

Wayanad tragedy: Landslides natural.. can’t prevent them but impact can be minimised
WAYANAD: The devastating landslides in Wayanad that killed hundreds and left thousands homeless have once again brought climate change to the limelight.
Amid the raging debate over whether the landslides were caused by natural fury or human intervention, TNIE brings together four experts to ponder over the causes.
In a freewheeling chat, Dr V Ambili, deputy director general, Geological Survey of India, Kerala unit, Dr K G Thara, former head, Disaster Management Centre, Kerala, Dr Sajin Kumar K S assistant professor in geology, University of Kerala, and Kerala State Landslide Advisory Committee member and Sridhar Radhakrishnan, environmentalist and policy observer, deliberate on the reasons, contributing factors, Kerala’s vulnerability, and disaster preparedness.
We’ve just witnessed two devastating landslides in Wayanad. What caused them?
Ambili: Many factors influence landslides. Kerala’s terrain, with its tropical humid climate, is prone to landslides. This landslide started at an altitude of 1,500m and later had a sharp drop to 800m. Besides intense soil formation and a sharp slope, the entire terrain is a tectonically active zone, especially towards the northern part. My study of the Chaliyar basin has clearly shown the river exhibiting old-age symptoms (like braiding and delta formation at the confluence with the sea) in the early stages of its course. The presence of a valley in Nilambur in the upper reaches shows signs of tectonic activity. It has been proved that the area comes under Zone-2 for neotectonic activity. This means there are fractures in the rocks that get reactivated under weather conditions (heavy rain).
What symptoms are usually seen in a landslide-prone area?
Ambili: The river is seen braiding here, in its upper reaches. Water always follows gravity. But if the river takes a turn, it is only because of a groove-like structure to facilitate it. During the landslide, the debris came down en masse, breaching the normal course.
What are the characteristics of such a zone?
Ambili: Weather is a major factor in determining landslides in a susceptible area. Other factors are slope, type of soil, structure of rocks, aspect etc. When there is a geological change, all such factors get reactivated. Water has ways to seep out of rocks as springs. But when the rain is heavy and continuous, it goes beyond the capacity of rocks to withhold water. When it crosses the threshold limit, these burst with disastrous effect.
Does human intervention figure in this?
Ambili: Human intervention is not the reason behind this landslide. The rocks couldn’t hold the water due to torrential rain in a place with a high slope.
What kind of human activities contribute to landslides?
Ambili: Quarries are a major contributor. We operate quarries without studying the geology of the area. There are many fractured quarries. The blasting that takes place in quarries will result in rock-fall in other locations. Plantation also contributes to landslides. It should be suited to the location. Plants should be chosen according to the soil and rock types. People have the habit of obstructing first-order streams to prevent them from entering their property. If we block the flow, the water seeps down and exerts pressure, resulting in smaller landslides. The landslides at Erattupetta were due to such human interventions. In Thrissur, we have seen people converting streams into pathways and roads.
Dr Thara, how do you see the current landslides from a disaster-management perspective?
Thara: Human interventions aggravate landslides. This landslide happened in a forest area above a populated area. The township was developed to facilitate plantations. The valley marked by such mono-crop cultivation dilutes the biodiversity required to prevent a landslide. There are large-scale human interventions in the name of promoting tourism. A lineament map gives a picture of rock-fractures beneath the soil. Calamities happen at places with maximum lineaments, the impact of which increases through human interventions.
You spoke about tourist inflow. Have we conducted any studies on the carrying capacity of Wayanad?
Thara: No, we haven’t. Any place with a slope greater than 20 degrees is landslide-prone. In Kerala, more than 50% of places are like that. When we construct multi-storey buildings atop hills, they exert greater pressure because of their weight, and landslides happen. Similarly, cutting the toe-end of the slope to build houses or canals also causes landslides.
The Wayanad landslide should have been declared a national calamity the very next day. The fact that they haven’t done it can be termed as apathy or a lack of political will. We may also have to term the construction of national highway as a national calamity... there are no safety precautions adopted.
Sridhar, in your opinion, has human intervention aggravated landslides at Mundakkai and Chooralmala?
Sridhar: I have gone through the landslide data between 1950 and 2018. Wayanad had a forest area of 85% in the 50s. By 2018, 62% of it was lost. At the same time, the plantation area has increased by 1,500% during the period. We should look at the Madhav Gadgil panel report in the light of the seven-decade long interventions made. According to him, 75% of the Western Ghats should be included in the list of ecologically sensitive areas. Both Meppadi panchayat (under which falls Mundakkai and Chooralmala) and Vellarimala were included in Zone-I. The areas where the landslides happened were tea estates. The second issue that we are clearly facing is the impact of climate change. According to a report by the Hume Centre for Ecology and Biodiversity, a staggering 4,000mm rain occured in areas where they had issued warnings, from June to July 31. Why then were no steps taken for preparation? Is this man-made or caused by nature? That climate change is human-induced, is a settled scientific position. To correct this, we need time. Instead, we are aggravating it all the more. For instance, if 10 lakh people had travelled to these landslide-prone areas, what does it mean?
Dr Sajin, what, according to you, are the causes for the landslides in Wayanad?
Sajin: I can emphatically say that it is not human-induced. I have been fully engaged with landslides, exclusively in the Kerala context, since 2018. There were 4,726 landslides — big and small — in the state in 2018 alone. Half of them occurred in Idukki district – 2,223 — including many in deep forests. How do we explain these? If these were induced by human interference, it could have occurred even in the months of March, April and May. However, 99.9% of our landslides are seen from June to October/November. Rain is hence the real trigger. But the rain that usually falls within a year’s time, is now pouring with high intensity in a much shorter period, and landslides happen.
Is it possible to predict landslides?
Sajin: We may be able to predict a landslide. However, no one can identify the exact route the runoff-rainwater would take. The best example of this was seen at Pettimudi. The same thing happened at Meppadi. Both Pettimudi township and Chooralmala are not landslide-prone areas. In addition to the landslide-susceptibility map, we also need a landslide-route map. Frankly, this is not a human-made landslide. But it doesn’t mean that human activities played no role at all.
Sridhar, if you believe that the landslides are down to human intervention, what do you have to say to Dr Sajin’s observations?
Sridhar: I didn’t say that the landslide happened because of human interventions. What I meant was the extent of casualty that occurred was purely due to human error.
What’s the role of human activities in the Wayanad landslides?
Sridhar: Though the landslides in Wayanad are a natural disaster, human actions significantly contributed to increased casualties. One major factor is the increasing tourism activities in ecologically vulnerable areas like Meppadi. Allowing construction in such areas is a grave human error that exacerbates the impact of landslides. Attributing these disasters solely to rain, as some scientists and the public do, oversimplifies the issue and turns it into a mere political statement. It’s crucial to acknowledge that climate change, driven by human interventions, does play a significant role in such events.
What are the solutions to avoid such occurrences in the future?
Ambili: The Geological Survey of India launched a National Landslide Forecasting Centre in Kerala on July 19. This system utilises historical landslide data from Kerala to provide more accurate landslide forecasts. The system has been operational in Darjeeling and Nilgiris since 2020 and it’s now a full-fledged system there. Trial runs for landslide alerts in Kerala began on July 20. The centre offers an online portal and application where both officials and the public can provide real-time data inputs, including rainfall observations, which is crucial for precise predictions. This initiative aims to enhance landslide preparedness and mitigation efforts through collaborative data-sharing and analysis. Reliable rainfall data is key for accurate landslide predictions. The primary data we use to generate predictions is the rainfall data provided by IMD (India Meteorological Department). In this case, based on the rainfall data provided by IMD at 2.30pm on July 29, we issued a green alert. However, there was a technical glitch. We are now planning to take data from other agencies too, as there are active rain gauges in the region. Making real-time satellite data available will also be crucial, allowing us to continuously monitor the situation.
Do you think insufficient funding for post-disaster research activities and limited sharing of scientific data between agencies pose a threat to mitigating disasters?
Sajin: There is a lack of funding for post-disaster research activities in India. While disasters in Kerala, like the Wayanad landslides, attract global research attention, there’s a noticeable absence of dedicated funding for such studies within the country. This hinders in-depth investigations and the development of locally relevant mitigation strategies. Sharing data between agencies is crucial for research activities. While international agencies openly share data, it remains unavailable in the public domain here. This hinders collaborative research and the development of effective solutions.
The experiences from the 2018 flood and the 2024 landslides indicate that rainfall predictions by IMD are unreliable…
Thara: The claims by Union Defence Minister Amit Shah that the Centre issued a landslide warning four days in advance are unscientific. Predicting a landslide four days prior is impossible. The IMD upgraded the warning to a red alert only after the landslide occurred, proving the claims to be false. Pinpointing the exact moment a landslide will be triggered is impossible, making the issuing of alerts extremely challenging. While we cannot prevent such disasters, we can mitigate their impact.
Sajin: Existing rain gauges were installed to support farming activities, and not specifically to monitor landslide-prone areas. Consequently, their placement and data collection may not be adequate to provide effective landslide warnings.
Ambili: Adequate and strategically located rain gauges are not there in critically vulnerable landslide-prone areas in Wayanad.
What can be done to avoid a repetition of such tragedies in future?
Ambili: Landslides are a natural phenomenon, and while we cannot prevent them entirely, we can take steps to minimise their impact. As experts suggest, reducing human interventions in ecologically sensitive areas can help avoid exacerbating these disasters.
Sajin: We should create awareness on the lines of what was done in the ‘80s and ‘90s. We should visit landslide-prone areas in April-May. Video classes by experts should be shown in schools. Students will spread the message on what needs to be done before and after a landslide. Such video demonstrations can be a viable solution if we don’t have funds for mock drills.
Do you mean to say that it should be made part of the curriculum?
Ambili: This has been implemented well in the northeastern states. Students are equipped to predict landslides when there is any suspicious movement and take precautionary action. Such training is necessary.
Can we predict landslides accurately using rain gauges?
Ambili: We cannot do it accurately. If heavy rain stops suddenly, a landslide may not happen. So, it is highly sensitive. When the alert is not up to the mark a few times, people will lose their trust.
Sajin: I beg to differ on that aspect. We now consider the rainfall of the previous day. Actually, the world over, what is being checked is the rainfall in the last 30 days. By analysing one month of rain, you will make a trial and error combination. We should look at how much rainfall is needed to saturate a certain amount of soil. When the level reaches 50%, you should give the first warning and ask people to evacuate.
Thara: Even if you give an alert when it reaches the threshold, you are going to speak to people who are asleep. An alert system is the most important factor. Unless the alert reaches the common man, nothing can be done. Secondly, rainfall is not the only factor. We construct national highways, homestays, and remove large amounts of soil. Each day, the vulnerability is increasing. I don’t think setting up rain gauges will solve the problem. People should know where to go when alerts reach them. There should be a socially-oriented disaster management plan.
But people wake up after the landslide and are clueless where to go...
Thara: In the matter of landslides, we have limitations. There is very little you can do after getting an alert but there is a lot of scope in things that can be done before that. In the case of a flood, you may get time but not in the case of a landslide. We should examine how many public offices are located in flood plains, which means an area of half a kilometre on either side. This area is for the river to flow freely or else it will bring huge rocks when it flows through a narrow strip. There should be efforts to relocate people from flood plains.
Dr Sajin, as a landslide expert, do you think quarrying and plantations contribute to landslides?
Sajin: If we look at Kavalappara, the other side is a rubber plantation. I could see rainwater pits after the landslide. The government supports rainwater pits but many don’t know where it should be done, scientifically. For rubber, farmers dig huge pits to hold water. After 20 years, the rubber plantation is destroyed. Earthmovers remove the root, totally disturbing the area. Instead, stubble mulching is the ideal method to be followed, where the root is retained. Plantation is a real cause, except for tea plantations.
Ambili: Usually, areas under tea-coffee cultivation are relatively less problematic. But when replantation happens, it is disturbing. Also, in places with more than a 20-degree slope, ideally, there should be no pits for rainwater-harvesting.
What about quarrying?
Sajin: All hard rocks seen in Kerala are at least 250 crore years old. But the top soil is only 10,000 to 15,000 years old. Studies have shown that the waves of uncontrolled blast cause a vibration of up to 200 metres. There will also be a lot of cracks due to quarrying. I won’t say it causes landslides, but it can facilitate the same. During monsoons, quarrying should be stopped. They can conduct sales, but shouldn’t go for blasting during the rainy season.
Thara: We cannot toe the approach that quarries are unavoidable as building materials are required for developmental activities. The Building Material Technology Council has come up with several alternatives to rock, sand, and other such building materials. There are many options available now. Why don’t we use them? We can look at options like fly ash and hollow bricks. In fact, we can re-use materials from demolished buildings and illegal constructions. We can also say that in landslide-susceptible zones, private buildings shouldn’t exceed a stipulated area. Also, do we need a six-lane road? We should look at alternatives, like improving the public transport system.
We all need good roads and we all travel by cars on these roads. So, is it correct to take such a hypocritical stand?
Sridhar: It is not a hypocritical stand. Per se, we are not saying no to roads. We need good roads. But what we now have are murderous roads where people die because of potholes.
Sajin: Those who oppose six-lane highways will go to Bengaluru via Tamil Nadu (laughs out).
Now that landslides are a reality, we can only adapt to them...
Sridhar: It’s not just about adapting. It’s about preparing for the same and creating resilience. Preparedness is an important aspect. The authorities should have data on who all are equipped to move out, who all should be evacuated, and how to facilitate the same. We have become experts in post-disaster management. We learnt that lesson the hard way. We have also become a sensitive society in providing relief. We should establish a similar mechanism for disaster-preparedness too.
Thara: We need measures in place to mitigate the same.
Sridhar: We should look at the fact that the government is planning a tunnel project in this area. The 6.9km four-lane road project will require blasting. There’s also a 1,000-acre project facilitated by the government — Asia’s biggest tourism destination with an airport — coming up in the same panchayat.
Will the proposed tunnel project accentuate the vulnerability of Wayanad?
Sajin: Not really. We now have many sophisticated technologies. If we drill using sophisticated technology, it won’t harm the environment. When a new path is created, we save a lot of money. If there are good roads, all of us will use the same. Let’s say there’s no tunnel, and if you want to go to Wayanad, will you risk it? We would be scared to go via the mountain pass. When you have a tunnel, you feel safe.
Sridhar: If you look at the alignment of the tunnel project, it’s not without a mountain pass. Once the project is completed, we save 22km of travel. You are going to spend about Rs 2,500 crore for that. Using this money, we could move at least 10,000 people. That should be the priority of the government.
The tunnel road connects three districts and the people of these districts need this... what you are saying may be considered as environmental fundamentalism…
Sridhar: No. I’m questioning developmental fundamentalism. Environmental fundamentalism is not going to kill you. Everywhere, developmental fundamentalism is what’s killing you now.
Sajin: We do need better roads and other infrastructure that are necessary for human beings.
TNIE team: Cithara Paul, Anil S, K S Sreejith, Unnikrishnan S, Shainu Mohan
Follow The New Indian Express channel on WhatsApp
Download the TNIE app to stay with us and follow the latest
Related Stories
- Share full article
Advertisement
Supported by
What to Know About the Deadly Landslides in Southern India
The death toll has steadily risen in Kerala state after heavy rain sent huge mudslides through a scenic area known as a tourist destination.

