .
- We're Hiring!
- Help Center

Video Processing
- Most Cited Papers
- Most Downloaded Papers
- Newest Papers
- Last »
- Image Processing Follow Following
- Video Analysis Follow Following
- Web Programming Follow Following
- Computer Vision Follow Following
- Pattern Recognition Follow Following
- Digital Image Processing Follow Following
- Video Games Follow Following
- MySQL Follow Following
- Computer Science Follow Following
- Machine Learning Follow Following
Enter the email address you signed up with and we'll email you a reset link.
- Academia.edu Journals
- We're Hiring!
- Help Center
- Find new research papers in:
- Health Sciences
- Earth Sciences
- Cognitive Science
- Mathematics
- Computer Science
- Academia ©2024
Grab your spot at the free arXiv Accessibility Forum
Help | Advanced Search
Computer Vision and Pattern Recognition
Authors and titles for recent submissions.
- Tue, 13 Aug 2024
- Mon, 12 Aug 2024
- Fri, 9 Aug 2024
- Thu, 8 Aug 2024
- Wed, 7 Aug 2024
See today's new changes
Tue, 13 Aug 2024 (showing first 25 of 152 entries )
ORIGINAL RESEARCH article
Using sea lion-borne video to map diverse benthic habitats in southern australia.
- 1 Ecology and Evolutionary Biology, School of Biological Sciences, The University of Adelaide, Adelaide, SA, Australia
- 2 South Australian Research and Development Institute (SARDI) (Aquatic Sciences), West Beach, SA, Australia
- 3 Division of Aquatic Resources, Department of Land and Natural Resources, Honolulu, HI, United States
- 4 Department for Environment and Water, Port Lincoln, SA, Australia
Across the world’s oceans, our knowledge of the habitats on the seabed is limited. Increasingly, video/imagery data from remotely operated underwater vehicles (ROVs) and towed and drop cameras, deployed from vessels, are providing critical new information to map unexplored benthic (seabed) habitats. However, these vessel-based surveys involve considerable time and personnel, are costly, require favorable weather conditions, and are difficult to conduct in remote, offshore, and deep marine habitats, which makes mapping and surveying large areas of the benthos challenging. In this study, we present a novel and efficient method for mapping diverse benthic habitats on the continental shelf, using animal-borne video and movement data from a benthic predator, the Australian sea lion ( Neophoca cinerea ). Six benthic habitats (between 5-110m depth) were identified from data collected by eight Australian sea lions from two colonies in South Australia. These habitats were macroalgae reef, macroalgae meadow, bare sand, sponge/sand, invertebrate reef and invertebrate boulder habitats. Percent cover of benthic habitats differed on the foraging paths of sea lions from both colonies. The distributions of these benthic habitats were combined with oceanographic data to build Random Forest models for predicting benthic habitats on the continental shelf. Random forest models performed well (validated models had a >98% accuracy), predicting large areas of macroalgae reef, bare sand, sponge/sand and invertebrate reef habitats on the continental shelf in southern Australia. Modelling of benthic habitats from animal-borne video data provides an effective approach for mapping extensive areas of the continental shelf. These data provide valuable new information on the seabed and complement traditional methods of mapping and surveying benthic habitats. Better understanding and preserving these habitats is crucial, amid increasing human impacts on benthic environments around the world.
1 Introduction
Across much of the marine environment, our understanding of the structure and distribution of habitats on the seabed is limited ( Kostylev, 2012 ; Mayer et al., 2018 ; Menandro and Bastos, 2020 ). For marine habitats at depth, remotely operated underwater vehicles (ROVs), and towed and drop cameras, deployed from vessels, are gaining increasing use to collect high-resolution video and imagery data, enabling detailed mapping and surveying of benthic (seabed) environments ( López-Garrido et al., 2020 ; Button et al., 2021 ; Vigo et al., 2023 ). However, these vessel-based surveys are costly, time and personnel-intensive, and rely on suitable weather conditions, which makes mapping large expanses of the benthos challenging ( Mayer et al., 2018 ; Menandro and Bastos, 2020 ). In this study, we present a novel and effective method to map diverse benthic habitats on the continental shelf in southern Australia, using animal-borne video from a benthic foraging marine mammal, the Australian sea lion ( Neophoca cinerea ).
For mapping and surveying marine habitats, animal-borne video from Australian sea lions offers unique advantages. Video can be recorded across large areas of the benthos in short timeframes, deployments can be conducted from shore with reduced personnel at a relatively low cost, and deployments are less subject to weather conditions. Additionally, video can be collected from depths, habitats, and marine areas that are difficult or impossible to access using more conventional methods, such as diver surveys, and towed and drop camera deployments. Animal-borne video from Australian sea lions also provides a novel way to understand the ecological value of different benthic habitats from a predator’s perspective, complementing more traditional approaches and ecological criteria that assess habitat quality and importance ( Diaz et al., 2004 ; Monk et al., 2010 ; Torn et al., 2017 ).
For the waters in South Australia, our knowledge of the benthos is limited and patchy. In sheltered embayments, such as the Gulf St. Vincent ( Figure 1 ), towed camera and diver surveys have been used to map benthic habitats, highlighting large regions of bare sand plains and seagrass meadows ( Shepherd and Sprigg, 1976 ; Tanner, 2005 ). Elsewhere in South Australia, in regions such as the Spencer Gulf and the Great Australian Bight, sled and grab sampling have provided some insight into sediment composition and benthic community structure ( Ward et al., 2006a ; Currie et al., 2007 ; O'Connell et al., 2016 ). However, for most of the state’s waters, the distribution and structure of benthic habitats is unknown. In this study, we use animal-borne video, collected from eight adult female Australian sea lions from two colonies in South Australia, to identify and map diverse benthic habitats on the continental shelf.
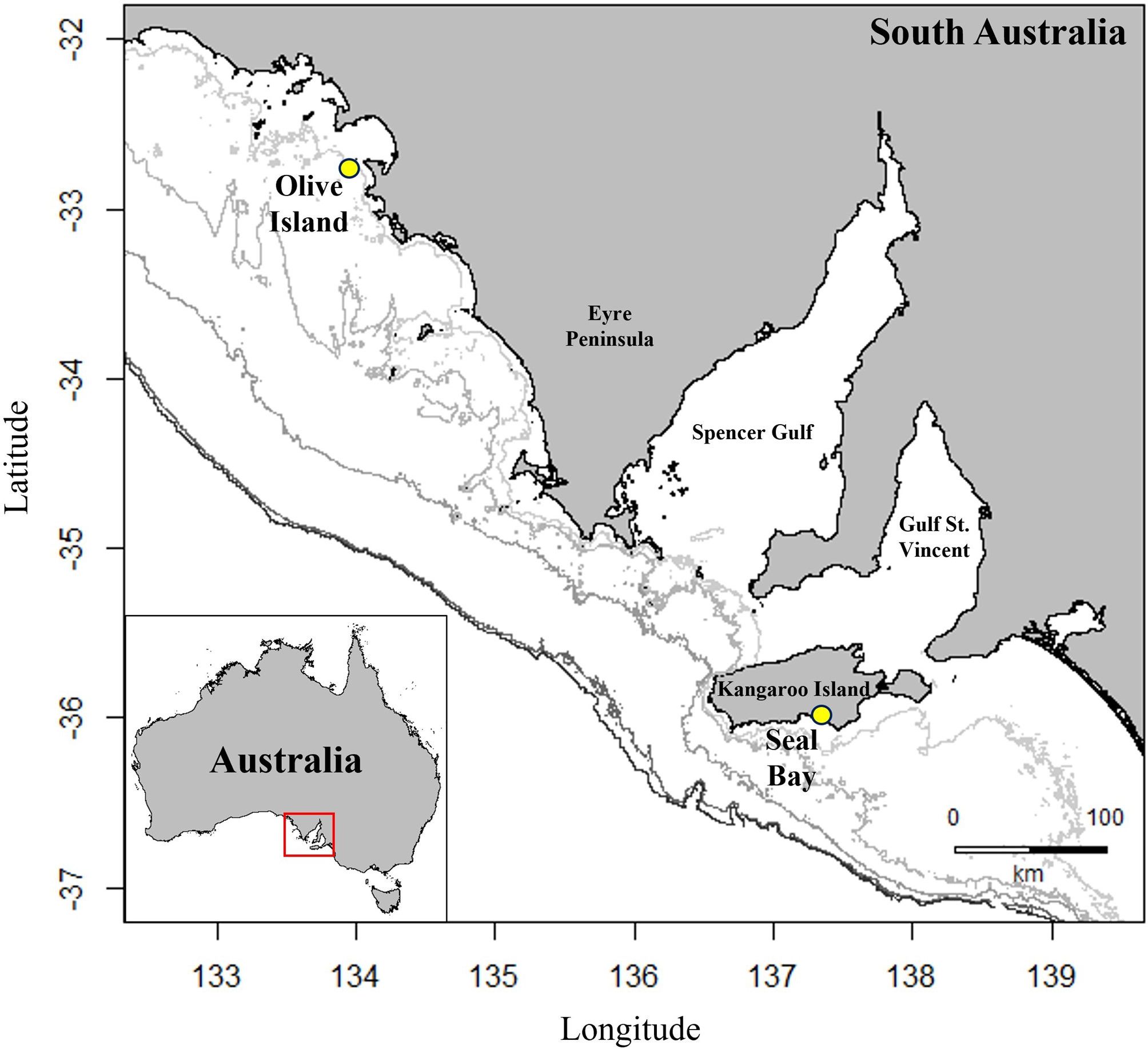
Figure 1 Location of colonies for deployment of animal-borne cameras, Argos-linked GPS loggers and accelerometers/magnetometers on eight adult female Australian sea lions from Olive Island, western Eyre Peninsula (32.721 ° S, 133.968°E) and Seal Bay, Kangaroo Island (35.994°S, 137.317°E) in South Australia (yellow circles). Isobaths represent depth contours at 50, 75, 100, 150 and 200m (light to dark grey).
Australian sea lions are benthic predators ( Peters et al., 2015 ; Berry et al., 2017 ; Goldsworthy et al., 2019 ), that maximize time on the seabed ( Costa and Gales, 2003 ; Fowler et al., 2006 ), restricting foraging effort to the continental shelf ( Goldsworthy et al., 2007 ; 2022 ). Animal-borne video has also revealed that Australian sea lions forage across diverse benthic habitats, including sponge gardens, bare sand plains, macroalgae reefs, and seagrass meadows (Angelakis, in review). Australian sea lions are therefore an ideal platform for quantitatively assessing and mapping the distribution and structure of benthic habitats across continental shelf waters in southern Australia. Studies mapping and surveying benthic habitats from animal-borne video and imagery are limited. However, recent deployments on white sharks ( Carcharodon carcharias ) and grey reef sharks ( Carcharhinus amblyrhynchos ) have been used to map kelp forests and assess growth forms and percent cover of different corals ( Jewell et al., 2019 ; Chapple et al., 2021 ), and deployments on tiger sharks ( Galeocerdo cuvier ) have mapped seagrass ecosystems ( Gallagher et al., 2022 ). These approaches therefore represent an emerging area of ecological research for marine environments ( Moll et al., 2007 ).
Like in many regions of the world ( Brown et al., 2017 ; Sweetman et al., 2017 ; Yoklavich et al., 2018 ), benthic habitat surveys in South Australia have identified major changes to the marine environment, as a result of human activity ( Tanner, 2005 ; Connell et al., 2008 ; Bryars and Rowling, 2009 ; Alleway and Connell, 2015 ). Critically, the documentation of these human-induced changes to habitat sparked policy developments by government and private investment for habitat restoration ( McAfee et al., 2020 ). Such information also underpins the planning and management of marine reserve networks ( Stewart et al., 2003 ; Thomas and Hughes, 2016 ). Furthermore, habitat surveys have highlighted diverse and endemic benthic communities in South Australia ( Edyvane, 1999 ; McLeay et al., 2003 ; Currie et al., 2009 ; MacIntosh et al., 2018 ). As Australian sea lions utilize the continental shelf ( Goldsworthy et al., 2007 ; 2022 ), the application of animal-borne video provides an efficient way to explore large areas of unmapped benthic habitats, find reefs, and locate ecologically important areas (e.g. valuable sea lion habitat), both within and outside marine reserves. Hence, this approach provides a complementary technique to existing methods for mapping benthic environments ( López-Garrido et al., 2020 ; Vigo et al., 2023 ) and managing marine reserves ( Stewart et al., 2003 ; Thomas and Hughes, 2016 ).
Australian sea lions are an endangered species (The International Union for Conservation of Nature IUCN Red List of Threatened Species and the Australian Environmental Protection and Biodiversity Conservation Act, 1999) ( Goldsworthy, 2015 ), whose populations have declined by more than 60% over the last 40 years ( Goldsworthy et al., 2021 ). The use of animal-borne video from Australian sea lions can therefore serve two major functions, providing new benthic habitat data for unknown/unmapped areas of the marine environment and identifying and mapping critical habitats for an endangered marine predator ( Goldsworthy et al., 2021 ).
In this study, we aim to use animal-borne video and movement data to 1) calculate the percent cover of different benthic habitats on Australian sea lion foraging paths, 2) develop a model for predicting and mapping diverse benthic habitats on the continental shelf in southern Australia, and 3) assess the predicted distribution of these habitats, relative to our current understanding of benthic environments in South Australia.
2 Materials and methods
2.1 study sites and deployment of instruments.
Data were collected from eight adult female Australian sea lions from two colonies in South Australia at Olive Island Conservation Park (32.721 ° S, 133.968°E) on the western Eyre Peninsula ( n = 4) and Seal Bay Conservation Park (35.994°S, 137.317°E) ( n = 4) on Kangaroo Island ( Figure 1 ), between December 2022 and August 2023. Olive Island and Seal Bay are two of the largest Australian sea lion colonies and are key monitoring sites for the species ( Goldsworthy et al., 2021 ). Morphometric, condition, and reproductive history data for each sea lion are provided ( Table 1 ).
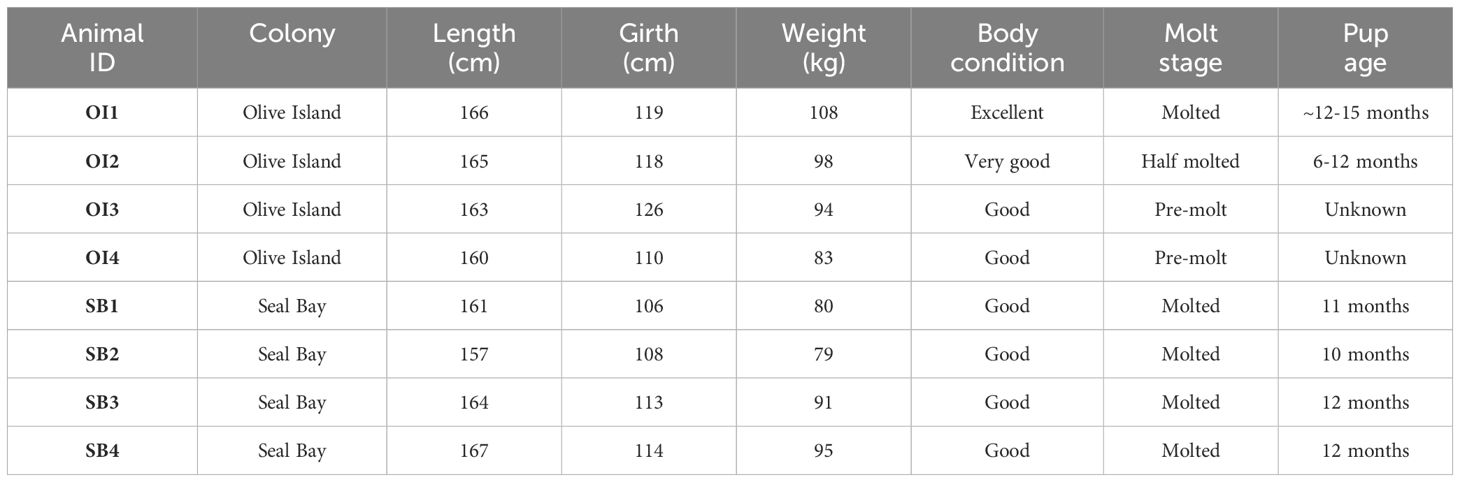
Table 1 Morphometric, condition, and reproductive data (at deployment) for eight adult female Australian sea lions from Olive Island (OI1, OI2, OI3, OI4) and Seal Bay (SB1, SB2, SB3, SB4) in South Australia.
Sea lions were sedated with Zoletil® (~1.3mg/kg, Virbac, Australia), administered intramuscularly via a syringe dart (Paxarms, 3.0ml syringe body with a 14-gauge 25mm barbed needle), delivered remotely by a dart gun (MK24c Projector, Paxarms). After a light level of sedation was attained (~10-15 minutes), sea lions could be approached, allowing application of an anesthetic mask over the muzzle. Sea lions were anesthetized using Isoflurane® (5% induction, 2-3% maintenance with medical-grade oxygen), for ~20 minutes, while instruments were attached. Isoflurane was delivered via a purpose-built gas anesthetic machine, using a Cyprane Tec III vaporizer (The Stinger™ Backpack anesthetic machine, Advanced Anaesthesia Specialists). Throughout anesthesia, vital signs of the sea lions were continuously monitored (e.g. respiratory rate, gum refill, and palpebral reflex), a pulse oximeter was also clipped to the tongue of anesthetized sea lions to monitor heart rate and blood oxygen levels. Following attachment of the instruments, sea lions were maintained on pure oxygen for several minutes until head/body movement indicated imminent recovery.
All biologging (animal-borne) instruments were pre-adhered to neoprene patches, that were then glued to the pelage (fur) on the dorsal midline of sea lions, using a two-part quick-setting epoxy (Selleys Araldite® 5 Minute Epoxy Adhesive). An archival animal-borne camera (Customized Animal Tracking Solutions, 135 x 96 x 40mm, 400g) was fitted to each sea lion, positioned at the base of the scapula, as well as an Argos-linked GPS logger with an integrated time-depth recorder (SPLASH-10, Wildlife Computers, 100 x 65 x 32mm, 200g), which was positioned posterior to the camera. In addition, a triaxial accelerometer/magnetometer (Axy-5 XS, TechnoSmArt, 28 x 12 x 9mm, 4g) was adhered to the crown of the head. Small, light, and low profile biologging instruments were used, where the combined weight of the instruments was <1% of the sea lions’ total body weight, to minimize drag impacts. Instrumented sea lions were recaptured after 2-10 days. Instruments were removed by cutting them from their neoprene patches to avoid damage to the pelage (the neoprene is shed during the subsequent molt).
2.2 Data collection and processing
High-definition color video (forward facing) was collected while sea lions were at sea, at depths greater than 5 meters, during daylight hours (0800-1800 local time). Batteries in the cameras allowed up to 12-13 hours of filming in total, which enabled the collection of video to be spread over 2-3 days of time spent at sea.
Satellite-linked GPS loggers collected Fastloc® locations when sea lions surfaced, by capturing a subsecond snapshot of signals from orbiting satellite constellations at two minute intervals (the minimum rate programmable). When dive durations exceeded two minutes, locations were sought when sea lions next surfaced. Locations obtained from four or fewer satellites were not included in analyses and erroneous locations (identified by unrealistic swimming speeds, >6ms −1 ) were removed, using a speed filter ( McConnell et al., 1992 ; Sumner, 2011 ). Transmissions (including those of GPS location data) from the loggers were received and passed on by Argos systems on polar-orbiting satellites, allowing monitoring of each sea lion’s position in real time. Time-depth recorders measured depth every second.
Triaxial accelerometer/magnetometer data were used in combination with the GPS data to dead-reckon at-sea movement, using the methods outlined in Angelakis et al. (2023) . Accelerometers measured head movement (G-force), for surge (anterior-posterior), sway (lateral) and heave (dorsal-ventral) axes at 25 Hz and 8-bit resolution (maximum and minimum acceleration value ±4G). Magnetometers measured the earth’s magnetic field in microteslas (µT) for roll (longitudinal), pitch (transverse) and yaw (vertical) axes at 2Hz.
2.3 Mapping of benthic habitats in southern Australia
Analysis of animal-borne video was conducted using the open source Behavioral Observation Research Interactive Software (version 7.12.2). A habitat key was used to classify benthic habitats ( Figure 2 ), following the Collaborative and Annotation Tools for Analysis of Marine Imagery and Video classification scheme, which provides a national (Australian) framework for classifying marine biota and substrata ( Althaus et al., 2013 ). The duration of time sea lions spent in different benthic habitats was recorded. All video analysis was performed by a single observer.