By Anupreeta Das Pragati K.B. and Hari Kumar
Reporting from New Delhi
Earlier this week, multiple landslides hit the Wayanad district of India’s Kerala state, killing at least 144 people and injuring hundreds of others. The landslides were caused by torrential rains that lasted for days, uprooting trees, burying villages and cutting off roads and communication lines. State officials described it as one of the worst natural disasters Kerala has ever witnessed.
Rescue workers continue to recover and identify bodies, and with more than 190 people still missing, the death toll is likely to rise. The state government has set up temporary hospitals and dozens of shelters, which are housing more than 8,000 displaced people. It also sent rations and clean water to the area, and declared a two-day mourning period on Tuesday.
What led to the disaster?
Wayanad is a hilly region in the northeastern part of Kerala known for its natural beauty and wildlife. A big tourist destination, its slopes are covered by tea and spice plantations and its valleys contain rice paddies.
But the elevation, the steepness of the slopes, a thick bed of loose soil that sits atop hard rock and rivulets created by heavy rainfall create the perfect conditions for landslides, said S. Sreekumar, a geologist who has worked with government bodies on disaster management. New construction and irrigation methods used by farmers have also compromised the natural drainage system, he said.
“There are high slopes and people are settled at the base of the slope,” Mr. Sreekumar said. Extreme and more frequent rainfall owing to climate change are also “a big contributor, no doubt about that.”
The area experienced unusually heavy rainfall for 48 hours, much more than what the India Meteorological Department had forecast.
We are having trouble retrieving the article content.
Please enable JavaScript in your browser settings.
Thank you for your patience while we verify access. If you are in Reader mode please exit and log into your Times account, or subscribe for all of The Times.
Thank you for your patience while we verify access.
Already a subscriber? Log in .
Want all of The Times? Subscribe .
Information
- Author Services
Initiatives
You are accessing a machine-readable page. In order to be human-readable, please install an RSS reader.
All articles published by MDPI are made immediately available worldwide under an open access license. No special permission is required to reuse all or part of the article published by MDPI, including figures and tables. For articles published under an open access Creative Common CC BY license, any part of the article may be reused without permission provided that the original article is clearly cited. For more information, please refer to https://www.mdpi.com/openaccess .
Feature papers represent the most advanced research with significant potential for high impact in the field. A Feature Paper should be a substantial original Article that involves several techniques or approaches, provides an outlook for future research directions and describes possible research applications.
Feature papers are submitted upon individual invitation or recommendation by the scientific editors and must receive positive feedback from the reviewers.
Editor’s Choice articles are based on recommendations by the scientific editors of MDPI journals from around the world. Editors select a small number of articles recently published in the journal that they believe will be particularly interesting to readers, or important in the respective research area. The aim is to provide a snapshot of some of the most exciting work published in the various research areas of the journal.
Original Submission Date Received: .
- Active Journals
- Find a Journal
- Proceedings Series
- For Authors
- For Reviewers
- For Editors
- For Librarians
- For Publishers
- For Societies
- For Conference Organizers
- Open Access Policy
- Institutional Open Access Program
- Special Issues Guidelines
- Editorial Process
- Research and Publication Ethics
- Article Processing Charges
- Testimonials
- Preprints.org
- SciProfiles
- Encyclopedia
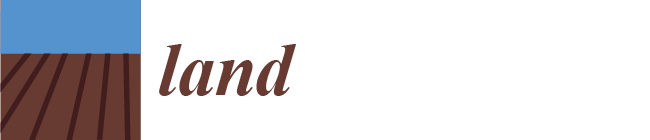
Article Menu
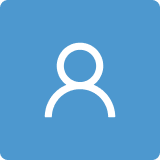
- Subscribe SciFeed
- Recommended Articles
- Google Scholar
- on Google Scholar
- Table of Contents
Find support for a specific problem in the support section of our website.
Please let us know what you think of our products and services.
Visit our dedicated information section to learn more about MDPI.
JSmol Viewer
Temporal and spatial variations in rainfall erosivity on hainan island and the influence of the el niño/southern oscillation.
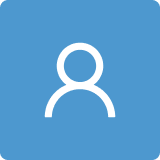
1. Introduction
2. materials and methods, 2.1. study area, 2.2.1. daily rainfall data, 2.2.2. enso indicators, 2.3. methods, 2.3.1. calculation of the re, 2.3.2. evaluation of the suitability of the re models, 2.3.3. spatial interpolation analysis method, 2.3.4. time-series change analysis method, 2.3.5. correlation analysis method, 3.1. evaluation of the suitability of the re calculation models, 3.2. change in re, 3.2.1. relationships among rainfall, erosive rainfall, and re, 3.2.2. spatiotemporal variation in re, 3.3. characteristics of the enso and its effect on re, 3.3.1. temporal distribution of different enso periods, 3.3.2. correlation between re and the enso, 3.3.3. effect of the enso on re, 4. discussion, 4.1. evaluation of the suitability of the re calculation model, 4.2. characteristics of variations in re, 4.3. re is affected by enso, 5. conclusions, supplementary materials, author contributions, data availability statement, acknowledgments, conflicts of interest.
- Bertol, I.; Schick, J.; Bandeira, D.H.; Paz-Ferreiro, J.; Vázquez, E.V. Multifractal and joint multifractal analysis of water and soil losses from erosion plots: A case study under subtropical conditions in Santa Catarina highlands, Brazil. Geodermia 2017 , 287 , 116–125. [ Google Scholar ] [ CrossRef ]
- Lai, C.; Chen, X.; Wang, Z.; Wu, X.; Zhao, S.; Wu, X.; Bai, W. Spatio-temporal variation in rainfall erosivity during 1960–2012 in the Pearl River Basin, China. Catena 2016 , 137 , 382–391. [ Google Scholar ] [ CrossRef ]
- Demissie, S.; Meshesha, D.T.; Adgo, E.; Haregeweyn, N.; Tsunekawa, A.; Ayana, M.; Mulualem, T.; Wubet, A. Effects of soil bund spacing on runoff, soil loss, and soil water content in the Lake Tana Basin of Ethiopia. Agric. Water Manag. 2022 , 274 , 107926. [ Google Scholar ] [ CrossRef ]
- Chen, S.; Zha, X. Effects of the ENSO on rainfall erosivity in the Fujian Province of southeast China. Sci. Total Environ. 2018 , 621 , 1378–1388. [ Google Scholar ] [ CrossRef ]
- Zhang, W.; Xie, Y.; Liu, B. Rainfall erosivity estimation using daily rainfall amounts. Sci. Geogr. Sin. 2002 , 22 , 705–711, (In Chinese with English Abstract). [ Google Scholar ]
- Shi, D.; Jiang, G.; Peng, X.; Jin, H.; Jiang, N. Relationship between the periodicity of soil and water loss and erosion-sensitive periods based on temporal distributions of rainfall erosivity in the Three Gorges Reservoir Region, China. Catena 2021 , 202 , 105268. [ Google Scholar ] [ CrossRef ]
- Liu, S.; Huang, S.; Xie, Y.; Leng, G.; Huang, Q.; Wang, L.; Xue, Q. Spatial-temporal changes of rainfall erosivity in the loess plateau, China: Changing patterns, causes and implications. Catena 2018 , 166 , 279–289. [ Google Scholar ] [ CrossRef ]
- Mahmood, A.; Alireza, O.; Mark, A.N. Expected climate change impacts on rainfall erosivity over Iran based on CMIP5 climate models. J. Hydrol. 2021 , 593 , 125826. [ Google Scholar ]
- Chang, Y.; Lei, H.; Zhou, F.; Yang, D. Spatial and temporal variations of rainfall erosivity in the middle Yellow River Basin based on hourly rainfall data. Catena 2022 , 216 , 106406. [ Google Scholar ] [ CrossRef ]
- Wischmeier, W.H.; Smith, D.D. Predicting rainfall erosion losses: A guide to conservation planning. In Agriculture Handbook ; Department of Agriculture: Washington, DC, USA, 1978. [ Google Scholar ]
- Chen, Y.; Xu, M.; Wang, Z.; Chen, W.; Lai, C. Reexamination of the Xie model and spatiotemporal variability in rainfall erosivity in mainland China from 1960 to 2018. Catena 2020 , 195 , 104837. [ Google Scholar ] [ CrossRef ]
- Panagos, P.; Ballabio, C.; Borrelli, P.; Meusburger, K.; Klik, A.; Rousseva, S.; Alewell, C. Rainfall erosivity in Europe. Sci. Total Environ. 2015 , 511 , 801–814. [ Google Scholar ] [ CrossRef ] [ PubMed ]
- Renard, K.G.; Freimund, J.R. Using monthly precipitation data to estimate the R factor in the revised USLE. J. Hydrol. 1994 , 157 , 287–306. [ Google Scholar ] [ CrossRef ]
- Ballabio, C.; Borrelli, P.; Spinoni, J.; Meusburger, K.; Michaelides, S.; Beguería, S.; Klik, A.; Petan, S.; Janeček, M.; Olsen, P.; et al. Mapping monthly rainfall erosivity in Europe. Sci. Total Environ. 2017 , 579 , 1279–1315. [ Google Scholar ] [ CrossRef ] [ PubMed ]
- Fu, B.; Zhao, W.; Chen, L.; Zhang, Q.; Lü, Y.; Gulinck, H.; Poesen, J. Assessment of soil erosion at large watershed scale using RUSLE and GIS: A case study in the Loess Plateau of China. Land Degrad. Dev. 2010 , 16 , 73–85. [ Google Scholar ] [ CrossRef ]
- Gu, Z.; Duan, X.; Liu, B.; Hu, J.; He, J. The spatial distribution and temporal variation of rainfall erosivity in the Yunnan Plateau, Southwest China:1960–2012. Catena 2016 , 145 , 291–300. [ Google Scholar ]
- Knisei, W.G. Creams: A Field Scale Model for Chemical, Runoff and Erosion from Agricultural Management Systems ; USDA Conservation Research Report 26(5); Department of Agriculture, Science and Education Administration: Corvallis, Oregon, 1980; pp. 133–138. [ Google Scholar ]
- Shi, Z.; Guo, G.; Zeng, Z.; Chen, J.; Wang, T.; Cai, C. Study on rainfall erosivity of the characteristics and daily rainfall erosivity model in Wuhan City. Soil Water Conserv. China 2006 , 1 , 22–24. (In Chinese) [ Google Scholar ]
- Xie, Y.; Liu, B.; Zhang, W. Study on standard of erosive rainfall. J. Soil Water Conserv. 2000 , 14 , 6–11, (In Chinese with English Abstract). [ Google Scholar ]
- Yu, B.; Rosewell, C.J. Rainfall erosivity estimation using daily rainfall amounts for South Australia. Aust. J. Soil Res. 1996 , 53 , 721–733. [ Google Scholar ] [ CrossRef ]
- Teng, H.; Rossel, A.V.R.; Shi, Z.; Behrens, T.; Chappell, A.; Bui, E. Assimilating satellite imagery and visible near infrared spectroscopy to model and map soil loss by water erosion in Australia. Environ. Model. Softw. 2016 , 77 , 156–167. [ Google Scholar ] [ CrossRef ]
- Lee, S.; Bae, J.H.; Hong, J.Y.; Yang, D.; Panagos, P.; Borrelli, P.; Yang, J.E.; Kim, J.; Lim, K.J. Estimation of rainfall erosivity factor in Italy and Switzerland using Bayesian optimization based machine learning models. Catena 2022 , 211 , 105957. [ Google Scholar ] [ CrossRef ]
- Diodato, N.; Borrelli, P.; Fiener, P.; Bellocchi, G.; Romano, N. Discovering historical rainfall erosivity with a parsimonious approach: A case study in Western Germany. J. Hydrol. 2017 , 544 , 1–9. [ Google Scholar ] [ CrossRef ]
- Johannsen, L.L.; Schmaltz, E.M.; Mitrovits, O.; Klik, A.; Smoliner, W.; Wang, S.; Strauss, P. An update of the spatial and temporal variability of rainfall erosivity (R-factor) for the main agricultural production zones of Austria. Catena 2022 , 215 , 106305. [ Google Scholar ] [ CrossRef ]
- WMO. El Niño/Southern Oscillation (WMO-No.1145) ; WMO: Geneva, Switzerland, 2014; pp. 2–4. [ Google Scholar ]
- Ortega, C.; Vargas, G.; Rojas, M.; Rutllant, J.A.; Muñoz, P.; Lange, C.B.; Pantoja, S.; Dezileau, L.; Ortlieb, L. Extreme ENSO-driven torrential rainfalls at the southern edge of the Atacama Desert during the Late Holocene and their projection into the 21th century. Glob. Planet. Change 2019 , 175 , 226–237. [ Google Scholar ] [ CrossRef ]
- Lee, J.H.; Julien, P.Y.; Lee, S.H. Rainfall erosivity variability over the United States associated with large-scale climate variations by El Niño/Southern Oscillation. Catena 2023 , 226 , 107050. [ Google Scholar ] [ CrossRef ]
- Sui, Y.; Xie, G. Projected relationship between ENSO and following-summer rainfall over the middle reaches of the Yangtze River valley based on CMIP6 simulations. Atmos. Ocean. Sci. Lett. 2023 , 11 , 100374. [ Google Scholar ] [ CrossRef ]
- Jiménez-Esteve, B.; Domeisen, D.I.V. Nonlinearity in the North Pacific Atmospheric Response to a Linear ENSO Forcing. Geophys. Res. Lett. 2019 , 46 , 2271–2281. [ Google Scholar ] [ CrossRef ]
- Zhang, Y.; Hao, Z.; Feng, S.; Zhang, X.; Hao, F. Changed relationship between compound dry-hot events and ENSO at the global scale. J. Hydrol. 2023 , 621 , 129559. [ Google Scholar ] [ CrossRef ]
- Rasmusson, E.M.; Carpenter, T.H. The relationship between eastern equatorial Pacific Sea Surface Temperatures and Rainfall over India and Sri Lanka. Mon. Weather Rev. 1983 , 111 , 517–528. [ Google Scholar ] [ CrossRef ]
- Westra, S.; Alexander, L.V.; Zwiers, F.W. Global increasing trends in annual maximum daily precipitation. J. Clim. 2013 , 26 , 3904–3918. [ Google Scholar ] [ CrossRef ]
- Krishnamurthy, L.; Vecchi, G.A.; Yang, X.; van der Wiel, K.; Balaji, V.; Kapnick, S.B.; Jia, L.; Zeng, F.; Paffendorf, K.; Underwood, S. Causes and probability of occurrence of extreme precipitation events like Chennai 2015. J. Clim. 2018 , 31 , 3831–3848. [ Google Scholar ] [ CrossRef ]
- Ma, F.; Ye, A.; You, J.; Duan, Q. 2015-16 floods and droughts in China, and its response to the strong El Niño. Sci. Total Environ. 2018 , 627 , 1473–1484. [ Google Scholar ] [ CrossRef ] [ PubMed ]
- Lavado-Casimiro, W.; Espinoza, J.C. Impactos de El Niño y La Niña en las lluvias del Perú (1965–2007). Rev. Bras. Meteorol. 2014 , 29 , 171–182. [ Google Scholar ] [ CrossRef ]
- Christine, T.Y.C.; Scott, B.P. The non-linear impact of El Niño, La Niña and the Southern Oscillation on seasonal and regional Australian precipitation. J. South. Hemisph. Earth Syst. Sci. 2017 , 67 , 25–45. [ Google Scholar ]
- Cai, W.; van Rensch, P.; Cowan, T.; Hendon, H.H. Teleconnection pathways of ENSO and the IOD and the mechanisms for impacts on Australian rainfall. J. Clim. 2011 , 24 , 3910–3923. [ Google Scholar ] [ CrossRef ]
- Lee, J.H.; Julien, P.Y. Teleconnections of the ENSO and South Korean precipitation patterns. J. Hydrol. 2016 , 534 , 237–250. [ Google Scholar ] [ CrossRef ]
- Wang, L.; Yang, Z.; Gu, X.; Li, J. Linkages between tropical cyclones and extreme precipitation over China and the role of ENSO. Int. J. Disaster Risk Sci. 2020 , 11 , 538–553. [ Google Scholar ] [ CrossRef ]
- Cao, Q.; Hao, Z.; Yuan, F.; Su, Z.; Berndtsson, R.; Hao, J.; Nyima, T. Impact of ENSO regimes on developing and decaying phase precipitation during rainy season in China. Hydrol. Earth Syst. Sci. 2017 , 21 , 5415–5426. [ Google Scholar ] [ CrossRef ]
- Xie, X.; Zhou, S.; Zhang, J.; Huang, P. The role of background SST changes in the ENSO-driven rainfall variability revealed from the atmospheric model experiments in CMIP5/6. Atmos. Res. 2021 , 261 , 105732. [ Google Scholar ] [ CrossRef ]
- Paula, G.M.; Streck, N.A.; Zanon, A.J.; Eltz, F.L.; Heldwein, A.B.; Ferraz, S.E. Effect of El Niño/Southern Oscillation on rainfall erosivity in Santa Maria (RS). Rev. Bras. Ciência Do Solo 2010 , 34 , 1315–1323. [ Google Scholar ] [ CrossRef ]
- Ma, L.; Huang, S.; Huang, Q.; Xue, Q.; Li, P.; Liu, S. Causes analyzing of the change of rainfall and rainfall erosivity in Wei River basin. J. Soil Water Conserv. 2018 , 32 , 74–189, (In Chinese with English Abstract). [ Google Scholar ]
- Zhu, D.; Xiong, K.; Xiao, H.; Gu, X. Variation characteristics of rainfall erosivity in Guizhou Province and the correlation with the El Niño Southern Oscillation. Sci. Total Environ. 2019 , 691 , 835–847. [ Google Scholar ] [ CrossRef ] [ PubMed ]
- Jiang, C.; Tian, Z.; Zhang, Y.; Keiko, U.D.O. Risk map of typhoon induced wave fields around Hainan Island. Appl. Ocean Res. 2023 , 137 , 103603. [ Google Scholar ] [ CrossRef ]
- Yin, C.; Huang, H.; Wang, D.; Liu, Y. Tropical cyclone-induced wave hazard assessment in Hainan Island, China. Nat. Hazards 2022 , 113 , 103–123. [ Google Scholar ] [ CrossRef ]
- Bai, T.; Xu, D.; Wu, S. Spatial and temporal changes of ecosystem services and driving forces: A case study of Hainan Island. China Environ. Sci. 2023 , 43 , 5961–5973, (In Chinese with English Abstract). [ Google Scholar ]
- Geng, J.; Xu, D.; Wu, Y.; Ren, B.; Yang, F. Spatio-temporal evolution of eco-environment quality and the response to climate change and human activities in Hainan Island. Acta Ecol. Sin. 2022 , 42 , 4795–4806, (In Chinese with English Abstract). [ Google Scholar ]
- Wang, G.; Cheng, H.; Zhang, Y.; Yu, H. ENSO analysis and prediction using deep learning: A review. Neurocomputing 2023 , 520 , 216–229. [ Google Scholar ] [ CrossRef ]
- Pompa-García, M.; Némiga, X.A. ENSO index teleconnection with seasonal precipitation in a temperate ecosystem of northern Mexico. Atmósfera 2015 , 28 , 43–50. [ Google Scholar ] [ CrossRef ]
- Espinoza, C.V.; Hernández-Miranda, E.; Gilabert, H.; Ojeda, F.P. Temporal and spatial dissimilarities in an intertidal fish assemblage in the South Pacific Ocean: The role of the ENSO process and intrinsic habitat conditions in emerging patterns. Sci. Total Environ. 2023 , 872 , 162220. [ Google Scholar ] [ CrossRef ] [ PubMed ]
- Zhou, P.; Liu, Z.; Cheng, L. An alternative approach for quantitatively estimating climate variability over China under the effects of ENSO events. Atmos. Res. 2020 , 238 , 104897. [ Google Scholar ] [ CrossRef ]
- Lu, S.; Chen, Y.; Duan, X.; Yin, S. Rainfall erosivity estimation models for the Tibetan Plateau. Catena 2023 , 229 , 1071186. [ Google Scholar ] [ CrossRef ]
- Rutebuka, J.; de Taeye, S.; Kagabo, D.; Verdoodt, A. Calibration and validation of rainfall erosivity estimators for application in Rwanda. Catena 2020 , 190 , 104538. [ Google Scholar ] [ CrossRef ]
- Chen, Z.; Shi, D.; He, W.; Xia, J.; Jin, H.; Lou, Y. Spatial-temporal Distribution and Trend of Rainfall Erosivity in Yunnan Province. Trans. Chin. Soc. Agric. Eng. 2017 , 48 , 209–219, (In Chinese with English Abstract). [ Google Scholar ]
- Alves, G.J.; Mello, C.R.; Guo, L.; Thebaldi, M.S. Natural disaster in the mountainous region of Rio de Janeiro state, Brazil: Assessment of the daily rainfall erosivity as an early warning index. Int. Soil Water Conserv. Res. 2022 , 10 , 547–556. [ Google Scholar ] [ CrossRef ]
- Richardson, C.W.; Foster, G.R.; Wright, D.A. Estimation of erosion index from daily rainfall amount. Trans. ASAE 1983 , 26 , 153–156. [ Google Scholar ] [ CrossRef ]
- Cui, T.; Li, Y.; Yang, L.; Nan, Y.; Li, K.; Tudaji, M.; Hu, H.; Long, D.; Shahid, M.; Mubeen, A.; et al. Non-monotonic changes in Asian Water Towers’ streamflow at increasing warming levels. Nat. Commun. 2023 , 4 , 1176. [ Google Scholar ] [ CrossRef ] [ PubMed ]
- Coşkun, Ö.; Citakoglu, H. Prediction of the standardized precipitation index based on the long short-term memory and empirical mode decomposition-extreme learning machine models: The Case of Sakarya, Türkiye. Phys. Chem. Earth Parts A/B/C 2023 , 131 , 103418. [ Google Scholar ] [ CrossRef ]
- Kyaw, A.K.; Hamed, M.M.; Shahid, S. Spatiotemporal changes in Universal Thermal Climate Index over South Asia. Atmos. Res. 2023 , 292 , 106838. [ Google Scholar ] [ CrossRef ]
- Ahmadi, A.; Daccache, A.; Snyder, R.L.; Suvočarev, K. Meteorological driving forces of reference evapotranspiration and their trends in California. Sci. Total Environ. 2022 , 849 , 157823. [ Google Scholar ] [ CrossRef ] [ PubMed ]
- Alashan, S. Combination of Modified Mann-Kendall Method and Sen Innovative Trend Analysis. Eng. Rep. 2020 , 2 , 12131. [ Google Scholar ] [ CrossRef ]