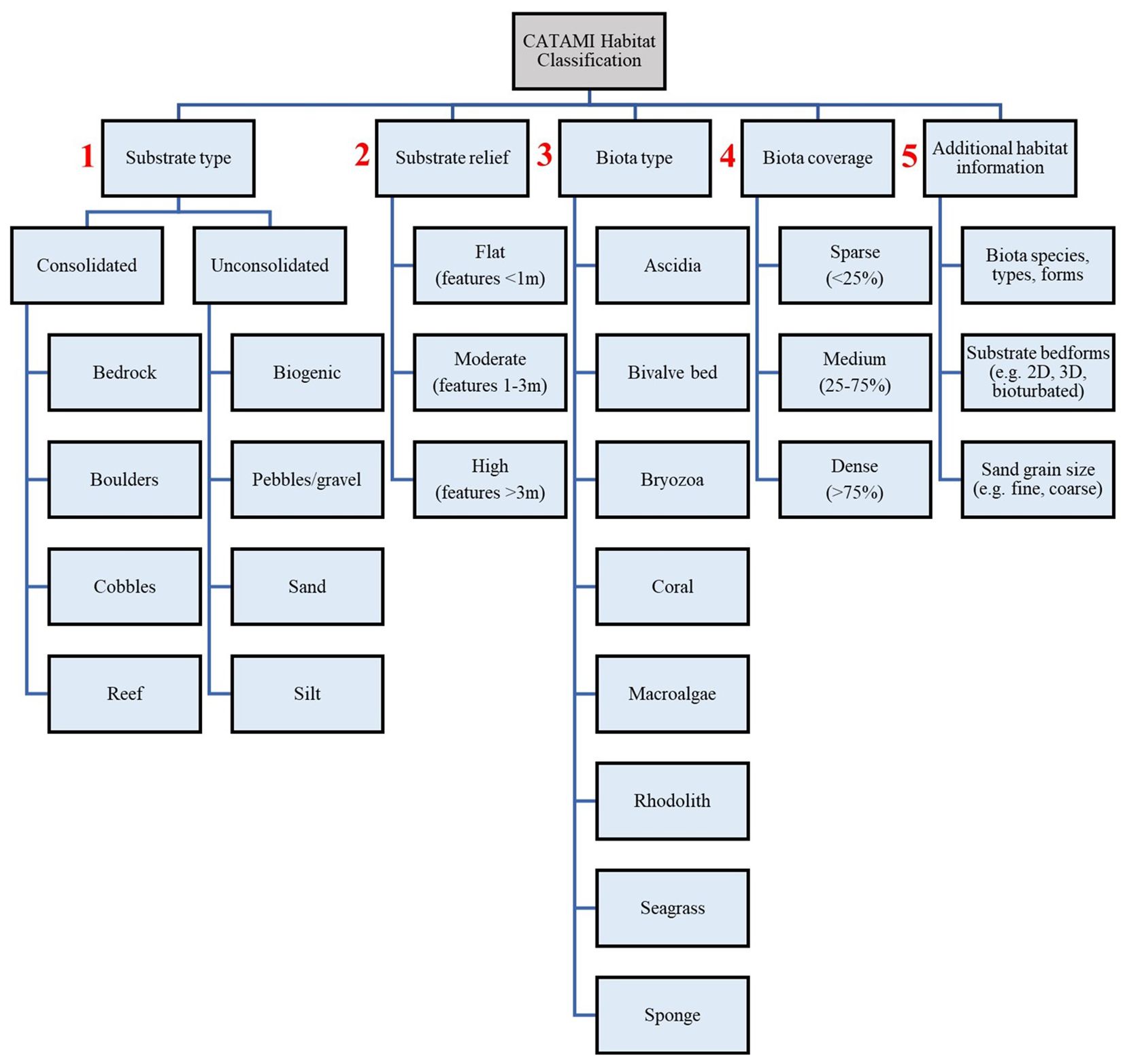
Figure 2 Habitat key used to classify benthic habitats, as identified from animal-borne video from adult female Australian sea lions. Numbers in red highlight the order of stages for habitat classification. Habitat classification was conducted in line with the Collaborative and Automated Tools for Analysis of Marine Imagery scheme.
Benthic habitat data for each sea lion were then georeferenced by time matching and amalgamation with their dead reckoned foraging paths. Georeferencing of the benthic habitat data enabled calculation of the distance travelled (km) in each habitat, from which, percent cover of different habitats could be quantified.
Georeferenced benthic habitat data were then spatially interpolated with available oceanographic/environmental data, to model benthic habitats on the continental shelf, across the sea lions’ foraging ranges from each colony, for Olive Island (32.550 to 32.850°S, 133.720 to 134.050°E, 1,023km 2 ) and Seal Bay (35.980 to 36.800°S, 137.000 to 137.500°E, 4,004km 2 ). The South Australian coast experiences significant coastal upwelling during the austral spring-autumn (November-May), which drives enhanced chlorophyll-a concentrations within the photic layer, leading to highly productive marine conditions ( Kämpf et al., 2004 ; McClatchie et al., 2006 ; Middleton and Bye, 2007 ). Therefore, sea surface temperature and chlorophyll-a data were utilized to assess how they may drive the distribution of benthic habitats on the continental shelf in southern Australia. Sea surface temperature data (index), collected from polar-orbiting and geostationary satellites, were obtained from the National Aeronautics and Space Administration (NASA) Multiscale Ultrahigh Resolution Data (1km grid resolution) ( Chin et al., 2017 ). Chlorophyll-a data (ocean color index) ( Hu et al., 2012 ), also collected via satellites, were obtained from the National Oceanic and Atmospheric Administration (NOAA) Ocean Color Data (1km grid resolution). To model benthic habitats, we used long-term averaged sea surface temperature and chlorophyll-a data, over the previous ~21 years (between May 2002 and November 2023), across the two study regions. To assess how depth may drive the distribution of benthic habitats on the continental shelf, bathymetric data (m) were obtained from the General Bathymetric Chart of the Ocean (GEBCO) (15 arc-second grid resolution). Kriging was used to interpolate sea surface temperature, chlorophyll-a and bathymetry data across both study regions, using the gstat package in R ( Pebesma and Graeler, 2015 ). This interpolation allowed the matching of data for each ‘presence’ location (where we had benthic habitat data), and each ‘absence’ location (where the benthic habitat was unknown) and scaled each predictor variable to the same spatial resolution, for all presences and absences. Additionally, for each presence and absence location, distance from the nearest coastline and distance from the continental slope (at the 200m depth contour), were calculated in R using the Haversine formula ( Robusto, 1957 ), to assess how the distributions of these benthic habitats may be driven by the geomorphometry of the continental shelf.
Sea surface temperature, chlorophyll-a, bathymetry, distance from the coast and distance from the slope, were then used to predict benthic habitats for the study regions around Olive Island (1,023km 2 ) and Seal Bay (4,004km 2 ). Random Forest models ( randomForest package in R ) were chosen to predict benthic habitats, as they are suitable for modelling scenarios where variables have complex interactions and nonlinear relationships ( Breiman, 2001 ; Liaw and Wiener, 2002 ) and thus are particularly useful for ecological studies. Random forests, which can be used for both regression and classification tasks, are widely used for habitat modelling ( Juel et al., 2015 ; Rather et al., 2020 ; Shanley et al., 2021 ).
Firstly, individual random forest models for Olive Island and Seal Bay were validated by randomly subsetting the presence data into a ‘training’ dataset (using two-thirds of the presence data) and a ‘test’ dataset (using a third of the presence data). A confusion matrix was calculated to assess the predictive performance of both models (their accuracy in correctly classifying known habitats in their test datasets). Trained and tested random forest models were then used to predict benthic habitats for the absence data. The optimal number of classification trees (300), used in models, was identified by comparing mean squared error rates with an increasing number of classification trees, until error rates stabilized ( Supplementary Figure 1 ). Random forest models were then ‘tuned’ using the tuneRF function in the randomForest package, which uses out-of-bag error estimates to find the optimal ‘mtry’ parameter entry (2), which represents the optimal number of features to consider at each ‘split’ in the model. Finally, models were cross validated, which tested their performance by iteratively reducing the number of predictor variables within the model, to find the optimal selection of parameters, determined by their mean squared error rates ( Supplementary Figure 2 ). Variable importance in random forest models was assessed by the mean decrease in the Gini coefficient, which measured the influence of each predictor variable on the models’ ability to distinguish different benthic habitats (higher values indicating greater influence on the models’ benthic habitat predictions).
3.1 Foraging paths and cover of benthic habitats
From the eight adult female Australian sea lions observed from Olive Island (OI1, OI2, OI3, OI4) and Seal Bay (SB1, SB2, SB3, SB4), a total of 89 hours and 9 minutes of animal-borne video from 1,935 dives was available for analysis. A summary of animal-borne video data available for each sea lion are provided in the Supplementary Material ( Supplementary Table 1 ). Animal-borne video recorded a total of ~560km of the benthos (Olive Island= ~223km, Seal Bay= ~337km) ( Figure 3 ) and captured benthic habitats at depths between 5-110m.
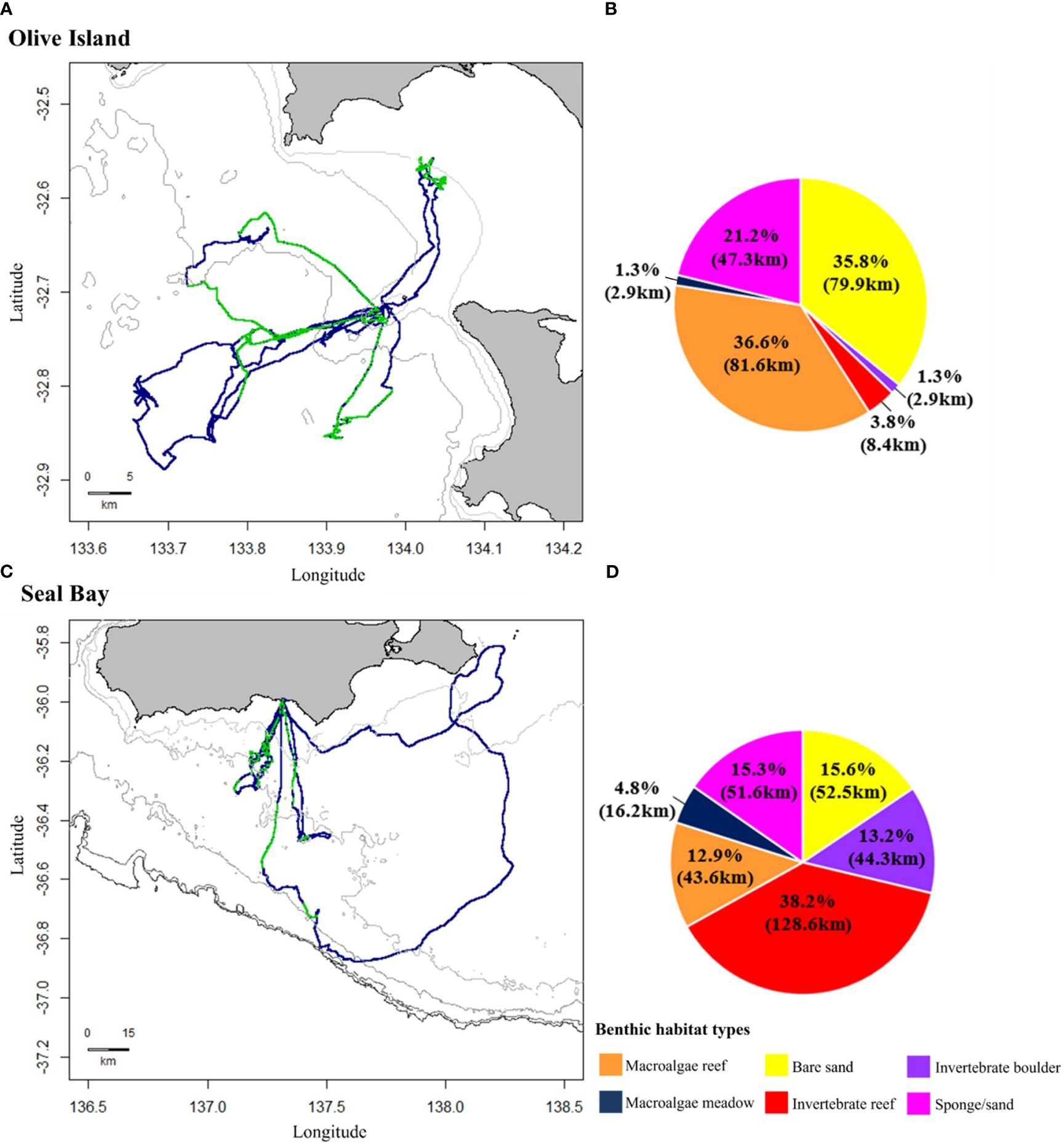
Figure 3 Movement and benthic habitat data from adult female Australian sea lions from Olive Island, western Eyre Peninsula ( n = 4) and Seal Bay, Kangaroo Island ( n = 4) in South Australia. Dead reckoned foraging paths represent at-sea movement in blue, with regions where animal-borne video data were available in green for sea lions from (A) Olive Island and (C) Seal Bay. Isobaths represent depth contours at 10, 25 and 50m for Olive Island (A) and 50, 75, 100, 150 and 200m for Seal Bay (C) (light to dark grey). Pie charts represent percent cover (km) of different benthic habitats on the foraging paths of sea lions from (B) Olive Island and (D) Seal Bay: macroalgae reef (orange), macroalgae meadow (navy), bare sand (yellow), invertebrate reef (red), invertebrate boulder (purple) and sponge/sand habitats (pink).
Six benthic habitats were identified from animal-borne video from Australian sea lions from Olive Island and Seal Bay: macroalgae reef, macroalgae meadow, bare sand, sponge/sand, invertebrate reef and invertebrate boulder habitats. Percent cover of these benthic habitats differed on the foraging paths of sea lions from Olive Island and Seal Bay ( Figure 3 ). For sea lions from Olive Island, macroalgae reef (36.6%, 81.6km), bare sand (35.8%, 79.9km) and sponge/sand habitats (21.2%, 47.3km) accounted for most of the habitat cover ( Figure 3B ). For sea lions from Seal Bay, invertebrate reef (38.2%, 128.6km), bare sand (15.6%, 52.5km), sponge/sand (15.3%, 51.6km) and invertebrate boulder habitats (13.2%, 44.3km) accounted for most of the habitat cover ( Figure 3D ).
Of the macroalgae habitats, many of the reef environments were dominated by Ecklonia radiata (golden kelp) ( Figure 4 ), other macroalgae habitats consisted of varying assemblages of different brown, red and green algae taxa, such as Sargassum , Cystophora , Plocamium and Ulva species. Sponge/sand habitats were dominated by Demospongiae sponges, such as Callyspongia and Echinodictyum species ( Figure 4 ). Invertebrate reef and boulder habitats were also dominated by Demospongiae sponges, as well as bryozoans such as Phidoloporidae (lace coral) species, ascidians from Phlebobranchia and the Pyura genus (sea tulips) and soft corals from Alcyonacea (gorgonian species) and the Dendronephythya genus ( Figure 4 ).
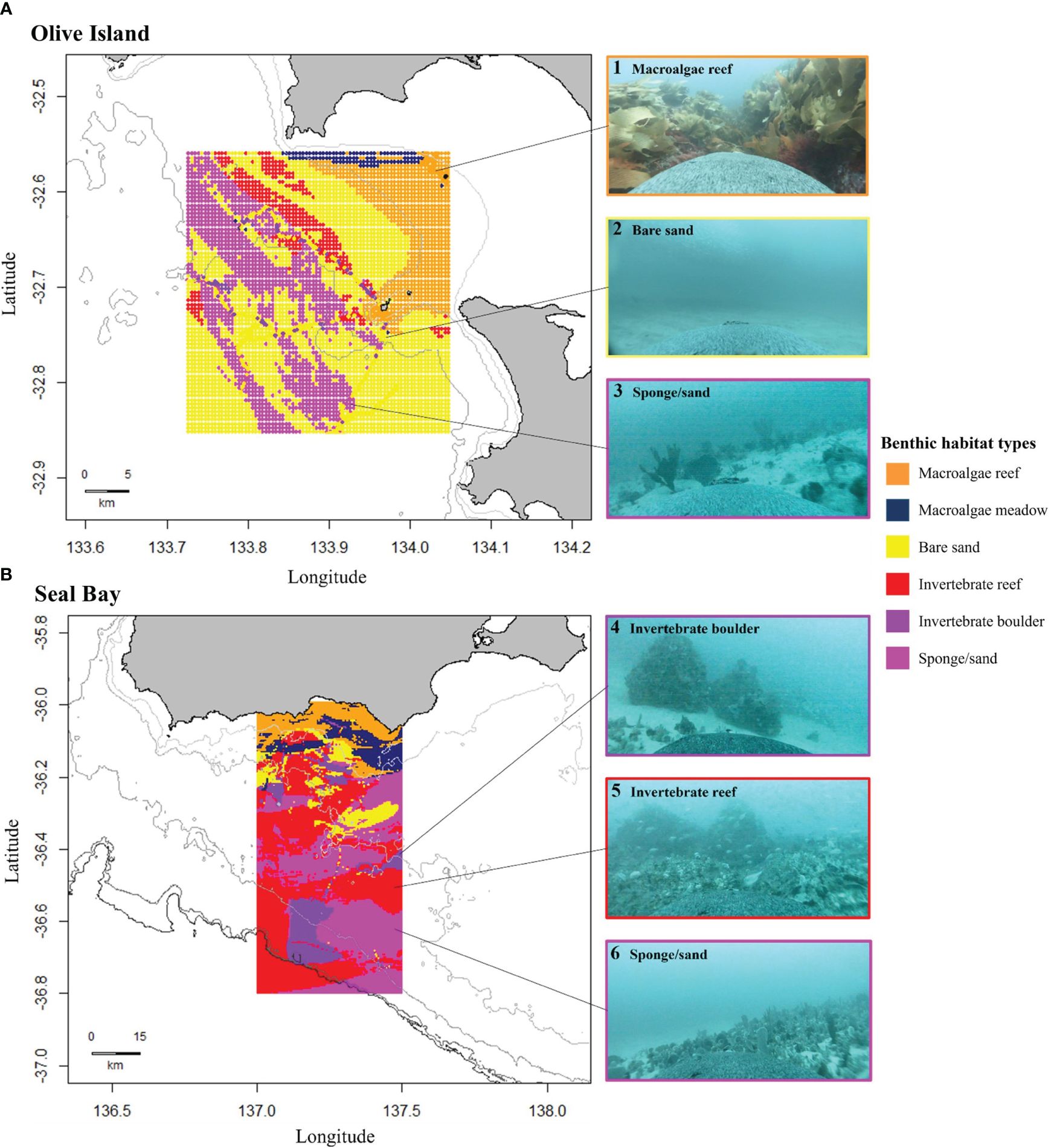
Figure 4 Modelled distributions of benthic habitats for (A) Olive Island, western Eyre Peninsula, and (B) Seal Bay, Kangaroo Island in South Australia. Maps show predicted distributions of benthic habitats from random forest modelling of animal-borne video data from adult female Australian sea lions ( n = 8). Habitat distributions are: macroalgae reef (orange), macroalgae meadow (navy), bare sand (yellow), invertebrate reef (red), invertebrate boulder (purple) and sponge/sand (pink) habitats. Isobaths represent depth contours at 10, 25 and 50m for Olive Island (A) and 50, 75, 100, 150 and 200m for Seal Bay (B) . Examples of captured images are: 1) macroalgae reef, 2) bare sand, 3 and 6) sponge/sand, 4) invertebrate boulder and 5) invertebrate reef habitats.
The percent cover of flat (features <1m), moderate (features 1-3m) and high relief reefs (features >3m) differed between Olive Island and Seal Bay ( Supplementary Figure 3 ). Additionally, the percent biota cover of sparse (<25% cover), medium (25-75% cover) and dense (>75% cover) macroalgae, invertebrate and sponge habitats also differed between Olive Island and Seal Bay ( Supplementary Figure 3 ).