- Nkunzimana, A.; Bi, S.; Wang, G.; Alriah, M.A.A.; Sarfo, I.; Xu, Z.; Vuguziga, F.; Ayugi, B.O. Assessment of drought events, their trend and teleconnection factors over Burundi, East Africa. Theor. Appl. Climatol. 2021 , 145 , 1293–1316. [ Google Scholar ] [ CrossRef ]
- Zhang, J.; Sun, F.; Xu, J.; Chen, Y.; Sang, Y.; Liu, C. Dependence of trends in and sensitivity of drought over China (1961–2013) on potential evaporation model. Geophys. Res. Lett. 2016 , 43 , 206–213. [ Google Scholar ] [ CrossRef ]
- Yu, H.; Lin, Y. Analysis of space-time non-stationary patterns of rainfall-groundwater interactions by integrating empirical orthogonal function and cross wavelet transform methods. J. Hydrol. 2015 , 525 , 585–597. [ Google Scholar ] [ CrossRef ]
- Hu, W.; Si, B.C.; Biswas, A.; Chau, H.W. Temporally stable patterns but seasonal dependent controls of soil water content: Evidence from wavelet analyses. Hydrol. Progresses 2017 , 31 , 3697–3707. [ Google Scholar ] [ CrossRef ]
- Su, L.; Miao, C.; Duan, Q.; Lei, X.; Li, H. Multiple-wavelet coherence of world’s large rivers with meteorological factors and ocean signals. J. Geophys. Res.-Atmos. 2019 , 124 , 4932–4954. [ Google Scholar ] [ CrossRef ]
- Lee, J.H.; Julien, P.Y.; Lee, S.H. Teleconnection of ENSO extreme events and precipitation variability over the United States. J. Hydrol. 2023 , 619 , 129206. [ Google Scholar ]
- Olorunfemi, I.E.; Komolafe, A.A.; Fasinmirin, J.T.; Olufayo, A.A.; Akande, S.O. A GIS-based assessment of the potential soil erosion and flood hazard zones in Ekiti State, Southwestern Nigeria using integrated RUSLE and HAND models. Catena 2020 , 194 , 104725. [ Google Scholar ] [ CrossRef ]
- Grinsted, A.; Moore, J.; Jevrejeva, S. Application of the cross wavelet transform and wavelet coherence to geophysical time series. Nonlinear Process. Geophys. 2004 , 11 , 561–566. [ Google Scholar ] [ CrossRef ]
- Thomas, E.; Abraham, N.P. Relationship between sunspot number and seasonal rainfall over Kerala using wavelet analysis. J. Atmos. Sol.-Terr. Phys. 2022 , 240 , 105943. [ Google Scholar ] [ CrossRef ]
- Yang, W.; Jin, F.; Si, Y.; Li, Z. Runoff change controlled by combined effects of multiple environmental factors in a headwater catchment with cold and arid climate in northwest China. Sci. Total Environ. 2020 , 756 , 143995. [ Google Scholar ] [ CrossRef ] [ PubMed ]
- De Sousa Teixeira, D.B.; Cecílio, R.A.; Moreira, M.C.; Pires, G.F.; Fernandes Filho, E.I. Assessment, regionalization, and modeling rainfall erosivity over Brazil: Findings from a large national database. Sci. Total Environ. 2023 , 891 , 164557. [ Google Scholar ] [ CrossRef ] [ PubMed ]
- Lu, X. Simulation of Rainfall Erosivity in Purple Soil Hilly Region ; Southwest University: Chongqing, China, 2006. (In Chinese) [ Google Scholar ]
- Xu, X.; Yan, Y.; Dai, Q.; Yi, X.; Hu, Z.; Cen, L. Spatial and temporal dynamics of rainfall erosivity in the karst region of southwest China: Interannual and seasonal changes. Catena 2023 , 221 , 106763. [ Google Scholar ] [ CrossRef ]
- Zhang, J.; Ren, Y.; Jiao, P.; Xiao, P.; Li, Z. Changes in rainfall erosivity from combined effects of multiple factors in China’s Loess Plateau. Catena 2022 , 216 , 106373. [ Google Scholar ] [ CrossRef ]
- Wei, C.; Dong, X.; Yu, D.; Zhang, T.; Zhao, W.; Ma, Y.; Su, B. Spatio-temporal variations of rainfall erosivity, correlation of climatic indices and influence on human activities in the Huaihe River Basin, China. Catena 2022 , 217 , 106486. [ Google Scholar ] [ CrossRef ]
- Li, D.; Qi, Y.; Zhou, T. Changes in rainfall erosivity over mainland China under stabilized 1.5 °C and 2 °C warming futures. J. Hydrol. 2021 , 603 , 126996. [ Google Scholar ] [ CrossRef ]
- Huang, J.; Zhang, J.; Zhang, Z.; Xu, C. Spatial and temporal variations in rainfall erosivity during 1960–2005 in the Yangtze River basin. Stoch. Environ. Risk Assess. 2013 , 27 , 337–351. [ Google Scholar ] [ CrossRef ]
- Zhang, R. Changes in East Asian summer monsoon and summer rainfall over eastern China during recent decades. Sci. Bull. 2015 , 60 , 1222–1224. [ Google Scholar ] [ CrossRef ]
- Chen, L.; Chen, Y.; Zhang, Y.; Xu, S. Spatial patterns of typhoon rainfall and associated flood characteristics over a mountainous watershed of a tropical island. J. Hydrol. 2022 , 613 Pt A , 128421. [ Google Scholar ] [ CrossRef ]
- Zhang, Y.; Qin, X.; Qiu, Q.; Yu, R.; Yao, Y.; Li, H.; Shao, M.; Wei, X. Soil and water conservation measures reduce erosion but result in carbon and nitrogen accumulation of red soil in Southern China. Agric. Ecosyst. Environ. 2023 , 346 , 108346. [ Google Scholar ] [ CrossRef ]
- Trenberth, K.E. El Niño/Southern Oscillation (ENSO). Encycl. Ocean Sci. (Third Ed.) 2019 , 6 , 420–432. [ Google Scholar ]
- Worako, A.W.; Haile, A.T.; Taye, M.T. Streamflow variability and its linkage to ENSO events in the Ethiopian Rift Valley Lakes Basin. J. Hydrol. Reg. Stud. 2021 , 35 , 100817. [ Google Scholar ] [ CrossRef ]
- Xu, Z.; Pan, B.; Han, M. Spatial-temporal distribution of rainfall erosivity, erosivity density and correlation with El Niño-Southern Oscillation in the Huaihe River Basin China. Ecol. Inform. 2019 , 52 , 14–25. [ Google Scholar ] [ CrossRef ]
Click here to enlarge figure
Parameters | Calculation Models of RE | ||||
---|---|---|---|---|---|
Model 1 | Model 2 | Model 3 | Model 4 | Model 5 | |
Annual average RE (MJ·mm·ha ·h ) | 18,021.10 | 17,354.09 | 1580.76 | 12,664.37 | 16,735.37 |
Standard deviation (mm) | 4498.62 | 4320.15 | 3025.89 | 2731.11 | 3562.90 |
Coefficient of variation (C ) | 0.52 | 0.55 | 0.27 | 0.30 | 0.43 |
Effectiveness coefficient (E ) | 0.24 | 0.36 | 0.81 | −0.15 | −0.14 |
Relative deviation coefficient (E ) | 0.26 | 0.41 | 0.08 | 0.60 | 0.35 |
No. | Station | R with ER | R with RE | ER with RE |
---|---|---|---|---|
1 | Danxian | 0.98 *** | 0.87 *** | 0.91 *** |
2 | Mutang | 0.93 *** | 0.80 *** | 0.87 *** |
3 | Lingao | 0.98 *** | 0.88 *** | 0.92 *** |
4 | Yubao | 0.94 *** | 0.83 *** | 0.90 *** |
5 | Fucai | 0.94 *** | 0.84 *** | 0.89 *** |
6 | Dafeng | 0.96 *** | 0.85 *** | 0.90 *** |
7 | Dunya | 0.97 *** | 0.86 *** | 0.92 *** |
8 | Meiting | 0.93 *** | 0.82 *** | 0.85 *** |
9 | Jialetan | 0.95 *** | 0.83 *** | 0.89 *** |
10 | Yongfeng | 0.92 *** | 0.79 *** | 0.82 *** |
11 | Dabao | 0.92 *** | 0.80 *** | 0.84 *** |
12 | Haikou | 0.98 *** | 0.86 *** | 0.93 *** |
13 | Dongpo | 0.95 *** | 0.87 *** | 0.90 *** |
14 | Wengtian | 0.93 *** | 0.84 *** | 0.91 *** |
15 | Qinglan | 0.98 *** | 0.88 *** | 0.92 *** |
16 | Heshui | 0.93 *** | 0.80 *** | 0.82 *** |
17 | Shenwang | 0.95 *** | 0.81 *** | 0.88 *** |
18 | Heluo | 0.91 *** | 0.77 *** | 0.80 *** |
19 | Wupo | 0.94 *** | 0.83 *** | 0.84 *** |
20 | Zhongpingzai | 0.96 *** | 0.85 *** | 0.91 *** |
21 | Gangbei | 0.96 *** | 0.82 *** | 0.92 *** |
22 | Nanqiao | 0.93 *** | 0.80 *** | 0.85 *** |
23 | Lingshui | 0.93 *** | 0.79 *** | 0.83 *** |
24 | Jinjiang | 0.97 *** | 0.89 *** | 0.92 *** |
25 | Songhe | 0.98 *** | 0.87 *** | 0.92 *** |
26 | Nanbing | 0.93 *** | 0.80 *** | 0.83 *** |
27 | Yinggehai | 0.96 *** | 0.85 *** | 0.84 *** |
28 | Ganen | 0.96 *** | 0.82 *** | 0.83 *** |
29 | Datian | 0.94 *** | 0.83 *** | 0.85 *** |
30 | Gongguan | 0.93 *** | 0.79 *** | 0.81 *** |
31 | Lezhong | 0.96 *** | 0.85 *** | 0.87 *** |
32 | Baoxian | 0.93 *** | 0.78 *** | 0.85 *** |
33 | Baojie | 0.94 *** | 0.83 *** | 0.82 *** |
34 | Sanpai | 0.97 *** | 0.86 *** | 0.93 *** |
35 | Shilu | 0.93 *** | 0.81 *** | 0.85 *** |
36 | Changhua | 0.92 *** | 0.78 *** | 0.86 *** |
37 | Haitougang | 0.95 *** | 0.82 *** | 0.87 *** |
38 | Yaxing | 0.96 *** | 0.85 *** | 0.90 *** |
Mean | - | 0.95 | 0.83 | 0.87 |
No. | Station | Rainfall (mm) | Erosive Rainfall (mm) | RE (MJ·mm·ha ·h ) |
---|---|---|---|---|
1 | Danxian | 4.43 * | 4.86 * | 50.78 * |
2 | Mutang | 7.39 | −7.30 | −200.14 |
3 | Lingao | 4.77 | 5.06 | 92.39 |
4 | Yubao | 11.48 * | 10.00 * | 64.10 |
5 | Fucai | 1.73 * | −1.78 | −35.72 |
6 | Dafeng | −5.80 | −5.63 | −67.39 |
7 | Dunya | 5.09 | 5.57 | 67.25 |
8 | Meiting | 2.82 | 3.02 | 49.11 |
9 | Jialetan | −0.17 | 0.35 | 7.20 * |
10 | Yongfeng | 0.57 | −0.43 | 25.27 |
11 | Dabao | 2.62 | 2.01 | 65.34 |
12 | Haikou | 0.88 | 1.54 | 46.43 |
13 | Dongpo | 4.21 | 3.07 | 16.60 |
14 | Wengtian | −5.22 | −5.21 | −47.53 |
15 | Qinglan | −3.41 | −3.11 | −53.52 |
16 | Heshui | 3.04 * | 2.31 * | 38.50 * |
17 | Shenwang | 4.43 ** | 3.16 ** | 31.14 ** |
18 | Heluo | −0.46 | 0.42 * | 3.84 ** |
19 | Wupo | 5.03 | 4.02 | 55.06 |
20 | Zhongpingzai | 0.79 ** | 0.80 ** | −30.94 ** |
21 | Gangbei | 5.57 * | 4.16 * | 28.21 * |
22 | Nanqiao | 12.79 ** | 13.43 ** | 187.26 *** |
23 | Lingshui | 2.56 | 2.89 ** | 24.88 * |
24 | Jinjiang | 2.59 | 4.58 * | 58.56 |
25 | Songhe | 7.53 | 8.22 | 126.61 |
26 | Nanbing | −0.54 | 0.80 | −10.22 |
27 | YingGeHai | 2.38 | 2.61 | −4.15 |
28 | Ganen | −4.33 * | −4.38 * | −49.46 * |
29 | Datian | 7.02 | −7.20 | 100.82 |
30 | Gongguan | 0.28 * | 0.55 * | 24.74 * |
31 | Lezhong | 4.47 | 4.85 | 30.15 |
32 | Baoxian | 6.43 | 5.44 | 43.59 |
33 | Baojie | −3.67 * | −3.91 | −32.88 |
34 | Sanpai | 2.14 | 2.97 | 46.16 |
35 | Shilu | −1.07 | −1.45 | −23.82 |
36 | Changhua | −3.24 * | −2.35 * | −63.16 * |
37 | Haitougang | −1.00 | −1.08 * | −7.88 * |
38 | Yaxing | 0.85 | 1.61 | 29.86 |
No. | Climate Events | Time Interval | Duration in Months | Average Monthly RE (MJ·mm·ha ·h ) |
---|---|---|---|---|
1 | La Niña | 1971.01–1972.01 | 13 | 1238.59 |
2 | El Niño | 1972.05–1973.03 | 11 | 1828.15 |
3 | La Niña | 1973.05–1974.07 | 15 | 1566.05 |
4 | La Niña | 1974.10–1976.03 | 18 | 1025.16 |
5 | El Niño | 1976.09–1977.02 | 6 | 2033.39 |
6 | El Niño | 1977.09–1978.01 | 5 | 970.70 |
7 | El Niño | 1982.05–1983.06 | 14 | 1173.38 |
8 | La Niña | 1984.10–1985.06 | 9 | 580.76 |
9 | El Niño | 1986.09–1988.02 | 18 | 820.94 |
10 | La Niña | 1988.05–1989.05 | 13 | 1126.87 |