3.2 Predicting distributions of benthic habitats
A trained random forest model for Olive Island predicted benthic habitats on a test dataset with a 99.5% accuracy rate (out-of-bag error rate= 0.5%) and for Seal Bay, benthic habitats were predicted at a 98.6% accuracy rate (out-of-bag error rate= 1.4%). Both random forest models for Olive Island and Seal Bay showed high precision when predicting across all identified benthic habitats ( Supplementary Table 2 ). For Olive Island and Seal Bay, prediction accuracies were highest when all five predictor variables (sea surface temperature, chlorophyll-a, bathymetry, distance from the coast and distance from the continental slope), were included in their models ( Supplementary Figure 2 ).
Predicted benthic habitats varied between the regions around Olive Island and Seal Bay ( Figure 4 ). For Olive Island, macroalgae reefs were predicted for inshore waters to the northeast, constituting most of the predicted habitat at depths shallower than ~25-30m ( Figure 4A ). Bare sand and sponge/sand habitats were predicted as the dominant habitats at depths greater than ~25-30m, with smaller areas of invertebrate reefs, mostly predicted to the northwest of Olive Island ( Figure 4A ). For Seal Bay, macroalgae reef and macroalgae meadow habitats were predicted as the dominant benthic habitats at depths shallower than ~50-60m ( Figure 4B ). For depths greater than ~50-60m, sponge/sand and invertebrate reef habitats were the dominant predicted habitats, with smaller areas of bare sand and invertebrate boulder habitats south of Seal Bay ( Figure 4B ). For Olive Island, these habitats also appeared to have a distinct southeast-northwest orientation, corresponding with local bathymetry and/or the aspect of the continental slope ( Figure 4A ).
For Olive Island, a random forest model showed that chlorophyll-a and distance from the continental slope were the most important variables for predicting benthic habitat (mean decrease in the Gini coefficient= 31280.28 and 23514.66 respectively) ( Figure 5A ). For Seal Bay, distance from the coast and chlorophyll-a were the most important variables for predicting benthic habitat (mean decrease in the Gini coefficient= 18136.47 and 18019.99 respectively) ( Figure 5B ).
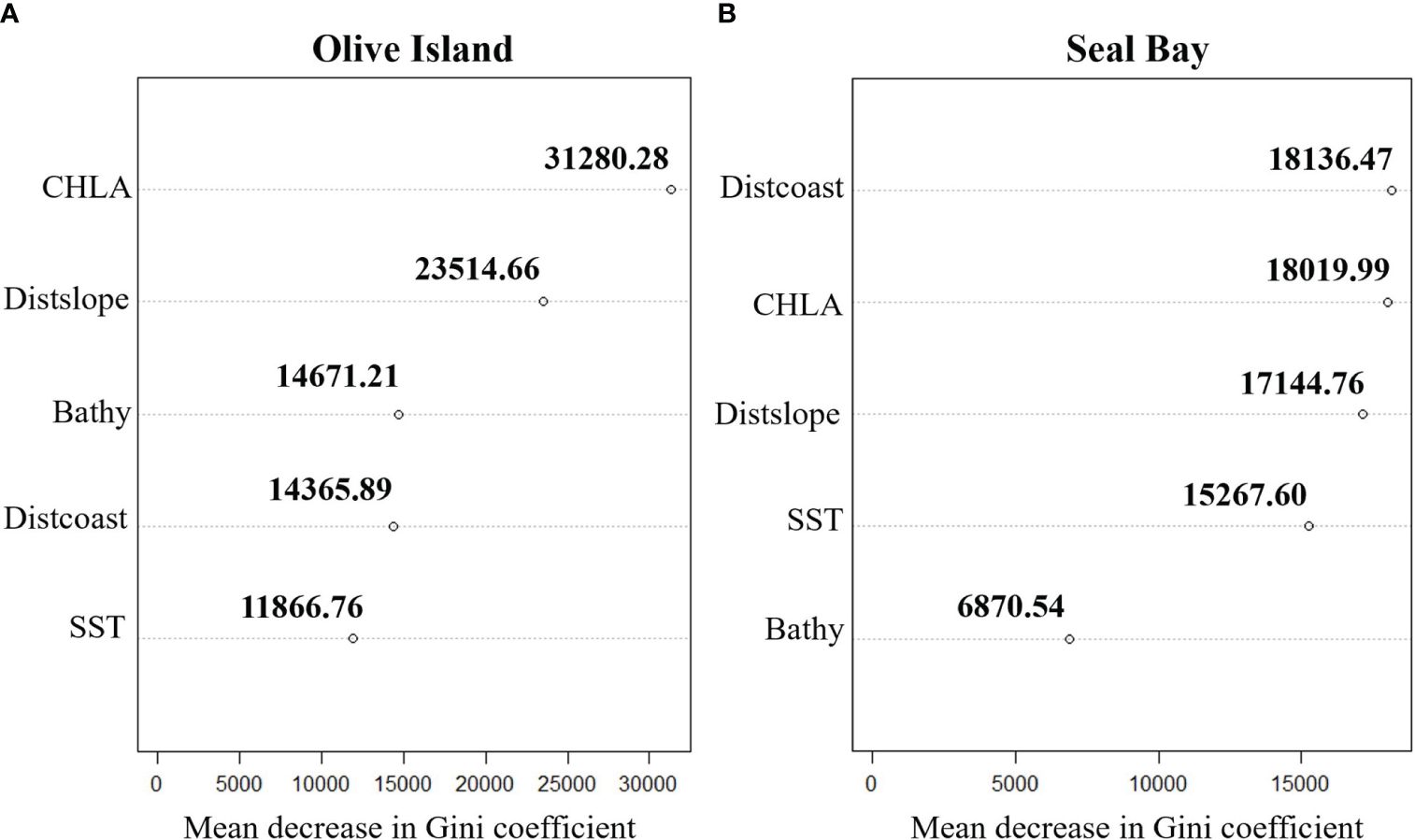
Figure 5 Cleveland dot plots highlighting the importance of predictor variables: chlorophyll-a (CHLA), distance from the continental slope (Distslope), bathymetry (Bathy), distance from the coastline (Distcoast) and sea surface temperature (SST) in random forest models for predicting benthic habitats for (A) Olive Island and (B) Seal Bay in South Australia. Mean decrease in Gini coefficient values represent the importance of each predictor variable in random forest models, where higher values indicate a greater importance in predicting benthic habitats.
4 Discussion
4.1 distribution and structure of benthic habitats in south australia.
In this study, benthic habitat data collected from animal-borne video was used in a random forest model to predict the spatial distribution of diverse benthic habitats on the continental shelf in southern Australia. From these sea lions, six benthic habitats (between 5-110m depth) were identified at Olive Island and Seal Bay: macroalgae reef, macroalgae meadow, bare sand, sponge/sand, invertebrate reef and invertebrate boulder habitats. Random forest models predicted that large regions of the continental shelf in southern Australia are covered by invertebrate reef, bare sand and sponge/sand habitats. Animal-borne video and movement data from Australian sea lions was also useful in locating reefs, highlighting significant high relief reef systems, for example, the area at 36.100 to 36.300°S and 137.170 to 137.280°E, south of Kangaroo Island ( Figure 6 ).
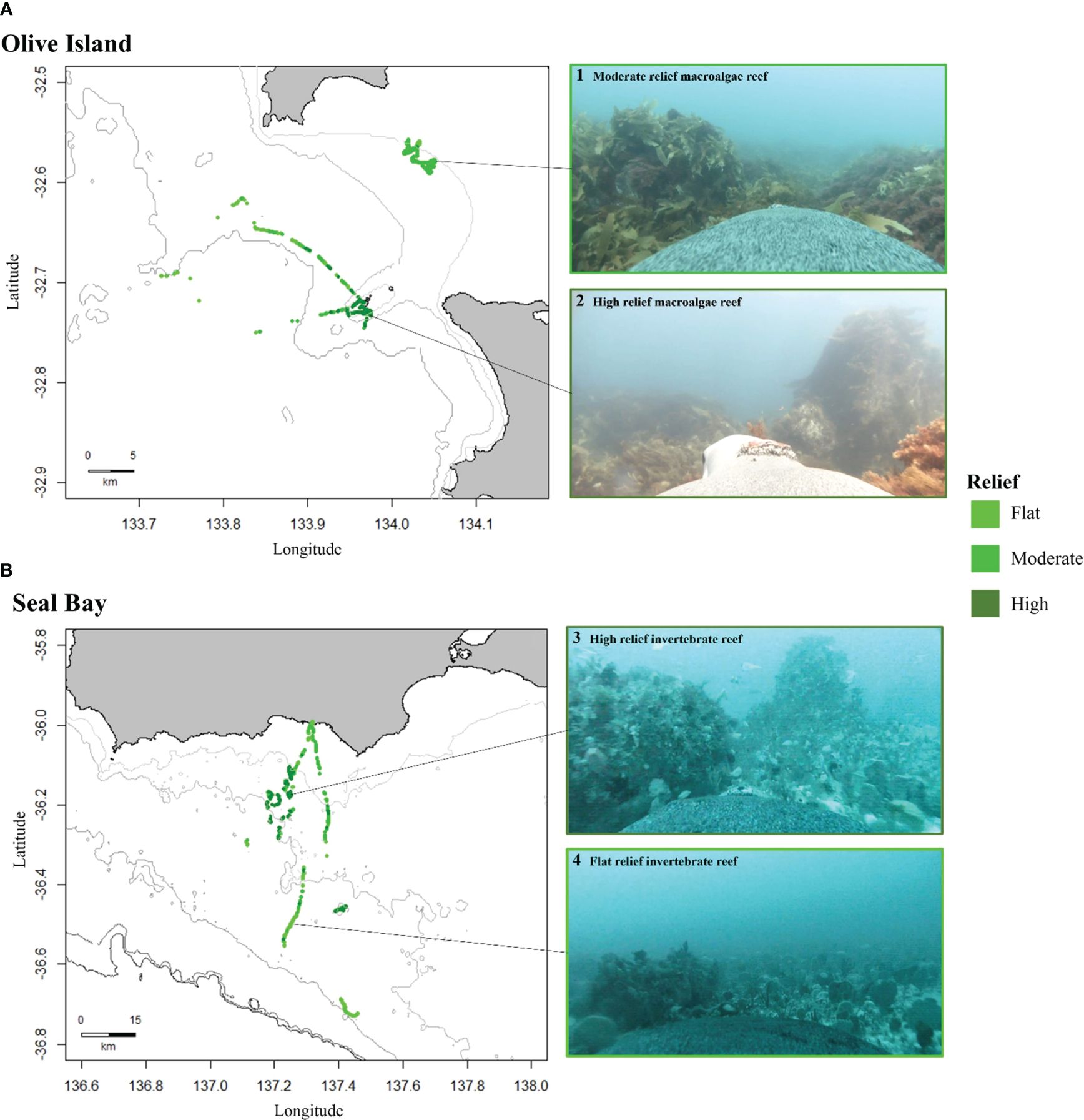
Figure 6 Distribution of reef habitats for (A) Olive Island, western Eyre Peninsula, and (B) Seal Bay, Kangaroo Island in South Australia. Maps show the distribution of flat (features <1m, light green), moderate (features 1-3m, green) and high relief reefs (features >3m, dark green), as identified from animal-borne video data from adult female Australian sea lions ( n = 8). Isobaths represent depth contours at 10, 25 and 50m for Olive Island (A) and 50, 75, 100, 150 and 200m for Seal Bay (B) (light to dark grey). Examples of captured images are: 1) moderate relief macroalgae reef, 2) high relief macroalgae reef, 3) high relief invertebrate reef and 4) flat relief invertebrate reef.
The habitat assemblages identified in this study differ from other regions in South Australia where benthic habitats have been mapped across broad spatial scales, which has been restricted to the sheltered embayments of its two gulfs. Bare sand plains and seagrass meadows cover large areas of the Gulf St. Vincent ( Shepherd and Sprigg, 1976 ; Tanner, 2005 ), and sediment surveys have inferred that a combination of seagrass meadows, sand and gravel plains and rhodolith pavements are prevalent in the Spencer Gulf ( O'Connell et al., 2016 ). In this study of continental shelf waters, particular benthic habitats like seagrass meadows (such as Posidonia and Amphibolis sp.) were not observed. However, animal-borne video has previously identified seagrass meadows as foraging habitat for Australian sea lions from Dangerous Reef in the southern Spencer Gulf (Angelakis, in review). Light penetration, water depth, wave energy and turbidity in the high energy waters around Olive Island and Seal Bay, are all factors which likely explain the apparent absence of seagrass habitat in these regions ( Shepherd and Sprigg, 1976 ; Tanner, 2005 ; O'Connell et al., 2016 ). However, some inherent spatial biases may exist with the data presented in this study, as Australian sea lions may prefer particular benthic habitats over others. Therefore, other benthic habitats may occupy these regions but were not observed in the video data if sea lions did not target them or transit over them and hence were not accounted for in random forest models.
4.2 Environmental drivers of benthic habitat
We found that invertebrate communities dominated depths where macroalgae reefs and macroalgae meadows were absent. The sponge, bryozoan, ascidian and soft coral taxa identified in this study align with those taxa previously described in the region ( Sorokin et al., 2007 ; Sorokin and Currie, 2008 ; Burnell et al., 2015 ). In the Great Australian Bight, benthic habitat surveys have identified invertebrate communities with a diverse range of sponge, ascidian, and bryozoan species ( McLeay et al., 2003 ; Ward et al., 2006a ; Sorokin et al., 2007 ). The distribution and structure of these invertebrate communities is likely influenced by a range of environmental factors, including nutrient supply, bathymetry, substrate availability, seawater conditions and hydrodynamics ( Ward et al., 2006 ; Currie et al., 2009 ; James and Bone, 2010 ; Przeslawski et al., 2011 ). The environmental variables used to predict benthic habitats in this study, potentially provide insights into the suite of oceanographic processes driving the distribution and structure of these invertebrate communities.
Nutrient supply is key for supporting filter-feeding benthic invertebrates ( Ward et al., 2006 . 2006a ; Middleton et al., 2014 ). During the austral spring-autumn (November-May), South Australia experiences extensive coastal upwelling of cold nutrient-rich waters, which drive enhanced chlorophyll-a concentrations within the photic layer, leading to highly productive marine conditions ( Kämpf et al., 2004 ; McClatchie et al., 2006 ; Middleton and Bye, 2007 ). Therefore, the large sponge, ascidian, bryozoan and soft coral communities identified here, may be supported in part by strong seasonal upwelling conditions, where there is an enhanced supply of nutrients to the benthos ( James et al., 2001 ; James and Bone, 2010 ; Middleton et al., 2014 ). Changes in water circulation across southern Australia, due to temporal shifts in current patterns, outflows from gulf waters, eddies and salinity and temperature fronts, also drive nutrient transport ( James et al., 2001 ; Middleton and Bye, 2007 ; van Ruth et al., 2018 ). The distribution of invertebrate communities across southern Australia is therefore likely influenced by a range of complex and highly dynamic oceanographic processes, that drive the supply of nutrients and trophic resources to different areas and habitats. In random forest models for both Olive Island and Seal Bay, chlorophyll-a was one of the most important variables for predicting benthic habitat, supporting the notion that supply of nutrients/trophic resources is one key determinant of benthic habitats at depth.
Conversely, some regions of the continental shelf, observed in this study, were dominated by bare sand plains. These regions may represent areas where benthic environments receive less nutrient input ( Middleton et al., 2014 ; Menge et al., 2019 ), or are subject to regular swell/current impacts, compared with benthic habitats dominated by diverse sponge, ascidian, bryozoan and soft coral communities. Presumably, substrate availability ( James et al., 2001 ), turbidity, swell/current action ( Ward et al., 2006a ) and seawater conditions ( Middleton et al., 2014 ) are also critical in determining suitable areas where sessile invertebrates can establish. In this study, fine sand (no shell fragments) and coarse sand (with shell fragments) ( Althaus et al., 2013 ), dominated sediment composition. The absence of mud/silt sediments is likely due to the high level of exposure and water movement on the continental shelf ( James et al., 2001 ; Currie et al., 2009 ), at depths where the sea lions foraged at (<110m). This aligns with data for the Great Australian Bight, where coarser sediments dominated shallower waters ( Ward et al., 2006a ; Currie et al., 2009 ) and mud sediments dominated depths below 150m, where direct influences from swell and current action are much smaller ( James et al., 2001 ; Currie et al., 2009 ). A better understanding of how different oceanographic and environmental processes influence the structure and distribution of benthic habitats will be key for future research and habitat assessment.
4.3 Future applications
Using animal-borne video to explore and map benthic habitats provides information that complements traditional benthic survey methods, such as ROV, towed and drop camera deployments, acoustic mapping, and sled and grab sampling ( Ward et al., 2006a ; Kostylev, 2012 ; López-Garrido et al., 2020 ). Animal-borne video from a benthic foraging marine mammal, such as the Australian sea lion, provides an efficient and cost-effective method for mapping and surveying benthic habitats, particularly for those at depths that are expensive, difficult, and impossible to access by more conventional survey approaches. Animal-borne video highlights the ecological value of different habitats from a predator’s perspective, valuable sea lion habitat may also highlight ecologically important areas more broadly. In future, combining animal-borne video with data from these existing survey methods will support a more comprehensive understanding of benthic habitats, and the species that use them. Furthermore, future animal-borne camera deployments on Australian sea lions, which expand the spatial extent of available benthic habitat data, will enhance the robustness and generalizability of the models developed in this study. Benthic habitats can be significantly altered by human activity ( Brown et al., 2017 ; Sweetman et al., 2017 ; Yoklavich et al., 2018 ). In southern Australia, benthic habitats have undergone major changes, since the arrival of Europeans, from land-based release of nutrients, and from the impacts of fisheries ( Tanner, 2005 ; Bryars and Rowling, 2009 ; Gorman et al., 2009 ; Alleway and Connell, 2015 ). Yet, our knowledge of benthic habitats, including how quickly they may recover from degradation, is poorly understood. The benthic habitats in southern Australia are highly diverse and endemic, with many local undescribed taxa ( Edyvane, 1999 ; McLeay et al., 2003 ; Currie et al., 2009 ). Across southern Australia, large spatially connected temperate reef systems have been identified, which support extensive kelp and fucoid forests and diverse benthic invertebrate communities that have significant ecological, social and economic importance ( Bennett et al., 2015 ; Coleman and Wernberg, 2017 ; Wong et al., 2023 ). Considering the gaps in our knowledge around benthic habitats ( Mayer et al., 2018 ; Menandro and Bastos, 2020 ) and the human impacts on them ( Tanner, 2005 ; Bryars and Rowling, 2009 ; Gorman et al., 2009 ; Alleway and Connell, 2015 ), there is a necessity to better understand their structure and distribution, throughout southern Australia and globally. Where such information has been gathered, it has led to better policies and investment to recover these habitats and the economies and social benefits derived from them ( Gorman et al., 2009 ; McAfee et al., 2020 ). In South Australia, an improved knowledge of benthic habitats can support wide-ranging fields of marine science, from improving placement of marine reserves, habitat restoration planning and management of endangered species, such as the Australian sea lion.
4.4 Conclusions
This study presents novel findings on previously unmapped areas of the continental shelf in southern Australia. Random forest models demonstrated strong performance in predicting diverse benthic habitats across extensive regions of the continental shelf. To ground truth these models, predicted habitats in the absence locations could be compared with future benthic habitat data collected from various sources, such as animal-borne video, ROVs, towed and drop cameras and autonomous underwater vehicles (AUVs), provided there is spatial overlap. This research highlights the utility of random forest models in mapping and predicting habitats observed through animal-borne video, particularly those associated with benthic predators such as the Australian sea lion. Furthermore, this study highlights the value of ancillary data collected from animal-borne video, beyond solely investigating animal behavior, and illustrates how future research could repurpose such data in novel ways, to address important research objectives in the marine environment.
Data availability statement
The datasets presented in this study can be found in online repositories. The names of the repository/repositories and accession number(s) can be found below: https://metadata.imas.utas.edu.au/geonetwork/srv/eng/catalog.search#/metadata/84cb1709-a669-4f2c-b97b-5eceb7929349 .
Ethics statement
The animal study was approved by The University of Adelaide Animal Ethics Committee (#S-2021-001), Primary Industries and Regions South Australia Animal Ethics Committee (#16/20), and the Department for Environment and Water (Permit/Licence to Undertake Scientific Research #A24684-22/23 and Marine Parks Permit to Undertake Scientific Research #MR00071-7-R). The study was conducted in accordance with the local legislation and institutional requirements.