11 | El Niño | 1991.06–1992.06 | 13 | 1384.28 |
12 | El Niño | 1994.09–1995.03 | 7 | 941.24 |
13 | La Niña | 1995.08–1996.03 | 8 | 1528.90 |
14 | El Niño | 1997.05–1998.04 | 12 | 1095.89 |
15 | La Niña | 1998.07–2001.02 | 32 | 1310.41 |
16 | El Niño | 2002.06–2003.02 | 9 | 1432.35 |
17 | El Niño | 2004.08–2005.02 | 7 | 2334.50 |
18 | La Niña | 2005.11–2006.03 | 5 | 298.74 |
19 | El Niño | 2006.09–2007.01 | 5 | 1305.12 |
20 | La Niña | 2007.07–2008.06 | 12 | 1126.46 |
21 | La Niña | 2008.11–2009.03 | 5 | 490.14 |
22 | El Niño | 2009.08–2010.03 | 8 | 1849.70 |
23 | La Niña | 2010.06–2011.05 | 12 | 1489.59 |
24 | La Niña | 2011.08–2012.03 | 8 | 1666.49 |
25 | El Niño | 2015.03–2016.04 | 14 | 1038.44 |
26 | La Niña | 2016.08–2016.12 | 5 | 1469.98 |
27 | La Niña | 2017.10–2018.04 | 7 | 939.39 |
28 | El Niño | 2018.10–2019.05 | 8 | 1298.30 |
29 | La Niña | 2020.08–2020.12 | 5 | 1922.96 |
Mean RE in El Niño | 1393.31 | |||
Mean RE in La Niña | 1185.37 | |||
Mean RE in ENSO | 1285.75 | |||
Mean RE in Neutral period | 1349.67 | |||
Mean RE during the period 1971–2020 | 1306.02 |
Analytical Method | Characteristic Indices of ENSO | AWC | PASC (%) |
---|---|---|---|
WTC | MEI | 0.85 | 18.6 |
SOI | 0.79 | 5.9 | |
ONI | 0.83 | 9.1 | |
MWC | MEI-SOI | 0.94 | 3.6 |
MEI-ONI | 0.95 | 19.0 | |
SOI-ONI | 0.92 | 5.7 | |
MEI-SOI-ONI | 0.97 | 4.9 |
The statements, opinions and data contained in all publications are solely those of the individual author(s) and contributor(s) and not of MDPI and/or the editor(s). MDPI and/or the editor(s) disclaim responsibility for any injury to people or property resulting from any ideas, methods, instructions or products referred to in the content. |
Share and Cite
Lu, X.; Chen, J.; Guo, J.; Qi, S.; Liao, R.; Lai, J.; Wang, M.; Zhang, P. Temporal and Spatial Variations in Rainfall Erosivity on Hainan Island and the Influence of the El Niño/Southern Oscillation. Land 2024 , 13 , 1210. https://doi.org/10.3390/land13081210
Lu X, Chen J, Guo J, Qi S, Liao R, Lai J, Wang M, Zhang P. Temporal and Spatial Variations in Rainfall Erosivity on Hainan Island and the Influence of the El Niño/Southern Oscillation. Land . 2024; 13(8):1210. https://doi.org/10.3390/land13081210
Lu, Xudong, Jiadong Chen, Jianchao Guo, Shi Qi, Ruien Liao, Jinlin Lai, Maoyuan Wang, and Peng Zhang. 2024. "Temporal and Spatial Variations in Rainfall Erosivity on Hainan Island and the Influence of the El Niño/Southern Oscillation" Land 13, no. 8: 1210. https://doi.org/10.3390/land13081210
Article Metrics
Article access statistics, supplementary material.
ZIP-Document (ZIP, 128 KiB)
Further Information
Mdpi initiatives, follow mdpi.
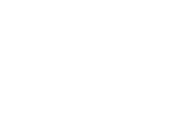
Subscribe to receive issue release notifications and newsletters from MDPI journals
- 0 Shopping Cart
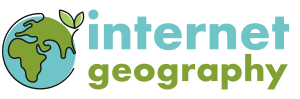
Kerala flood case study
Kerala flood case study.
Kerala is a state on the southwestern Malabar Coast of India. The state has the 13th largest population in India. Kerala, which lies in the tropical region, is mainly subject to the humid tropical wet climate experienced by most of Earth’s rainforests.
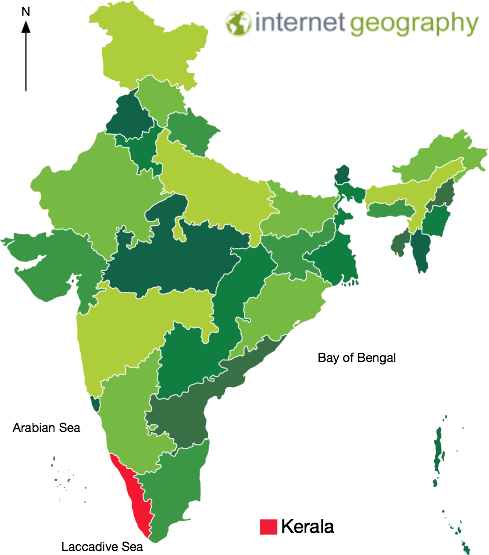
A map to show the location of Kerala
Eastern Kerala consists of land infringed upon by the Western Ghats (western mountain range); the region includes high mountains, gorges, and deep-cut valleys. The wildest lands are covered with dense forests, while other areas lie under tea and coffee plantations or other forms of cultivation.
The Indian state of Kerala receives some of India’s highest rainfall during the monsoon season. However, in 2018 the state experienced its highest level of monsoon rainfall in decades. According to the India Meteorological Department (IMD), there was 2346.3 mm of precipitation, instead of the average 1649.55 mm.
Kerala received over two and a half times more rainfall than August’s average. Between August 1 and 19, the state received 758.6 mm of precipitation, compared to the average of 287.6 mm, or 164% more. This was 42% more than during the entire monsoon season.
The unprecedented rainfall was caused by a spell of low pressure over the region. As a result, there was a perfect confluence of the south-west monsoon wind system and the two low-pressure systems formed over the Bay of Bengal and Odisha. The low-pressure regions pull in the moist south-west monsoon winds, increasing their speed, as they then hit the Western Ghats, travel skywards, and form rain-bearing clouds.
Further downpours on already saturated land led to more surface run-off causing landslides and widespread flooding.
Kerala has 41 rivers flowing into the Arabian Sea, and 80 of its dams were opened after being overwhelmed. As a result, water treatment plants were submerged, and motors were damaged.
In some areas, floodwater was between 3-4.5m deep. Floods in the southern Indian state of Kerala have killed more than 410 people since June 2018 in what local officials said was the worst flooding in 100 years. Many of those who died had been crushed under debris caused by landslides. More than 1 million people were left homeless in the 3,200 emergency relief camps set up in the area.
Parts of Kerala’s commercial capital, Cochin, were underwater, snarling up roads and leaving railways across the state impassable. In addition, the state’s airport, which domestic and overseas tourists use, was closed, causing significant disruption.
Local plantations were inundated by water, endangering the local rubber, tea, coffee and spice industries.
Schools in all 14 districts of Kerala were closed, and some districts have banned tourists because of safety concerns.
Maintaining sanitation and preventing disease in relief camps housing more than 800,000 people was a significant challenge. Authorities also had to restore regular clean drinking water and electricity supplies to the state’s 33 million residents.
Officials have estimated more than 83,000km of roads will need to be repaired and that the total recovery cost will be between £2.2bn and $2.7bn.
Indians from different parts of the country used social media to help people stranded in the flood-hit southern state of Kerala. Hundreds took to social media platforms to coordinate search, rescue and food distribution efforts and reach out to people who needed help. Social media was also used to support fundraising for those affected by the flooding. Several Bollywood stars supported this.
Some Indians have opened up their homes for people from Kerala who were stranded in other cities because of the floods.
Thousands of troops were deployed to rescue those caught up in the flooding. Army, navy and air force personnel were deployed to help those stranded in remote and hilly areas. Dozens of helicopters dropped tonnes of food, medicine and water over areas cut off by damaged roads and bridges. Helicopters were also involved in airlifting people marooned by the flooding to safety.
More than 300 boats were involved in rescue attempts. The state government said each boat would get 3,000 rupees (£34) for each day of their work and that authorities would pay for any damage to the vessels.
As the monsoon rains began to ease, efforts increased to get relief supplies to isolated areas along with clean up operations where water levels were falling.
Millions of dollars in donations have poured into Kerala from the rest of India and abroad in recent days. Other state governments have promised more than $50m, while ministers and company chiefs have publicly vowed to give a month’s salary.
Even supreme court judges have donated $360 each, while the British-based Sikh group Khalsa Aid International has set up its own relief camp in Kochi, Kerala’s main city, to provide meals for 3,000 people a day.
International Response
In the wake of the disaster, the UAE, Qatar and the Maldives came forward with offers of financial aid amounting to nearly £82m. The United Arab Emirates promised $100m (£77m) of this aid. This is because of the close relationship between Kerala and the UAE. There are a large number of migrants from Kerala working in the UAE. The amount was more than the $97m promised by India’s central government. However, as it has done since 2004, India declined to accept aid donations. The main reason for this is to protect its image as a newly industrialised country; it does not need to rely on other countries for financial help.
Google provided a donation platform to allow donors to make donations securely. Google partners with the Center for Disaster Philanthropy (CDP), an intermediary organisation that specialises in distributing your donations to local nonprofits that work in the affected region to ensure funds reach those who need them the most.
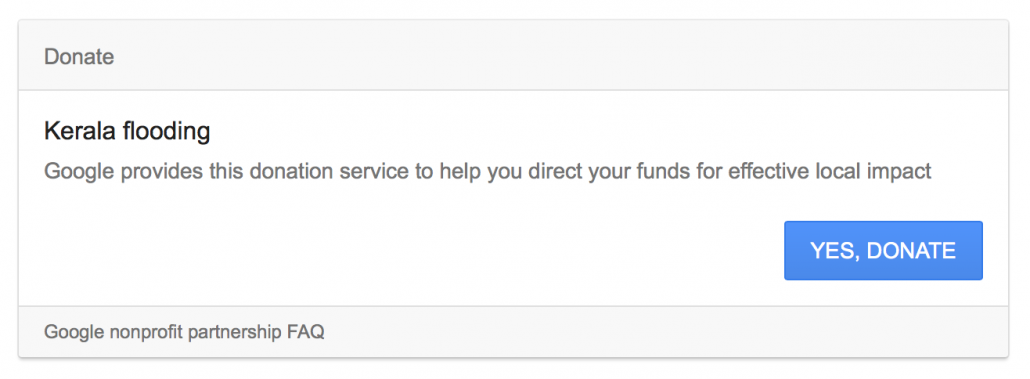
Google Kerala Donate
Tales of humanity and hope
Check your understanding.
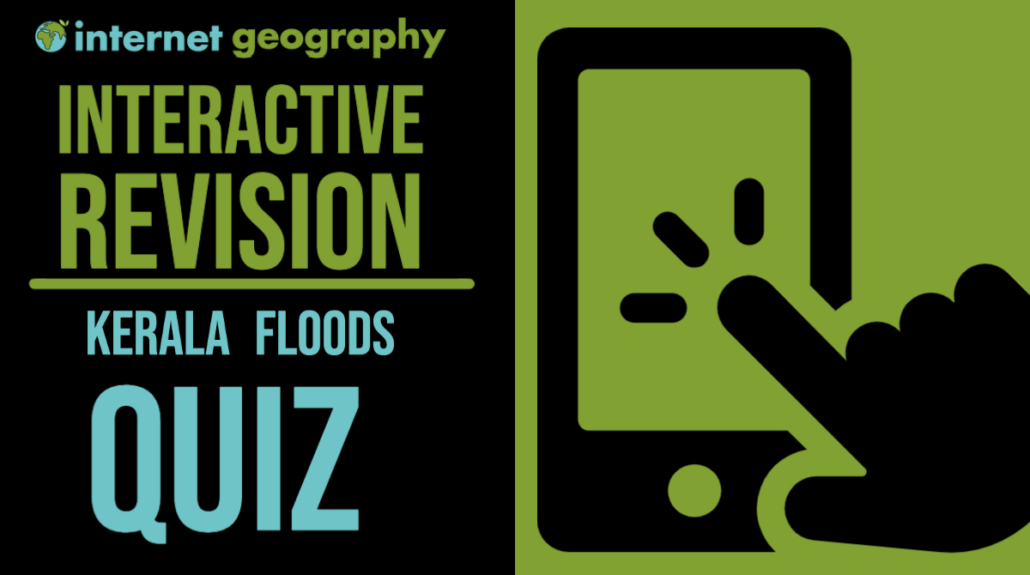
Premium Resources
Please support internet geography.
If you've found the resources on this page useful please consider making a secure donation via PayPal to support the development of the site. The site is self-funded and your support is really appreciated.
Related Topics
Use the images below to explore related GeoTopics.
River flooding and management
Topic home, wainfleet floods case study, share this:.
- Click to share on Twitter (Opens in new window)
- Click to share on Facebook (Opens in new window)
- Click to share on Pinterest (Opens in new window)
- Click to email a link to a friend (Opens in new window)
- Click to share on WhatsApp (Opens in new window)
- Click to print (Opens in new window)
If you've found the resources on this site useful please consider making a secure donation via PayPal to support the development of the site. The site is self-funded and your support is really appreciated.
Search Internet Geography
Top posts & pages.