Author contributions
NA: Conceptualization, Data curation, Formal analysis, Funding acquisition, Investigation, Methodology, Project administration, Validation, Visualization, Writing – original draft, Writing – review & editing. GG: Conceptualization, Formal analysis, Methodology, Visualization, Writing – review & editing, Investigation. SC: Conceptualization, Formal analysis, Methodology, Project administration, Supervision, Validation, Writing – review & editing. FB: Conceptualization, Formal analysis, Methodology, Visualization, Writing – review & editing. LD: Formal analysis, Investigation, Methodology, Validation, Visualization, Writing – review & editing. RK: Data curation, Visualization, Writing – review & editing. DH: Data curation, Investigation, Resources, Writing – review & editing. SG: Data curation, Funding acquisition, Project administration, Supervision, Writing – review & editing.
The author(s) declare financial support was received for the research, authorship, and/or publication of this article. Research for this manuscript was funded by the Australian Government under the National Environmental Science Program (NESP), Marine and Coastal Hub (Project 2.6, Mapping critical Australian sea lion habitat to assess ecological value and risks to population recovery). Additional operating costs were funded by the Ecological Society of Australia under the Holsworth Wildlife Research Endowment, awarded to Nathan Angelakis (006010901).
Acknowledgments
We would like to acknowledge Mel Stonnill, Ashleigh Wycherley and the Department for Environment and Water (DEW) staff at Seal Bay Conservation Park and the staff of the Kangaroo Island Veterinary Clinic. Thanks are also extended to Carey Kuhn (National Oceanic and Atmospheric Administration NOAA), Hugo Oliveira de Bastos (SARDI Aquatic Sciences), Dale Furley (deceased) and the staff of the Far-West Coast Aboriginal Corporation (FWCAC), Tobin Woolford (Eyrewoolf Abalone) and Bec Souter. We also thank SARDI Aquatic Sciences and The University of Adelaide for their continued support.
Conflict of interest
The authors declare that the research was conducted in the absence of any commercial or financial relationships that could be construed as a potential conflict of interest.
Publisher’s note
All claims expressed in this article are solely those of the authors and do not necessarily represent those of their affiliated organizations, or those of the publisher, the editors and the reviewers. Any product that may be evaluated in this article, or claim that may be made by its manufacturer, is not guaranteed or endorsed by the publisher.
Supplementary material
The Supplementary Material for this article can be found online at: https://www.frontiersin.org/articles/10.3389/fmars.2024.1425554/full#supplementary-material
Alleway H. K., Connell S. D. (2015). Loss of an ecological baseline through the eradication of oyster reefs from coastal ecosystems and human memory. Conserv. Biol. 29 (3), 795–804. doi: 10.1111/cobi.12452
PubMed Abstract | CrossRef Full Text | Google Scholar
Althaus F., Hill N., Edwards L., Ferrari R., Case M., Colquhoun J., et al. (2013). CATAMI Classification Scheme for scoring marine biota and substrata in underwater imagery—A pictorial guide to the Collaborative and Annotation Tools for Analysis of Marine Imagery and Video (CATAMI) classification scheme. Version 1.3 .
Google Scholar
Angelakis N., Goldsworthy S. D., Connell S. D., Durante L. M. (2023). A novel method for identifying fine-scale bottom-use in a benthic-foraging pinniped. Movement Ecol. 11, 1–11. doi: 10.1186/s40462-023-00386-1
CrossRef Full Text | Google Scholar
Bennett S., Wernberg T., Connell S. D., Hobday A. J., Johnson C. R., Poloczanska E. S. (2015). The ‘Great Southern Reef’: social, ecological and economic value of Australia’s neglected kelp forests. Mar. Freshw. Res. 67, 47–56. doi: 10.1071/MF15232
Berry T. E., Osterrieder S. K., Murray D. C., Coghlan M. L., Richardson A. J., Grealy A. K., et al. (2017). DNA metabarcoding for diet analysis and biodiversity: A case study using the endangered Australian sea lion ( Neophoca cinerea ). Ecol. Evol. 7 (14), 5435–5453. doi: 10.1002/ece3.3123
Breiman L. (2001). Random forests. Mach. Learn. 45, 5–32. doi: 10.1023/A:1010933404324
Brown K. T., Bender-Champ D., Bryant D. E., Dove S., Hoegh-Guldberg O. (2017). Human activities influence benthic community structure and the composition of the coral-algal interactions in the central Maldives. J. Exp. Mar. Biol. Ecol. 497, 33–40. doi: 10.1016/j.jembe.2017.09.006
Bryars S., Rowling K. (2009). Benthic habitats of eastern Gulf St Vincent: major changes in benthic cover and composition following European settlement of Adelaide. Trans. R. Soc. S. Aust. 133 (2), 318–338.
Burnell O., Barrett S., Hooper G., Beckmann C., Sorokin S., Noell C. (2015). Report to PIRSA Fisheries and Aquaculture (South Australian Research and Development Institute (Aquatic Sciences), Adelaide. SARDI Publication No. F2015/ 000414-1. SARDI Research Report Series No. 860). Spatial and temporal reassessment of by-catch in the Spencer Gulf prawn fishery.
Button R. E., Parker D., Coetzee V., Samaai T., Palmer R. M., Sink K., et al. (2021). ROV assessment of mesophotic fish and associated habitats across the continental shelf of the Amathole region. Sci. Rep. 11 (1), 18171. doi: 10.1038/s41598-021-97369-2
Chapple T. K., Tickler D., Roche R. C., Bayley D. T., Gleiss A. C., Kanive P. E., et al. (2021). Ancillary data from animal-borne cameras as an ecological survey tool for marine communities. Mar. Biol. 168 (7), 106. doi: 10.1007/s00227-021-03916-w
Chin T. M., Vazquez-Cuervo J., Armstrong E. M. (2017). A multi-scale high-resolution analysis of global sea surface temperature. Remote Sens. Environ. 200, 154–169. doi: 10.1016/j.rse.2017.07.029
Coleman M. A., Wernberg T. (2017). Forgotten underwater forests: The key role of fucoids on Australian temperate reefs. Ecol. Evol. 7, 8406–8418. doi: 10.1002/ece3.3279
Connell S. D., Russell B. D., Turner D. J., Shepherd S. A., Kildea T., Miller D., et al. (2008). Recovering a lost baseline: missing kelp forests from a metropolitan coast. Mar. Ecol. Prog. Ser. 360, 63–72. doi: 10.3354/meps07526
Costa D. P., Gales N. J. (2003). Energetics of a benthic diver: seasonal foraging ecology of the Australian sea lion, Neophoca cinerea. Ecol. Monogr. 73 (1), 27–43. doi: 10.1890/0012-9615(2003)073[0027:EOABDS]2.0.CO;2
Currie D. R., Sorokin S. J., Ward T. M. (2009). Infaunal macroinvertebrate assemblages of the eastern Great Australian Bight: effectiveness of a marine protected area in representing the region’s benthic biodiversity. Mar. Freshw. Res. 60 (5), 459–474. doi: 10.1071/MF08239
Currie D. R., Ward T. M., Sorokin S. J. (2007). Infaunal assemblages of the eastern Great Australian Bight: Effectiveness of a Benthic Protection Zone in representing regional biodiversity (South Australian Research and Development Institute (Aquatic Sciences), Adelaide. SARDI Publication No. F2007/ 001079-1. SARDI Research Report Series No. 250).
Diaz R. J., Solan M., Valente R. M. (2004). A review of approaches for classifying benthic habitats and evaluating habitat quality. J. Environ. Manage. 73, 165–181. doi: 10.1016/j.jenvman.2004.06.004
Edyvane K. S. (1999). Conserving Marine Biodiversity in South Australia - Part 1 - Background, status and review of approach to marine biodiversity conservation in South Australia (South Australian Research and Development Institute. SARDI Research Report Series No. 38).
Fowler S. L., Costa D. P., Arnould J. P., Gales N. J., Kuhn C. E. (2006). Ontogeny of diving behaviour in the Australian sea lion: trials of adolescence in a late bloomer. J. Anim. Ecol. 75 (2), 358–367. doi: 10.1111/j.1365-2656.2006.01055.x
Gallagher A. J., Brownscombe J. W., Alsudairy N. A., Casagrande A. B., Fu C., Harding L., et al. (2022). Tiger sharks support the characterization of the world’s largest seagrass ecosystem. Nat. Commun. 13, 6328. doi: 10.1038/s41467-022-33926-1
Goldsworthy S. D. (2015). “Neophoca cinerea,” in The IUCN Red List of Threatened Species . doi: 10.2305/IUCN.UK.2015-2.RLTS.T14549A45228341.en
Goldsworthy S. D., Bailleul F., Nursey-Bray M., Mackay A. I., Oxley A., Reinhold S.-L., et al. (2019). Assessment of the impacts of seal populations on the seafood industry in South Australia (South Australian Research and Development Institute (Aquatic Sciences), Adelaide, June).
Goldsworthy S. D., Hamer D. J., Page B. (2007). Assessment of the implications of interactions between fur seals and sea lions and the southern rock lobster and gillnet sector of the Southern and Eastern Scalefish and Shark Fishery (SESSF) in South Australia (South Australian Research and Development Institute (Aquatic Sciences), Adelaide. SARDI Publication No. F2007/000711. SARDI Research Report Series No. 225).
Goldsworthy S. D., Page B., Hamer D. J., Lowther A. D., Shaughnessy P. D., Hindell M. A., et al. (2022). Assessment of Australian sea lion bycatch mortality in a gillnet fishery, and implementation and evaluation of an effective mitigation strategy. Front. Mar. Sci. 9, 799102. doi: 10.3389/fmars.2022.799102
Goldsworthy S. D., Shaughnessy P. D., Mackay A. I., Bailleul F., Holman D., Lowther A. D., et al. (2021). Assessment of the status and trends in abundance of a coastal pinniped, the Australian sea lion. Neophoca cinerea. Endang. Species Res. 44, 421–437. doi: 10.3354/esr01118
Gorman D., Russell B. D., Connell S. D. (2009). Land-to-sea connectivity: linking human-derived terrestrial subsidies to subtidal habitat change on open rocky coasts. Ecol. Appl. 19, 1114–1126. doi: 10.1890/08-0831.1
Hu C., Lee Z., Franz B. (2012). Chlorophyll a algorithms for oligotrophic oceans: A novel approach based on three-band reflectance difference. J. Geophys. Res. Oceans 117, C1. doi: 10.1029/2011JC007395
James N. P., Bone Y. (2010). Neritic carbonate sediments in a temperate realm: Southern Australia . Springer Science & Business Media. doi: 10.1007/978-90-481-9289-2
James N. P., Bone Y., Collins L. B., Kyser T. K. (2001). Surficial sediments of the Great Australian Bight: facies dynamics and oceanography on a vast cool-water carbonate shelf. J. Sediment. Res. 71 (4), 549–567. doi: 10.1306/102000710549
Jewell O. J., Gleiss A. C., Jorgensen S. J., Andrzejaczek S., Moxley J. H., Beatty S. J., et al. (2019). Cryptic habitat use of white sharks in kelp forest revealed by animal-borne video. Biol. Lett. 15 (4), 20190085. doi: 10.1098/rsbl.2019.0085
Juel A., Groom G. B., Svenning J.-C., Ejrnaes R. (2015). Spatial application of Random Forest models for fine-scale coastal vegetation classification using object based analysis of aerial orthophoto and DEM data. Int. J. Appl. Earth Obs. Geoinf. 42, 106–114. doi: 10.1016/j.jag.2015.05.008
Kämpf J., Doubell M., Griffin D., Matthews R. L., Ward T. M. (2004). Evidence of a large seasonal coastal upwelling system along the southern shelf of Australia. Geophys. Res. Lett. 31, 9. doi: 10.1029/2003GL019221
Kostylev V. E. (2012). Benthic habitat mapping from seabed acoustic surveys: do implicit assumptions hold? Sediments Morphology Sedimentary Processes Continental Shelves: Adv. Technologies Research Appl. 44, 405–416. doi: 10.1002/9781118311172.ch20
Liaw A., Wiener M. (2002). Classification and regression by randomForest. R News 2 (3), 18–22.
López-Garrido P. H., Barry J. P., González-Gordillo J. I., Escobar-Briones E. (2020). ROV’s video recordings as a tool to estimate variation in megabenthic epifauna diversity and community composition in the Guaymas Basin. Front. Mar. Sci. 7, 154. doi: 10.3389/fmars.2020.00154
MacIntosh H., Althaus F., Williams A., Tanner J. E., Alderslade P., Ahyong S. T., et al. (2018). Invertebrate diversity in the deep Great Australian Bight (200–5000 m). Mar. Biodiversity Records 11, 1–21. doi: 10.1186/s41200-018-0158-x
Mayer L., Jakobsson M., Allen G., Dorschel B., Falconer R., Ferrini V., et al. (2018). The Nippon Foundation—GEBCO seabed 2030 project: The quest to see the world’s oceans completely mapped by 2030. Geosciences 8 (2), 63. doi: 10.3390/geosciences8020063
McAfee D., Alleway H. K., Connell S. D. (2020). Environmental solutions sparked by environmental history. Conserv. Biol. 34, 386–394. doi: 10.1111/cobi.13403
McClatchie S., Middleton J. F., Ward T. M. (2006). Water mass analysis and alongshore variation in upwelling intensity in the eastern Great Australian Bight. J. Geophys. Res. Oceans 111 (C8). doi: 10.1029/2004JC002699
McConnell B., Chambers C., Fedak M. (1992). Foraging ecology of southern elephant seals in relation to the bathymetry and productivity of the Southern Ocean. Antarct. Sci. 4 (4), 393–398. doi: 10.1017/S0954102092000580
McLeay L. J., Sorokin S. J., Rogers P. J., Ward T. M. (2003). Benthic Protection Zone of the Great Australian Bight Marine Park: 1. Literature Review. Final Report to National Parks and Wildlife South Australia and the Commonwealth Department of the Environment and Heritage (South Australian Research and Development Institute (Aquatic Sciences), Adelaide).
Menandro P. S., Bastos A. C. (2020). Seabed mapping: A brief history from meaningful words. Geosciences 10 (7), 273. doi: 10.3390/geosciences10070273
Menge B. A., Caselle J. E., Milligan K., Gravem S. A., Gouhier T. C., White J. W., et al. (2019). Integrating coastal oceanic and benthic ecological approaches for understanding large-scale meta-ecosystem dynamics. Oceanography 32, 38–49. doi: 10.5670/oceanog.2019.309
Middleton J. F., Bye J. A. (2007). A review of the shelf-slope circulation along Australia’s southern shelves: Cape Leeuwin to Portland. Prog. Oceanogr. 75 (1), 1–41. doi: 10.1016/j.pocean.2007.07.001
Middleton J. F., James N. P., James C., Bone Y. (2014). Cross-shelf seawater exchange controls the distribution of temperature, salinity, and neritic carbonate sediments in the Great Australian Bight. J. Geophys. Res. Oceans 119 (4), 2539–2549. doi: 10.1002/2013JC009420
Moll R. J., Millspaugh J. J., Beringer J., Sartwell J., He Z. (2007). A new ‘view’ of ecology and conservation through animal-borne video systems. Trends Ecol. Evol. 22, 660–668. doi: 10.1016/j.tree.2007.09.007
Monk J., Ierodiaconou D., Versace V. L., Bellgrove A., Harvey E., Rattray A., et al. (2010). Habitat suitability for marine fishes using presence-only modelling and multibeam sonar. Mar. Ecol. Prog. Ser. 420, 157–174. doi: 10.3354/meps08858
O'Connell L. G., James N. P., Doubell M., Middleton J. F., Luick J., Currie D. R., et al. (2016). Oceanographic controls on shallow-water temperate carbonate sedimentation: Spencer Gulf, South Australia. Sedimentology 63 (1), 105–135. doi: 10.1111/sed.12226
Pebesma E., Graeler B. (2015). Package ‘gstat’. Comprehensive R Archive Network (CRAN), 1-0 .
Peters K. J., Ophelkeller K., Bott N. J., Deagle B. E., Jarman S. N., Goldsworthy S. D. (2015). Fine-scale diet of the Australian sea lion ( Neophoca cinerea ) using DNA-based analysis of faeces. Mar. Ecol. 36 (3), 347–367. doi: 10.1111/maec.12145
Przeslawski R., Currie D. R., Sorokin S. J., Ward T. M., Althaus F., Williams A. (2011). Utility of a spatial habitat classification system as a surrogate of marine benthic community structure for the Australian margin. ICES J. Mar. Sci. 68 (9), 1954–1962. doi: 10.1093/icesjms/fsr106
Rather T. A., Kumar S., Khan J. A. (2020). Multi-scale habitat modelling and predicting change in the distribution of tiger and leopard using random forest algorithm. Sci. Rep. 10 (1), 11473. doi: 10.1038/s41598-020-68167-z
Robusto C. C. (1957). The cosine-haversine formula. Am. Math. Monthly 64, 38–40. doi: 10.2307/2309088
Shanley C. S., Eacker D. R., Reynolds C. P., Bennetsen B. M., Gilbert S. L. (2021). Using LiDAR and Random Forest to improve deer habitat models in a managed forest landscape. Forest Ecol Manag. 499, 119580. doi: 10.1016/j.foreco.2021.119580
Shepherd S. A., Sprigg R. (1976). “Substrate, sediments and subtidal ecology of Gulf St. Vincent and Investigator Strait” in Natural History of the Adelaide Region , 161–174.
Sorokin S. J., Currie D. R. (2008). Report to Nature Foundation SA Inc, 68. The distribution and diversity of sponges in Spencer Gulf .
Sorokin S., Fromont J., Currie D. (2007). Demosponge biodiversity in the benthic protection zone of the Great Australian Bight. Trans. R. Soc. S. Aust. 131 (2), 192–204. doi: 10.1080/03721426.2007.10887083
Stewart R. R., Noyce T., Possingham H. P. (2003). Opportunity cost of ad hoc marine reserve design decisions: an example from South Australia. Mar. Ecol. Prog. Ser. 253, 25–38. doi: 10.2254/meps253025
Sumner M. (2011). trip: Tools for the Analysis of Animal Track Data . R package version 1.8.5.
Sweetman A. K., Thurber A. R., Smith C. R., Levin L. A., Mora C., Wei C.-L., et al. (2017). Major impacts of climate change on deep-sea benthic ecosystems. Elementa: Science of the Anthropocene 5, 4. doi: 10.1525/elementa.203
Tanner J. E. (2005). Three decades of habitat change in Gulf St. Vincent, South Australia. Habitat Modification and its Influence on Prawn and Crab Fisheries. Final Report to the Fisheries Research and Development Corporation (South Australian Research and Development Institute (Aquatic Sciences), Adelaide).
Thomas C., Hughes V. (2016). “South Australia’s experience: establishing a network of nineteen marine parks,” in Big, Bold and Blue: Lessons from Australia’s Marine Protected Areas , 139–152.
Torn K., Herkül K., Martin G., Oganjan K. (2017). Assessment of quality of three marine benthic habitat types in northern Baltic Sea. Ecol. Indic. 73, 772–783. doi: 10.1016/j.ecolind.2016.10.037
van Ruth P. D., Patten N. L., Doubell M. J., Chapman P., Rodriguez A. R., Middleton J. F. (2018). Seasonal-and event-scale variations in upwelling, enrichment and primary productivity in the eastern Great Australian Bight. Deep Sea Res. Part II: Topical Stud. Oceanography 157, 36–45. doi: 10.1016/j.dsr2.2018.09.008
Vigo M., Navarro J., Aguzzi J., Bahamón N., García J. A., Rotllant G., et al. (2023). ROV-based monitoring of passive ecological recovery in a deep-sea no-take fishery reserve. Sci. Total Environ. 883, 163339. doi: 10.1016/j.scitotenv.2023.163339
Ward T. M., McLeay L. J., Dimmlich W. F., Rogers P. J., McClatchie S., Matthews R., et al. (2006). Pelagic ecology of a northern boundary current system: effects of upwelling on the production and distribution of sardine ( Sardinops sagax ), anchovy ( Engraulis australis ) and southern bluefin tuna ( Thunnus maccoyii ) in the Great Australian Bight. Fish. Oceanogr. 15 (3), 191–207. doi: 10.1111/j.1365-2419.2006.00353.x
Ward T. M., Sorokin S. J., Currie D. R., Rogers P. J., McLeay L. J. (2006a). Epifaunal assemblages of the eastern Great Australian Bight: Effectiveness of a benthic protection zone in representing regional biodiversity. Cont. Shelf Res. 26 (1), 25–40. doi: 10.1016/j.csr.2005.09.006
Wong R. H., Monk J., Perkins N. R., Barrett N. S. (2023). A systematic review on the anthropogenic stressors on sessile benthic mesophotic reef communities: implications for temperate reef management in Australia. Front. Mar. Sci. 10. doi: 10.3389/fmars.2023.1276072
Yoklavich M. M., Laidig T. E., Graiff K., Clarke M. E., Whitmire C. E. (2018). Incidence of disturbance and damage to deep-sea corals and sponges in areas of high trawl bycatch near the California and Oregon border. Deep Sea Res. Part II: Top. Stud. Oceanogr. 150, 156–163. doi: 10.1016/j.dsr2.2017.08.005
Keywords: habitat mapping, benthic, pinniped, animal-borne video, southern Australia, continental shelf, mesophotic reefs, biologging
Citation: Angelakis N, Grammer GL, Connell SD, Bailleul F, Durante LM, Kirkwood R, Holman D and Goldsworthy SD (2024) Using sea lion-borne video to map diverse benthic habitats in southern Australia. Front. Mar. Sci. 11:1425554. doi: 10.3389/fmars.2024.1425554
Received: 30 April 2024; Accepted: 24 June 2024; Published: 07 August 2024.