Latest Blog Entries

Pin It on Pinterest
- Click to share
- Print Friendly

SAR based flood risk analysis: a case study Kerala Flood 2018
- Advances in Space Research 69(3)

- Jadavpur University

- Kazimierz Wielki University in Bydgoszcz
- This person is not on ResearchGate, or hasn't claimed this research yet.
Abstract and Figures

Discover the world's research
- 25+ million members
- 160+ million publication pages
- 2.3+ billion citations
- REMOTE SENS ENVIRON
- Sarath Suresh

- Sanchit Minocha
- B S Pavithra

- Abhishek A Pathak
- NAT HAZARDS

- Jinfei Wang

- Amar Siddiq

- Mehmet Demirci

- Biosci., Biotechnol. Res. Asia
- Jithesh Krishnan R
- Fouzia Hilal
- Abhilash Sivadasan
- Jalaja Vidya

- Bharat Jhamnani
- ENVIRON MONIT ASSESS

- Vipin T. Raj

- CURR SCI INDIA

- C.L. Vishnu

- Thomas Oommen

- P. Prajeesh

- Recruit researchers
- Join for free
- Login Email Tip: Most researchers use their institutional email address as their ResearchGate login Password Forgot password? Keep me logged in Log in or Continue with Google Welcome back! Please log in. Email · Hint Tip: Most researchers use their institutional email address as their ResearchGate login Password Forgot password? Keep me logged in Log in or Continue with Google No account? Sign up
Content Search
Study report: kerala floods of august 2018 (september, 2018).
- Govt. India
Attachments