Reviewed by:
Copyright © 2024 Angelakis, Grammer, Connell, Bailleul, Durante, Kirkwood, Holman and Goldsworthy. This is an open-access article distributed under the terms of the Creative Commons Attribution License (CC BY) . The use, distribution or reproduction in other forums is permitted, provided the original author(s) and the copyright owner(s) are credited and that the original publication in this journal is cited, in accordance with accepted academic practice. No use, distribution or reproduction is permitted which does not comply with these terms.
*Correspondence: Nathan Angelakis, [email protected]
Disclaimer: All claims expressed in this article are solely those of the authors and do not necessarily represent those of their affiliated organizations, or those of the publisher, the editors and the reviewers. Any product that may be evaluated in this article or claim that may be made by its manufacturer is not guaranteed or endorsed by the publisher.
Thank you for visiting nature.com. You are using a browser version with limited support for CSS. To obtain the best experience, we recommend you use a more up to date browser (or turn off compatibility mode in Internet Explorer). In the meantime, to ensure continued support, we are displaying the site without styles and JavaScript.
- View all journals
- Explore content
- About the journal
- Publish with us
- Sign up for alerts
- Open access
- Published: 12 August 2024
A new dataset for video-based cow behavior recognition
- Daoerji Fan 1 ,
- Huijuan Wu 1 &
- Aruna Zhao 1
Scientific Reports volume 14 , Article number: 18702 ( 2024 ) Cite this article
Metrics details
- Electrical and electronic engineering
- Information theory and computation
A new video based multi behavior dataset for cows, CBVD-5, is introduced in this paper. The dataset includes five cow behaviors: standing, lying down, foraging,rumination and drinking. The dataset comprises 107 cows from the entire barn, maintaining an 80% stocking density. Monitoring occurred over 96 h for these 20-month-old cows, considering varying light conditions and nighttime data to ensure standardization and inclusivity.The dataset consists of ranch monitoring footage collected by seven cameras, including 687 video segment samples and 206,100 image samples, covering five daily behaviors of cows. The data collection process entailed the deployment of cameras, hard drives, software, and servers for storage. Data annotation was conducted using the VIA web tool, leveraging the video expertise of pertinent professionals. The annotation coordinates and category labels of each individual cow in the image, as well as the generated configuration file, are also saved in the dataset. With this dataset,we propose a slowfast cow multi behavior recognition model based on video sequences as the baseline evaluation model. The experimental results show that the model can effectively learn corresponding category labels from the behavior type data of the dataset, with an error rate of 21.28% on the test set. In addition to cow behavior recognition, the dataset can also be used for cow target detection, and so on.The CBVD-5 dataset significantly influences dairy cow behavior recognition, advancing research, enriching data resources, standardizing datasets, enhancing dairy cow health and welfare monitoring, and fostering agricultural intelligence development. Additionally, it serves educational and training needs, supporting research and practical applications in related fields. The dataset will be made freely available to researchers world-wide.
Introduction
Behavior recognition, harnessing the prowess of computer vision and related technologies, extracts and interprets behavioral cues from video data, playing a pivotal role in applications such as anomaly alerts, agricultural breeding, and animal behavior analytics. In the realm of dairy farming, automating the identification of cow behaviors within expansive pastures promises to augment bovine health, optimize resource allocation, and bolster the overall productivity of the livestock sector.
In the Inner Mongolia Autonomous Region, blessed with expansive grasslands and a favorable climate, dairy cow breeding plays a pivotal role, with the region serving as a major hub in China. According to the Regional Bureau of Statistics, by 2023, the dairy cow population escalated to 1.687 million, witnessing a 6.1% growth, accompanied by a milk production increase to 7.926 million tons, up 8.0%. Furthermore, large-scale dairy output surged to 4.73 million tons, representing a significant 13.2% annual increase 1 .
While the thriving dairy industry bolsters rural livelihoods and contributes to the transformation of agriculture, it grapples with challenges such as enhancing feed efficiency, managing diseases effectively, and advancing sustainability practices 2 . Amid these pressing issues, the importance of understanding cow behavior escalates, underscoring the necessity for comprehensive datasets to facilitate the development of efficient recognition models that can address these complexities.
A comprehensive dataset on cow behavior would enhance model effectiveness, improve farm management, boost productivity, and support sustainable livestock practices. By standardizing data collection, researchers can gather diverse, large-scale information covering feeding, resting, movement, and social behaviors.
To tackle the dearth of a high-quality dataset, a streamlined system integrating camera surveillance, data storage, custom software, and server infrastructure was deployed, leading to the development of a robust dataset. With expert dairy farmers’ insights, meticulous annotation refined the dataset, eliminating abnormalities to ensure purity and relevance for superior model training and livestock management enhancements. Establishing such a practical, all-encompassing database, rooted in real-ranch scenarios, is vital for progressing cow welfare, optimizing resource allocation, boosting dairy productivity, and fostering a global understanding of cow behavior among researchers.
Therefore, this study introduces a comprehensive cows behavior dataset named “CBVD-5”. This dataset is intended to serve as a benchmark for cow behavior recognition.The CBVD-5 dataset incorporates five atomic behavioral categories: standing and lying as posture-related behaviors, with foraging, rumination, and drinking classified as state behaviors. This taxonomy captures the intricate complexity of cow behavior, integrating both postural and state elements to accurately depict daily activities. Rigorously validated by empirical evidence gathered through close collaboration with dairy farmers, our classification schema ensures alignment with genuine farming scenarios, thereby enhancing its practical applicability in the field..
Categories were meticulously selected via dialogues with pasture proprietors and surveys of farmers to accurately reflect cows’ activity, rest, hydration, and feeding habits, collectively presenting a holistic view of their living standards and health. The dataset will be freely disseminated to the research community, facilitating advancements in cow behavior studies such as multi-animal detection, individual tracking, and multiplex behavior recognition, thereby stimulating innovations in this domain. The main contributions of this paper thus can be summarized as follows:
A benchmark dataset, CBVD-5, is introduced in this paper for identifying cow behavior under standardized ranches. The dataset was collected using seven cameras, comprising 687 video segment samples and 206,100 image samples.The monitoring was conducted for 96 h on 20-month-old cows, taking into account different light conditions and nighttime data to ensure standardization and inclusiveness.
An integral component of our work entails the development of specialized code tools and innovative device design strategies. These methodologies are pivotal for the streamlined collection, preprocessing, and meticulous cleansing of the cow behavior video dataset, thereby enhancing data quality, reducing noise, and optimizing the dataset’s utility for advanced research purposes.
Lastly, we present the modification and adaptation of a benchmark recognition model leveraging the SlowFast architecture. Tailored specifically for recognizing and analyzing cow behaviors in standardized ranch settings, this adjusted model harnesses the temporal and spatial prowess of SlowFast to deliver enhanced accuracy in behavior recognition, thereby advancing the precision and applicability of livestock behavior analytics.
The remainder of the paper is organized as follows. “ Related work ” presents a literature review of Cows Behavior Videos Dataset. In “ Overview of CBVD-5 ”, we present the data collection steps used in this study and the dataset statistics. “ Benchmark evaluation ” details the data preprocessing and framing process. Also it presents the adapted model and the experiment results of the cow behavior recognition algorithm using the CBVD-5 dataset. Lastly, we present the conclusions of this study in “ Conclusion ”. Finally, in “ Prospects ”, we also provide an outlook on and discuss extensions to our future research endeavors.
Related work
Recent advancements in cow behavior recognition research can be broadly categorized into sensor-based methodologies and computer vision-based approaches. Sensor-based studies have utilized GPS positioning sensors for non/-intrusive monitoring of cow activities, achieving high classification accuracies through preprocessing and machine learning techniques 3 . Expanding upon this, Riaboff et al. combined accelerometer and GPS data to explore pasture behavior insights in dairy cows 4 . Sensor research has also ventured into multi-sensor and wearable devices, with Tian et al. achieving real-time recognition through geomagnetic and acceleration fusion models 5 , and Lovarelli et al. developing a sophisticated wearable sensor node for cow behavior classification 6 .
On the computational front, Li et al. integrated multiple strategies for comprehensive dairy cow behavior analysis 7 , while Guo et al. detected mounting behaviors via video analysis 8 , and Girish et al. focused on static image action recognition 9 . Avola et al. advanced 2D skeleton-based recognition with LSTM-RNNs 10 , showcasing the versatility and adaptability of computer vision for real-time monitoring and detailed behavioral analysis in various settings.
Sensor and vision-based studies have also seen applications in individual animal recognition, as demonstrated by Bhole et al. 11 , and health monitoring, such as lameness detection by Jiang et al. 12 , and respiratory behavior monitoring by Wu et al. 13 .Fuentes et al. developed a deep learning approach for recognizing hierarchical cattle behavior using spatio-temporal information 14 . Bai et al. introduced X3DFast, a 3D convolution-based model for efficient behavior classification 15 .
Despite these progresses, the availability of public datasets remains limited. Notable exceptions include COW-VID 16 for calving prediction, COW-IMU 17 for IMU data classification, and COW-Act 18 for barn behavior tracking.
To address the scarcity of comprehensive datasets, we proudly introduce CBVD-5, featuring meticulous recordings of atomic behaviors: standing, lying, grazing, rumination, and drinking, all within a meticulously managed pasture environment. Rigorously validated, this standardized video dataset establishes a solid foundation for advancing our insights into cow behavior and improving livestock management practices.
Overview of CBVD-5
Cow behavior selection.
The dataset utilized in this study serves as a foundational resource for research and experimentation with deep learning algorithms. Over the years, researchers have proposed various human behavior datasets such as HMDB-51, UCF-101 19 , and Kinetics-400 20 , leading to the development of numerous novel behavior recognition algorithms.
However, there is currently no publicly available video behavior dataset specifically tailored to cows under standardized ranch conditions. Therefore, the primary objective of this study is to construct a comprehensive cow behavior dataset. To determine the specific behaviors to include, extensive investigations, evidence collection, and consultations with ranch owners were conducted. As a result, five key behaviors were identified: standing, lying down, foraging, drinking water, and rumination.
In our research, we adopt a systematic classification approach, defining the five fundamental behaviors as elemental behaviors: standing and lying down are labeled as “postural behaviors,” representing the basic stances cows adopt. Meanwhile, the actions of foraging, drinking, and rumination fall under the category of “state behaviors,” elucidating the active processes or physiological conditions they undergo at any given time. This framework serves to decode more complex behavioral patterns by focusing on their essential components.
Observing a cow’s standing behavior helps understand its activity patterns and vitality; metrics such as duration and recurrence frequency indicate comfort and exercise adequacy. Lying down, a pivotal rest period, rejuvenates energy, with close monitoring of rest intervals contributing to wellness assessments. Analyzing foraging behavior, central to nutrition and health, requires careful examination for assessing dietary adoption efficiency and digestive health, achieved by observing the frequency, duration, and intervals of foraging activities. Similarly, scrutinizing drinking patterns offers insights into hydration levels and water preferences, critical for overall health maintenance. Furthermore, evaluating rumination, a foundation of digestive health, through assessing its frequency, duration, and patterns, highlights the suitability of feeding conditions, including aspects like forage quality, timing, and frequency. Collectively, meticulously tracking these behaviors provides a thorough understanding of the cows’ health, welfare, and productivity.
Table 1 provides a video-level description and understanding of the five behaviors exhibited by cows. These behaviors were carefully chosen to be comprehensive and representative. Studying and identifying these behaviors can optimize feeding management strategies, enable timely measures to reduce disease occurrence and spread, and facilitate prompt adjustments to feeding management practices, ensuring the health and productivity of cows. Furthermore, this dataset enables in-depth research on behavior patterns, individual differences, and group dynamics of cows. It aids in monitoring their activity levels, rest conditions, dietary intake, and digestive functions, thereby facilitating the timely identification of health issues, optimization of feeding management, and improvement of production performance and welfare levels. Ultimately, this dataset supports decision-making processes for relevant personnel, leading to enhanced cow health and production efficiency.
Data collection
Due to the increasing demand for surveillance and security, we have made the decision to utilize surveillance cameras for the purpose of collecting videos capturing cow behavior. By installing cameras on ranches, we can facilitate real-time monitoring and effective livestock management. The experimental equipment includes 2.8 mm and 3.6 mm Dahua cameras (M/K models), Dahua DH-S3000C-16GT 16-port gigabit network switches, and Dahua DH-NVR2216-HDS3 network hard disk recorders. For broader coverage areas, we have selected 2.8 mm lenses, while for monitoring distant targets, we have opted for 3.6 mm lenses. The layout plan for camera installation on the ranch, as well as the data storage and acquisition plan, have been designed and finalized. The overall system architecture is illustrated in Fig. 1 .
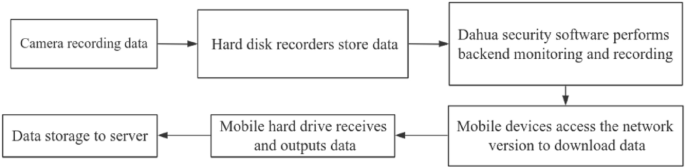
Architecture diagram of camera data storage and acquisition system.
The monitoring system’s installation and data acquisition commence with demarcating the surveillance zone, comprising feeding, watering, and other key areas. Fixed infrared cameras are strategically selected to enable uninterrupted 24/7 video monitoring and recording. Positioned approximately every 10 m with a 17 \(\circ \) downward tilt, these cameras, mounted securely on walls to avoid obstructions, ensure comprehensive coverage of the targeted zones.
To connect the cameras to the network, a wired connection is selected, utilizing Power over Ethernet (PoE) for power supply. A hard disk recorder is chosen as the storage device for storing the surveillance footage. The cameras are connected to the existing distribution box in the ranch to ensure a stable power supply. Additionally, auxiliary facilities such as distribution boxes, clamps, network cables, and network switches are installed as necessary to enhance monitoring effectiveness and security.
Video data from surveillance is integrated into the Dahua Security Software (depicted in Fig. 2 ), enabling remote access via its web interface on mobile devices. Subsequently, data is extracted onto a portable hard drive and relayed to the central server, thus concluding the data gathering phase. Fundamentally, the system is tasked with dual operations: continuous data acquisition and routine monitoring.
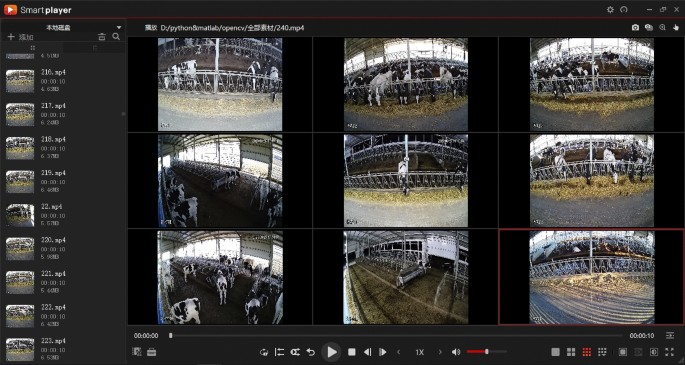
Main page of Dahua player.
Data annotations
After collecting the video data, to reduce the workload of manual annotation, the decision was made to extract frames and select keyframes for manual annotation. The annotation tool chosen was via 3.0.11, and the annotation format followed that of the ava dataset. A total of 206,100 image data were generated from the frame extraction process, out of which 4122 keyframes were selected for annotation. The annotations included checkboxes for selecting targets and category labels. To minimize labeling errors, the images were annotated in batches by our researchers, and the labeled data was later reviewed and improved by experienced personnel. As a result, we obtained a total of 27,501 valid labeled data.
Dataset statistics
Now, the CBVD-5 dataset is publicly available at https://www.kaggle.com/datasets/fandaoerji/cbvd-5cow-behavior-video-dataset to all researchers.
The dataset encompasses labeled data, keyframe images, and raw video footage procured via camera installations, as illustrated in Fig. 3 . These keyframe images depict two categories under the umbrella of “postural behaviors”: standing and lying down. Further, Fig. 4 exhibits keyframe illustrations for three classifications categorized as “state behaviors” within the dataset: foraging, rumination, and drinking.The CBVD-5 dataset contains a total of 206,100 image samples, and no separation of training and test sets. Users can divide the test set and training set according to their own needs.
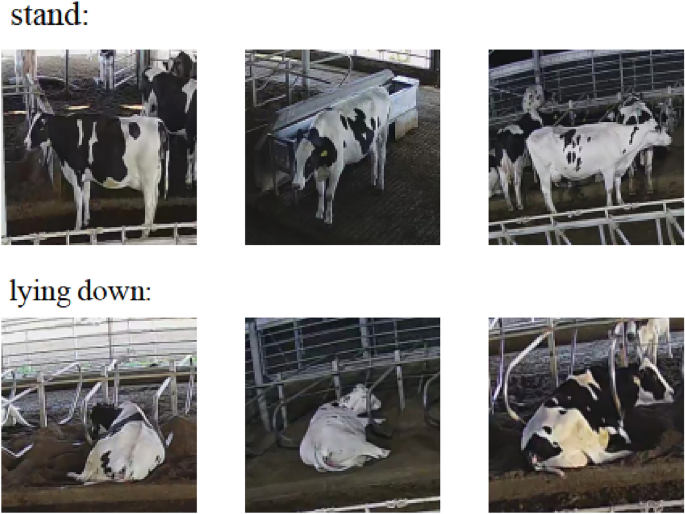
Two postural behavior samples in CBVD-5.
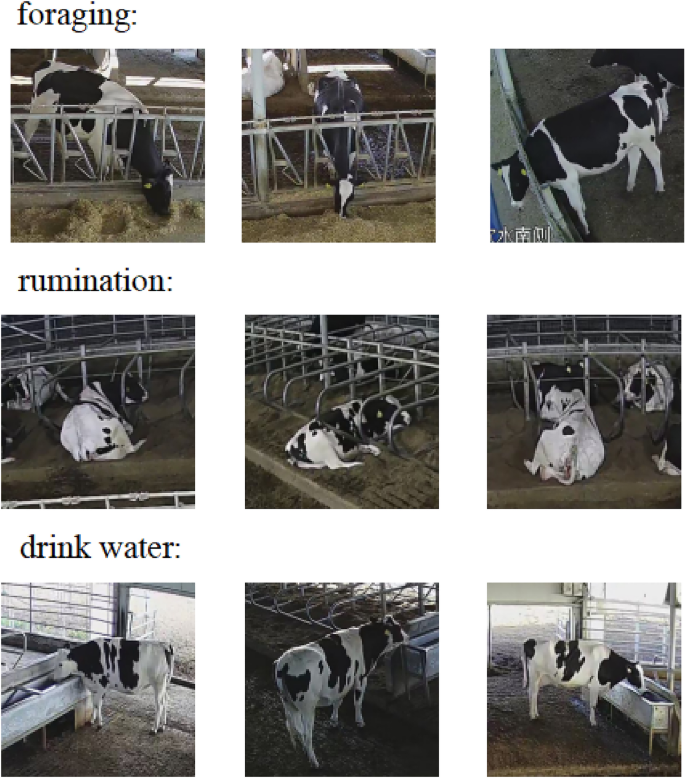
Three state behavioral samples in CBVD-5.
We performed a discrete statistical analysis on the count of all “atomic behavior” categories within the dataset. As illustrated in Fig. 5 , the diagram furnishes an overview of the compositional proportions of both “postural behaviors” and “state behaviors,” offering insights into their respective representations within the dataset.
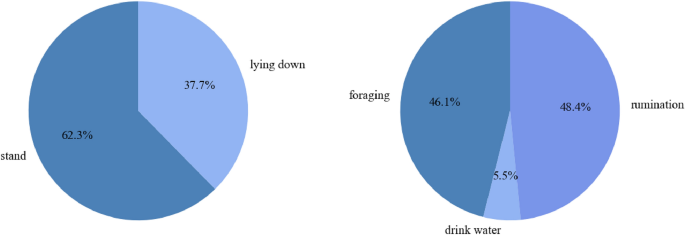
Proportional composition of five categorized behavioral samples.
The dataset comprises 206,100 image samples, and a detailed presentation of the distribution of these samples based on category labels from the annotation files is depicted in Fig. 6 ,which shows the number of occurrences of each atomic behavior in the sample files.
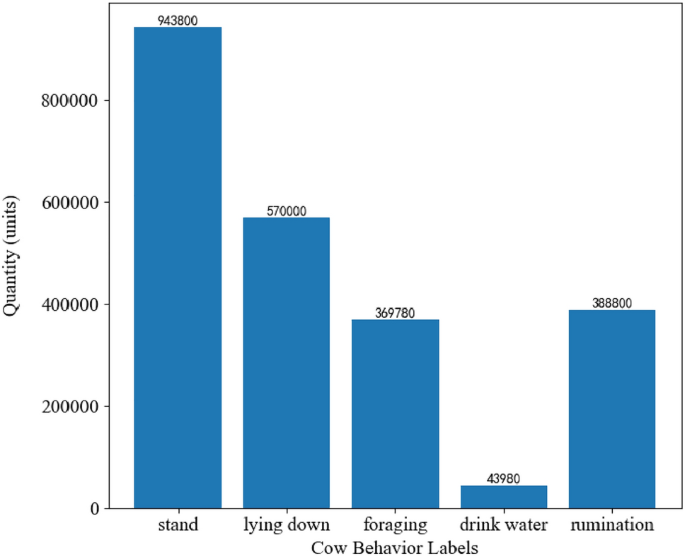
Sample count statistics for five classes of atomic behaviors.
It is noteworthy that the CBVD-5 dataset contains a significant number of keyframe image samples labeled as “lying down,” indicating that lying down is a common atomic behavior among cows in the dataset.Furthermore, the dataset encompasses diverse combinations of both postural and state behaviors within the realm of atomic actions, including illustrative pairings such as standing coupled with drinking and lying down accompanied by rumination, among others. This comprehensive inclusion fosters a deeper understanding of the intricate interplay between these fundamental behaviors in cows.
In addition, our research team has validated and analyzed the behavioral patterns and regularities of cows in the pasture where the data set was collected over a 24-hour period. We have represented these patterns using a bar chart, as shown in Fig. 7 . By comparing and analyzing the composition of our CVBD-5 dataset, we have found that the proportions of different behavioral categories in the CBVD-5 dataset are scientifically grounded.
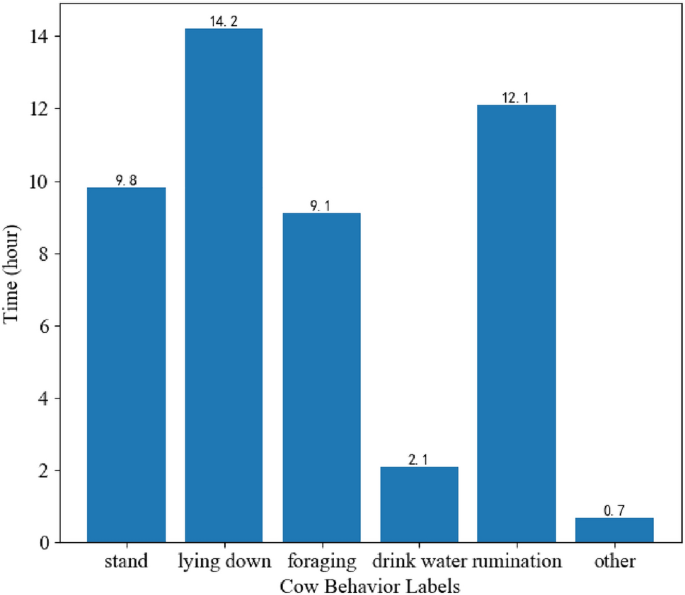
Statistical chart of daily cow behaviors.
The dataset consists of five folders, namely “videos”, “video_cut”, and “rawframes”,“Labelframes”, “annotations”. Among them, videos represent the original video, and video_cut is the cropped 10s video segment sample.Rawframes are images generated by video sample frame segmentation for training, while labelframes are generated images for keyframe extraction for annotation.Annotations contains 8 files, which are all annotation files generated by annotations. Among them, there are 5 types of atomic behaviors in “ava-action_listv2.1.pbtxt.txt”, among which the most important is the. csv file for annotating the data. Taking “avatrain_v2.2. csv” as an example, it is the training dataset file, where the key information includes 5 parts: [Video_id, middle_Frame_timestamp, Object_detection_box, Action_ID, Target_ID]
Video_ID: Video Name
Middle Frame Timestamp: The position of the keyframe (in seconds)
Object_detection_box: This includes four columns, (x1, y1, x2, y2), which represent the positions of the upper left and lower right points after normalization of the original labeled data.
Action_Id: ava Action List The corresponding IDs in v2.1. pbtxt are for five category labels.
Target_ID: Animal category: cow, so its default value is 1. Each row is labeled with only one box, one task, and corresponding multiple category labels.
“ava_train_excluded_timesampsv2.1.csv” is the frame in which the problem occurred in the evaluated dataset, used for validation “ava_dense_Proposals_train.FAIR.recall_93.9.pkl” and “ava_dense_Proposals_val.FAIR recall_93.9.pkl” are used to cache data and reduce the computation time of Python programs for subsequent processing.
Benchmark evaluation
Cow behavior recognition can be seen as a process combining object detection and behavior classification. As a pilot recognition task, we chose to use the SlowFast model from the mmaction2 toolkit to complete this task.
Before selecting the SlowFast model, we thoroughly investigated the shortcomings of other behavior recognition models in handling datasets like AVA. While 2D CNNs excel at extracting spatial features from video frames, they lack the ability to capture temporal information, limiting their efficacy in dynamic behavior analysis 21 . 3D CNNs directly process spatiotemporal information from video sequences, but their high computational cost and training difficulties hinder their efficiency, particularly when dealing with lengthy video sequences 22 .Although C3D models have achieved some success, their performance diminishes when confronted with long sequence videos, struggling to effectively capture intricate details and long-term dependencies 23 .
The SlowFast model analyzes a sequence of images based on the preceding and succeeding frames to determine the performed action. For behavior recognition, where actions vary while the environment remains constant, the idea behind the SlowFast model is to separately extract action information and environmental information, and then fuse them together to accomplish behavior recognition.
Data of video preprocessing
After completing the data collection, the video data needs to undergo preprocessing.Referring to the impressive performance of SlowFast on AVA data, we aim to apply transfer learning to cows and construct a dataset similar to AVA data types.
Firstly, we need to preprocess the video data. The original surveillance videos are encoded in H.265/HEVC format. To facilitate subsequent processing, we will utilize the Moviepy library to convert them to H.264/AVC encoding.Next, we will select data segments from the videos. The original surveillance videos generate a recording every hour, resulting in a total of 700 videos from all cameras with a combined duration of 42,000 min. To ensure randomness, we will create the dataset by randomly selecting a starting time point within a long video segment. We will then capture a fixed duration of 10 s of video segments. We will exclude the segments before IP allocation, resulting in a remaining count of 687 video segments.Finally, since the original SlowFast model requires video processing with 30 frames and a frame rate of 25 frames per second, we will utilize the ffmpeg tool to increase the frame rate of the video segments. This step ensures that the video segments meet the requirements for subsequent processing and generation tasks.
Preprocessing and framing:
Convert the original surveillance videos from H.265/HEVC format to H.264/AVC encoding for subsequent processing.
Randomly select a starting time point to create a dataset from long video segments.
Capture fixed-duration video segments of 10 seconds.
Exclude segments before IP allocation to ensure dataset quality and accuracy.
Use the ffmpeg tool to increase the frame rate of video segments to meet the requirements of subsequent processing and generation tasks.
To comply with the requirements of the SlowFast model, ensure that the frame rate of the video segments is increased to 30 frames per second using video processing techniques.
These steps lay the foundation for subsequent transfer learning and building a cow dataset similar to the AVA data type.
Details of the proposed model
The SlowFast model takes a video as input and undergoes feature extraction through the Slow Branch and Fast Branch. The Slow Branch processes the temporal information of the video by capturing long-term dynamics with a lower frame rate. The Fast Branch handles the spatial information of the video by capturing short-term dynamics with a higher frame rate 24 . The features extracted from the two branches represent temporal and spatial features, respectively.Next, the Slow Feature Fusion and Fast Feature Fusion modules merge the temporal and spatial features to integrate the two types of information. This fusion process can be achieved in various ways, such as merging feature maps or feature vectors.Finally, the fused features are fed into a classifier or regressor for the final classification or regression prediction. The output is the predicted result for the input video.As shown in the Fig. 8 , this is the network architecture of SlowFast. The design of the SlowFast model enables it to simultaneously process the temporal and spatial information of videos, leading to a better understanding of video content and dynamic changes. It has achieved excellent performance in tasks such as video classification and action recognition.
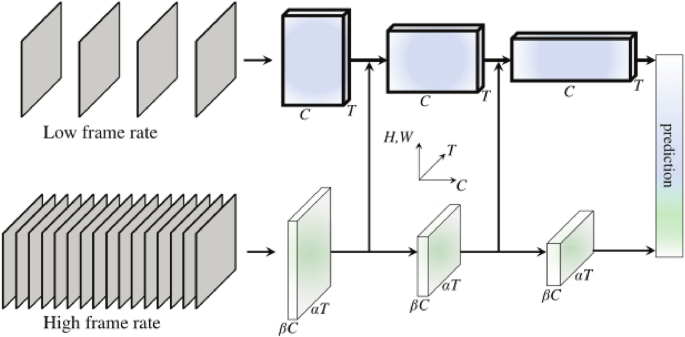
A SlowFast network.
In Table 2 is a detailed design of SlowFast based on ResNet50 as the backbone network. It can be observed that the frame rate of Fast is 8 times that of Slow (lightgreen), while the channel number of Slow is 8 times that of Fast (orange). Fast always maintains a relatively low channel capacity for lightweight consideration (faster), and Fast focuses more on temporal information, so it can ignore some spatial information.
SlowFast Networks use the Slow pathway to extract spatial semantic features (such as color, size, shape, etc.) from sparse RGB frames, and the Fast pathway to capture motion information (extracting temporal features) while reducing the number of channels to make it lightweight. The direct connection from Fast to Slow can fuse finer-grained spatiotemporal information at an early stage.
In the SlowFast model, the steps for object detection and recognition using Faster R-CNN and ResNet-50 are as follows:
Data preprocessing: resize, crop, and normalize images, and convert them into the required tensor format.
Feature extraction: use ResNet-50 to extract high-level features from the images.
Region proposal network (RPN): generate candidate object regions on the feature map and filter high-probability candidates.
Region of interest pooling (RoIPool): map selected candidate bounding boxes to a fixed-size feature map.
Object classification and bounding box regression: classify objects and refine their bounding box positions.
Non-maximum suppression (NMS): filter out duplicate detections based on confidence scores and overlapping areas.
Object recognition: optionally perform further recognition or attribute classification on the detected objects.
Our subsequent experiments on data set evaluation are conducted based on this network structure and model, with further adjustments of hyperparameters to complete the subsequent experiments.
Experiment results
From preprocessed CBVD-5 dataset, we randomly selected 70% as the training set, 20% as the test set and the remaining 10% as the validation set. The cross-entropy loss function is used during training, and the training is stopped when the loss on the validation set is no longer reduced, and the epoch with the smallest loss on the validation set is selected as the optimal model. Each training epoch took nearly 160 s on the GPU NVIDIA Quadro P5000.
The SlowFast model has demonstrated impressive accuracy in the field of behavior recognition. For cow behavior recognition, we placed greater emphasis on adjusting hyperparameters such as the initial factor, adjustment factor, and learning rate in the training configuration file. The configuration file utilizes the parameter scheduler “param_scheduler” and the optimizer wrapper “optim_wrapper”. Initially, we employed a “LinearLR” type parameter scheduler to linearly adjust the learning rate based on the initial factor. Next, we used a “MultiStepLR” type parameter scheduler to adjust the learning rate based on the adjustment factor between the total training epochs according to the specified interval. Finally, we used an optimizer of type “SGD” to determine the learning rate, momentum, and weight decay. Additionally, we set the configuration for gradient clipping to ensure more stable model convergence during training.
During adjustment, a ResNet3D network with a depth of 5 and lateral connections was used for the slow pathway. The kernel size of the first convolutional layer was (1, 7, 7), and the spatial stride was (1, 1, 1, 1). The first pooling layer had a stride of 1 and a spatial stride of (1, 2, 2, 1). The fast pathway also used a ResNet3D network with a depth of 50, without lateral connections, and 8 channels. The kernel size of the first convolutional layer was (5, 7, 7), and the first pooling layer had a stride of 1 and a spatial stride of (1, 2, 2, 1).
After conducting our experiments, we found that the recognition accuracy for standing, lying, and feeding behaviors was relatively high, while rumination behavior was more difficult to recognize at the video level. Therefore, we selected the accuracy of rumination behavior as the evaluation metric. We chose mAP (mean Average Precision) and rAP (rumination Average Precision) as the evaluation metrics for the five types of behaviors, where rAP refers to the accuracy of rumination behavior.
The mean Average Precision (mAP) comprehensively evaluates a model’s accuracy and recall across different categories by computing the average precision, providing a comprehensive assessment of the model’s recognition accuracy across diverse behavior categories in multi-class behavior recognition tasks.
Moreover, the use of the rumination Average Precision (rAP) as an evaluation metric allows for a more focused assessment of the model’s performance specifically on rumination behavior recognition, which is crucial for reflecting the health of cows. The rumination Average Precision facilitates a nuanced understanding of the model’s accuracy in this specific behavior and can be utilized to compare the relative performance of different model structures or algorithms in rumination behavior recognition.
We conducted experiments to determine the optimal initial factor(start_factor), using only the initial factor as the independent variable. The results, shown in Table 3 ,indicate that the model performs best on the test set when the initial factor is set to 0.2. Therefore, all subsequent experiments were conducted with an initial factor of 0.2.
The second hyperparameter to be tuned is the adjustment factor gamma. The adjustment factor gamma is used to intervene in the specified training interval based on the adjustment factor. When tuning, other hyperparameters are selected empirically, and gamma is selected around 0.2. The experimental results are shown in Table 4 , which demonstrate that the best performance is achieved when the adjustment factor gamma is set to 0.1.
Next, we fine-tuned the learning rate and compared the results when it was set to 0.1, 0.2, 0.5, and 0.8. The results, shown in Table 5 , indicate that the model achieved the highest accuracy in behavior recognition when the learning rate was set to 0.5. Furthermore, we conducted an extensive comparison of the impact of using ResNet50 and ResNet18 in the slow and fast pathways on the accuracy of behavior recognition. We found that the impact on accuracy was negligible.
Ultimately, by fine-tuning the SlowFast network structure with the appropriate hyperparameters, we achieved the best performance.
So far, we have determined the optimal values of three hyperparameters in the model training process: initial factor, adjustment factor, and learning rate, which are 0.2, 0.1, and 0.5, respectively. In addition, we also attempted to replace the model with ResNet18 and ResNet50, but found that they did not significantly affect the accuracy of behavior recognition, although they did increase training time. Currently, the accuracy of each behavior on the training and testing sets is shown in Table 6 . The final accuracy achieved for standing, lying down, and eating was good, while accuracy for drinking and rumination was relatively low, indicating that further research is needed.
To yield these experimental results and analyze their implications, it’s evident that the model’s training process is skewed towards behaviors with a higher volume due to data imbalance, resulting in subpar recognition performance for less frequent behaviors. Furthermore, the similarity between behaviors confounds the model, leading to a decrease in recognition accuracy.
To enhance the model’s efficacy, future endeavors could entail data augmentation techniques, such as rotation, scaling, and cropping, to diversify the dataset, aiding the model in discerning between various behaviors. Addressing the data imbalance issue could involve employing resampling techniques to balance sample quantities or utilizing a weighted loss function to prioritize behaviors with fewer instances, thereby increasing the model’s focus on these behaviors. Additionally, enriching feature representations could assist the model in distinguishing between behaviors more effectively. Exploring more intricate model architectures or optimization algorithms, such as deep neural networks, transfer learning, or ensemble learning, could also be pursued to bolster the model’s performance and generalizability.
Furthermore, we ventured to utilize object detection models in an innovative manner for assessing behavior recognition capabilities. These models were challenged with complex scenarios, encompassing bounding boxes that featured an aggregation of several elementary behaviors, including both posture-related and state behaviors. The resultant findings from these assessments are visually presented in Fig. 9 , showcasing the efficacy of our approach.
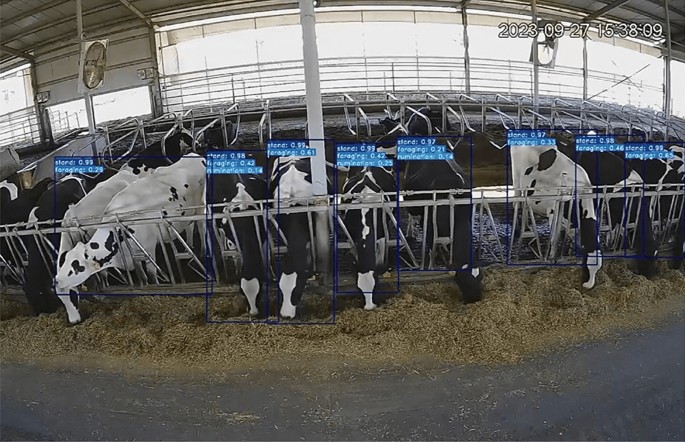
Composite atomic behavior detection results.
Error analysis
For the experimental results of the our baseline model, we give the error analysis on the test set. And the error types are divided into three categories:
Missed detection behavior: this error typically occurs when rumination behavior and lying down behavior occur simultaneously, and the model fails to capture the subtle variations in rumination behavior during video-level processing.This type of error typically arises from factors like multiple overlapping actions, brief action durations, or actions resembling the background in the video. In experimental results, this error is evident when the model fails to correctly label any actions in certain video frames.
Missed bounding box behavior: this error occurs when there is a change in the small target box or keyframe annotation box, and the model fails to capture the target due to the small size of the annotation box or the fast movement of the target in the video.This error stems from factors like changes in target size, fast motion, or target occlusion. The model struggles to promptly capture the target’s position and bounding box, leading to inaccuracies in labeling them. In experiments, this error is seen when the model correctly identifies actions in the video but fails to accurately label the target’s position and bounding box.
False bounding box behavior: this error occurs when the target is similar to the surrounding environment, and the model mistakenly identifies objects in the surrounding environment as the target.This error arises due to factors such as target-background similarity, target complexity, or changes in target posture. The model may misidentify objects in the background as the target, resulting in inaccurate bounding box annotations. In experimental findings, this error manifests when the model annotates the target in the video but with imprecise bounding box positions or misidentifies the target.
Taking into account these three types of errors, as depicted in Fig. 10 , the cow in the top left corner exhibits an error characterized by partial loss of the target label category. The cow in the bottom left corner, on the other hand, demonstrates an error involving the complete loss of the target label category. Lastly, the cow in the top right corner is associated with an error related to the loss of the target detection box.