1.0 Introduction
Kerala State has an average annual precipitation of about 3000 mm. The rainfall in the State is controlled by the South-west and North-east monsoons. About 90% of the rainfall occurs during six monsoon months. The high intensity storms prevailing during the monsoon months result in heavy discharges in all the rivers. The continuous and heavy precipitation that occurs in the steep and undulating terrain finds its way into the main rivers through innumerable streams and water courses.
Kerala experienced an abnormally high rainfall from 1 June 2018 to 19 August 2018. This resulted in severe flooding in 13 out of 14 districts in the State. As per IMD data, Kerala received 2346.6 mm of rainfall from 1 June 2018 to 19 August 2018 in contrast to an expected 1649.5 mm of rainfall. This rainfall was about 42% above the normal. Further, the rainfall over Kerala during June, July and 1st to 19th of August was 15%, 18% and 164% respectively, above normal. Month-wise rainfall for the period, as reported by IMD, are given in Table-1.
Due to heavy rainfall, the first onset of flooding occurred towards the end of July. A severe spell of rainfall was experienced at several places on the 8th and 9th of August 2018. The 1- day rainfall of 398 mm, 305 mm, 255 mm, 254 mm, 211 mm and 214 mm were recorded at Nilambur in Malappuram district, Mananthavadi in Wayanad district, Peermade, Munnar KSEB and Myladumparain in Idukki district and Pallakad in Pallakad district respectively on 9 August 2018. This led to further flooding at several places in Mananthavadi and Vythiri in Wayanad district during 8-10, August 2018. Water was released from several dams due to heavy rainfall in their catchments. The water levels in several reservoirs were almost near their Full Reservoir Level (FRL) due to continuous rainfall from 1st of June. Another severe spell of rainfall started from the 14th of August and continued till the 19th of August, resulting in disastrous flooding in 13 out of 14 districts. The water level records at CWC G&D sites for some of the rivers in Kerala are given at Annex-I. As per the rainfall records of IMD, it has been found that the rainfall depths recorded during the 15-17, August 2018 were comparable to the severe storm that occurred in the year 1924.
1.1 Earlier floods in Kerala
The 1924 witnessed unprecedented and very heavy floods in almost all rivers of Kerala. Heavy losses to life, property and crops etc. had been reported. The rainstorm of 16-18, July 1924 was caused by the South-west monsoon that extended to the south of peninsula on 15th July and caused rainfall in Malabar. Under its influence, heavy rainfall occurred in almost entire Kerala. The area under the storm recorded 1-day maximum rainfall on 17th of July, 2- day maximum rainfall for 16-17, July 1924 and 3-day maximum rainfall for 16-18, July 1924. The centre of the 1-day and 2-day rainstorm was located at Devikulam in Kerala which recorded 484 mm and 751 mm of rainfall respectively. The centre of 3-day rainstorm was located at Munnar in Kerala which recorded a rainfall of 897 mm in 3 days.
The fury of 1924 flood levels in most of the rivers was still fresh in the memory of people of Kerala, the year 1961 also witnessed heavy floods and rise in the water levels of reservoirs. Usually in the State, heavy precipitation is concentrated over a period of 7 to 10 days during the monsoon when the rivers rise above their established banks and inundate the low lying areas. But in 1961, floods were unusually heavy not only in duration, but also in the intensity of precipitation. During the year 1961, the monsoon started getting violent towards the last week of June and in the early days of August, the precipitation was concentrated on most parts of the southern region of Kerala. By the first week of July, the intensity gradually spread over the other parts of the State and the entire State was reeling under severe flood by the second week of July. The worst affected area was Periyar sub-basin and it also impacted other sub-basins. Many of the important infrastructures like highways etc were submerged. After a brief interval, by the middle of July, the monsoon became more violent, affecting the northern parts of the State. The average rainfall was 56% above normal. The maximum daily intensities recorded at four districts in 1961 are given in Table-2.
Related Content
Leaving no one behind: lessons from the kerala disasters, india: kerala floods dref n° mdrin020 emergency plan of action final report, kerala flood victims receive wash, shelter & livelihood support under athijeevana launch at bodhana, athijeevan: fighting back to attain stability after kerala flood.
Kerala Floods 2018: Impacts and Lessons Drawn
- Living reference work entry
- First Online: 27 October 2022
- Cite this living reference work entry
- Ajinder Walia 2 ,
- Pallvi Sharma 2 &
- Naima Nusrat 3
113 Accesses
2 Citations
Kerala, the southwest coastal state of India which ranks high on the Human Development Index, became vulnerable to severe flooding during the southwest monsoons of 2018. The state faced the worst floods in the century due to above-normal rainfall from June to August 2018. The above-normal rainfall was supplemented by a lack of integration of sustainable development practices and disaster risk management strategies. The floods affected all the districts of the state and led to the loss of over 400 precious lives along with extensive damage to infrastructure and property. It also triggered about 341 landslides in the area. The community including fishermen and women-centric organizations like Kudumbashree participated actively in responding to the disaster. Technology including WhatsApp, GIS, and crowdsourcing was used actively by the community during the search, rescue, and relief phase. The floods highlighted many constraints like lack of proper management and monitoring of critical natural resources such as water and land which left the state unprepared for major disasters caused by natural hazards. The disaster also highlighted crucial lessons to be adopted by other coastal states of the country which are expected to face increased flooding in the coming times due to climatic changes.
This is a preview of subscription content, log in via an institution to check access.
Access this chapter
Institutional subscriptions
Similar content being viewed by others
State Machinery, SDGs and Flood Control: A Case Study of Srinagar Floods (2014)
Contextualising the Increasing Risk of Floods and Tornadoes
Ajay, A. (2019). Role of technology in responding to disasters: Insights from the Great Deluge in Kerala. Current Science, 116 (6), 913.
Article Google Scholar
Anand, G. (2018, August 19). When help dropped from the skies: How IAF rescued 1000s from flood-ravaged Kerala. The Hindu . Retrieved December 1, 2021, from https://www.thehindu.com/news/national/kerala/when-help-dropped-from-the-skies-how-iaf-rescued-1000s-from-flood-ravaged-kerala/article24732423.ece
Anandan, S. (2018, August 28). Kerala floods: Kudumbashree Women Play Big Role in clean-up . The Hindu. Retrieved October 22, 2021, from https://www.thehindu.com/news/national/kerala/kudumbasree-collects-5-cr-for-flood-relief/article24801984.eceaspx
Azzali, S., Kamble, Z., Thirumaran, K., Wong, C., & Wood, J. (2021). Mitigating impact from natural disasters, building resilience in tourism: The case of Kerala. Economic Effects of Natural Disasters, 120 . https://doi.org/10.1016/b978-0-12-817465-4.00008-x
Chief Executive Officer. (2019). Kerala rebuild initiative . Rebuild Kerala Initiative-RKI. Retrieved August 29, 2021, from https://rebuild.kerala.gov.in/en/
Google Scholar
Committee, G. (2011). (rep.). Report of the Western Ghats ecology expert panel part I . Kerala State Biodiversity Board.
Duncombe, J. (2018). Making sense of landslide danger after Kerala’s floods. Economic and Political Weekly, 53 (34). https://doi.org/10.1029/2018eo108061 . e9-127m.pdf.
ENVIS Hub: Kerala state of environment and related issues . Physiography. (n.d.). Retrieved February 20, 2021., from http://www.kerenvis.nic.in/Database/ENVIRONMENT_824
Government of Kerala. (2018). Kerala post disaster needs assessment: Floods and landslides - August 2018 (October 2018) - India. Relief Web . Kerala: World Bank. Retrieved October 12, 2021, from https://reliefweb.int/report/India/kerala-post-disast er-needs-assessment-floods-and-landslides-august-2018-October-2018
Hydrological Studies Organization Hydrology (S) Directorate. (2018). Kerala floods of August 2018 . Central Water Commission. Retrieved December 23, 2021, from https://reliefweb.int/sites/reliefweb.int/files/resources/Rev-0.pdf
ICAR. (2018, September). Report on impact of rain induced natural calamity on spice crops in Kerala. ICAR-Indian Institute of Spices Research. Retrieved March 38, 2021, from http://spices.res.in/pages/report-impact-rain-induced-natural-calamity-spice-crops-kerala
IMD. (2018). Kerala floods 2018. Indian Meteorological Department, Ministry of Earth Sciences, Government of India. Retrieved September 16, 2021, from https://mausam.imd.gov.in/search_RV/index.php
IMF. (2021). Implied PPP conversion rate . Imf.org. IMF. Retrieved January 13, 2021, from https://www.imf.org/external/datamapper/pppex@weo/oemdc/advec/weoworld/da/ind?year=2018
Indian Navy. (2018). SNC initiates operation ‘Madad’ at Wayanad, Kerala. SNC Initiates Operation ‘Madad’ at Wayanad, Kerala | Indian Navy. Retrieved February 9, 2022, from https://www.indiannavy.nic.in/content/snc-initiates-operation-madad-wayanad-kerala
IPCC. (2021). Chapter 4: Sea level rise and implications for low-lying islands. IPCC. Retrieved September 16, 2022, from https://www.ipcc.ch/srocc/chapter/chapter-4-sea-level-rise-and-implications-for-low-lying-islands-coasts-and-communities/
Kerala State Disaster Management Authority. (2016). Kerala State Disaster Management Plan . KSDMA. Kerala: Department of Revenue and Disaster Management.
Kerala State Government. (2018). Report on the exceptionally heavy rainfall over Kerala during 1st – 19th Aug. 2018. Meteorological Centre, Thiruvananthapuram. Retrieved April 11, 2021, from https://sdma.kerala.gov.in/wp-content/uploads/2020/08/IMD-Monsoon-Extreme-Rainfall-2018.pdf
Kumar, R. (2018, October 12). Kerala floods: From the eyes of Wetlands . Wetlands International.
Ministry of Health and Family Welfare. (2017). State fact sheet Kerala. Rchiips.org . Mumbai: International Institute for Population Sciences. Retrieved December 29, 2021 from http://rchiips.org/nfhs/pdf/NFHS4/KL_FactSheet.pdf
Mohanti, M., & Sheikh, S. (2019, March 17). Kerala floods: What led to deluge that reminded people of the great flood of 1999 . News18. Cable News Network LP.
Narayanan, K. (2018, August 19). What caused the Kerala floods? Could we have done anything to prevent it? Technology News, Firstpost. Retrieved August 19, 2018, from https://www.firstpost.com/tech/news-analysis/what-caused-the-kerala-floods4993041.html#:~:text=Some%20reasons%20for%20its%20vulnerability
NDRF. (2018). Kerala floods - 2018: NDRF - National Disaster Response Force. National Disaster Response Force, Ministry of Home Affairs, Government of India. Retrieved September 16, 2022, from https://ndrf.gov.in/operations/kerala-floods-2018
Pr Cell. (2018, August 18). Kerala floods: NDRF scaled up its rescue & evacuation operation, 194 rescued & over 10,400 evacuated till now | NDRF - National Disaster Response Force. NDRF HQ, New Delhi. Retrieved September 28, 2020, from http://www.ndrf.gov.in/pressrelease/kerala-floods-ndrf-scaled-its-rescue-evacuation-operation-194-rescued-over-10400
RBI. (2020). Handbook of statistics on Indian states . Reserve Bank of India.
SBI. (2019). Human development index across Indian states: Is the glass still half empty? Ecowrap, 94 (FY19).: https://www.sbi.co.in/documents/13958/14472/Ecowrap_20190308.pdf
SDMA. (2021). Kerala Floods 2018. SDMA Kerala. Retrieved September 15, 2022, from https://sdma.kerala.gov.in/wp-content/uploads/2019/03/PDNA-report-FINAL-FEB-2019_compressed.pdf
Special Correspondent. (2018, August 20). Centre says Kerala floods ‘Calamity of severe nature’. The Hindu . https://www.thehindu.com/news/national/kerala-floods-declared-calamity-of-severenature/article24738072.ece
State Government of Kerala. (2019). Rebuild Kerala development program: A resilient recovery policy framework and action plan for shaping Kerala’s resilient, risk-informed development and recovery from 2018 floods . Rebuild.kerala.gov.in. Kerala: Government of Kerala. https://rebuild.kerala.gov.in/reports/RKDPMaster%2021May2019.pdf
State Level Bankers’ Committee Kerala. (2019). Slbckerala.com . Retrieved June 19, 2021, from http://slbckerala.com/images/Meetings/23826648-aa8a-4e5f-ac2c-059b418e71
State Relief Commissioner. (2018). Additional memorandum . Sdma.kerala.gov.in . Kerala: Disaster Management (Additional Chief Secretary) Government of Kerala. https://sdma.kerala.gov.in/wp-content/uploads/2019/08/Memorandum2-Floods-2018.pdf
United Nations Development Programme. (2018, December 12). Kerala post disaster needs assessment floods and landslides - August 2018 . Retrieved January 23, 2021 from https://www.undp.org/content/undp/en/home/librarypage/crisis-prevention-andrecovery/post-disaster-needs-assessment-kerala.html on June 2018.
Walia, A., & Nusrat, N. (2020). Kerala floods 2018 . National Institute of Disaster Management.
Welankar, P. (2018, August 19). Illegal Forest land acquisition major reason for floods in Kerala, says ecologist Madhav Gadgil, calls it man-made disaster. Hindustan Times . Hindustan Times Pvt. Ltd.
WRI INDIA (2018, September 03). Kerala Flooding: Natural Calamity or Manmade Disaster? Retrieved July 2021, from https://wri-india.org/blog/kerala-flooding-natural-calamity-ormanmadedisaster
Download references
Author information
Authors and affiliations.
National Institute of Disaster Management (NIDM), Ministry of Home Affairs, Government of India, New Delhi, India
Ajinder Walia & Pallvi Sharma
Red Orange Media and Communications, Dhaka, Bangladesh
Naima Nusrat
You can also search for this author in PubMed Google Scholar
Editor information
Editors and affiliations.
Centre for the Study of Law and Governance, Jawaharlal Nehru University, New Delhi, Delhi, India
Amita Singh
Section Editor information
Indian Administrative Service (Retired), Delhi, India
Nivedita Haran
Rights and permissions
Reprints and permissions
Copyright information
© 2022 Springer Nature Singapore Pte Ltd.
About this entry
Cite this entry.
Walia, A., Sharma, P., Nusrat, N. (2022). Kerala Floods 2018: Impacts and Lessons Drawn. In: Singh, A. (eds) International Handbook of Disaster Research. Springer, Singapore. https://doi.org/10.1007/978-981-16-8800-3_188-1
Download citation
DOI : https://doi.org/10.1007/978-981-16-8800-3_188-1
Received : 18 May 2022
Accepted : 30 July 2022
Published : 27 October 2022
Publisher Name : Springer, Singapore
Print ISBN : 978-981-16-8800-3
Online ISBN : 978-981-16-8800-3
eBook Packages : Springer Reference Business and Management Reference Module Humanities and Social Sciences Reference Module Business, Economics and Social Sciences
- Publish with us
Policies and ethics
- Find a journal
- Track your research
- DOI: 10.5194/HESS-2018-480
- Corpus ID: 134183448
The Kerala flood of 2018: combined impact of extreme rainfall and reservoir storage
- V. Mishra , Saran Aaadhar , +3 authors A. Tiwari
- Published 14 September 2018
- Environmental Science
- Hydrology and Earth System Sciences Discussions
96 Citations
Evaluating the 2018 extreme flood hazard events in kerala, india, hydroclimatological perspective of the kerala flood of 2018, the anomalous weather parameters that lead to the extreme rainfall of kerala in august 2018, the 2018 kerala floods: a climate change perspective, an investigation into the impact of reservoir management kerala floods 2018: a case study of the kakki reservoir.
- Highly Influenced
Modeling the concurrent impact of extreme rainfall and reservoir storage on Kerala floods 2018: a Copula approach
Catastrophic flood of august 2018, kerala, india: study of partitioning role of lineaments in modulating flood level using remote sensing data, sar based flood risk analysis: a case study kerala flood 2018, analytical study of kerala floods, a diagnostic study of extreme precipitation over kerala during august 2018, 25 references, on the frequency of the 2015 monsoon season drought in the indo‐gangetic plain, impact of climate change on extreme rainfall events and flood risk in india, observed and projected urban extreme rainfall events in india, the heavy precipitation event of december 2015 in chennai, india, rapidly assessing flood damage in uttarakhand, india, soil moisture droughts under the retrospective and projected climate in india., a threefold rise in widespread extreme rain events over central india, increased human and economic losses from river flooding with anthropogenic warming.
- Highly Influential
Prospect of application of extended range forecast in water resource management: a case study over the Mahanadi River basin
Human contribution to more-intense precipitation extremes, related papers.
Showing 1 through 3 of 0 Related Papers

IMAGES
VIDEO
COMMENTS
Situation Report in English on India about Food and Nutrition, Health, Flood, Land Slide and more; published on 1 Aug 2024 by HAI LOCAL Situation Report 010-2024 - Landslide in Kerala (August 01 ...
2024 Wayanad landslides were the multiple landslides that occurred at Punjirimattom, Mundakkai, Chooralmala, Attamala, Meppadi and Kunhome villages of Wayanad district, Kerala, India, in the early hours of 30 July 2024.Heavy rains triggered the collapse of hillsides, resulting in torrents of mud, water, and boulders cascading down onto the area.
Extreme weather events leading to more frequent rains, combined with Kerala's topography, have only exacerbated its problems, according to experts. In recent years, cyclones, severe flooding and ...
This year has seen a series of landslides around the world — from Yunnan in China in January to Papua New Guinea in May that killed more than 2,000 to Sulawesi island in Indonesia and Wayanad in Kerala in July. The death toll from the series of landslides in Meppadi in Wayanad district had reached ...
In a freewheeling chat, Dr V Ambili, deputy director general, Geological Survey of India, Kerala unit, Dr K G Thara, former head, Disaster Management Centre, Kerala, Dr Sajin Kumar K S assistant ...
Flooding is the most frequent phenomenon that leads to social and economic disruption worldwide. Effective flood management necessitates an understanding of the spatial distribution of flood-susceptible areas. Currently, the delineation of flood-susceptible areas and their effective management are a significant theme of flood research. However, in order to ensure sustainable flood management ...
One of Kerala's biggest natural disasters occurred in 2018, when heavy floods and landslides killed 500 people. The World Meteorological Organization listed it as one of five major extreme ...
Kerala's summers tend to be very wet, and these totals are standard for this time of year. ... The Ministry of Water Resources maintains a Level IV emergency response for flood prevention in 9 ...
Rainfall erosivity (RE), a pivotal external force driving soil erosion, is impacted by El Niño/Southern Oscillation (ENSO). Studying the spatiotemporal variations in RE and their response to ENSO is essential for regional ecological security. In this study, a daily RE model was identified as a calculation model through an evaluation of model suitability. Daily precipitation data from 1971 to ...
Kerala flood case study Kerala. Kerala is a state on the southwestern Malabar Coast of India. The state has the 13th largest population in India. Kerala, which lies in the tropical region, is mainly subject to the humid tropical wet climate experienced by most of Earth's rainforests.
1-day m aximum rainfall aver aged over the entire state in August 2018 had a return period of ab out 75 years. However, 2 and 3-day maximum rainfall had the return per iod of about 200 and 100 ...
Kerala Floods August 2018 Page 1 Kerala Flood of August 2018 1.0 Introduction Kerala State has an average annual precipitation of about 3000 mm. The rainfall in the State is controlled by the South-west and North-east monsoons. About 90% of the rainfall occurs during six monsoon months. The high intensity storms prevailing during the monsoon months
Abstract and Figures. A short span of abnormal precipitation in the year 2018 has ravaged the southwestern state of Kerala. It has resulted in widespread flooding and landslides with more than 400 ...
2003: Flood occurred on 24th June. Kerala received 1722.6 mm rainfall. Caused due to heavy rains and Landslides. This flood affected 11 out of 14 districts ... P. Srija et al A Case Study on Kerala Floods 29| International Journal of Current Engineering and Technology, Special Issue-9 (Aug 2021) Embankments are the oldest known forms of flood ...
KERALA FLOOD CASE STUDY - Free download as Powerpoint Presentation (.ppt / .pptx), PDF File (.pdf), Text File (.txt) or view presentation slides online. The Kerala flood of August 2018 was the worst flood the state had seen in nearly a century, killing over 483 people and displacing around a million. Extreme rainfall during the monsoon season caused widespread flooding that required a massive ...
A short span of abnormal precipitation in the year 2018 has ravaged the southwestern state of Kerala. It has resulted in widespread flooding and landslides with more than 400 casualties. The present study has implemented the SAR (Synthetic Aperture Radar)-based Sentinel 1C band satellite data. The researchers have analyzed the remotely sensed ...
The flood was very unprecedented and rainfall in hilly districts were three times more, and the State was also not prepared such mega scalefor , as it has not experienced a flood of this magnitude, except the recorded one in 1924. This flood can be as the called 'Flood of this Century', which hit Kerala continuously from 8th to 18th of August.
Kerala experienced an abnormally high rainfall from 1 June 2018 to 19 August 2018. This resulted in severe flooding in 13 out of 14 districts in the State. As per IMD data, Kerala received 2346.6 ...
The document provides a summary of the Kerala floods of August 2018. It notes that Kerala experienced abnormally high rainfall from June to August 2018, receiving 42% more rain than normal. This caused severe flooding in 13 of 14 districts. The document analyzes rainfall data, reservoir operations, and estimates runoff generated during the heavy rainfall events. It finds that the August 15-17 ...
As per the preliminary estimates, the Kerala flood caused the death of more than 440 people (Gulf News, 30th August 2018) and economic damage exceeding $3 billion (News18, 17 August 2018). Despite the state -wide extreme rainfall in Kerala in August 2018, potential causes (heavy rain and reservoir operations) of
For example, the state's literacy rate is 94%, compared to the national average of 73%. The country's average life expec-tancy at birth was 68.8 years in 2013 -2017, whereas Kerala at the same time had 75.2 year life expectancy (RBI, 2020, 15 -16). Among all the states, it has the highest life expectancy at birth.
Kerala Floods Case Study - Free download as Word Doc (.doc / .docx), PDF File (.pdf), Text File (.txt) or read online for free. The document summarizes the severe flooding that occurred in the Indian state of Kerala in 2018 due to unusually heavy monsoon rains. Over 483 people died and over 1 million people were left homeless. The Indian government launched a massive rescue and relief ...
Abstract. Extreme precipitation events and flooding that cause losses to human lives and infrastructure have increased under the warming climate. In August 2018, the state of Kerala (India) witnessed large-scale flooding, which affected millions of people and caused 400 or more deaths. Here, we examine the return period of extreme rainfall and the potential role of reservoirs in the recent ...
Beginning on August 15th 2018, severe floods were affected in Kerala due to unusually high rain fall during the monsoon season. It was the worst flooding in Kerala nearly a century. Almost 370 people died and 33000 peoples are rescued. According to Kerala Govt. one sixth of the total population of Kerala had been directly affected by flood.
life and property.The Central Water Commission, in its report on Kerala Floods 2018 states that "it can be seen that the 2-day and 3-day rainfall depths of 15-17, August 2018 rainfall in Pamba, Periyar and Bharathapuzha sub-basins are most comparable to the Devikulam storm o.
The document summarizes the 2018 Kerala floods in India. Heavy monsoon rains caused widespread flooding across the state of Kerala in August 2018, with rainfall over 164% of the average for that month. Over 410 people died in flooding up to 3-4.5 meters deep. Over 1 million people were displaced to emergency relief camps. Infrastructure like roads, bridges, and airports were damaged. The ...