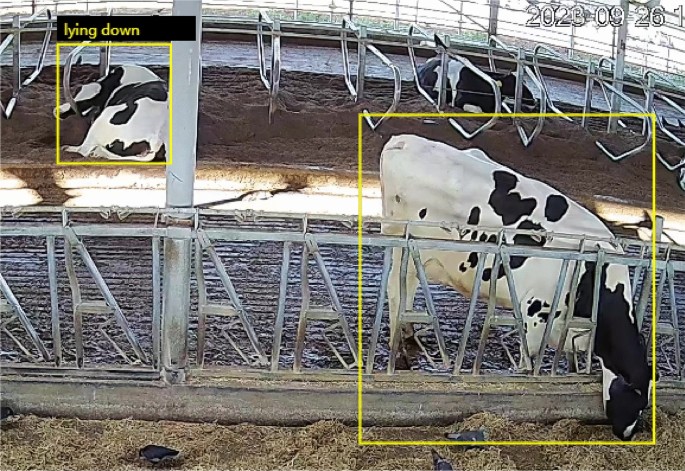
Partial error sample examples.
When dealing with limited data, one of the primary factors impacting the accuracy of water drinking behavior recognition is the model’s inadequate generalization ability. This limitation results in the model’s real-world performance falling short of expectations, as it struggles to accurately adapt to new scenarios. Another potential issue lies in sample imbalance. In the context of water drinking behavior, certain types of such behavior may occur infrequently, leading to an unequal distribution of samples across different categories in the training data, consequently affecting recognition accuracy.
To address the challenges inherent in recognizing the “drinking” state behavior, future research endeavors are underway to augment the dataset via supplementary sample collection and employment of data augmentation techniques. Specifically, in the fixed drinking zones within pastures, we are reinstalling and readjusting camera strategies to isolate and meticulously capture drinking behaviors. This dedicated effort ensures real-time updates on our Kaggle platform, facilitating vibrant discussions among researchers and thereby promoting a more holistic understanding and learning experience for all stakeholders involved.
Overall, this dataset holds significant value as it ensures that the five behavior classes exhibit variations within reasonable ranges while fine-tuning the model’s hyperparameters. Furthermore, we are committed to continuously updating and optimizing our dataset to support advancements in various related research endeavors.
In this manuscript, we present the pioneering CBVD-5 dataset, which consolidates a rich repository of five unique cow behavior categories. Comprising 687 meticulously recorded video segments and 206,100 images sourced from a strategic layout of seven cameras across a standardized pasture, this dataset represents a substantial contribution to the field.
To rigorously validate the CBVD-5 dataset’s efficacy, we leveraged transfer learning techniques with the cutting-edge SlowFast model, mirroring the structuring of the esteemed AVA dataset for enhanced cow behavior recognition. Our rigorous experimentation following the dataset’s fine-tuning for this purpose revealed that the model excels not only in accurately discerning various cow behaviors, but also demonstrates sensitivity in detecting minute targets and subtle motion nuances.
We contend that the CBVD-5 dataset constitutes a pivotal benchmark for studies centered around cow behavior recognition, significantly contributing also to advancements in object detection and tracking. This dataset, reflecting meticulous curation and scientific rigor, is made freely accessible to eager scholars upon request, fostering a collaborative ecosystem in advancing our comprehension and management of livestock behaviors for improved welfare and optimized agricultural output.
By providing this invaluable resource, we aim to invigorate global research initiatives, enabling scientists to delve deeper into the intricacies of animal behavior, thereby catalyzing innovations in farming practices and animal welfare science.
Throughout our investigative journey and experimental expansions, we have successfully implemented the YOLO+DeepSort framework for preliminary object detection and real-time tracking of cows. Our upcoming research trajectory aims to refine this focus from an aggregate herd perspective to a more granular, individual-centric approach.
The future plan entails leveraging the YOLO+DeepSort system to assign a unique identifier (ID) to each cow, enabling tailored behavioral recognition centered around these IDs. This individualized strategy is expected to yield more intricate understandings, thereby playing a pivotal role in enhancing the management and health of each dairy cow specifically.
Integrating such an advanced tracking mechanism with intricate behavioral recognition has vast implications for transforming livestock management, facilitating early health issue detection, optimizing feeding schedules, and ultimately improving herd welfare. This underscores the importance of precision livestock farming amidst technological progress. Our ongoing exploration in this vein is poised to set new benchmarks in personalized animal care and significantly contribute to sustainable agricultural practices.
Ethics statement
The data were gathered from a single location. The staff at that location provided their consent for the use of the data and images in our analysis, dataset, and online publication. This study was assessed by the Laboratory Animal Welfare and Ethics Committee of the Laboratory Animal Center, Inner Mongolia University, and it was confirmed that the study complies with animal protection, animal welfare, and ethical principles, in accordance with national regulations on laboratory animal welfare and ethics, allowing the experiment to proceed.
Data availability
All relevant codes included in this study are available upon request by contacting with the corresponding author.And the CBVD-5 dataset is now publicly available at https://www.kaggle.com/datasets/fandaoerji/cbvd-5cow-behavior-video-dataset .
Weinstein, B. G. A computer vision for animal ecology. J. Anim. Ecol. 87 (3), 533–545 (2018).
Article PubMed Google Scholar
Yan, B., Li, Y., Qin, Y., Yan, J. & Shi, W. Spatial-temporal analysis of the comparative advantages of dairy farming: Taking 18 provinces or municipalities in china as an example. Comput. Electron. Agric. 180 , 105846 (2021).
Article Google Scholar
Godsk, T., & Kjærgaard, M. B. High classification rates for continuous cow activity recognition using low-cost GPS positioning sensors and standard machine learning techniques. In Industrial Conference on Data Mining . 174–188 (Springer, 2011).
Riaboff, L. et al. Use of predicted behavior from accelerometer data combined with GPS data to explore the relationship between dairy cow behavior and pasture characteristics. Sensors 20 (17), 4741 (2020).
Article ADS PubMed PubMed Central Google Scholar
Tian, F. et al. Real-time behavioral recognition in dairy cows based on geomagnetism and acceleration information. IEEE Access 9 , 109497–109509 (2021).
Lovarelli, D. et al. Development of a new wearable 3D sensor node and innovative open classification system for dairy cows’ behavior. Animals 12 (11), 1447 (2022).
Article PubMed PubMed Central Google Scholar
Li, C. et al. Integrated data augmentation for accelerometer time series in behavior recognition: Roles of sampling, balancing, and Fourier surrogates. IEEE Sens. J. 22 (24), 24230–24241 (2022).
Article ADS Google Scholar
Guo, Y., Zhang, Z., He, D., Niu, J. & Tan, Y. Detection of cow mounting behavior using region geometry and optical flow characteristics. Comput. Electron. Agricult. 163 , 104828 (2019).
Girish, D., Singh, V., & Ralescu, A. Understanding action recognition in still images. In Proceedings of the IEEE/CVF Conference on Computer Vision and Pattern Recognition Workshops . 370–371 (2020).
Avola, D. et al. 2-D skeleton-based action recognition via two-branch stacked LSTM-RNNS. IEEE Trans. Multimed. 22 (10), 2481–2496 (2019).
Bhole, A., Falzon, O., Biehl, M., & Azzopardi, G. A computer vision pipeline that uses thermal and RGB images for the recognition of Holstein cattle. In Computer Analysis of Images and Patterns: 18th International Conference, CAIP 2019, Salerno, Italy, September 3–5, 2019, Proceedings, Part II 18 . 108–119 (Springer, 2019).
Jiang, B., Yin, X. & Song, H. Single-stream long-term optical flow convolution network for action recognition of lameness dairy cow. Comput. Electron. Agricult. 175 , 105536 (2020).
Wu, D., Han, M., Song, H., Song, L. & Duan, Y. Monitoring the respiratory behavior of multiple cows based on computer vision and deep learning. J. Dairy Sci. 106 (4), 2963–2979 (2023).
Article CAS PubMed Google Scholar
Fuentes, A., Yoon, S., Park, J. & Park, D. S. Deep learning-based hierarchical cattle behavior recognition with spatio-temporal information. Comput. Electron. Agricult. 177 , 105627 (2020).
Bai, Q. et al. X3dfast model for classifying dairy cow behaviors based on a two-pathway architecture. Sci. Rep. 13 (1), 20519 (2023).
Article ADS CAS PubMed PubMed Central Google Scholar
McDonagh, J. et al. Detecting dairy cow behavior using vision technology. Agriculture 11 (7), 675 (2021).
Liu, M. et al. Classification of cow behavior patterns using inertial measurement units and a fully convolutional network model. J. Dairy Sci. 106 (2), 1351–1359 (2023).
Meunier, B. et al. Image analysis to refine measurements of dairy cow behaviour from a real-time location system. Biosyst. Eng. 173 , 32–44 (2018).
Sharma, S., Kiros, R., & Salakhutdinov, R. Action recognition using visual attention. arXiv preprint arXiv:1511.04119 (2015).
Carreira, J., Noland, E., Banki-Horvath, A., Hillier, C., & Zisserman, A. A short note about kinetics-600. arXiv preprint arXiv:1808.01340 (2018).
Hoang, V.-D., Hoang, D.-H., & Hieu, C.-L. Action recognition based on sequential 2D-cnn for surveillance systems. In IECON 2018-44th Annual Conference of the IEEE Industrial Electronics Society . 3225–3230 (IEEE, 2018).
Guan, Y., Hu, W. & Hu, X. Abnormal behavior recognition using 3D-cnn combined with LSTM. Multimed. Tools Appl. 80 (12), 18787–18801 (2021).
Pengcheng, D., Siyuan, C., Zhenyu, Z., Zhigang, Z., Jingqi, M., & Huan, L. Human behavior recognition based on ic3d. In 2019 Chinese Control and Decision Conference (CCDC) . 3333–3337 (IEEE, 2019).
Feichtenhofer, C., Fan, H., Malik, J., & He, K. Slowfast networks for video recognition. In Proceedings of the IEEE/CVF International Conference on Computer Vision . 6202–6211 (2019).
Download references
Acknowledgements
This work was funded by National Natural Science Foundation of China (Grant No. 62261041).
Author information
Authors and affiliations.
College of Electronic Information Engineering, Inner Mongolia University, College Road No. 235, Hohhot, 010021, Inner Mongolia Autonomous Region, China
Kuo Li, Daoerji Fan, Huijuan Wu & Aruna Zhao
You can also search for this author in PubMed Google Scholar
Contributions
D.F. conceived the experiment, K.L. and H.W. conducted the experiment , A.Z. analysed the results. All authorsparticipated in the construction of the dataset and reviewed the manuscript.
Corresponding author
Correspondence to Daoerji Fan .
Ethics declarations
Competing interests.
The authors declare no competing interests.
Additional information
Publisher's note.
Springer Nature remains neutral with regard to jurisdictional claims in published maps and institutional affiliations.
Rights and permissions
Open Access This article is licensed under a Creative Commons Attribution 4.0 International License, which permits use, sharing, adaptation, distribution and reproduction in any medium or format, as long as you give appropriate credit to the original author(s) and the source, provide a link to the Creative Commons licence, and indicate if changes were made. The images or other third party material in this article are included in the article’s Creative Commons licence, unless indicated otherwise in a credit line to the material. If material is not included in the article’s Creative Commons licence and your intended use is not permitted by statutory regulation or exceeds the permitted use, you will need to obtain permission directly from the copyright holder. To view a copy of this licence, visit http://creativecommons.org/licenses/by/4.0/ .
Reprints and permissions
About this article
Cite this article.
Li, K., Fan, D., Wu, H. et al. A new dataset for video-based cow behavior recognition. Sci Rep 14 , 18702 (2024). https://doi.org/10.1038/s41598-024-65953-x
Download citation
Received : 07 January 2024
Accepted : 25 June 2024
Published : 12 August 2024
DOI : https://doi.org/10.1038/s41598-024-65953-x
Share this article
Anyone you share the following link with will be able to read this content:
Sorry, a shareable link is not currently available for this article.
Provided by the Springer Nature SharedIt content-sharing initiative
- Video sequence
- Dairy cow behavior recognition
- SlowFast model
By submitting a comment you agree to abide by our Terms and Community Guidelines . If you find something abusive or that does not comply with our terms or guidelines please flag it as inappropriate.
Quick links
- Explore articles by subject
- Guide to authors
- Editorial policies
Sign up for the Nature Briefing newsletter — what matters in science, free to your inbox daily.

Main Navigation
- Contact NeurIPS
- Code of Ethics
- Code of Conduct
- Create Profile
- Journal To Conference Track
- Diversity & Inclusion
- Proceedings
- Future Meetings
- Exhibitor Information
- Privacy Policy
NeurIPS 2024, the Thirty-eighth Annual Conference on Neural Information Processing Systems, will be held at the Vancouver Convention Center
Monday Dec 9 through Sunday Dec 15. Monday is an industry expo.

Registration
Pricing » Registration 2024 Registration Cancellation Policy »
Conference Hotels NeurIPS has contracted Hotel guest rooms for the Conference at group pricing, requiring reservations only through this page. Please do not make room reservations through any other channel, as it only impedes us from putting on the best Conference for you. We thank you for your assistance in helping us protect the NeurIPS conference.
Announcements
- See the Visa Information page for changes to the visa process for 2024.
- The call for High School Projects has been released
- The Call For Papers has been released
- The accepted competitions have been released.
Latest NeurIPS Blog Entries [ All Entries ]
Aug 02, 2024 | |
Jun 19, 2024 | |
Jun 04, 2024 | |
May 17, 2024 | |
May 07, 2024 | |
Apr 17, 2024 | |
Apr 15, 2024 | |
Mar 03, 2024 | |
Dec 11, 2023 | |
Dec 10, 2023 |
Important Dates
Mar 15 '24 11:46 AM PDT * | ||
Apr 05 '24 (Anywhere on Earth) | ||
Apr 21 '24 (Anywhere on Earth) | ||
Main Conference Paper Submission Deadline | May 22 '24 01:00 PM PDT * | |
May 22 '24 01:00 PM PDT * | ||
Jun 14 '24 (Anywhere on Earth) | ||
Aug 09 '24 06:00 PM PDT * | ||
Sep 05 '24 (Anywhere on Earth) | ||
Main Conference Author Notification | Sep 25 '24 06:00 PM PDT * | |
Datasets and Benchmarks - Author Notification | Sep 26 '24 (Anywhere on Earth) | |
Workshop Accept/Reject Notification Date | Oct 09 '24 (Anywhere on Earth) | |
Oct 23 '24 (Anywhere on Earth) | ||
Oct 30 '24 (Anywhere on Earth) | ||
Nov 15 '24 11:00 PM PST * | ||
Timezone: |
If you have questions about supporting the conference, please contact us .
View NeurIPS 2024 exhibitors » Become an 2024 Exhibitor Exhibitor Info »
Organizing Committee
General chair, program chair, workshop chair, workshop chair assistant, tutorial chair, competition chair, data and benchmark chair, affinity chair, diversity, inclusion and accessibility chair, ethics review chair, communication chair, social chair, journal chair, creative ai chair, workflow manager, logistics and it, mission statement.
The Neural Information Processing Systems Foundation is a non-profit corporation whose purpose is to foster the exchange of research advances in Artificial Intelligence and Machine Learning, principally by hosting an annual interdisciplinary academic conference with the highest ethical standards for a diverse and inclusive community.
About the Conference
The conference was founded in 1987 and is now a multi-track interdisciplinary annual meeting that includes invited talks, demonstrations, symposia, and oral and poster presentations of refereed papers. Along with the conference is a professional exposition focusing on machine learning in practice, a series of tutorials, and topical workshops that provide a less formal setting for the exchange of ideas.
More about the Neural Information Processing Systems foundation »
NeurIPS uses cookies to remember that you are logged in. By using our websites, you agree to the placement of cookies. |
VIDEO PROCESSING AND ITS APPLICATION

- Galgotias University

- Jagannath University
Discover the world's research
- 25+ million members
- 160+ million publication pages
- 2.3+ billion citations

- INT J COMPUT VISION

- Hans-Hellmut Nagel
- IMAGE VISION COMPUT
- Geoffrey D. Sullivan

- G. D. Sullivan
- W. Enkelmann
- PATTERN RECOGN

- Ka-Ming Leung

- Azriel Rosenfeld
- J SYST ARCHITECT
- Massimo Bertozzi

- Stefano Castelluccio
- Recruit researchers
- Join for free
- Login Email Tip: Most researchers use their institutional email address as their ResearchGate login Password Forgot password? Keep me logged in Log in or Continue with Google Welcome back! Please log in. Email · Hint Tip: Most researchers use their institutional email address as their ResearchGate login Password Forgot password? Keep me logged in Log in or Continue with Google No account? Sign up
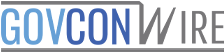
- Executive Mosaic
- Executive Biz
- Executive Gov
- Submit your news
- Tuesday, August 13, 2024
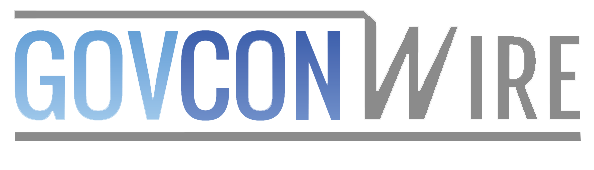
AFRL Seeks White Papers for $500M PRECIOUS Research Program
- August 8, 2024
- Acquisition & Procurement , DOD , News
The Materials and Manufacturing Directorate within the Air Force Research Laboratory is calling for white papers for the Pervasive Research & Evaluation for Complex-solutions In Operational & Urgent Systems program.
The move is the first in a two-step closed call for proposals for the PRECIOUS program , which aims to ensure the mission readiness of various Department of the Air Force customers by researching, prototyping, demonstrating and transitioning materials and process technologies, according to a solicitation posted Monday on SAM.gov.
The M&P technologies the program has in view include specialty materials; composite and polymer systems; metals and mechanical systems; electrical and electronics materials; nondestructive evaluation; chemical analysis; other nonmetallic aerospace materials; manufacturing technologies; energy and resource technologies; and biomaterials and biomanufacturing.
The PRECIOUS program is expected to result in a multiple-award, indefinite-delivery/indefinite-quantity contract with a maximum value of $500 million, which will be accompanied by an initial pair of task orders to be awarded in January 2025.
Interested parties have until Sept. 6 to submit white papers.
Video of the Day
Register Here
- Apply to UMaine
Watershed Process and Estuary Sustainability Research Group
Flow regimes in the east branch of the penobscot river are a focus of wpes summer research activities.
Undergraduate research assistant, Izaak Krause, from the Dept. of Civil and Environmental Engineering is working with Bea Van Dam and Sean Smith to assemble historic information, compile hydrologic and spatial data, and parameterize a watershed hydrologic model to evaluate flow regimes affecting Atlantic salmon habitat in the East Branch of the Penobscot River. Sponsored by Maine Sea Grant and led by the Penobscot Nation, the project is focused on the examination of scenarios related to watershed conditions, climate, civil infrastructure, and human generated disturbances to help guide river management decision-making. Progress this summer has included field observations, data development, and watershed model construction to advance our understanding of the many factors affecting watershed and river conditions.
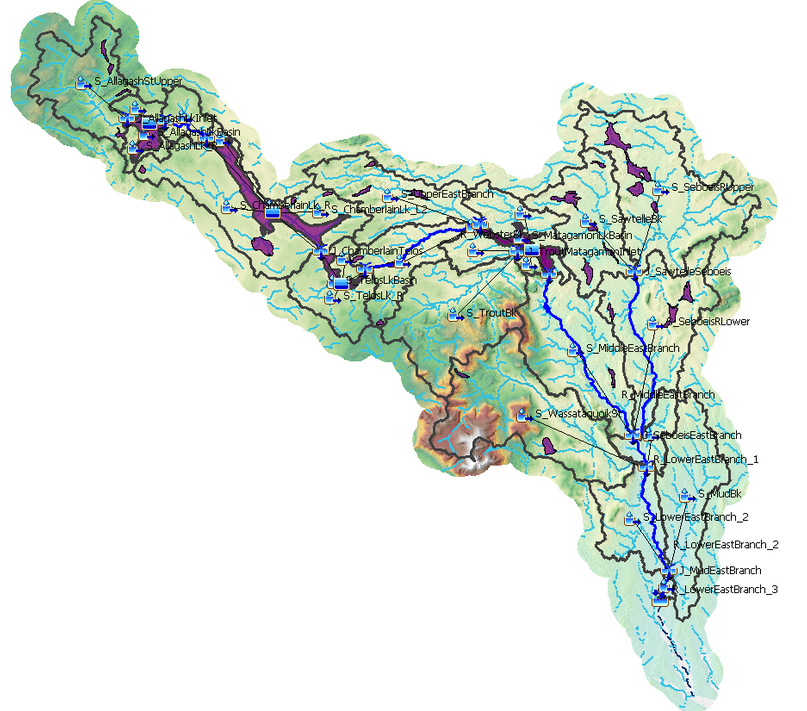
Video Signal Processing
- First Online: 10 September 2017
Cite this chapter
- Yung-Lin Huang 2
836 Accesses
Watching video is the most popular way for people to enjoy multimedia contents. However, videos are required to be displayed at different rate because there are many different display devices nowadays. In this chapter, we introduce the background knowledge, applications, and technical details of current multirate video systems. First, we introduce the basic concept and knowledge of the video system. In addition, several examples of multirate video applications are shown. Afterward, we explain the key techniques to achieve these applications from the fundamental frame rate conversion (FRC) and the advanced frame rate up-conversion (FRUC). We also state the motivation of higher frame rate before the FRUC techniques are presented. The general flow diagram of the FRUC techniques is given, and the technical details are then discussed and stated. Moreover, several evaluation methods are explained, and the popular video datasets are shown. In addition to different algorithms, we discuss the requirement and recent researches of hardware implementation for FRUC techniques. Finally, a conclusion and discussion are given.
This is a preview of subscription content, log in via an institution to check access.
Access this chapter
Subscribe and save.
- Get 10 units per month
- Download Article/Chapter or eBook
- 1 Unit = 1 Article or 1 Chapter
- Cancel anytime
- Available as PDF
- Read on any device
- Instant download
- Own it forever
- Available as EPUB and PDF
- Compact, lightweight edition
- Dispatched in 3 to 5 business days
- Free shipping worldwide - see info
- Durable hardcover edition
Tax calculation will be finalised at checkout
Purchases are for personal use only
Institutional subscriptions
Similar content being viewed by others
Video Compression
TV and Video Processing
Wiegand, T., Sullivan, G. J., Bjontegaard, G., & Luthra, A. (2003). Overview of the H. 264/AVC video coding standard. IEEE Transactions on Circuits and Systems for Video Technology, 13 (7), 560–576.
Article Google Scholar
Sullivan, G. J., Ohm, J., Han, W. J., & Wiegand, T. (2012). Overview of the high efficiency video coding (HEVC) standard. IEEE Transactions on Circuits and Systems for Video Technology, 22 (12), 1649–1668.
Poynton, C. (2012). Digital video and HD: Algorithms and interfaces . Amsterdam: Elsevier.
Google Scholar
Pan, H., Feng, X., & Daly, S. (2005). 51.4: Quantitative analysis of LCD motion blur and performance of existing approaches. In SID symposium digest of technical papers (Vol. 36, No. 1, pp. 1590–1593). Blackwell Publishing Ltd, UK.
Pan, H., Feng, X. F., & Daly, S. (2005). LCD motion blur modeling and analysis . In Proceedings of IEEE international conference of image processing ( ICIP) . (Vol. 2, pp. II–21).
De Haan, G., Biezen, P. W., Huijgen, H., & Ojo, O. A. (1993). True-motion estimation with 3-D recursive search block matching. IEEE Transactions on Circuits and Systems for Video Technology, 3 (5), 368–379.
Wang, J., Wang, D., & Zhang, W. (2003). Temporal compensated motion estimation with simple block-based prediction. IEEE Transactions on Broadcasting, 49 (3), 241–248.
Tourapis, A. M. (2002, January). Enhanced predictive zonal search for single and multiple frame motion estimation. In Proceedings of SPIE visual communications and image processing (VCIP) (pp. 1069–1079).
Kim, U. S., & Sunwoo, M. H. (2014). New frame rate up-conversion algorithms with low computational complexity. IEEE Transactions on Circuits and Systems for Video Technology, 24 (3), 384–393.
Min, K. Y., & Sim, D. G. (2013). Confidence-based adaptive frame rate up-conversion. EURASIP Journal on Advances in Signal Processing, 2013 (1), 1–12.
Liu, H., Xiong, R., Zhao, D., Ma, S., & Gao, W. (2012). Multiple hypotheses Bayesian frame rate up-conversion by adaptive fusion of motion-compensated interpolations. IEEE Transactions on Circuits and Systems for Video Technology, 22 (8), 1188–1198.
Kaviani, H., & Shirani, S. (2016). Frame rate up-conversion using optical flow and patch-based reconstruction. IEEE Transactions on Circuits and Systems for Video Technology, 26 (9), 1581–1594.
Yang, Y. T., Tung, Y. S., & Wu, J. L. (2007). Quality enhancement of frame rate up-converted video by adaptive frame skip and reliable motion extraction. IEEE Transactions on Circuits and Systems for Video Technology, 17 (12), 1700–1713.
Huang, A. M., & Nguyen, T. Q. (2008). A multistage motion vector processing method for motion-compensated frame interpolation. IEEE Transactions on Image Processing, 17 (5), 694–708.
Article MathSciNet Google Scholar
Liu, Y. N., Wang, Y. T., & Chien, S. Y. (2011). Motion blur reduction of liquid crystal displays using perception-aware motion compensated frame rate up-conversion. In Proceedings of IEEE workshop on signal processing systems (SiPS) (pp. 84–89).
Gao, X. Q., Duanmu, C. J., & Zou, C. R. (2000). A multilevel successive elimination algorithm for block matching motion estimation. IEEE Transactions on Image Processing, 9 (3), 501–504.
Chen, F. C., Huang, Y. L., & Chien, S. Y. (2012). Hardware-efficient true motion estimator based on Markov Random Field motion vector correction. In Proceedings of IEEE international symposium on VLSI design, automation, and test (VLSI-DAT) (pp. 1–4).
Li, R., Zeng, B., & Liou, M. L. (1994). A new three-step search algorithm for block motion estimation. IEEE Transactions on Circuits and Systems for Video Technology, 4 (4), 438–442.
Po, L. M., & Ma, W. C. (1996). A novel four-step search algorithm for fast block motion estimation. IEEE Transactions on Circuits and Systems for Video Technology, 6 (3), 313–317.
Lucas, B. D., & Kanade, T. (1981). An iterative image registration technique with an application to stereo vision. In Proceedings of the 7th international joint conference on Artificial intelligence - Volume 2 (IJCAI'81) (Vol. 2, pp. 674–679). San Francisco, CA: Morgan Kaufmann Publishers Inc.
Tang, C. W., & Au, O. C. (1998, May). Comparison between block-based and pixel-based temporal interpolation for video coding. In Proceedings of IEEE international symposium on circuits and systems (ISCAS) , (Vol. 4, pp. 122–125).
Lee, W. H., Choi, K., & Ra, J. B. (2014). Frame rate up conversion based on variational image fusion. IEEE Transactions on Image Processing, 23 (1), 399–412.
Liu, C. (2009). Beyond pixels: exploring new representations and applications for motion analysis . Doctoral dissertation, Massachusetts Institute of Technology.
Huang, Y. L., Liu, Y. N., & Chien, S. Y. (2010, October). MRF-based true motion estimation using H. 264 decoding information. In IEEE workshop on signal processing systems (SIPS) (pp. 99–104).
H.264/AVC Software Coordination. http://iphome.hhi.de/suehring/tml/
Astola, J., Haavisto, P., & Neuvo, Y. (1990). Vector median filters. Proceedings of the IEEE, 78 (4), 678–689.
Dane, G., & Nguyen, T. Q. (2004). Smooth motion vector resampling for standard compatible video post-processing. In Proceedings of IEEE Asilomar conference on signals, systems and computers (Vol. 2, pp. 1731–1735).
Wang, D., Zhang, L., & Vincent, A. (2010). Motion-compensated frame rate up-conversion—Part I: Fast multi-frame motion estimation. IEEE Transactions on Broadcasting, 56 (2), 133–141.
Li, S. Z. (1994, May). Markov random field models in computer vision. In European conference on computer vision (pp. 361–370). Berlin/Heidelberg: Springer.
Huang, Y. L., Chen, F. C., & Chien, S. Y. (2016). Algorithm and architecture design of multi-rate frame rate up-conversion for ultra-HD LCD systems. IEEE Transactions on Circuits and Systems for Video Technology, PP (99), 1–1. doi: 10.1109/TCSVT.2016.2596198 .
Jeon, B. W., Lee, G. I., Lee, S. H., & Park, R. H. (2003). Coarse-to-fine frame interpolation for frame rate up-conversion using pyramid structure. IEEE Transactions on Consumer Electronics, 49 (3), 499–508.
Choi, B. T., Lee, S. H., & Ko, S. J. (2000). New frame rate up-conversion using bi-directional motion estimation. IEEE Transactions on Consumer Electronics, 46 (3), 603–609.
Choi, B. D., Han, J. W., Kim, C. S., & Ko, S. J. (2007). Motion-compensated frame interpolation using bilateral motion estimation and adaptive overlapped block motion compensation. IEEE Transactions on Circuits and Systems for Video Technology, 17 (4), 407–416.
Min, K. Y., Ma, J. H., Sim, D. G., & Bajic, I. V. (2015). Bidirectional mesh-based frame rate up-conversion. IEEE Multimedia, 22 (2), 36–45.
Orchard, M. T., & Sullivan, G. J. (1994). Overlapped block motion compensation: An estimation-theoretic approach. IEEE Transactions on Image Processing, 3 (5), 693–699.
Ling, Y., Wang, J., Liu, Y., & Zhang, W. (2008). A novel spatial and temporal correlation integrated based motion-compensated interpolation for frame rate up-conversion. IEEE Transactions on Consumer Electronics, 54 (2), 863–869.
Hsu, K. Y., & Chien, S. Y. (2008). Frame rate up-conversion with global-to-local iterative motion compensated interpolation. In Proceedings of IEEE international conference on multimedia and expo (ICME) , (pp. 161–164).
Huang, A. M., & Nguyen, T. (2009). Correlation-based motion vector processing with adaptive interpolation scheme for motion-compensated frame interpolation. IEEE Transactions on Image Processing, 18 (4), 740–752.
Xiph.org Video Test Media. https://media.xiph.org/video/derf/
YUV Video Sequences. http://trace.eas.asu.edu/yuv/
Baker, S., Scharstein, D., Lewis, J. P., Roth, S., Black, M. J., & Szeliski, R. (2011). A database and evaluation methodology for optical flow. International Journal of Computer Vision, 92 (1), 1–31.
Middlebury Optical Flow Dataset. http://vision.middlebury.edu/flow/
Heinrich, A., de Haan, G., & Cordes, C. N. (2008). A novel performance measure for picture rate conversion methods. In Proceedings of IEEE international conference on consumer electronics (ICCE) , (pp. 1–2).
Wang, Z., Bovik, A. C., Sheikh, H. R., & Simoncelli, E. P. (2004). Image quality assessment: From error visibility to structural similarity. IEEE Transactions on Image Processing, 13 (4), 600–612.
Kang, S. J., Yoo, D. G., Lee, S. K., & Kim, Y. H. (2008, November). Hardware implementation of motion estimation using a sub-sampled block for frame rate up-conversion. In IEEE international SoC design conference (Vol. 2, pp. II-101).
Cetin, M., & Hamzaoglu, I. (2011). An adaptive true motion estimation algorithm for frame rate conversion of high definition video and its hardware implementations. IEEE Transactions on Consumer Electronics, 57 (2), 923–931.
Lee, G. G., Chen, C. F., Hsiao, C. J., & Wu, J. C. (2014). Bi-directional trajectory tracking with variable block-size motion estimation for frame rate up-convertor. IEEE Journal on Emerging and Selected Topics in Circuits and Systems (JETCAS), 4 (1), 29–42.
Wang, Y.T. (2010). Algorithm and hardware architecture design of perception-aware motion compensated frame rate up-conversion . Master’s thesis, National Taiwan University.
Hsu, K. Y., & Chien, S. Y. (2011). Hardware architecture design of frame rate up-conversion for high definition videos with global motion estimation and compensation. In Proceedings of IEEE workshop on signal processing systems (SiPS) , (pp. 90–95).
Download references
Author information
Authors and affiliations.
National Taiwan University, Taipei City, Taiwan
Yung-Lin Huang
You can also search for this author in PubMed Google Scholar
Corresponding author
Correspondence to Yung-Lin Huang .
Editor information
Editors and affiliations.
Department of Electronics, Institute National INAOE, Tonantzintla, Puebla, Mexico
Gordana Jovanovic Dolecek
Rights and permissions
Reprints and permissions
Copyright information
© 2018 Springer International Publishing AG
About this chapter
Huang, YL. (2018). Video Signal Processing. In: Dolecek, G. (eds) Advances in Multirate Systems . Springer, Cham. https://doi.org/10.1007/978-3-319-59274-9_6
Download citation
DOI : https://doi.org/10.1007/978-3-319-59274-9_6
Published : 10 September 2017
Publisher Name : Springer, Cham
Print ISBN : 978-3-319-59273-2
Online ISBN : 978-3-319-59274-9
eBook Packages : Engineering Engineering (R0)
Share this chapter
Anyone you share the following link with will be able to read this content:
Sorry, a shareable link is not currently available for this article.
Provided by the Springer Nature SharedIt content-sharing initiative
- Publish with us
Policies and ethics
- Find a journal
- Track your research

IMAGES
COMMENTS
Studies show lots of advanced research on various data types such as image, speech, and text using deep learning techniques, but nowadays, research on video processing is also an emerging field of computer vision. Several surveys are present on video processing using computer vision deep learning techniques, targeting specific functionality such as anomaly detection, crowd analysis, activity ...
This paper aims to present a Systematic Literature Review (SLR) on video processing using deep learning to investigate the applications, functionalities, techniques, datasets, issues, and challenges by formulating the relevant research questions (RQs). This systematic mapping includes 93 research articles from reputed databases published ...
Review (SLR) on video processing using deep learning to in vestigate the applications, functionalities, techniques, datasets, issues, and challenges by formulating the relevant research questions ...
Stay informed on the latest trending ML papers with code, research developments, libraries, methods, and datasets. ... In this paper, we introduce a network architecture that takes long-term content into account and enables fast per-video processing at the same time. 6.
Overview. Signal, Image and Video Processing is an interdisciplinary journal focusing on theory and practice of signal, image and video processing. Sets forth practical solutions for current signal, image and video processing problems in engineering and science. Features reviews, tutorials, and accounts of practical developments.
A Systematic Literature Review (SLR) on video processing using deep learning to investigate the applications, functionalities, techniques, datasets, issues, and challenges by formulating the relevant research questions (RQs). Studies show lots of advanced research on various data types such as image, speech, and text using deep learning techniques, but nowadays, research on video processing is ...
Explore the latest full-text research PDFs, articles, conference papers, preprints and more on IMAGE AND VIDEO PROCESSING. Find methods information, sources, references or conduct a literature ...
One of the critical multimedia analysis problems in today's digital world is video summarization (VS). Many VS methods have been suggested based on deep learning methods. Nevertheless, These are inefficient in processing, extracting, and deriving information in the minimum amount of time from long-duration videos. Detailed analysis and investigation of numerous deep learning approach ...
carolineec/EverybodyDanceNow • • ICCV 2019. This paper presents a simple method for "do as I do" motion transfer: given a source video of a person dancing, we can transfer that performance to a novel (amateur) target after only a few minutes of the target subject performing standard moves. 13. Paper. Code.
His research areas include signal and image processing, real-time processing on embedded processors, deep learning, and machine learning. He has authored or co-authored more than 400 publications and 9 other books pertaining to signal and image processing, and regularly teaches the signals and systems laboratory course, for which this book is ...
Video processing is a significant field of research interest in recent years. Before going into the recent advancement of video processing, an overview about the traditional video processing is a matter of interest. Knowing about this, its advantages and limitations help to give a strong base and invoke an insight into the further development of this research area. This paper introduces the ...
The features of big data could be captured by DL automatically and efficiently. The current applications of DL include computer vision (CV), natural language processing (NLP), video/speech recognition (V/SP), and finance and banking (F&B). Chai and Li (2019) provided a survey of DL on NLP and the advances on V/SP. The survey emphasized the ...
Image and Video Processing Authors and titles for July 2024 . Total of 76 entries : 1-25 26-50 51-75 76-76. Showing up to 25 entries per page: ... Comments: Best oral paper award at ISBI 2024 Subjects: Image and Video Processing (eess.IV); Computer Vision and Pattern Recognition (cs.CV); Machine Learning (cs.LG)
EURASIP Journal on Image and Video Processing (JIVP) welcomes Special Issues on timely topics related to the field of signal processing.The objective of Special Issues is to bring together recent and high quality works in a research domain, to promote key advances in the multidisciplinary field of image and video processing that covers all theoretical and practical aspects of the domain, from ...
[email protected]. Abstract. Image and Video Processing are hot topics in the field of research and development. Image processing is any form of signal processing for which the input is an image ...
Added 6 November 2014 • Updated 30 May 2019. A peer-reviewed, open access journal in computer vision, medical imaging, image and video processing, video segmentation and analysis, computer graphics and visualization & pattern recognition.
We are pleased to announce that the following paper published in EURASIP Journal on Image and Video Processing has been awarded a EURASIP best paper award! Research. DIBR synthesized image quality assessment based on morphological multiscale approach Dragana Sandić-Stanković, Dragan Kukolj, and Patrick Le Callet
Year-wise distribution of the publication. list of publications we considered purely to answer the RQs is between the time range 2011-2020, and few papers which are beyond time range are used only for background Study III. RESULTS A total of 93 peer-reviewed research papers on video processing using deep learning techniques were studied.
Modèles et méthodes de traitement d'images pour l'analyse de la langue des signes. This paper focuses on methods applied for sign language video processing. In the first part, we present a robust traking method which detects and tracks the hands and face of a person performing Signs' language communication.
Subjects:Computer Vision and Pattern Recognition (cs.CV) [7] arXiv:2408.04594 [ pdf, html, other ] Title: Img-Diff: Contrastive Data Synthesis for Multimodal Large Language Models. Qirui Jiao, Daoyuan Chen, Yilun Huang, Yaliang Li, Ying Shen. Comments: 14 pages, 9 figures, 7 tables.
Chengcheng Wang. Xiaofeng Wang. Original Paper 22 July 2024 Pages: 7369 - 7381. 1. 2. …. 69. Next. Signal, Image and Video Processing is an interdisciplinary journal focusing on theory and practice of signal, image and video processing.
1 Introduction. Across much of the marine environment, our understanding of the structure and distribution of habitats on the seabed is limited (Kostylev, 2012; Mayer et al., 2018; Menandro and Bastos, 2020).For marine habitats at depth, remotely operated underwater vehicles (ROVs), and towed and drop cameras, deployed from vessels, are gaining increasing use to collect high-resolution video ...
A new video based multi behavior dataset for cows, CBVD-5, is introduced in this paper. The dataset includes five cow behaviors: standing, lying down, foraging,rumination and drinking. The dataset ...
The Neural Information Processing Systems Foundation is a non-profit corporation whose purpose is to foster the exchange of research advances in Artificial Intelligence and Machine Learning, principally by hosting an annual interdisciplinary academic conference with the highest ethical standards for a diverse and inclusive community.
Introduction. Video proce ssing is a specific instance of sign p rocessing, whic h frequently utilizes video channels and where the info and yield. signals are video records or video tr ansfers ...
The Materials and Manufacturing Directorate within the Air Force Research Laboratory is calling for white papers for the Pervasive Research & Evaluation for Complex-solutions In Operational ...
IET Image Processing journal publishes the latest research in image and video processing, covering the generation, processing and communication of visual information. ... The model in this paper was trained 100 epochs on NVIDIA RTX 3090 GPU and presented in Table 2, which described the basic setting for other parameters while training the model ...
Undergraduate research assistant, Izaak Krause, from the Dept. of Civil and Environmental Engineering is working with Bea Van Dam and Sean Smith to assemble historic information, compile hydrologic and spatial data, and parameterize a watershed hydrologic model to evaluate flow regimes affecting Atlantic salmon habitat in the East Branch of the Penobscot River. Sponsored by […]
In the current research, we ask whether sensorimotor engagement—operationalized as watching a video of hands manipulating paper representations—offers unique benefits beyond the visuospatial concreteness of a dynamic visualization of the same process. Participants were randomly assigned to one of three conditions to learn about the shuffle ...
For example, movie films are at 24 FPS, and the videos recorded by mobile phone are often at 30 or 60 FPS. Fig. 1. Illustration of image and video signal. A video signal consists of a sequence of images . It is a three-dimensional signal including x -direction, y -direction, and temporal domain. Full size image.